AI Technology News: Trends, Innovations, and Implications
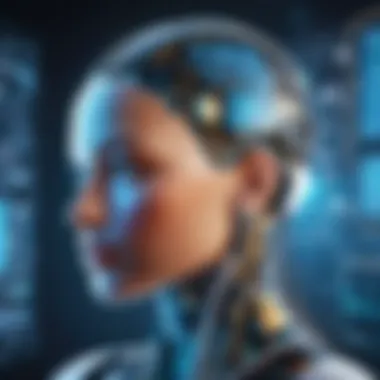
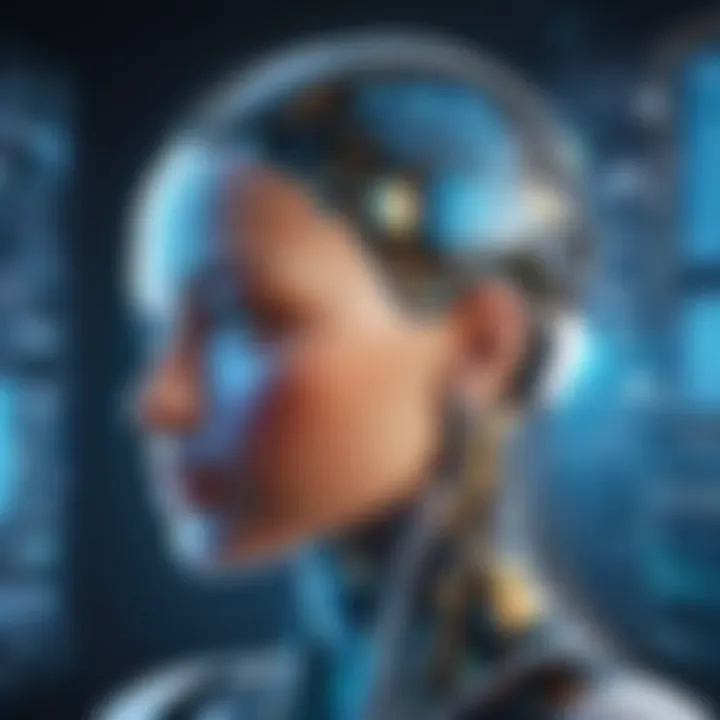
Intro
Artificial Intelligence (AI) continues to redefine numerous sectors, bringing along transformations that practitioners and enthusiasts alike are eager to understand. This exploration not only unveils the trends shaping the current landscape but also delves into the implications these innovations bear on our lives.
As AI technology evolves, it influences various fields including security, data management, and networking. These shifts are not just theoretical; they have practical ramifications for industries and individuals. This article serves as a comprehensive guide on the most current developments in AI, aimed at those who seek a deeper understanding of cutting-edge innovations and their broader impacts.
Understanding Storage, Security, or Networking Concepts
Prolusion to the Basics
In this context, understanding the foundational elements of storage, security, and networking is essential. Storage refers to methods and technologies that allow data to be stored, retrieved, and managed. Security ensures data integrity and confidentiality against unauthorized access. Networking relates to the systems that allow different hardware devices to communicate.
Key Terminology and Definitions
To communicate effectively within this field, it is important to grasp relevant terminology such as:
- Data Storage: Techniques for keeping digital information.
- Cybersecurity: Measures taken to protect systems from cyber threats.
- Networking Protocols: Rules that determine how data is transmitted over networks.
Overview of Important Concepts and Technologies
Each of these concepts interacts with AI technologies, particularly in improving efficiency and performance. For example, the advent of cloud storage solutions has revolutionized data storage. Similarly, AI-driven security systems are enhancing how organizations defend against threats.
Industry Trends and Updates
Latest Trends in Storage Technologies
Innovative storage solutions, like Solid State Drives (SSDs) and cloud-based systems, play a pivotal role. These technologies allow faster data access and improved reliability compared to traditional methods. As data continues to grow exponentially, scalable solutions become a necessity.
Cybersecurity Threats and Solutions
AI technologies are also critical in addressing cybersecurity threats. The rise of malware, phishing scams, and ransomware calls for adaptive learning and real-time response systems. AI algorithms can analyze patterns and respond more rapidly to potential threats than traditional methods.
Networking Innovations and Developments
In networking, significant advancements such as Software-Defined Networking (SDN) and network function virtualization (NFV) are altering operational frameworks. They enable more agile and flexible network management, essential in today’s rapidly changing technological environment.
Case Studies and Success Stories
Examining real-world applications highlights both successes and the lessons learned from failures.
Real-Life Examples of Successful Implementations
Organizations adopting cloud storage solutions have often reported enhanced operational efficiencies. For instance, businesses using Amazon Web Services (AWS) have benefited from scalable solutions tailored to their needs.
Cybersecurity Incidents and Lessons Learned
The 2020 SolarWinds cyberattack showcased vulnerabilities across sectors. Post-incident analysis illustrates the necessity for proactive security measures and the role AI can play in detecting anomalies earlier in the process.
Networking Case Studies Showcasing Effective Strategies
Companies utilizing SDN have reported improved performance and flexibility. This technology allows for real-time adjustments in response to network demands, demonstrating significant gains.
Reviews and Comparison of Tools and Products
In-Depth Reviews of Storage Software and Hardware
Various tools provide options for efficient storage solutions. Evaluating products such as Google Drive versus Dropbox can guide organizations to the most effective solution for their size and requirements.
Comparison of Cybersecurity Tools and Solutions
In cybersecurity, comparing solutions like CrowdStrike and McAfee can help professionals understand their strengths, weaknesses, and applicability to specific business needs.
Evaluation of Networking Equipment and Services
Selecting appropriate networking equipment is crucial for maintaining high-performance operations. Comparing Cisco routers with Juniper Networks can reveal best-fit technologies for specific organizational requirements.
"Emerging AI technologies are not just improving efficiency, but are also redefining our approach to security and networking." - AI Researcher
Intro to AI Technology News
Artificial Intelligence (AI) is no longer a futuristic concept. It is a present-day reality that influences a range of industries and fields. In this context, AI Technology News serves as a crucial source of information that helps stakeholders to stay updated on the rapid advancements in AI. This section emphasizes the significance of following AI developments, showcasing how these innovations alter business strategies and influence decision-making processes.
The landscape of AI is continuously evolving. Companies are adopting AI technologies to improve efficiency, enhance customer experiences, and gain competitive advantages. Therefore, being aware of current trends can provide substantial benefits. Organizations can leverage insights from recent news to make informed choices regarding technology investments and implementation strategies.
Moreover, understanding the nuances of AI advancements allows professionals to anticipate challenges and prepare for the implications of these technologies on their specific domains. As AI is increasingly integrated into various sectors such as finance, healthcare, and cybersecurity, its impact is broad and profound.
Specifically, the practical knowledge derived from AI Technology News can lead to:
- Strategic planning: Businesses can plan their strategies with the latest trends in mind, ensuring they remain relevant and efficient.
- Competitive intelligence: Keeping an eye on competitors’ use of AI helps organizations to innovate and improve their offerings.
- Risk management: Awareness of potential challenges posed by AI allows for proactive approaches, minimizing negative outcomes.
"Informed decisions today pave the way for sustainable growth tomorrow."
In summary, AI Technology News serves not only as a resource for catching up on the latest innovations but also as a vital tool for strategic insight in an increasingly tech-driven world. As the article unfolds, it will delve into the current state of AI technologies, market growth, key innovations, and their implications for various professional sectors.
Current State of AI Technologies
Artificial intelligence has evolved significantly in recent years, as it plays a crucial role in various sectors. Understanding the current state of AI technologies helps stakeholders stay updated about advancements and potential implications. These technologies not only enhance operational efficiency but also foster innovation across industries.
AI's significance lies in its ability to process vast amounts of data and derive insights that were previously unobtainable. This capability improves decision-making processes in real-time. It also allows businesses to tailor services to individual preferences, enhancing customer experience. However, AI also come with concerns regarding ethical implications, data privacy, and security which are vital for developers and users to consider.
Key Innovations in AI
Key innovations in AI have emerged from various domains, transforming how we interact with technology. One of the most notable breakthroughs is the development of deep learning, which enables machines to learn from complex data sets. This has significant applications in speech recognition, image classification, and even autonomous driving technology.
In recent times, innovations such as reinforcement learning have garnered attention. This approach allows AI systems to make decisions based on past experiences, optimizing their performance over time. Other advancements include natural language processing technologies which are now more reliable than ever, enhancing conversational interfaces and chatbots.
Here are some key innovations in AI that are shaping the current landscape:
- Generative Adversarial Networks (GANs): These enhance image generation tasks.
- Explainable AI (XAI): Focuses on transparency of AI decisions.
- Transfer Learning: Reduces the data needed for training models.
Market Growth and Trends
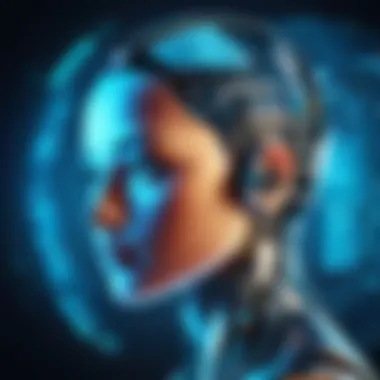
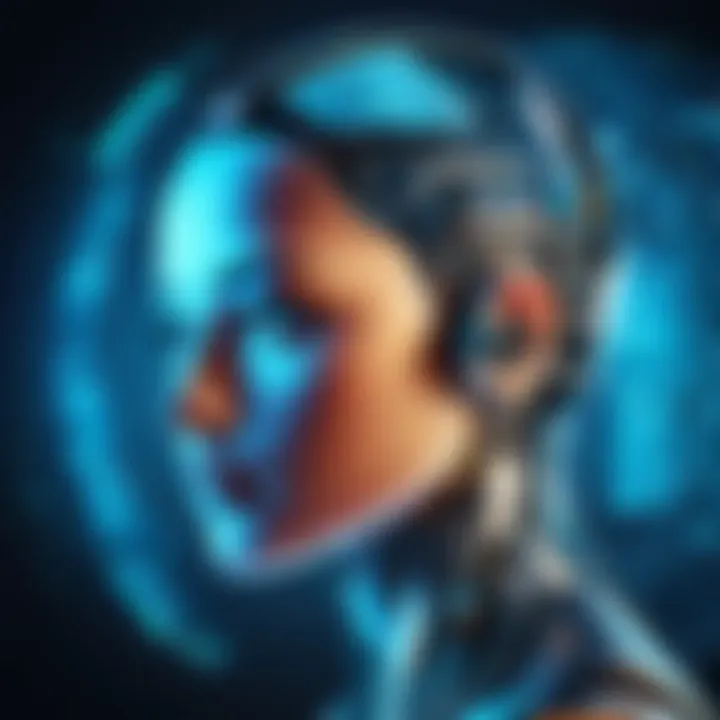
The market growth and trends in AI highlight its increasing significance in the global economy. Over recent years, investments in AI have surged, with forecasted growth continuing to rise. It is predicted that the AI sector will reach billions in revenue by the next decade.
Several trends define the current market landscape:
- Integration of AI across industries: From finance to healthcare, companies are adopting AI solutions to streamline operations.
- Increased focus on AI ethics: Firms are prioritizing ethical AI practices, acknowledging the responsibility that comes with leveraging powerful technologies.
- Collaboration between technology firms: Companies are striking partnerships to enhance their offerings and drive innovation.
This growing trend underscores the importance of keeping abreast of developments in AI technologies. Ignoring these advancements can lead to missed opportunities for business optimization and development.
"The AI sector is not just a technology trend; it represents a shift in how industries operate and compete in an increasingly data-driven world."
Understanding these elements is essential for IT professionals, cybersecurity experts, and students who seek to navigate this evolving landscape effectively.
AI in Cybersecurity
The realm of cybersecurity is rapidly evolving, and artificial intelligence plays a crucial role in this transformation. As data breaches and cyber threats grow in complexity, AI technologies offer solutions that enhance security measures and address potential vulnerabilities. By employing machine learning algorithms and predictive analytics, AI helps organizations to preemptively identify threats, respond with agility, and safeguard sensitive information. The integration of AI in cybersecurity is not just a trend; it is becoming a fundamental component of modern security protocols.
AI-Driven Threat Detection
AI-driven threat detection systems have revolutionized the way organizations monitor their networks. These systems utilize algorithms to analyze vast amounts of data in real time, identifying patterns that suggest abnormal activity. Here are some important advantages of AI-driven threat detection:
- Speed and Efficiency: AI algorithms can process information much faster than human analysts. This enables rapid detection of threats, reducing response times.
- Increased Accuracy: Traditional signature-based detection methods often lead to false positives. AI enhances accuracy by learning from past incidents and adapting to new types of threats.
- Behavioral Analysis: AI can establish baselines for normal user behavior, making it easier to detect anomalies that may indicate a security breach.
In a nutshell, AI-driven solutions replace outdated techniques, thus improving overall network security resilience.
Adversarial Machine Learning
Adversarial machine learning is an emerging field that highlights both the potential and the risks of AI technologies within cybersecurity. Essentially, it involves using machine learning techniques to craft deliberate attacks on AI models. Cybercriminals may exploit these weaknesses to mislead AI systems, thereby circumventing security measures.
Critical aspects to understand about adversarial machine learning include:
- Vulnerability: AI systems can be vulnerable to specific manipulation, which may result in misclassifying malicious activities as benign. This poses a serious risk to organizations relying on AI for cybersecurity.
- Evolving Threat Landscape: As adversaries become more sophisticated, organizations must continuously adapt their defenses. Regular testing and updating AI models are essential to counter new types of attacks.
- Defensive Strategies: Techniques such as adversarial training can help AI systems learn to recognize and resist potential attacks. Adopting robust models can mitigate risks associated with adversarial machine learning.
Maintaining a proactive approach towards these challenges ensures that AI remains a potent ally in the fight against cyber threats.
Impact of AI on Data Management
Artificial Intelligence (AI) is increasingly becoming a pivotal force in transforming data management practices. The integration of AI technologies streamlines processes, enhances accuracy, and drives efficiency within organizations. With vast amounts of data being generated daily, the ability to effectively manage this information is essential for informed decision-making. AI's role in data management cannot be understated, as it not only increases productivity but also reduces operational costs. Let’s explore two key aspects: automation of data processing and security concerns regarding data privacy.
Automation of Data Processing
Automation is a significant benefit brought by AI. Manually handling data can be labor-intensive and time-consuming, leading to potential human error. AI-powered tools can automate repetitive tasks such as data entry, cleansing, and reporting. For instance, platforms like IBM Watson and Microsoft Azure use machine learning to analyze data on a scale that human analysts cannot achieve.
Some specific advantages of automating data processing include:
- Increased Efficiency: AI tools can process large datasets quickly, freeing up human resources for more complex analytical tasks.
- Enhanced Accuracy: Automation reduces the risk of errors inherent in manual data manipulation. AI algorithms can identify anomalies and inconsistencies in data more effectively.
- Scalability: As an organization grows, the ability to handle increasing data volumes becomes crucial. Automated systems can easily scale to meet demand without compromising performance.
Implementing AI for data processing at scale allows companies to derive insights faster. Organizations can make agile business decisions that are data-driven. This agility becomes a competitive advantage in fast-paced industries.
Data Security and Privacy Concerns
With greater automation comes the responsibility of ensuring data security and privacy. The collection and processing of personal and sensitive data raise significant ethical questions. AI systems can leverage vast amounts of data, yet this can create vulnerabilities if not managed correctly. Business must pay close attention to compliance with regulations like GDPR or CCPA, which set strict guidelines for data protection.
Key concerns related to data security in AI data management include:
- Data Breaches: As AI systems become more integrated into business operations, they can become attractive targets for cybercriminals. Organizations need robust security protocols to protect AI models and the data they use.
- Informed Consent: Companies must ensure that users are aware of how their data is used and provide informed consent. Automation can hasten data collection processes, but ethical considerations must never be overlooked.
- Bias and Misuse: AI systems learn from the data they are provided. If the data contains biases, the AI may produce biased outcomes. This can have serious implications in fields such as healthcare and law enforcement.
The interplay between automated data processing and security concerns necessitates a balanced approach. On one hand, organizations reap the benefits of enhanced efficiency and insight; on the other, they must commit to ethical practices that prioritize data security and user privacy.
"AI technologies hold the potential to revolutionize data management, yet they come with a pressing responsibility to protect user information."
Ethical Considerations in AI Development
As artificial intelligence (AI) technology continues to evolve, it raises numerous ethical concerns that cannot be ignored. Ethical considerations in AI development are crucial to ensure that the technologies we create serve humanity positively and mitigate potential risks. The implications of these considerations are broad, affecting everything from algorithms and data processing to societal norms and legal frameworks.
Ethical AI development emphasizes fairness, accountability, and transparency. Without these principles, AI systems risk perpetuating biases and causing harm to individuals and communities. Therefore, it is paramount that developers, organizations, and policymakers collaborate on guidelines that foster ethical practices.
Bias in AI Algorithms
Bias in AI algorithms is a significant concern for many industries. It often stems from the data used to train these algorithms. If the training dataset is unbalanced or does not represent diverse populations accurately, the AI system can make unfair or discriminatory decisions. For example, biased algorithms in hiring processes can lead to underrepresentation of certain groups in the workforce, exacerbating existing inequalities.
Addressing this bias requires a commitment to diverse data collection and continuous monitoring of AI systems after implementation. Developers need to evaluate their algorithms against a variety of metrics and seek inputs from a diverse group of stakeholders to uncover biases. Failure to do so can lead to reputational damage and legal consequences for companies.
"Understanding and mitigating bias in AI algorithms is not just a technical issue; it is a societal imperative."
Transparency and Accountability
Transparency and accountability in AI development are essential for building trust among users and stakeholders. When AI systems operate as a 'black box', users may find it challenging to understand how decisions are made. This lack of transparency can hinder the ability to identify and correct errors or biases within systems. In certain scenarios, such as criminal justice or health care, opaque decision-making can lead to severe consequences, making it vital that developers and organizations adopt clear accountability measures.
Incorporating transparency increases usability and ensures that stakeholders feel confident in using AI technologies. Developers should implement explainable AI techniques, allowing users to comprehend the reasoning behind automated decisions. This could include clear documentation and visual tools that illustrate how algorithms function.
Ultimately, addressing transparency and accountability in AI not only aids users in understanding decisions but also enforces a sense of responsibility among developers to create reliable, just AI systems.
Recent AI Acquisitions and Partnerships
In recent years, the landscape of artificial intelligence has been rapidly evolving. Acquisitions and partnerships have become commonplace in the tech industry as companies strive to enhance their AI capabilities. Understanding these dynamics is crucial for stakeholders looking to navigate the growing complexities of the market.
Strategic Collaborations
Strategic collaborations between firms in the AI sector and those from other industries have proven to be advantageous. These partnerships allow companies to leverage each other's strengths, thus accelerating development and deployment of AI solutions.
For example, technology giants like Microsoft have partnered with various startups to refine their AI models and improve cloud-based services. Collaborations enable sharing of resources, knowledge, and innovations, fostering a more competitive ecosystem. This synergy often leads to enhanced products that can adapt to market needs more effectively. Thus, the interconnectedness formed by such partnerships is essential for rapid technological advancement.
Additionally, through these collaborations, businesses can tap into new customer bases and expand market reach. Engaging in strategic partnerships can also lead to shared research and development costs, which can be particularly beneficial for smaller companies that may lack significant funding.
Impact on Innovation Capacity
The impact of acquisitions and partnerships on innovation capacity cannot be understated. When established companies acquire smaller tech firms, they are not just buying technology; they are absorbing talent and new ideas that can be pivotal in driving future innovations. These acquisitions can significantly enhance a company's research and development capabilities.
- Increased Resources: Larger companies generally have more financial resources, which can be redirected towards innovative initiatives.
- Talent Acquisition: The talent pool grows as employees from acquired companies introduce fresh perspectives and skills.
- Faster Time to Market: Merging teams and ideas can speed up the development process, allowing firms to launch AI products more quickly.
"The absorbed innovation and talent from acquired firms often become a catalyst for new product development and market strategies."
However, it is also vital to be mindful of potential integration challenges post-acquisition. Ensuring that the cultures of both companies can blend efficiently is often critical for long-term success. If not managed properly, it can lead to disruptions that negate many of the benefits sought through the acquisition.
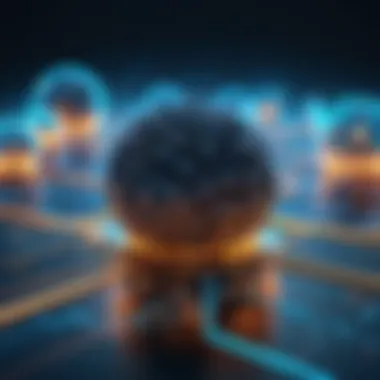
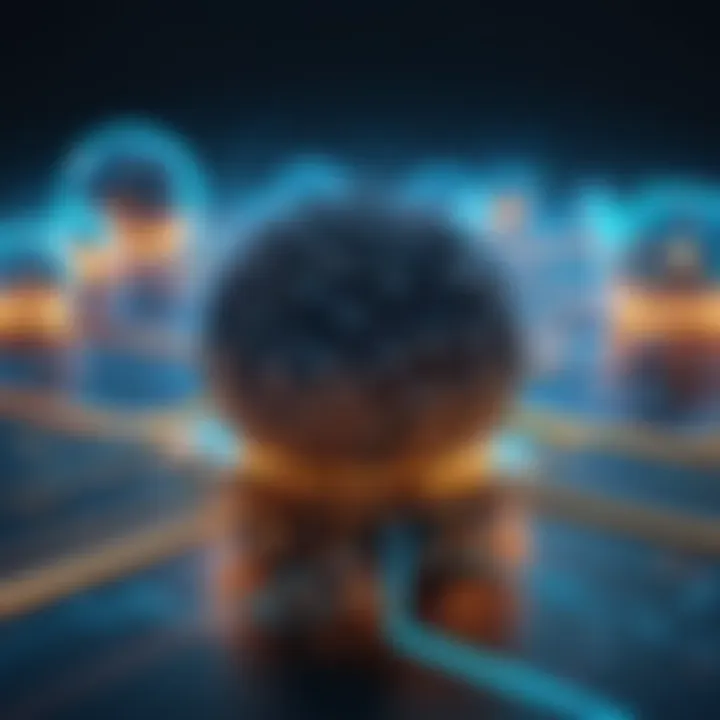
In summary, recent AI acquisitions and partnerships, characterized by strategic collaborations and enhanced innovation capacity, present significant opportunities and challenges. Understanding these elements can guide stakeholders as they navigate a landscape increasingly defined by rapid change and competitive pressures.
Machine Learning Breakthroughs
Machine learning is currently a key focus in the field of artificial intelligence. Recent breakthroughs are crucial as they redefine what technology can achieve, especially in areas that demand intricate pattern recognition and decision-making processes. These advancements not only enhance efficiency but also open up new avenues for innovation in various sectors. Understanding these breakthroughs helps IT professionals and businesses adapt to a rapidly changing technology landscape.
Advancements in Neural Networks
Neural networks have experienced significant upgrades. The introduction of deep learning has transformed many applications, leading to more accurate predictions and improved overall performance. New architectures such as convolutional neural networks (CNNs) and recurrent neural networks (RNNs) are being leveraged for diverse tasks.
For example:
- Convolutional Neural Networks: These are extensively applied in image recognition and computer vision. Their ability to process data through multiple layers enables them to recognize complex patterns better than traditional methods.
- Recurrent Neural Networks: RNNs are particularly useful in processing sequential data like time series or natural language. Their memory capabilities allow them to retain information from previous inputs, enhancing their predictive quality.
These advancements lead to a improved versatility in applications ranging from healthcare diagnostics to financial forecasting.
Real-World Applications
The real-world applications of machine learning breakthroughs are vast and varied. In the automotive industry, companies like Tesla utilize deep learning for their self-driving technology. This reliance on advanced neural networks facilitates real-time decision-making, significantly increasing safety and efficiency on the roads.
Moreover, in healthcare, machine learning models are being applied to diagnose diseases at earlier stages than ever before. Algorithms analyze medical images with precision that rivals human specialists, which can lead to faster treatments and improved outcomes.
Another notable application is in finance, where machine learning aids in detecting fraudulent transactions by identifying unusual patterns that humans might overlook.
Examples of real-world applications include:
- Predictive analytics for consumer behavior in marketing
- Risk assessment in lending processes
- Energy management and forecasting in utilities sector
Advancements in machine learning hold the key to future innovations. They promise not just improved outcomes but also enhanced capabilities across sectors.
"Machine learning is a powerful tool that, when properly harnessed, can lead to unprecedented advancements in technology and services."
In summary, breakthroughs in machine learning, particularly in advancements of neural networks and their applications, represent both progress and potential. As these technologies continue to evolve, they await further exploration in professional fields and everyday life.
The Role of AI in Cloud Computing
Artificial intelligence (AI) is not solely a product of cutting-edge technology; rather, it presents synergistic advances when integrated with cloud computing. By incorporating machine learning and intelligent analytics, cloud platforms become more efficient and capable, enabling organizations to extract significant insights from big data. This intersection holds great promise for enterprises looking to enhance operational efficiency, reduce costs, and foster innovation.
Optimization Techniques
AI plays a vital role in optimizing cloud resources. Through algorithms that predict usage patterns, businesses can allocate their resources more efficiently. This not only helps in maximizing uptime but also contributes to cost savings. By implementing these predictive models, organizations can avoid over-provisioning, which leads to wasted resources.
- Load Balancing: AI algorithms can dynamically balance workloads across servers, ensuring that no single server becomes a bottleneck. This improves application performance.
- Cost Management: AI can analyze spending patterns and suggest budget adjustments, granting businesses insights into their cloud expenses.
Furthermore, automation techniques powered by AI can streamline cloud operations. This covers everything from deploying infrastructure to managing updates and scalability. Organizations can focus on strategic initiatives as routine tasks are handled with minimal human intervention.
AI as a Service
The emergence of AI as a Service (AIaaS) is transforming how businesses leverage artificial intelligence without the need for extensive in-house resources or expertise. AIaaS enables organizations to access machine learning models, data analytics tools, and cognitive services via cloud platforms.
- Accessibility: Small to medium-sized enterprises can utilize cutting-edge AI tools without significant upfront investment, leveling the playing field.
- Flexibility: Companies can experiment with various AI projects without the commitment of long-term procurement. This encourages innovation and agility in operation.
AIaaS empowers organizations to apply machine learning solutions tailored to their specific needs, enhancing expertise in various domains. This democratization of powerful AI tools facilitates broad adoption across different industries.
The integration of AI with cloud computing not only transforms business operations, but also redefines how data is managed and utilized in a tech-driven world.
In summary, the intersection of AI and cloud computing is redefining operational landscapes. Businesses need to consider these advancements carefully as they look to harness the combined power of these technologies.
AI Technologies Changing Networking
The integration of artificial intelligence in networking is redefining how data is managed, transmitted, and secured. AI technologies are enhancing networking capabilities, presenting opportunities for optimization and growth. The importance of this topic lies in increasing efficiency, enhancing security, and reducing human error, which are essential for any organization relying on robust network infrastructure.
AI-driven solutions are now central to managing complex networks. The ability of AI to analyze vast quantities of data in real-time leads to more intelligent decision-making. As AI technologies evolve, they facilitate networks that are not merely reactive, but predictive and self-healing. This brings significant benefits when managing expansive networks, such as those in enterprises or cloud services.
Additionally, the rise of remote work and IoT devices amplifies the importance of AI in networking. These technologies help in maintaining optimal performance and security against emerging threats. There are several key aspects of how AI is changing networking:
- Enhanced Security: AI can detect anomalies much faster than traditional methods, providing strong defenses against cyber threats.
- Network Optimization: AI algorithms can optimize resource allocation, improving bandwidth usage and minimizing latency.
- Reduced Downtime: Automated network management reduces human intervention, which often leads to errors. By predicting potential faults, systems can preemptively address issues before they impact users.
AI technologies are key to transforming networking into an agile, responsive, and highly secure domain, crucial for modern organizational operations.
Intelligent Network Management
Intelligent network management involves utilizing AI to manage and optimize network operations. This encompasses monitoring, troubleshooting, and enhancing overall performance. Traditional network management methods often struggle with the complexities of modern networks. AI simplifies this by automating routine tasks.
Some features of intelligent network management include:
- Automated Monitoring: Continuous tracking of network performance allows for immediate adjustments as needed.
- Predictive Maintenance: Predicting when equipment may fail helps organizations to take action proactively, which can significantly reduce costs and risks.
- AI Insights: Utilizing machine learning, networks become better over time, adapting to the unique traffic patterns and user behaviors.
As networks grow in complexity, the necessity for intelligent management becomes clearer. Transitioning to intelligent network management improves reliability, and this efficiency is critical for any business that relies heavily on connectivity.
Predictive Analytics for Network Performance
Predictive analytics in network performance involves using AI algorithms to estimate future outcomes based on historical data. This technique can identify patterns and trends that might not be visible through traditional data assessment methods. The benefits of predictive analytics in networking include:
- Improved Resource Allocation: By anticipating high demand periods, organizations can allocate resources more effectively.
- Enhanced User Experience: Predictive analytics help ensure that network conditions meet user needs and expectations.
- Risk Management: Identifying potential issues before they materialize helps maintain optimal performance and mitigates risks.
Organizations can leverage predictive analytics tools to create a proactive approach toward network management. This transformation, driven by AI, equips businesses with the capability to respond swiftly to any challenges that may arise, maintaining seamless connectivity.
Global Trends in AI Regulation
Understanding the global trends in AI regulation is essential for stakeholders navigating the rapidly evolving landscape of artificial intelligence. The importance of this topic is underscored by the increasing integration of AI technologies across various sectors, from healthcare to finance and cybersecurity. As these technologies develop, regulatory frameworks must adapt to address ethical concerns, privacy issues, and the societal impact of AI.
AI is transforming industries, and with great power comes great responsibility. The lack of a cohesive set of guidelines can lead to misuse and ethical dilemmas. Therefore, governments and organizations across the world are recognizing the need for structured governance and regulatory frameworks.
Emergence of AI Governance Frameworks
AI governance frameworks are emerging as a response to the challenges posed by AI technologies. These frameworks aim to guide the development and implementation of AI in a way that prioritizes ethics and accountability. Countries are at different stages of developing these frameworks. Some countries have already established national AI strategies that emphasize transparency, fairness, and accountability.
For instance, Canada’s Directive on Automated Decision Making aims to ensure that the use of AI in government processes is fair and transparent. Similarly, the European Union's proposed AI regulation focuses on establishing a risk-based approach to AI applications. These frameworks typically include:
- Ethical Guidelines: Establishing principles that guide AI development.
- Risk Assessment Protocols: Identifying potential risks associated with AI technologies.
- Compliance Standards: Outlining the legal obligations for organizations utilizing AI.
The emergence of frameworks is a step forward, but there is also a significant gap between guidelines and actual implementation.
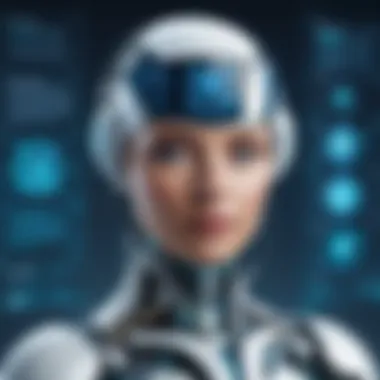
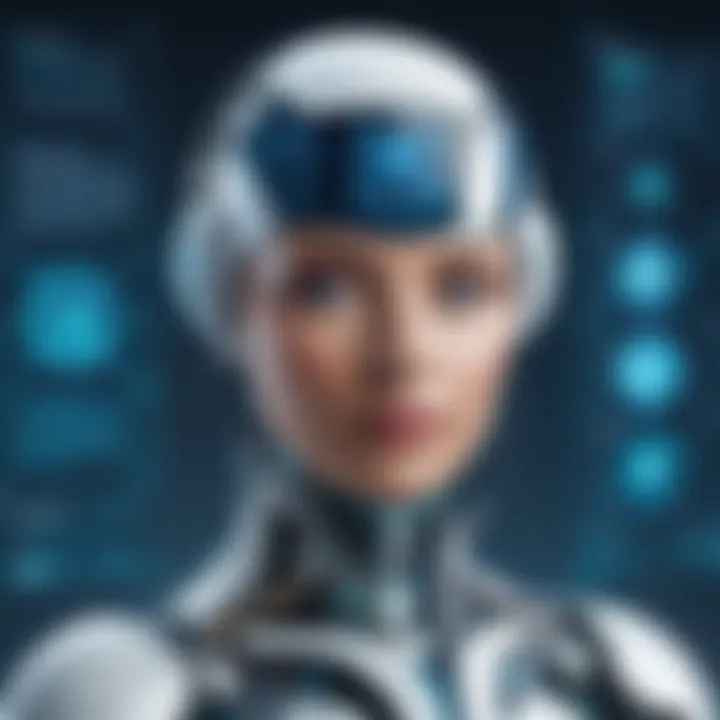
Compliance Challenges for Businesses
Businesses face multiple compliance challenges when incorporating AI technologies under newly established regulatory frameworks. Understanding these challenges is crucial for effective implementation. Some of the common hurdles include:
- Unclear Regulatory Requirements: Companies may struggle to understand the specific regulations that apply to their AI solutions.
- Rapidly Changing Regulations: The regulatory landscape is continually evolving, making it hard for organizations to stay compliant.
- Cost of Compliance: Adapting to new regulations may require substantial investment in resources and training.
"To ensure compliance, organizations must invest not only in technology but also in understanding the legal environment surrounding AI."
Organizations are asked to prioritize ethical considerations in their deployment of AI, but the ambiguity often leads to confusion and resistance. Thus, aligning business strategies with regulatory expectations is a complex but necessary task. Companies must develop comprehensive compliance programs that include regular training, risk assessments, and continuous monitoring to mitigate potential challenges.
Future Directions in AI Research
Research in artificial intelligence is continuously evolving. Understanding its future directions is crucial. Emerging technologies and methodologies can reshape industries. This section discusses significant trends and hurdles we must consider moving forward.
Trends Influencing Future Development
Many trends are appearing, driving the research landscape of AI. Here are some notable ones:
- Increased Focus on Explainability: As AI systems become more integral, explaining their decisions becomes essential. Users want to understand how AI makes choices. This demand encourages the development of transparent algorithms.
- Edge Computing Adoption: AI is moving from centralized data centers to edge devices. This shift allows real-time data processing. Faster decisions will be crucial in fields like autonomous driving and IoT applications.
- Integration with Other Technologies: AI will increasingly work alongside blockchain, IoT, and quantum computing. These integrations can create more robust systems. They also enhance security and data handling capabilities.
- Natural Language Processing (NLP) Advancements: NLP technologies are maturing, leading to better machine understanding of human languages. This will impact chatbots, virtual assistants, and other interactive systems.
By tracking these trends, professionals can align their efforts with the industry's direction, ensuring relevancy and fostering innovation.
Potential Technological Hurdles
While there is exciting potential, challenges remain. Understanding these hurdles helps stakeholders prepare and adapt.
- Data Privacy Issues: As AI systems rely on vast datasets, privacy concerns are paramount. Regulatory frameworks are developing, but compliance remains complex and challenging.
- Scalability Challenges: Many AI solutions are not easily scalable. This limits organization’s ability to deploy AI widely across their operations. Finding solutions that work at scale is crucial.
- Skills Gap: There is a talent shortage in AI. Finding qualified individuals to lead projects is increasingly difficult. Educational institutions must adapt programs to meet industry demand.
- Ethical Dilemmas: As AI continues to advance, ethical implications must be addressed. Questions surrounding bias in algorithms and the impact on jobs are significant. Organizations must focus on creating ethical frameworks.
"Understanding the future of AI research is not just for tech enthusiasts, but essential for organizations aiming to stay competitive."
Navigating these hurdles is essential for successful advancements. Those curious about AI's future need to stay informed and proactive.
AI in Healthcare
Artificial Intelligence in healthcare is crucial for enhancing patient outcomes and streamlining medical processes. With vast amounts of data generated daily, AI has the potential to transform these data into actionable insights. The integration of AI technologies can lead to more precise diagnoses, targeted treatments, and enhanced patient experiences. Moreover, it can reduce operational inefficiencies and lower costs, ultimately benefiting healthcare providers and patients alike.
Applications in Patient Care
AI applications in patient care are extensive and varied. Here are key areas where AI is making a significant impact:
- Diagnostic Tools: AI algorithms are capable of analyzing medical images with high accuracy. For example, systems like IBM Watson Health utilize deep learning to identify patterns in mammograms and X-rays, assisting radiologists in detecting conditions such as cancer earlier.
- Predictive Analytics: AI can analyze patient data to predict health outcomes. Predictive models help in identifying at-risk patients and implementing proactive care strategies. This is particularly vital in managing chronic diseases like diabetes and heart disease.
- Personalized Medicine: AI helps in tailoring treatment plans based on individual patient profiles. It can analyze genetic information to predict how a patient will respond to certain medications. This personalized approach increases the effectiveness of treatments and minimizes side effects.
- Virtual Health Assistants: Many healthcare providers have adopted AI-driven chatbots to handle patient inquiries and provide support. This not only streamlines communication but also helps in triaging patients based on their symptoms before they see a doctor.
Overall, AI applications are reshaping the patient care landscape, ultimately leading to improved healthcare delivery.
Ethical Dilemmas in Healthcare AI
Despite the promise of AI in healthcare, several ethical dilemmas arise, which healthcare stakeholders must navigate:
- Bias in Algorithms: AI systems may unintentionally reflect biases present in the training data. This can result in unequal care for diverse populations, exacerbating existing health disparities.
- Data Privacy Concerns: The use of AI relies heavily on patient data. Ensuring privacy and security of sensitive health information is paramount. Patients often worry about how their data is used and who has access to it.
- Accountability in Decision-Making: As AI takes a larger role in making clinical decisions, questions arise regarding accountability. If an AI system makes an error, who is responsible—the developers, healthcare providers, or the institution?
- Regulatory Compliance: Healthcare providers must navigate complex regulatory environments when using AI tools. Compliance with guidelines and standards is essential to safeguard patient welfare and promote transparency.
"AI in healthcare promises significant improvements but it must be balanced with ethical considerations to ensure fair and responsible use."
As AI technologies continue to develop, addressing these ethical dilemmas will be essential for building trust and ensuring that AI enhances rather than undermines patient care.
Ending and Implications for Stakeholders
The conclusion section provides a necessary summary and critical interpretation of the insights discussed in this article. It serves as a synthesis of the core themes presented around artificial intelligence technology developments, ensuring clarity on how these advancements affect various stakeholders.
In this evolving technological landscape, understanding AI's capabilities and limitations is crucial for decision-makers across industries. It allows organizations to align their strategies and leverage AI tools to boost productivity and enhance decision-making processes. Companies are recognizing the urgent need to integrate AI into their operations, as it provides competitive advantages. This integration can lead to operational efficiencies, cost reductions, and innovations in service delivery.
**Benefits associated with engaging with AI technology:
- Increased efficiency through automation
- Better data-driven decision making
- Enhanced customer insights and targeting
- Improved risk management and compliance**
Stakeholders need to consider various implications while adopting AI. On one hand, organizations must find a balance between leveraging AI's potential and ensuring ethical compliance as outlined in previous sections. Furthermore, awareness of biases that may arise from AI algorithms is essential for protecting brand integrity and maintaining trust with customers.
Moreover, the global regulatory landscape is changing rapidly as governments establish guidelines for AI usage and data privacy. Businesses must stay abreast of these regulations to avoid potential compliance issues, which can hinder growth and innovation.
Thus, the conclusion emphasizes the significance of strategic oversight in AI implementation. Stakeholders who prioritize continuous learning and adaptability will likely position themselves advantageously in this competitive market.
Strategic Insights for Businesses
Businesses must seek to understand the transformative potential of AI and the various ways it can reshape operations. Key insights include:
- Investing in Training: Companies should invest in training their workforce to navigate and implement AI technologies effectively. Investing in employee education mitigates resistance to change and fosters a culture of innovation.
- Data Governance: Establish solid data governance frameworks that address privacy concerns and ethical implications while capitalizing on data analytics.
- Collaboration Across Sectors: Engagement with AI research communities and startups allows businesses to stay ahead of trends and foster innovation.
Opportunities for Continuous Learning
Continuous learning is essential for adapting to the rapid pace of AI advancements. Stakeholders should pursue various avenues for professional development:
- Industry Reports: Regularly reviewing reports from organizations such as Gartner or McKinsey provides insights into market trends and benchmarking.
- Webinars and Podcasts: Participating in educational webinars and listening to expert podcasts keep stakeholders informed about the latest insights and methodologies in AI.
- Online Courses: Platforms like Coursera and edX offer courses designed to enhance skills in AI and machine learning for all levels of expertise.
"The need for continuous learning in AI is not just an option anymore, it is a necessity for forward-thinking professionals."
By actively engaging in these learning opportunities, stakeholders can remain relevant and effectively respond to the changing demands of their industries.
Resources for Further Exploration
In the field of artificial intelligence, staying informed is crucial. The nature of AI technology is continually evolving, and professionals must stay updated on the latest developments, trends, and regulations. This section provides resources that will help IT professionals, cybersecurity experts, and students to enhance their knowledge and skills.
Industry Reports on AI
Industry reports on AI serve as invaluable resources, providing insights into market trends, innovations, and forecasts. These reports often include comprehensive data analyses, case studies, and expert commentary, making them essential for decision-makers and strategists. Some key elements to consider include:
- Market Analysis: Understand the competitive landscape and identify key players in the AI domain.
- Emerging Trends: Reports can reveal upcoming trends that may impact sectors such as finance, healthcare, and manufacturing.
- Benchmarking: Utilize reports to compare your organization’s performance against industry standards.
Organizations like McKinsey, Deloitte, and Gartner release annual reports that are quite insightful. Additionally, sites like Wikipedia and Britannica can provide historical context and foundational knowledge about AI.
Educational Platforms and Courses
Professional development in AI is fundamentally supported by modern educational platforms. Available courses cover a range of topics from machine learning basics to deep learning applications. Key platforms include:
- Coursera: Provides a variety of courses from top universities and companies on AI and machine learning.
- edX: Offers both free and paid courses, including MicroMasters programs in AI-related fields.
- Udacity: Focuses on nano-degree programs specifically in AI, data science, and programming.
These platforms not only provide content but also interactive assignments, quizzes, and community forums to enhance the learning experience. Engaging in these courses can foster a deeper understanding of complex AI concepts, equipping professionals with the skills they need in this competitive landscape.
"AI is not just a fad; it is a fundamental shift in how we will work and live in the future."
In summary, leveraging both industry reports and educational platforms can play a critical role in navigating the complexities of AI technology. Utilization of these resources will enable professionals to make informed decisions, advance their careers, and contribute to the efficacy of their organizations.