Unlocking the Potential of Big Data Analytics: An In-Depth Guide
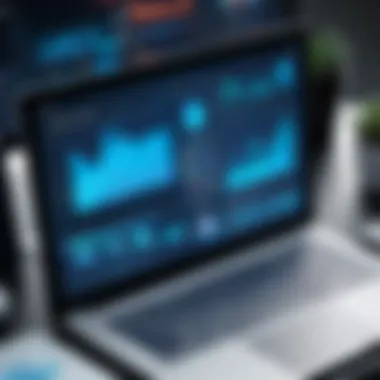
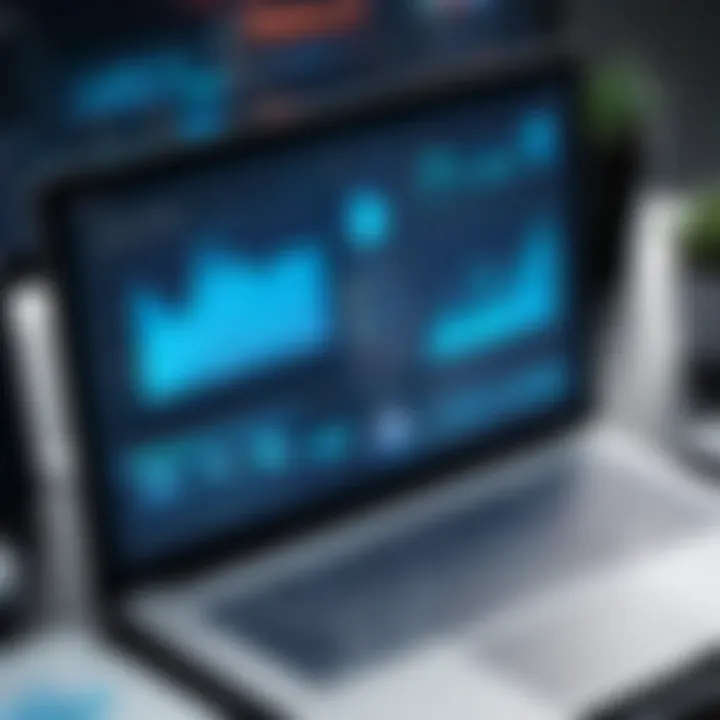
Understanding Storage, Security, or Networking Concepts
As we delve into the world of big data analytics, it is paramount to grasp the fundamental principles of storage, security, and networking. These concepts form the backbone of data management and must be comprehended to harness the power of analytics fully. Storage refers to the preservation of data, be it structured or unstructured, in a systematic manner. Security entails safeguarding this data from unauthorized access or breaches. Networking involves the interconnection of devices for seamless data transfer and communication. Understanding these pillars is crucial for effective data utilization and protection.
Industry Trends and Updates
In the dynamic landscape of data analytics, staying abreast of industry trends and updates is essential for professionals and experts alike. Constant evolution characterizes storage technologies with the emergence of cloud storage and distributed systems revolutionizing data management practices. Cybersecurity poses a perennial challenge, with evolving threats necessitating innovative solutions to safeguard sensitive information. Similarly, networking witnesses continuous advancements with the rise of virtual networks and software-defined networking optimizing performance. Acknowledging these trends is imperative for professionals to adapt and thrive in the ever-evolving realm of data analytics.
Case Studies and Success Stories
Illustrating practical implications of theoretical concepts, case studies and success stories provide real-life examples of effective data analytics implementation. These narratives offer actionable insights into utilizing storage resources efficiently, mitigating cybersecurity risks effectively, and optimizing networking strategies for enhanced performance. By analyzing successful cases and learning from past incidents, professionals can glean invaluable lessons and refine their approach to data management. Examining these case studies enriches comprehension and guides practitioners in navigating the complexities of data analytics with finesse.
Reviews and Comparison of Tools and Products
Selecting the appropriate tools and products is paramount in ensuring streamlined data analytics operations. Comprehensive reviews of storage software and hardware elucidate their features, functionalities, and compatibility with specific requirements. Similarly, comparing cybersecurity tools and solutions enables professionals to assess their efficacy in combating various threats and vulnerabilities effectively. Evaluating networking equipment and services unveils performance benchmarks and highlights the advantages of different options, aiding in informed decision-making for optimizing data connectivity and communication.
Introduction to Big Data Analytics
Big Data Analytics holds a crucial role in our digital era, revolutionizing the way we extract insights from vast collections of information. In this article, we will delve into the intricacies of Big Data Analytics, shedding light on its significance in enhancing decision-making processes, strategizing, and optimizing operational efficiencies. Understanding the core concepts and components of Big Data Analytics is paramount for professionals and enthusiasts seeking to harness the power of data innovation.
Defining Big Data Analytics
Understanding the concept of Big Data Analytics is fundamental to grasping its operational framework. It revolves around the sophisticated process of analyzing and interpreting extensive datasets to unearth valuable patterns, trends, and correlations. The essence lies in leveraging advanced algorithms and computational tools to extract actionable insights from these colossal data sets. This detailed exploration of Big Data Analytics serves as a cornerstone for strategic planning and data-driven decision-making.
Exploring the importance of analyzing large datasets presents a practical perspective on maximizing data utilization. By delving deep into vast information pools, organizations can gain profound insights into customer behavior, market trends, and operational efficiency. The ability to process and interpret large datasets allows businesses to tailor their strategies, predict outcomes, and drive innovation forward. However, the complexity of managing big data calls for robust analytical frameworks and adept data handling techniques.
Key Components of Big Data Analytics
Data Collection
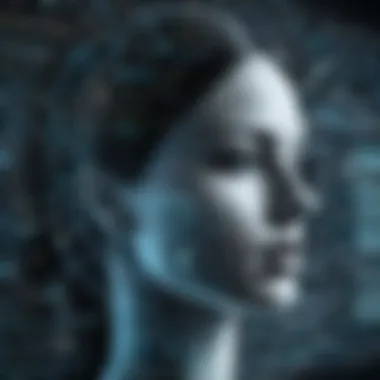
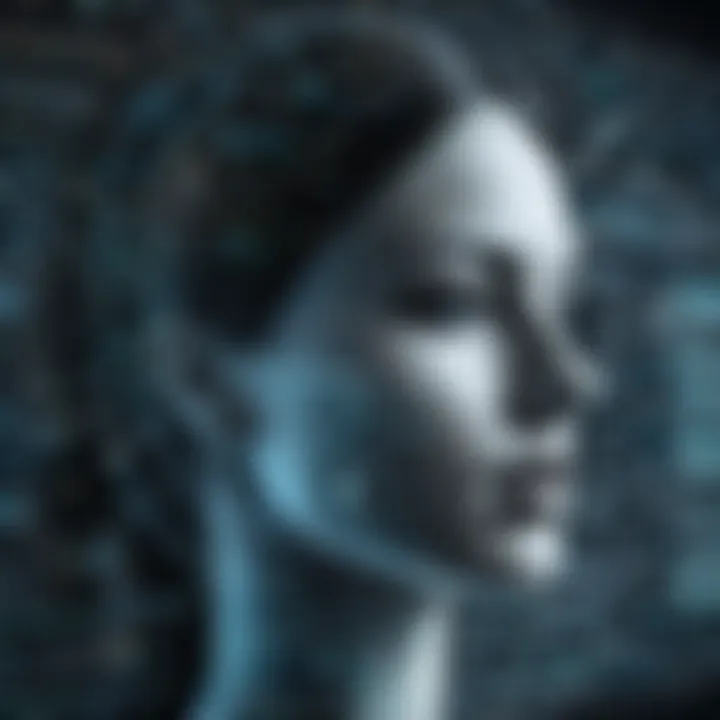
Data collection forms the foundation of Big Data Analytics, encompassing the process of gathering raw information from various sources. This critical stage involves harvesting structured and unstructured data points from sources such as Io T devices, social media platforms, sensors, and databases. Establishing a streamlined data collection mechanism ensures the availability of diverse data sets for analysis, enabling comprehensive insights.
Data Storage
Data storage entails the management and organization of vast amounts of data in structured repositories. Efficient data storage solutions such as data lakes or data warehouses facilitate easy access, retrieval, and preservation of data. By implementing scalable and secure storage systems, organizations can maintain data integrity and optimize retrieval processes, enhancing overall analytical efficiency.
Data Processing
Data processing involves the transformation of raw data into meaningful insights through cleansing, integration, and aggregation processes. This crucial phase ensures data accuracy, consistency, and relevance for subsequent analysis. Leveraging powerful processing tools accelerates analytical procedures, leading to faster decision-making and robust predictive modeling.
Data Analysis
Data analysis is the core of Big Data Analytics, involving the application of statistical and machine learning techniques to extract valuable insights. By dissecting data sets, identifying patterns, and generating meaningful interpretations, organizations can make informed decisions and discover untapped opportunities. The synergy between data analysis and business objectives forms the basis of data-driven strategies and innovative solutions.
Data Visualization
Data visualization plays a pivotal role in transforming complex data sets into visually engaging and interpretable representations. Through graphs, charts, and interactive dashboards, data visualization enhances data comprehension and facilitates seamless communication of insights. Visualizing data allows stakeholders to gain holistic perspectives, identify trends, and derive actionable conclusions from intricate datasets.
Significance of Big Data Analytics
Enabling data-driven decision-making
Data-driven decision-making empowers organizations to base their strategies and operations on factual insights derived from thorough data analysis. By cultivating a culture of data-driven decision-making, businesses can steer towards informed choices, optimize processes, and anticipate market trends effectively. The integration of data-driven practices fosters a dynamic and responsive organizational ecosystem, driving sustainable growth and competitiveness.
Facilitating predictive analytics
Predictive analytics leverages historical data and statistical algorithms to forecast future trends, behaviors, and outcomes. By applying predictive models, organizations can anticipate customer preferences, identify risks, and streamline operations for enhanced efficiency. The predictive capabilities of Big Data Analytics enable proactive decision-making, risk mitigation, and resource optimization, positioning businesses ahead in a fast-evolving landscape.
Enhancing operational efficiency
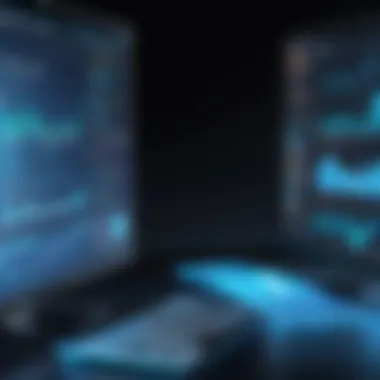
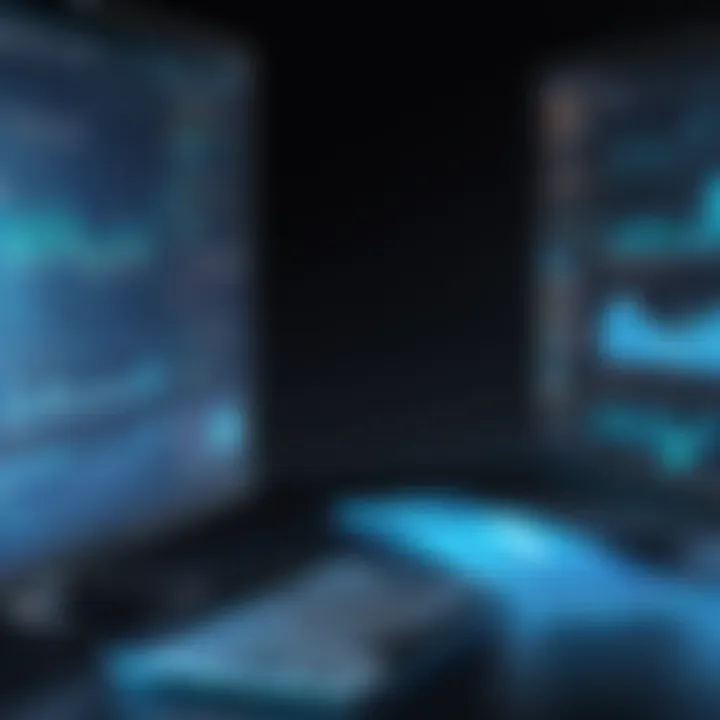
Enhancing operational efficiency through Big Data Analytics involves streamlining processes, optimizing resource allocation, and minimizing wastage. By harnessing data insights, organizations can identify bottlenecks, inefficiencies, and areas for improvement, leading to cost reductions and performance enhancements. The strategic deployment of analytics tools and techniques enhances operational workflows, fosters agility, and ensures sustainable business practices.
Driving business growth
Leveraging Big Data Analytics to drive business growth entails leveraging data insights to identify market opportunities, customer segments, and strategic pathways for expansion. By aligning data analytics with business objectives, organizations can innovate, adapt to changing market dynamics, and capitalize on emerging trends. The strategic deployment of analytics tools fuels innovation, improves customer experiences, and cultivates a competitive edge in the marketplace.
Applications of Big Data Analytics
In the landscape of modern technology, the Applications of Big Data Analytics hold a crucial position. By harnessing the power of large datasets, businesses can gain valuable insights to drive decision-making processes, enable predictive analytics, enhance operational efficiency, and spur business growth. The realm of Big Data Analytics is intricate yet essential in navigating the complexities of the digital era. Understanding its applications unveils a world of possibilities and strategic advantages for organizations seeking to leverage data-driven approaches.
Industry Applications:
- Finance: Within the domain of Finance, Big Data Analytics plays a pivotal role in risk assessment, fraud detection, and customer profiling. The ability to process vast amounts of financial data in real-time empowers financial institutions to make informed decisions swiftly. While this sector benefits greatly from predictive analytics, it also faces challenges such as data privacy concerns and regulatory compliance.
- Healthcare: In Healthcare, the use of Big Data Analytics revolutionizes patient care, disease prediction, and personalized medicine. By analyzing medical records, genomic data, and treatment outcomes, healthcare providers can tailor interventions to individual patients, leading to improved outcomes and operational efficiencies. However, the integration of Big Data Analytics in healthcare is met with challenges related to data security, ethical considerations, and ensuring patient confidentiality.
- Retail: The Retail sector capitalizes on Big Data Analytics for consumer behavior analysis, inventory management, and personalized marketing strategies. Understanding customer preferences and buying patterns through data analytics enables retailers to optimize pricing, promotions, and product offerings. While data-driven insights can drive profitability and customer satisfaction, retailers must address issues of data accuracy, privacy, and data integration across various platforms.
- Marketing: Big Data Analytics reshapes the landscape of Marketing by enabling targeted advertising, campaign optimization, and customer segmentation. Marketers leverage data analytics to refine marketing strategies, enhance customer engagement, and measure campaign performance effectively. Despite the benefits of personalized marketing initiatives, marketers face challenges related to data quality, consumer privacy concerns, and navigating the ever-evolving digital marketing platforms.
- Telecommunications: In the Telecommunications industry, Big Data Analytics drives network optimization, customer retention strategies, and predictive maintenance of infrastructure. By analyzing call data records, network traffic patterns, and customer interactions, telecom companies can enhance service quality, prevent network failures, and deliver personalized services to subscribers. However, challenges such as data security, network scalability, and regulatory compliance pose significant hurdles in leveraging Big Data Analytics effectively.
Use Cases:
- Customer Segmentation: Customer Segmentation through Big Data Analytics allows businesses to categorize customers based on behavior, preferences, and purchasing patterns. By segmenting customers, organizations can tailor marketing strategies, improve customer retention, and enhance the overall customer experience. The unique feature of Customer Segmentation lies in its ability to identify lucrative market segments and target them with personalized offerings. However, challenges in data collection, integration, and maintaining data accuracy impact the reliability of segmentation strategies.
- Fraud Detection: Big Data Analytics transforms Fraud Detection by enabling the identification of suspicious activities, anomalies, and irregular patterns in transactions. Financial institutions, e-commerce platforms, and insurance companies leverage data analytics to detect fraudulent behavior, mitigate risks, and safeguard financial assets. The key characteristic of Fraud Detection lies in its proactive approach to identifying fraud before extensive losses occur. Despite its effectiveness, fraud detection systems must continuously evolve to counter new fraud tactics, ensure data integrity, and minimize false positive alerts.
- Recommendation Systems: Recommendation Systems powered by Big Data Analytics enhance user experience, drive engagement, and boost sales through personalized recommendations. E-commerce platforms, streaming services, and social media utilize recommendation systems to offer relevant content, products, and services to users based on their preferences and behavior. The unique feature of Recommendation Systems lies in their ability to analyze vast amounts of user data in real-time to provide accurate and timely recommendations. However, challenges in data privacy, algorithm bias, and evolving user preferences influence the efficacy of recommendation systems.
- Predictive Maintenance: The implementation of Predictive Maintenance through Big Data Analytics revolutionizes asset management, reduces downtime, and improves operational efficiency. By analyzing equipment sensors, performance data, and historical maintenance records, organizations can predict potential failures, schedule maintenance proactively, and optimize resource allocation. The key characteristic of Predictive Maintenance is its proactive and cost-effective approach to maintenance, preventing unexpected breakdowns and optimizing asset lifecycle. Despite its benefits, predictive maintenance systems require accurate data collection, integration with existing maintenance processes, and skilled personnel to interpret predictive insights effectively.
- Market Basket Analysis: Market Basket Analysis leverages Big Data Analytics to uncover associations, correlations, and patterns in customer purchasing behavior. Retailers, supermarkets, and e-commerce platforms utilize market basket analysis to identify product affinities, optimize product placements, and enhance cross-selling initiatives. The unique feature of Market Basket Analysis lies in its ability to increase sales, improve customer satisfaction, and drive marketing strategies based on consumer preferences. However, challenges such as data volume, algorithm complexity, and maintaining data privacy influence the implementation and accuracy of market basket analysis.
Real-World Examples:
- Netflix's Content Recommendations: Netflix's Content Recommendation system utilizes Big Data Analytics to suggest personalized content to viewers based on their viewing history and preferences. By analyzing user interactions, ratings, and viewing habits, Netflix delivers tailored recommendations that enhance user engagement and satisfaction. The key characteristic of Netflix's Content Recommendations lies in its ability to predict user preferences accurately, leading to increased viewership and subscriber retention. Although popular, this recommendation system faces challenges related to privacy concerns, algorithm transparency, and balancing user preferences with content diversity.
- Amazon's Personalized Shopping Experience: Amazon's Personalized Shopping Experience incorporates Big Data Analytics to offer personalized product recommendations, targeted advertisements, and optimized search results for shoppers. By analyzing purchase history, browsing behavior, and demographic data, Amazon enhances the shopping experience by presenting relevant products and promotions to customers. The unique feature of Amazon's Personalized Shopping Experience is its dynamic algorithm that adapts in real-time to changes in user preferences and market trends. Despite its popularity, challenges in data privacy, algorithm bias, and maintaining data security influence customer trust and satisfaction.
- Google's Search Algorithms: Google's Search Algorithms rely on Big Data Analytics to deliver accurate and relevant search results to users worldwide. By analyzing website content, user queries, and search patterns, Google's algorithms rank web pages based on relevance and authority, enhancing the user search experience. The key characteristic of Google's Search Algorithms lies in their ability to process vast amounts of data rapidly to provide users with tailored search results. However, these algorithms face challenges related to data privacy, algorithm updates, and ensuring search result quality amidst evolving internet trends.
- Uber's Dynamic Pricing: Uber's Dynamic Pricing model utilizes Big Data Analytics to adjust ride fares based on supply and demand dynamics, traffic conditions, and user behavior. By analyzing rider demand patterns, driver availability, and external factors, Uber's algorithm optimizes pricing to balance rider interests and driver earnings. The unique feature of Uber's Dynamic Pricing lies in its ability to respond to market fluctuations in real-time, ensuring efficient matching of supply and demand. Despite its effectiveness in optimizing earnings, dynamic pricing models face challenges related to transparency, algorithm fairness, and user acceptance during peak demand periods.
Challenges and Limitations
In the realm of big data analytics, understanding and addressing challenges and limitations play a pivotal role in shaping data strategies. Recognizing these hurdles leads to informed decision-making and improved outcomes. Data Privacy and Security hold paramount importance in the digital landscape. Ensuring data confidentiality is a crucial aspect that demands meticulous attention. By safeguarding sensitive information from unauthorized access, organizations uphold integrity and trust. This proactive measure not only mitigates risks but also fortifies data governance frameworks. On the flip side, there are considerations regarding the trade-off between security and accessibility. Striking a balance between these aspects is imperative when devising robust security protocols. Mitigating cybersecurity risks is another critical facet that cannot be overlooked. Organizations must proactively combat potential threats to safeguard their data assets. Implementing stringent cybersecurity measures fortifies the data infrastructure against malicious activities. However, the challenge lies in adapting to evolving cyber threats and staying ahead of cybercriminal tactics. Constant vigilance and investment in cutting-edge security technologies are essential to counter sophisticated cyber-attacks.
Data Quality Issues
Maintaining data quality is a core concern in big data analytics. Managing unstructured data poses unique challenges in terms of organization and interpretation. As unstructured data lacks a predefined format, organizations face difficulties in extracting meaningful insights. Effective management of unstructured data involves structuring and categorizing information for streamlined analysis. Despite its complexity, leveraging unstructured data can uncover valuable patterns and trends, enhancing decision-making processes. Addressing data accuracy concerns is paramount for ensuring the reliability of insights derived from big data analytics. Inaccurate data can lead to erroneous conclusions and misguided strategies. Organizations need to implement robust data validation mechanisms to detect and rectify inaccuracies. By enhancing data accuracy, organizations can enhance the credibility of their analytical outcomes. However, achieving high data accuracy requires ongoing data quality assurance practices and validation protocols.
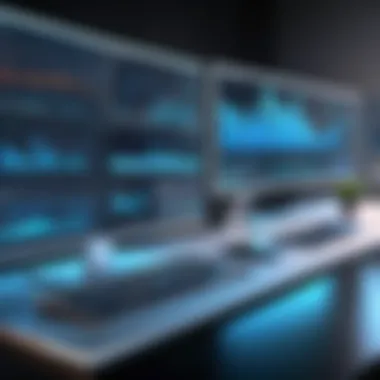
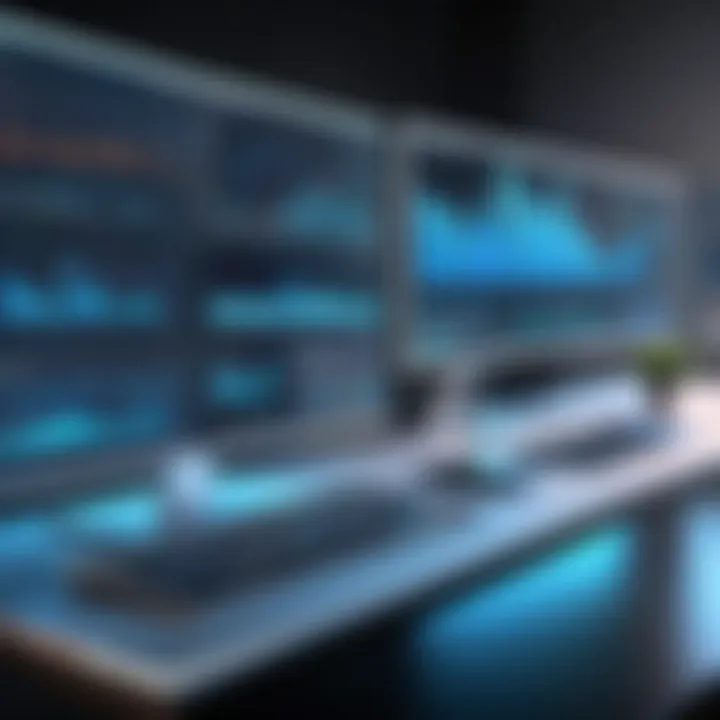
Scalability and Infrastructure
The scalability and infrastructure of big data analytics solutions are instrumental in handling the voluminous amount of data generated. Handling large volumes of data requires scalable infrastructure that can accommodate growing datasets. Scalability allows organizations to adjust their data processing capabilities based on demand fluctuations. Implementing scalable solutions ensures seamless data processing and analysis, improving operational efficiency. Optimizing hardware and software resources is crucial for maximizing the performance of big data analytics systems. Effective resource optimization minimizes latency issues and maximizes processing speeds. By fine-tuning hardware configurations and leveraging efficient software algorithms, organizations can achieve optimal performance. However, resource optimization necessitates a deep understanding of system requirements and constant monitoring to fine-tune resource allocation.
Future Trends in Big Data Analytics
Understanding the future trends in big data analytics is pivotal in staying abreast of technological advancements and capitalizing on emerging opportunities. As the digital landscape continues to evolve, the integration of artificial intelligence (AI) and machine learning stands out as a game-changer in the realm of data analytics. This fusion streamlines data analysis processes by automating intricate tasks, leading to enhanced efficiency and accuracy in decision-making. By leveraging AI and machine learning capabilities, organizations can delve deeper into data insights, predict trends with greater precision, and optimize operational strategies for sustainable growth.
AI and Machine Learning Integration
Automating Data Analysis Processes
Automating data analysis processes revolutionizes the way organizations handle vast datasets. This automated approach expedites data processing, minimizing manual intervention and human errors. The key characteristic of automating data analysis lies in its ability to analyze large volumes of data at unprecedented speeds. This facet proves especially beneficial in this context as it enables quick and accurate decision-making based on real-time data trends. However, challenges such as ensuring data quality and maintaining algorithm transparency must be navigated to fully harness the advantages of automation in data analysis.
Enhancing Predictive Modeling Capabilities
Enhancing predictive modeling capabilities equips organizations with the foresight needed to anticipate future trends and proactively address challenges. By integrating advanced algorithms and machine learning techniques, predictive modeling can forecast outcomes with remarkable accuracy. The key characteristic of this enhancement is its ability to identify patterns within data sets, enabling businesses to make informed decisions based on reliable projections. While enhancing predictive modeling fosters strategic planning and risk mitigation, consideration must be given to data privacy concerns and model interpretability to uphold ethical principles and regulatory compliance.
Edge Computing and Real-Time Analytics
Reducing Data Latency
The integration of edge computing plays a pivotal role in reducing data latency, allowing for faster data processing and real-time decision-making. By decentralizing data processing to the edge of the network, delays in data transmission are minimized, ensuring timely insights and responses to evolving data trends. The key characteristic of reducing data latency lies in its ability to optimize network efficiency and enhance user experiences by delivering instantaneous results. However, concerns regarding data security and network reliability need to be addressed to maximize the benefits of reduced data latency in analytical processes.
Enabling Instant Insights
Enabling instant insights empowers organizations to derive critical information promptly, facilitating agile decision-making and responsive action. By leveraging real-time analytics capabilities, businesses can extract valuable insights from live data streams, enabling proactive adjustments and interventions. The key characteristic of enabling instant insights is its capacity to provide up-to-the-minute data analysis, enabling organizations to capitalize on fleeting opportunities and mitigate risks swiftly. Nevertheless, data accuracy and system resilience are essential considerations to ensure the reliability and relevancy of instant insights for strategic decision-making.
Ethical Considerations
Regulating Data Usage
Regulating data usage involves establishing frameworks and policies to govern the collection, processing, and utilization of data resources responsibly. This aspect ensures compliance with legal regulations, safeguards data privacy, and promotes trust among stakeholders. The key characteristic of regulating data usage is its role in fostering data integrity and ethical data practices, underpinning organizational credibility and accountability. While regulating data usage engenders transparency and data governance, challenges such as data sovereignty and cross-border data transfer regulations necessitate a nuanced approach to uphold data ethics effectively.
Promoting Transparency and Accountability
Promoting transparency and accountability in data analytics instills trust and confidence in data-driven decision-making processes. Transparency initiatives aim to disclose the sources of data, algorithms used, and outcomes generated, fostering a culture of openness and trustworthiness. The key characteristic of promoting transparency and accountability is its emphasis on ethical data handling and clear communication of data practices to stakeholders. However, concerns regarding data biases, data breaches, and data misuse underscore the importance of continual monitoring and reinforcement of transparency and accountability measures.