The Role of Artificial Intelligence in Transforming Diagnostic Imaging Practices
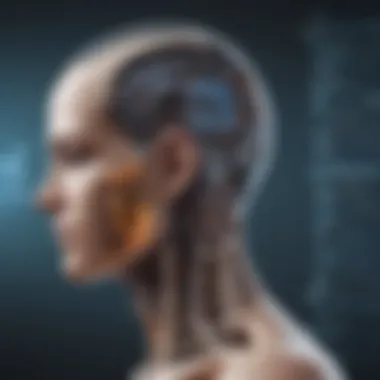
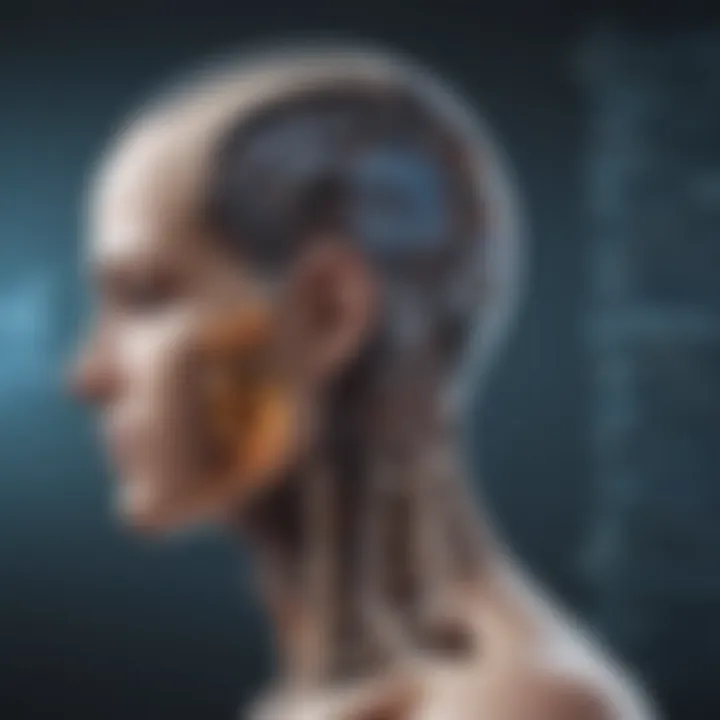
Understanding the Role of Artificial Intelligence in Diagnostic Imaging
The integration of artificial intelligence (AI) in diagnostic imaging represents a significant advancement in the medical field. AI technology holds the potential to enhance accuracy in diagnostics and streamline workflow efficiencies. Through the utilization of AI algorithms and machine learning techniques, medical professionals can access valuable insights that aid in making informed decisions and improving patient outcomes. This section will delve into the fundamental concepts of AI in diagnostic imaging, providing a detailed overview of its applications and benefits.
Key Terminology and Definitions
To comprehend the nuances of AI in diagnostic imaging, it is imperative to familiarize oneself with key terminology and definitions prevalent in this domain. Terms such as machine learning, deep learning, neural networks, and algorithmic models form the crux of AI applications in medical imaging. Understanding these terms is crucial for grasping the functioning of AI systems and their implications in diagnostic processes. By exploring these concepts, professionals can navigate the complexities of AI-powered diagnostic tools with proficiency.
Applications and Benefits of AI in Diagnostic Imaging
The realm of diagnostic imaging witnesses a transformation with the incorporation of AI technologies. AI facilitates image analysis, interpretation, and pattern recognition with unprecedented speed and accuracy. By automating routine tasks and reducing human error, AI minimizes the margin of diagnostic discrepancies, leading to improved patient care. Moreover, AI algorithms enable predictive analytics, aiding in the early detection of diseases and the formulation of personalized treatment plans. Medical professionals leverage AI to optimize resources, mitigate operational challenges, and enhance the overall efficiency of diagnostic imaging processes.
Challenges and Future Considerations
Despite the remarkable potential of AI in diagnostic imaging, several challenges confront its widespread adoption. Issues related to data privacy, regulatory compliance, algorithm bias, and integration complexities pose significant hurdles in harnessing the full capabilities of AI technologies. Moreover, the evolving nature of AI necessitates regular updates and continuous training to ensure optimal performance. This section will address these challenges while exploring future considerations for advancing AI integration in diagnostic imaging practices.
Synthesizing the Impact of AI on Diagnostic Imaging
Introduction to Artificial Intelligence in Diagnostic Imaging
Artificial Intelligence (AI) represents a pivotal component in revolutionizing the landscape of diagnostic imaging. Its integration with medical imaging entails a profound impact, not only in enhancing diagnostic accuracy but also in streamlining workflow efficiencies. Within this article, the significance of AI in diagnostic imaging will be thoroughly explored, shedding light on the transformative potential it holds.
Definition and Significance
Understanding AI in Healthcare:
Understanding AI in healthcare encompasses the utilization of advanced algorithms to interpret complex medical data and aid in decision-making processes. This subset of AI plays a crucial role in improving treatment outcomes and operational efficiency within healthcare settings. Its ability to analyze vast amounts of patient data swiftly and accurately makes it a valuable tool in enhancing diagnostic capabilities and treatment planning, which aligns perfectly with the overarching theme of this article.
Relevance of AI in Diagnostic Imaging:
AI's relevance in diagnostic imaging is profound, as it offers innovative solutions to longstanding challenges in traditional radiology practices. By leveraging AI technologies, diagnostic imaging processes can be augmented, leading to increased precision and reduced turnaround times. The incorporation of AI in this context represents a pioneering effort to enhance patient care and streamline healthcare operations. However, it also introduces considerations such as data security and ethical implications that warrant careful examination, reflecting the intricacies of this dynamic intersection.
Historical Context
Evolution of AI in Medical Imaging:
The evolution of AI in medical imaging traces back to the emergence of image analysis techniques and machine learning algorithms in the healthcare domain. This progression signifies a paradigm shift in diagnostic practices, transitioning from manual interpretation to automated analysis with AI assistance. The adoption of AI-driven tools in medical imaging has significantly improved diagnostic accuracy and paved the way for more personalized treatment approaches, setting the stage for in-depth discussions within this article.
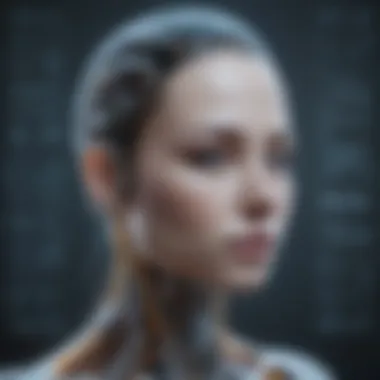
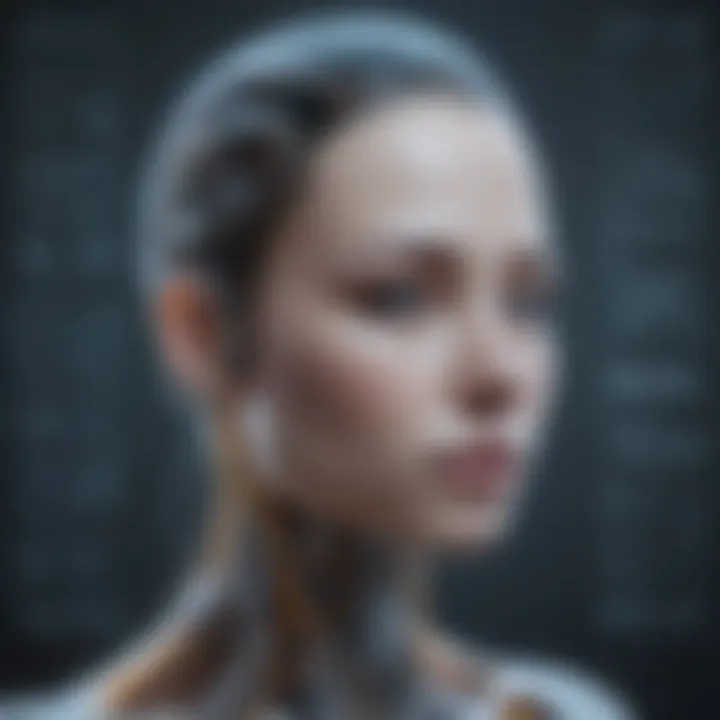
Key Milestones in AI Diagnostics:
Key milestones in AI diagnostics mark pivotal achievements in the integration of artificial intelligence within the diagnostic landscape. These milestones encompass breakthroughs in machine learning models, such as convolutional neural networks, heralding a new era of image analysis and pattern recognition. Such advancements not only elevate diagnostic accuracy but also offer insights into future trends and implications of AI in diagnostic imaging, underscoring the progressive nature of this field.
Advancements in AI Technologies for Diagnostic Imaging
In the realm of diagnostic imaging, the advancements in AI technologies play a crucial role in transforming healthcare practices. Leveraging cutting-edge machine learning algorithms, AI has the potential to revolutionize diagnostic accuracy, streamline workflows, and enhance patient outcomes. By harnessing the power of AI, healthcare providers can delve deeper into detecting abnormalities, identifying diseases, and optimizing treatment plans with unprecedented precision and efficiency. The integration of AI technologies in diagnostic imaging marks a significant shift towards personalized and evidence-based medicine, paving the way for a more proactive approach to healthcare delivery.
Machine Learning Algorithms
Deep Learning Models
Deep learning models form the backbone of AI technologies in diagnostic imaging, offering a sophisticated approach to data analysis and pattern recognition. Their ability to autonomously learn from vast amounts of imaging data enables them to identify complex patterns and features that may elude the human eye. Deep learning models excel in tasks such as image classification, segmentation, and anomaly detection, making them instrumental in enhancing diagnostic accuracy and expediting decision-making processes. Despite their computational complexity, deep learning models stand out for their adaptability and scalability, continually improving their performance with more data and training.
Convolutional Neural Networks
Convolutional neural networks (CNNs) represent a specialized class of deep learning models tailored specifically for image analysis tasks. Their hierarchical structure mimics the visual processing of the human brain, allowing them to effectively extract relevant features from medical images. CNNs have gained widespread popularity in diagnostic imaging due to their unparalleled performance in tasks like object detection, image recognition, and image segmentation. Their inherent ability to preserve spatial relationships within images makes them a preferred choice for tasks requiring fine-grained analysis and precise localization of abnormalities. However, the computational demands of training CNNs and the need for extensive datasets remain notable challenges in their implementation.
Image Analysis and Pattern Recognition
Automated Image Segmentation
Automated image segmentation plays a pivotal role in diagnostic imaging by automating the process of delineating structures of interest within medical images. This technology enables precise identification and segmentation of organs, tissues, and lesions, facilitating quantitative analysis and treatment planning. By leveraging sophisticated algorithms and machine learning techniques, automated image segmentation enhances workflow efficiency, reduces manual intervention, and ensures consistency in diagnostic interpretations. Its seamless integration with advanced visualization tools empowers healthcare providers to analyze complex anatomical structures with unprecedented accuracy and speed.
Lesion Detection and Classification
The detection and classification of lesions represent a critical aspect of diagnostic imaging, directly influencing treatment decisions and prognostic assessments. AI-powered systems for lesion detection leverage advanced image analysis algorithms to identify suspicious areas within medical images, aiding radiologists in early disease detection and characterization. By automating the detection and classification of lesions, these systems streamline diagnostic workflows, minimize interpretation errors, and expedite the reporting process. The integration of AI technologies for lesion detection and classification holds immense promise in enhancing diagnostic accuracy, reducing turnaround times, and ultimately improving patient care outcomes.
Enhanced Diagnostic Accuracy
Precision Medicine Applications
Precision medicine applications harness the capabilities of AI to tailor medical treatment strategies to individual patient characteristics, preferences, and genetic profiles. By integrating patient-specific data with advanced analytics, precision medicine applications enable healthcare providers to deliver personalized and targeted therapies with unprecedented accuracy and efficacy. These applications support clinicians in selecting optimal treatment regimens, monitoring treatment responses, and predicting disease progression with a high degree of precision. The incorporation of precision medicine approaches in diagnostic imaging empowers healthcare professionals to make informed clinical decisions, optimize treatment outcomes, and enhance the overall quality of patient care.
Minimizing Diagnostic Errors
AI technologies play a pivotal role in minimizing diagnostic errors by providing additional layers of decision support and error detection mechanisms. Through real-time analysis of medical images and clinical data, AI systems can flag inconsistencies, aberrations, or overlooked features that may escape human detection. By serving as a complementary tool to radiologists and healthcare practitioners, AI helps mitigate the risks of misdiagnosis, oversight, and variability in diagnostic interpretations. Emphasizing the importance of quality assurance and error reduction, AI applications in diagnostic imaging aim to enhance diagnostic accuracy, increase confidence in clinical assessments, and improve overall healthcare outcomes through proactive error prevention.
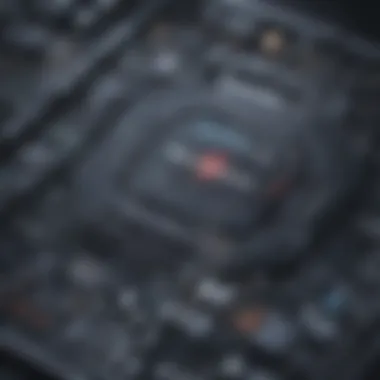
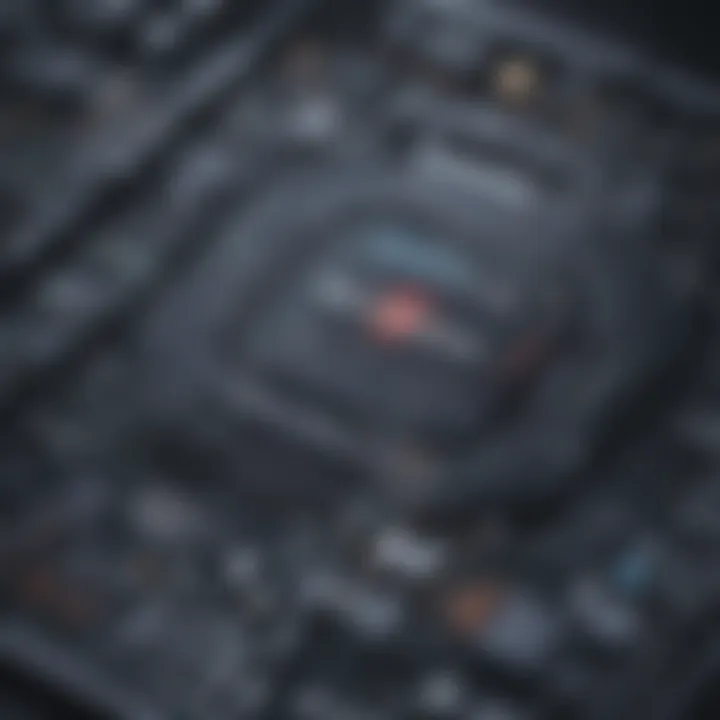
Challenges and Limitations in Implementing AI in Diagnostic Imaging
In the domain of diagnostic imaging, the integration of artificial intelligence presents a revolutionary shift in the approach to medical diagnostics. Understanding the challenges and limitations inherent in implementing AI in this context is crucial for optimizing its benefits. One of the primary considerations is the issue of data privacy and security concerns, which play a significant role in shaping the acceptance and adoption of AI technologies in healthcare settings. Ensuring compliance with regulations such as HIPAA (Health Insurance Portability and Accountability Act) is paramount in safeguarding patient information and maintaining confidentiality in diagnostic procedures. This adherence to HIPAA guidelines not only protects patient data but also establishes a framework for ethical and secure AI implementations within diagnostic imaging.
Data Privacy and Security Concerns
HIPAA Compliance
HIPAA Compliance stands as a fundamental pillar in preserving the privacy and security of patient health information within AI-supported diagnostic imaging processes. The meticulous adherence to HIPAA guidelines dictates the handling and safeguarding of sensitive medical data, assuring patients that their information is handled with the utmost confidentiality. By incorporating HIPAA-compliant practices into AI systems, healthcare providers can instill trust and credibility among patients, reinforcing the ethical foundation of diagnostic imaging practices. While ensuring HIPAA Compliance requires stringent measures and ongoing diligence, its integration is indispensable for the successful deployment of AI technologies in diagnostic imaging.
Protection of Patient Data
The protection of patient data in the realm of diagnostic imaging is a multifaceted aspect that necessitates comprehensive strategies to mitigate risks and vulnerabilities. Safeguarding patient data involves employing encryption methods, access controls, and data anonymization techniques to prevent unauthorized access and data breaches. Prioritizing the protection of patient data not only aligns with regulatory requirements but also bolsters patient trust and confidence in AI-driven diagnostic procedures. However, the challenge lies in balancing data security measures with seamless data accessibility for healthcare professionals, ensuring that patient data remains secure while enabling efficient diagnostic workflows. Achieving a harmonious equilibrium between data protection and accessibility is imperative for the ethical and successful implementation of AI in diagnostic imaging.
Interdisciplinary Collaboration
In the dynamic landscape of diagnostic imaging, effective interdisciplinary collaboration plays a pivotal role in bridging the gap between AI technologies and traditional radiology practices. Integrating AI with radiology practices offers a streamlined approach to diagnostics, enhancing the accuracy and efficiency of image interpretation. By intertwining AI algorithms with radiological expertise, healthcare providers can leverage the strengths of both technologies to deliver precise and timely diagnostic results. This collaborative synergy not only optimizes diagnostic accuracy but also augments the capabilities of healthcare professionals, empowering them to make informed clinical decisions based on AI-driven insights.
Integration with Radiology Practices
The integration of AI with radiology practices orchestrates a symbiotic relationship between technological advancements and clinical expertise. By seamlessly incorporating AI tools into radiological workflows, healthcare facilities can expedite the diagnostic process, reduce turnaround times, and improve patient outcomes. Radiologists benefit from AI-driven tools that assist in image analysis, interpretation, and decision-making, augmenting their diagnostic acumen and streamlining workflow efficiencies. This fusion of AI with radiology practices heralds a new era of precision diagnostics, where the amalgamation of human intellect and artificial intelligence converges to elevate diagnostic standards in healthcare settings.
Training and Education for Healthcare Professionals
Empowering healthcare professionals with the requisite training and education in AI technologies is paramount for ensuring proficient integration and utilization of AI in diagnostic imaging. Through specialized training programs and continuing education initiatives, healthcare practitioners can enhance their knowledge and skills in navigating AI-driven diagnostic tools. Equipping professionals with the competencies to interpret AI-generated insights and collaborate with intelligent systems fosters a harmonious synergy between human expertise and machine intelligence. By investing in comprehensive training programs, healthcare institutions can cultivate a workforce that is adept at harnessing the full potential of AI technologies, ultimately enhancing diagnostic accuracy and patient care outcomes.
Ethical Considerations
Ethical considerations form the ethical framework that guides the responsible deployment and utilization of AI technologies in diagnostic imaging. Transparent algorithmic decision-making ensures accountability and clarity in the reasoning behind diagnostic outcomes, enabling healthcare providers to comprehend and validate AI-generated results. Incorporating human oversight and accountability in AI systems establishes checks and balances that prevent erroneous diagnoses and mandate human intervention when necessary. By upholding principles of transparency, accountability, and human supervision, healthcare organizations can uphold ethical standards in diagnostic imaging practices, fostering trust and reliability in AI-driven healthcare solutions.
Transparency in Algorithmic Decision-making
The transparency of algorithmic decision-making processes is imperative for promoting trust and understanding in AI-driven diagnostic imaging. By elucidating the decision-making pathways of AI algorithms, healthcare professionals can ascertain the rationale behind diagnostic recommendations and validate the accuracy of AI-generated insights. Transparent algorithms engender confidence in the diagnostic process, enabling healthcare providers to interpret results effectively and make informed clinical decisions based on AI-driven analyses. Transparency not only enhances the explainability of AI systems but also facilitates the seamless integration of artificial intelligence into diagnostic workflows, promoting collaborative decision-making between human experts and intelligent algorithms.
Human Oversight and Accountability
Human oversight and accountability serve as foundational principles in the ethical governance of AI technologies within diagnostic imaging. While AI systems offer remarkable capabilities in augmenting diagnostic accuracy and efficiency, human oversight remains essential in ensuring the ethical conduct of diagnostic procedures. Healthcare professionals play a pivotal role in overseeing AI-generated results, verifying diagnoses, and intervening in cases where human judgment supersedes algorithmic recommendations. Human oversight acts as a safeguard against potential errors or biases in AI predictions, reinforcing the patient-centric approach to diagnostic imaging. By incorporating mechanisms for human accountability and intervention, healthcare institutions can uphold ethical standards, mitigate risks, and prioritize patient safety in AI-supported diagnostic practices.
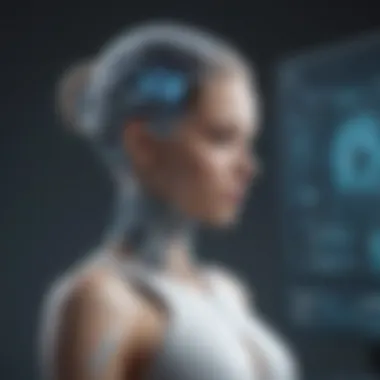
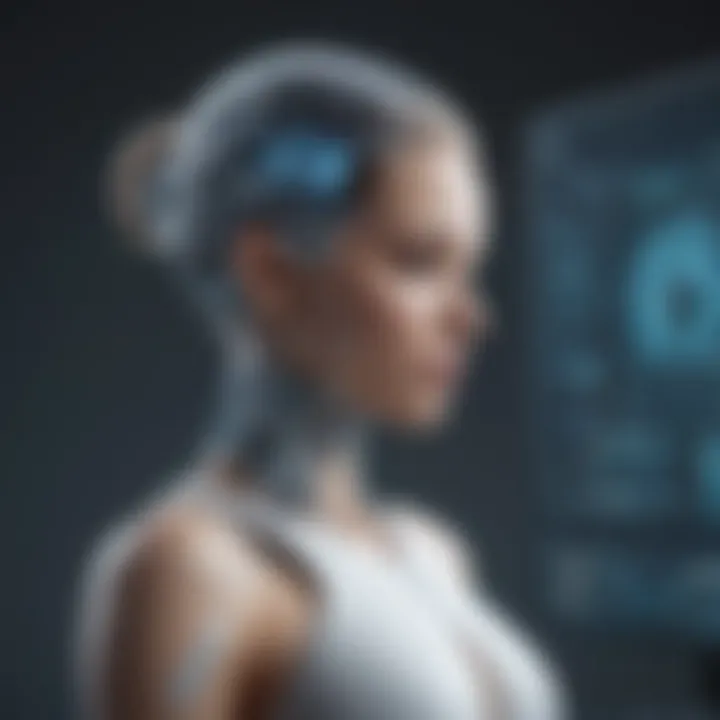
Impact of AI on Workflow Efficiency and Healthcare Outcomes
In the realm of diagnostic imaging, the incorporation of artificial intelligence (AI) has proven to be a game-changer, significantly influencing workflow efficiency and healthcare outcomes. The utilization of AI algorithms and machine learning techniques has streamlined processes, leading to enhanced precision in diagnostic procedures, ultimately facilitating better patient care. By automating repetitive tasks and reducing human error, AI ensures that healthcare professionals can focus their time and expertise where it matters most, resulting in improved efficiency throughout the healthcare system. Moreover, AI implementation fosters quicker decision-making, reduces turnaround times for diagnosis, and optimizes the allocation of resources, contributing to more timely treatments and interventions.
Streamlining Diagnostic Processes
Reducing Turnaround Time
One of the key components of enhancing workflow efficiency through AI is the reduction of turnaround time in diagnostic processes. By automating image analysis and report generation, AI accelerates the interpretation of medical images, speeding up the overall diagnostic timeline. This reduction in turnaround time is critical in urgent cases where swift diagnosis can be a matter of life and death. The ability of AI to rapidly process and identify abnormalities in medical scans significantly enhances the speed at which healthcare providers can make informed decisions, ultimately improving patient outcomes.
Optimizing Resources Allocation
Optimizing resources allocation is another significant advantage brought about by AI in diagnostic imaging. By effectively allocating resources such as imaging equipment, staff, and expertise based on real-time demand and workload analysis, AI ensures that each aspect of the diagnostic process operates at peak efficiency. This optimization leads to cost savings, improved patient throughput, and enhanced overall workflow within healthcare facilities. Additionally, the intelligent distribution of resources through AI integration allows for a more equitable distribution of workload among healthcare professionals, maximizing their productivity and job satisfaction.
Improving Patient Care
Personalized Treatment Plans
AI's contribution to improving patient care is highly exemplified through the development of personalized treatment plans. By analyzing vast amounts of patient data, AI can provide tailored treatment recommendations based on individual characteristics, medical history, and response to previous interventions. This personalized approach not only results in better treatment outcomes but also minimizes the risks of adverse effects or ineffective therapies, ultimately enhancing the overall quality of patient care.
Enhanced Clinical Decision Support
Another critical aspect of AI's impact on patient care is the provision of enhanced clinical decision support. AI systems can analyze complex data sets quickly and accurately, assisting healthcare providers in making well-informed clinical decisions. By offering evidence-based guidance, interpreting diagnostic results, and predicting potential outcomes, AI empowers healthcare professionals to deliver precise and timely interventions, leading to better patient management. The integration of AI-driven clinical decision support tools further ensures that medical decisions align with the latest research findings and best practices, enhancing the clinical outcomes and overall experience for patients.
Future Directions and Implications of AI in Diagnostic Imaging
Integration of AI with Radiomics and Genomics
Precision Imaging in Oncology
In the realm of diagnostic imaging, the convergence of artificial intelligence with radiomics and genomics ushers in a new era of precision and accuracy. Precision imaging in oncology, a pivotal aspect of this integration, fundamentally transforms how tumors are identified and characterized. The key characteristic of precision imaging lies in its ability to analyze minute details within medical images, providing clinicians with unprecedented insights into tumor biology and behavior. This depth of analysis not only enhances diagnostic accuracy but also plays a crucial role in tailoring personalized treatment plans for cancer patients. Although its utility is undeniable, precision imaging does pose challenges in terms of interpreting complex data patterns and integrating these insights seamlessly into clinical practice.
Predictive Analytics for Disease Progression
Predictive analytics, another vital component of AI integration in diagnostic imaging, offers a proactive approach to disease management and prognosis assessment. This nuanced tool harnesses historical patient data, imaging studies, and clinical outcomes to forecast disease progression with remarkable accuracy. By identifying patterns and trends, predictive analytics empowers healthcare providers to anticipate potential complications and intervene at an early stage. Its predictive power is a valuable asset in optimizing treatment strategies and resource allocation within healthcare settings. However, the reliance on historical data and algorithms raises ethical concerns regarding patient privacy and data security, underscoring the need for stringent safeguards and regulatory oversight.
Emerging Trends in AI Diagnostics
AI-Driven Radiology Interpretation
The emergence of AI-driven radiology interpretation signifies a paradigm shift in diagnostic imaging, amplifying the efficiency and precision of radiological evaluations. Leveraging advanced algorithms, AI systems can analyze complex imaging data rapidly and accurately, aiding radiologists in detecting subtle abnormalities and anomalies. The key characteristic of AI-driven interpretation lies in its ability to augment human expertise, reducing interpretation errors and enhancing diagnostic confidence. Despite its significant advantages in expedited diagnosis and reporting, the reliance on AI raises concerns about the potential supplanted role of radiologists and the preservation of clinical acumen in decision-making processes.
Virtual Biopsy Technologies
Virtual biopsy technologies epitomize the innovation and potential of AI applications in diagnostic imaging, offering non-invasive alternatives to traditional tissue sampling procedures. By reconstructing three-dimensional images from standard two-dimensional scans, virtual biopsies provide detailed insights into tissue structures and cellular dynamics without invasive interventions. The key characteristic of virtual biopsy lies in its capacity to enhance diagnostic precision and reduce patient discomfort associated with invasive biopsy procedures. However, the lack of histopathological confirmation and standardization in virtual biopsy procedures present challenges in terms of diagnostic accuracy and clinical validation, necessitating further research and validation studies for widespread adoption in clinical settings.