Data Enrichment Techniques for Informed Decision-Making
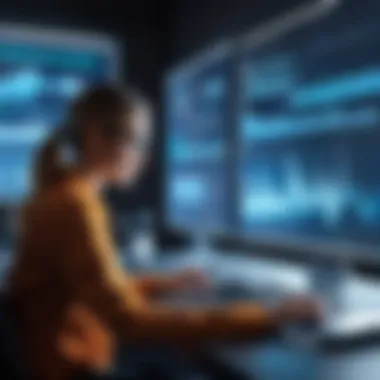
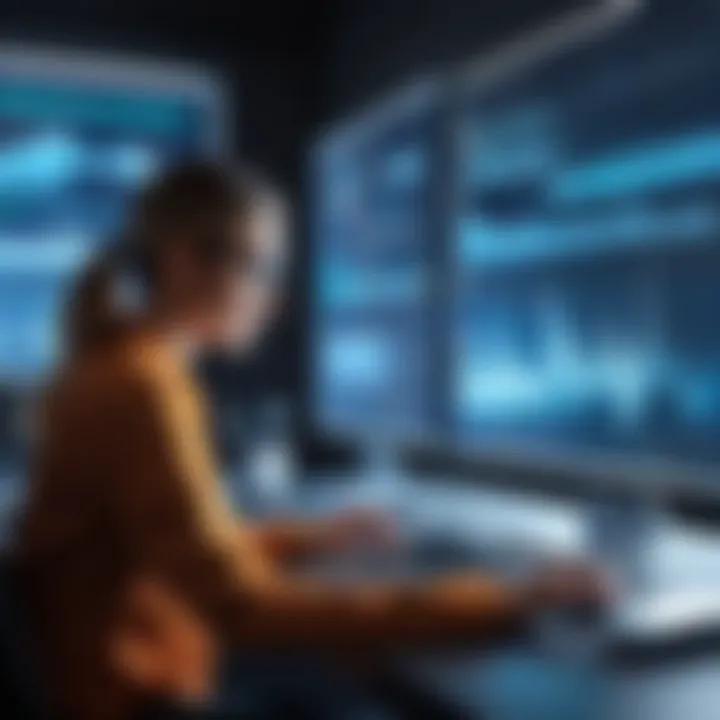
Intro
In today's digital age, data is the cornerstone of productivity and efficiency, impacting all levels of decision-making across various industries. However, raw data is often insufficient to drive effective strategies. This necessitates the application of data enrichment techniques, which enhance, refine, and amplify initial datasets, facilitating better insights and supporting essential business pursuits.
Data enrichment processes can transform basic information into rich, actionable data sets. They can include a wide variety of methods, from augmenting data sources to applying advanced analytical techniques. At its core, data enrichment aims to improve the accuracy, relevance, and usability of data to empower organizations with improved strategic insights. Without robust enrichment methods, decision-making can rely on incomplete or irrelevant data, leading to subpar results. Thus, understanding the techniques of data enrichment becomes critical for any entity seeking to leverage its data assets effectively.
The purpose of this article is to delve deep into the various data enrichment techniques currently in practice, underscore their significance, and showcase their applicability in the context of business and technology. Through this exploration, organizations can gain beneficial insights that shape their approach toward informed decision-making.
The role of enriched data in strategic business operations is particularly important. With enhanced datasets, companies can identify trends, predict outcomes, and optimize their resources effectively. Given the rapid developments and increasing complexity in data processing technologies, an effective data enrichment strategy can make a significant difference in achieving organizational goals.
Consequently, this article endeavors to furnish IT professionals, students, and cybersecurity experts with an understanding that transcends the usual information on data manipulation. This exploration will offer a complete guide conducive to informed decision-making that informs not only the tactical aspects of data management but also the strategic perspective necessary for future advancements.
A comprehensive examination of methods for augmenting data will be undertaken, alongside notable case studies demonstrating successful implementations. The conclusion will synthesize these insights, reiterating the importance of implementing robust data enrichment techniques in today’s data-driven landscape.
Prelude to Data Enrichment
Data enrichment is a crucial process that improves the value and quality of raw data. It focuses not only on data enhancement but also on making data more useful and relevant for decision-making. In an era driven by information, the importance of enriched data cannot be overstated.
By effectively utilizing data enrichment techniques, organizations can gain clearer insights from their data pools. This can lead to improved strategic decisions and better operational efficiencies. Simply put, raw data alone is often insufficient. It is essential to integrate additional dimensions that can transform simple facts into vital insights. In this sense, enriching data results in a more comprehensive and multidimensional view of business landscapes.
Importance of Data Enrichment
Data enrichment offers numerous benefits:
- Increased Accuracy: The refined data leads to less misunderstanding, which is essential for informed decision-making.
- Enhanced Relevance: Retiring obsolete or irrelevant information aids in establishing a context that is more useful for current needs.
- Usability Improvement: Training artificial systems to understand intricacies in the data often brings a significant edge.
This article will detail methods and different types of data enrichment techniques. Pockets of knowledge about the practical side of data enrichment encourage deeper relationships with the data itself, encompassing elements that may otherwise go unheard.
Key Takeaway: The underlying capability of enriched data lies in turning a valid observation into a significant decision, underscoring every action a business may take.
However, organizations should remain aware of the challenges associated with data enrichment. Integrating various data sources often raises concerns related to quality, privacy, and compatibility. Thus, comprehending these concepts is critical for leveraging enriched data effectively, promoting better decision-to-action conversion.
Understanding Data Enrichment Techniques
Understanding the processes and methodologies of data enrichment is pivotal for any organization looking to leverage its data effectively. Data enrichment techniques offer a structured way to enhance existing data, bringing additional context that can drive better decision-making. As markets grow more competitive and data grows more dispersed, understanding various data enrichment methods becomes critical. This section will explore the significance of data enrichment, discuss its definitions, and emphasize why organizations should embrace these techniques.
Definition of Data Enrichment
Data enrichment can be succinctly defined as the process of enhancing existing datasets by adding relevant data from various sources. It transforms raw data into actionable insights by augmenting it usually with third-party and potentially voluminous information. This can include appending demographic information, behavioral data, or geographical data, making the original data more robust and useful for end-users. In essence, data enrichment fills in gaps in a dataset, improving accuracy while delivering richer perspectives on the information available. This added layer of detail allows for more nuanced analysis, leading to targeted actions and informed predictions.
The Importance of Enriching Data
Enhancing data quality through enrichment is not only beneficial; it is often essential for effective decision-making. Here are several reasons why enriching data holds importance:
- Comprehensive Insights: Data that receives enrichment enables organizations to develop refined insights. Without enhancement, key characteristics and trends may be overlooked, ultimately leading to misinformed strategies.
- Improved Targeting: For marketers, enriched data offers the ability to craft precise segments for campaigns. This precise targeting translates to higher-efficiency marketing efforts and improved response rates.
- Predictive Accuracy: Enriched datasets enhance predictive models. This method ensures that predictions are based on relevant factors, helping mitigate risks inherent in poor forecasting.
- Utilizing Misinformation: Well-rounded data doesn't just throw more information at a situation; it siphons more clarity out of existing noise.
Types of Data Enrichment
Data enrichment is a critical element in the process of enhancing the quality of data. Various types exist, each offering distinct advantages and serving specific purposes. Understanding these types can help organizations select the appropriate method for their data enrichment needs.
Descriptive Enrichment
Descriptive enrichment provides additional context to existing data through enhanced textual descriptions or attributes. For instance, adding demographic information to customer records allows businesses to gain insights into who their customers are, helping in segmentation and targeted marketing. This type of enrichment often relies on profiling and categorization, increasing clarity and understanding of the underlying data.
Benefits of Descriptive Enrichment includes:
- Enhanced understanding of customer needs
- Better segmentation for personalized marketing
- Improved accuracy in reporting
Geospatial Enrichment
Geospatial enrichment involves the integration of geographical information with other data sets. By mapping data points to specific locations, organizations can visualize patterns and make informed decisions about operations, marketing, and resource allocation.
Data Sources for Enrichment
Data sources for enrichment play a vital role in the landscape of data enhancement. These sources can significantly impact the quality, relevance, and applicability of the data gathered by organizations. Understanding these diverse sources is essential for anyone involved in data practices, as it allows them to choose the best methods for activelty improving their data.
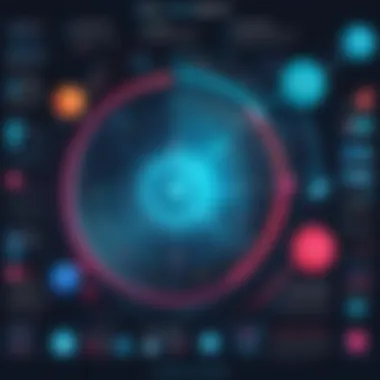
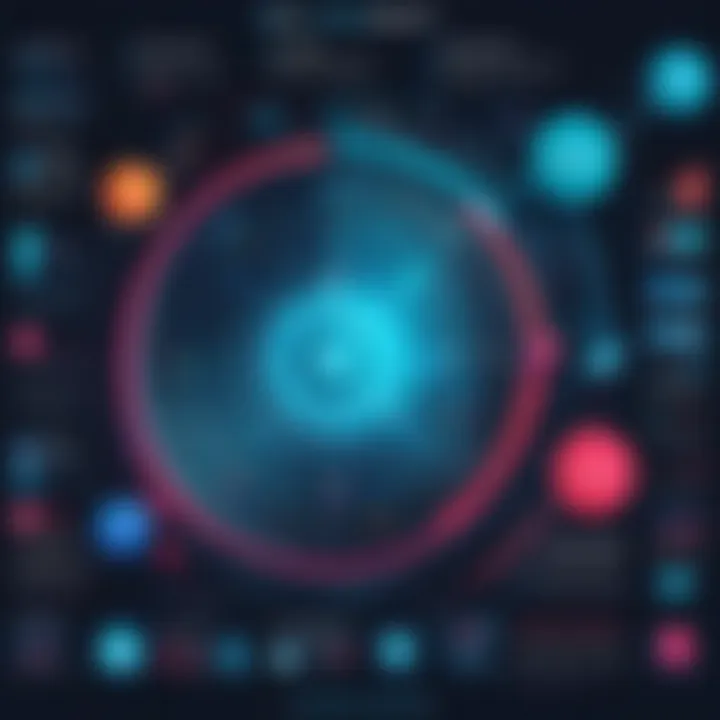
Utilizing internal, external, and public datasets offers organizations different perspectives and insights, which lead to more informed decision-making. These sources provide the foundational elements necessary for building a robust data profile.
Internal Data Sources
Internal data sources refer to the information generated within the organization. This may include transaction records, customer databases, employee data, and operational logs. Using these sources can provide context that enriches analysis with firsthand insights. Internal data is often consistent and reliable, as it relates directly to the operations of the organization.
The benefits of utilizing internal sources are many. Notably, it allows organizations to:
- Leverage existing data without needing to purchase or license external data sets
- Ensure greater consistency and reduced risk of data discrepancies
- Utilize unique organizational insights that may not be shared externally
Advantages measured, however, there are considerations too. It's important to maintain data integrity and to be mindful of data security during the enrichment process.
External Data Sources
External data sources encompass any information collected outside the organization. This includes commercially available databases, industry reports, and social media interactions. These sources can provide additional context and broader perspectives on a company's target audience or market dynamics.
Considering external data enables organizations to:
- Cross-reference internal findings with industry trends
- Gain insights from different geographical or demographic sectors
- Benchmark their performance against competitors
Nonetheless, relying too heavily on external sources may lead to issues involving incorrect interpretations if this data is not validated properly.
Public Datasets
Public datasets are valuable resources that are freely accessible. They include government databases, research institutions, and statistical agencies. This data can cover a wide variety of areas, spanning from environmental statistics to economic figures.
The main benefits of public datasets include:
- Accessibility increases research potential across various fields
- Cost savings, as these resources do not require monetary investment
- Contribute to transparency in research and reporting, as datasets can be independently verified by other researchers
Some challenges may arise concerning the quality and changes over time, so due diligence is necessary.
Successfully enriching data relies on a balanced approach, strategically selecting suitable sources according to the specific goals of the organization.
Methods of Data Enrichment
Data enrichment methods are crucial for organizations aiming to increase the utility and relevance of their data. Choosing the right approach depends on the organization’s specific needs and its current data landscape. Each method has unique elements, benefits, and considerations. The decision between manual and automated processes can influence not only efficiency but also data accuracy. Understanding application programming interfaces, data matching, merging techniques, and machine learning can further enhance one's data enrichment strategies.
Manual vs.
Automated Enrichment
Manual enrichment often involves human intervention to add data-based enhancements. People may research additional information, cross-reference datasets, or gather data from specialized databases. This method can achieve high accuracy but is labor-intensive. It may be suitable where nuances in data require contextual understanding.
In contrast, automated enrichment uses algorithms and software to enhance data. This approach is faster and reduces human error, making it ideal for large datasets. Automated systems can gather real-time data from various sources, providing a dynamic method for data enrichment. However, automated methods must be selected carefully to ensure data quality and relevance.
Key Benefits of Each Approach:
- Manual:
- Automated:
- Contextual Insights
- High accuracy in complex situations
- Speed and efficiency
- Scalability
Using Application Programming Interfaces (APIs)
APIs are gateways that allow systems to communicate and share data seamlessly. They play a crucial role in data enrichment by providing access to external datasets. With APIs, organizations can integrate various data sources, enhancing the richness of their datasets. Apart from growing the data volume, access through APIs ensures that the data is updated regularly, which is critical for maintaining accuracy.
For example, a company may leverage social media APIs to gain insights on consumer behavior. Data pulled from such platforms can provide profiles, demographics, and sentiment analysis. However, API limits on requests may lead an organization to develop robust data handling strategies. Thus, careful architectural design around API usage is necessary for optimal performance.
Data Matching and Merging
Data matching is the process of identifying and linking related records from different sources. This is vital in ensuring that duplicate records do not compromise the quality of the final dataset. Merging involves combining records to create a unified view of information.
Logic-based programming often drives this matching process. Thresholds such as exact matches or found similarities guide automated systems in deciding on data pairs. This method often relies on sound procedures for error handling, considering that there will be discrepancies in formats and languages, and resulting correlations can impact analysis significantly.
Considerations in Data Matching:
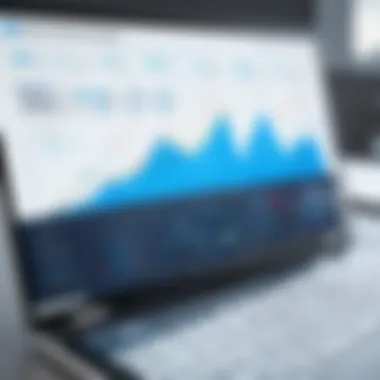
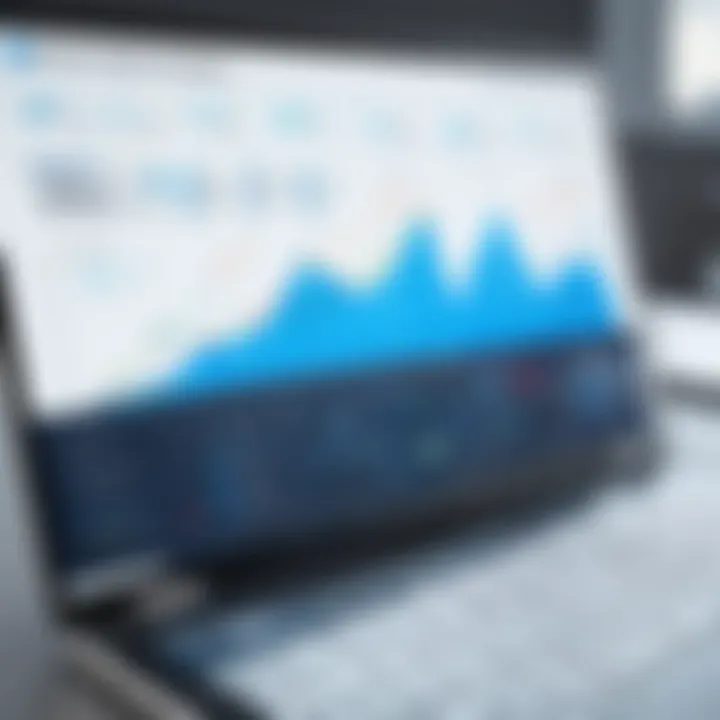
- Accuracy of identification: Reducing false positives is crucial.
- Data formats: Extensive variability requires preparatory normalization.
- Scalable systems: Economical in crafting systems for recurring data operations.
Machine Learning Techniques
Machine learning represents a progressive method for data enrichment. It enables systems to learn from patterns in data autonomously. By analyzing existing data to predict, categorize, or interpret, machine learning models can add depth to data without exhaustive manual programming.
Examples of application include customer segmentation and anomaly detection. Advanced models can learn as new data comes in, enhancing the capability to adapt to changing trends and conditions. However, model training requires robust data to be effective, and the intricacy increases with more sophisticated learning algorithms.
Benefits of Using Machine Learning:
- Adaptive processes: Quickly manage new trends.
- Data relevance: Help to spot features influencing specific outcomes.
- Increased accuracy: Diminish human error through learning from data.
Enriching data through various methods ultimately contributes to superior decision-making and strategic insights in the business environment. Choosing the best path of enrichment depends heavily on objectives, data conditions, and resource availability.
Challenges in Data Enrichment
Data enrichment can significantly transform how organizations use data. However, incorporating various enrichment techniques also brings its own share of challenges. Understanding these challenges is crucial for effective implementation. When data enters an organization, it comes with varying levels of accuracy, relevance, and completeness. Therefore, businesses need to undertake a strategic approach to ensure that enriched data serves its purpose effectively.
Data Quality Concerns
Data quality is a paramount element impacting data enrichment. Poor-quality data existing in databases can lead to flawed insights and, subsequently, misguided decision-making. Often, inconsistencies, missing values, or outdated information can multiply through the enrichment process, further deteriorating its integrity. Thus, organizations should build robust data quality assessments before adding layers of enrichment.
For sure, the financial cost of acting on corrupted data is enormous, especially for big businesses managing extensive data content. Regular audits and correcting errors can establish a more solid foundation. Adopting best practices in data governance plays a vital role in ensuring that data is collected, processed, and managed efficiently. Enhanced data quality evolves into additional valuable insights which, direct resultantly, then leads to better outcomes.
Privacy and Compliance Issues
As organizations look to enrich their data, compliance with data privacy regulations grows more critical. With laws such as the General Data Protection Regulation (GDPR) and the California Consumer Privacy Act (CCPA) enforcing strict rules regarding data usage and privacy, organizations must exercise caution. Data enrichment entails aggregating various data sources, which can easily lead to breaches, especially if sensitive information falls through mismanaged protocols.
Verifying that all data handling practices align with legal compliance is essential. Data controllers must prioritize anonymization and minimize data retention. By taking these steps, organizations not only uphold their legal obligations but establish trust with consumers, significantly enhancing their public reputation.
Integration Difficulties
Integration integrates an array of systems and data sources, thereby enhancing richness through a unified approach. However, achieving smooth integration encounters complex hurdles. Businesses often deal with disparate data formats, legacy systems, or incompatibilities within modern software and applications.
These adjustments can be technically demanding and may deter IT labor directly from helping to clarify enrichment challenges. To tackle this, having a clear strategy before integration helps support ongoing alignment. Standardizing data formats and implementing a focused API strategy can facilitate seamless data flow across platforms.
Ultimately, successful data enrichment relies as much on understanding its challenges as it does on employing the techniques themselves. Organizations must actively engage in addressing the concerns of data quality, compliance, and integration throughout the enrichment process.
Best Practices for Data Enrichment
Data enrichment is an essential process that adds value to raw data. Implementing best practices can enhance the effectiveness of this process. Best practices for data enrichment unify strategies that ensure better data quality and relevance.
By following these practices, organizations can achieve higher accuracy and usefulness of data. This fosters improved decision-making across the business. A clear framework guides teams in aligning enrichment activities with organizational goals. Furthermore, attention to best practices can minimize risks associated with bad data.
Establishing Clear Objectives
Creating specific goals for data enrichment can greatly influence outcomes. Clear objectives align stakeholders and data teams towards common aims. For instance, an organization may wish to improve customer profiles to personalize communications. Therefore, knowing exactly what to achieve directs focus on relevant enrichment strategies.
Key objectives evidence measurement: what data to enrich and why. Establishing urgent requirements can prioritize efforts, increasing overall efficiency. For example, if enhancing customer data leading to conversions is the goal, focusing on behavior and demographics is essential.
Consider these components when establishing objectives:
- Define what data sources to utilize.
- Identify data fields to enrich.
- Align with marketing or operational goals.
Ensuring Data Governance
Data enrichment is powerful, but it raises governance needs immensely. Data governance ensures responsible data handling and meets compliance standards. Best practices will incorporate policies guiding data collection and usage. This creates a balanced approach, prioritizing both enrichment benefits and data ethics.
Effective data governance can help manage data integrity, accuracy, and security. It also assures all stakeholders understand on roles as it relates to enrichment tasks. Organizations should establish data ownership and accountability across units.
Key focus areas include:
- Conducting regular audits of enriched data.
- Utilizing encryption and anonymization where required.
- Enforcing strict access policies to sensitive data.
Monitoring and Evaluating Enrichment Processes
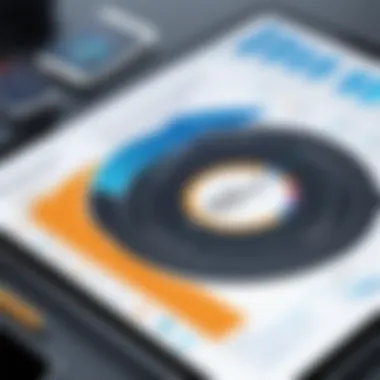
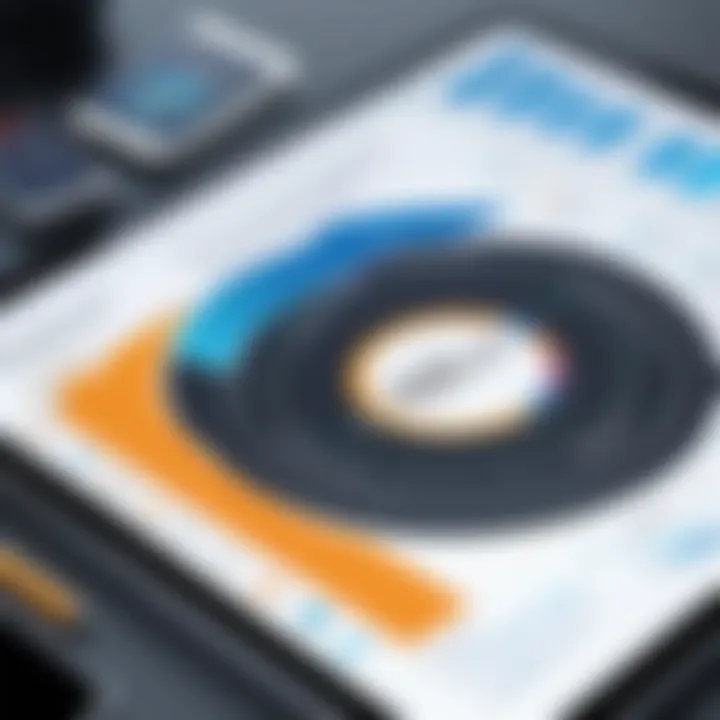
Effective monitoring is central for successful data enrichment. Continually evaluating enrichment processes ensures they are meeting objectives and producing noticeable results. This helps determine whether data projects yield expected outcomes or need adjustments.
Organizations should adopt quantitative metrics to gauge enrichment success. This involves key performance indicators for accuracy and relevancy of augmented data. Example KPIs can include:
- Percentage of data matches made successfully.
- User engagement levels based on enriched insights.
- Reduction rates in duplicitated entries or errors.
Utilizing analytics reduces failures by continually applying insight gained from previous efforts to new projects:
Impact of Data Enrichment on Business Intelligence
Data enrichment plays a crucial role in the domain of business intelligence. As organizations seek to extract meaningful insights from vast volumes of data, enriched data modalities serve as a foundation for informed decision-making. By augmenting raw data with additional context and attributes, businesses are better positioned to analyze trends and patterns effectively.
The integration of enriched data propels analytical processes, sharpens focus on customer behavior, and enhances predictive capabilities. Key benefits include improved clarity in data visualization, increased accuracy in reports, and superior communication across departments. Organizations empowered with data enrichment thus elucidate insights that cultivate growth, optimize operational efficiencies, all aim at leveraging competitive advantages.
Enhancing Analytical Capabilities
Enhanced analytical capabilities derive significant advantage from enriched data. Analysts confront challenges when dealing with unstructured or incomplete datasets. Data enrichment provides a structured, comprehensive lens to articulate complex queries. For instance, merging disparate data sources improves the robustness of analytics, allowing organizations to deploy multi-dimensional analyses. By converging internal, external, and public datasets, organizations surface interplay between variablers that might have remained hidden in isolated sets.
Many businesses adopt business intelligence tools such as Tableau or Power BI that fully exploit enriched data. This advancement empowers users to interact with data, facilitating personalized dashboards, customized reports, and intuitive visualizations. Furthermore, enriched data siliconizes machine learning models through refined features which often correlate with enhanced potential for actionable insights.
Improving Customer Insights
Understanding customers is paramount in sustaining competitive business strategies. Customer insights hinge on accurate, ongoing data aggregation that comprehends patterns across various channels. Data enrichment augments customer information repositories, coding deceptively simple interactions into complex behavioral profiles. Enriching customer transactions, demographic details, and engagement metrics allows the crafting of succinct marketing and sales cycles.
Analytical tools leverage these enriched insights to form actionable recommendations. The interoperability of data within Customer Relationship Management (CRM) platforms such as Salesforce puts organizations in a powerful position to tailor offering previous purchases, resulting in personalized customer experiences. Customers faced with services tailored to their specific needs display increased loyalty, leading to improved retention rates.
Supporting Predictive Analytics
Effective predictive analytics critically depends on the relevance and quality of input data. Leveraging data enrichment establishes precise baselines that amplify’s model performance. Predictive models work more effectively when trained on datasets that contain relevant attributes and indicators accumulated through the context of enriched information.
Implementing machine learning algorithms within a platform like TensorFlow requires enriched training datasets. These models predict future trends more accurately, providing valuable foresight into market needs and shifts. For example, augmenting sales data with seasonal trends supports stock forecasting, guiding decisions on production and inventory management. Consequently, rich datasets transcend historical evaluation, enabling organizations to iterate strategies preemptively.
By enriching the insights derived from data, organizations can navigate risks while maximizing opportunities, resulting in a more resilient business model in intensely competitive landscapes.
Future Trends in Data Enrichment
Data enrichment, essential for improving decision-making processes, remains dynamic. The continuing evolution of techniques and tools shapes the landscape of data usage across industries. Engaging with current trends allows organizations to stay competitive while addressing emerging challenges.
The Role of Artificial Intelligence
Artificial Intelligence is increasingly crucial in data enrichment strategies. It helps automate processes that can enhance both quality and efficiency. AI algorithms can recognize patterns and correlations that human analysis might miss. These advancements lead to smarter data preparation techniques. For example, customer segmentation, predictive modeling, and recommendation systems all rely on AI methodologies. They optimize responsiveness and precision in data projects.
AI-driven data enrichment improves the speed at which data is processed and refined. It provides insights that are more profound and actionable. Companies can achieve significant boosts in analytical capabilities, yielding better business outcomes. Moreover, AI can incorporate continual learning, improving over time through feedback and vast datasets. Scalability becomes smoother, making AI a timely partner in enrichment as data landscapes evolve.
Emerging Data Sources
Emerging data sources revolutionize how data is gathered and analyzed. Unlike traditional avenues like databases, businesses now use online platforms and Internet of Things devices for data. Social media channels, for example, provide real-time sentiment analysis which proves vital for market insights. These platforms yield rich, unstructured data that can encircle customer behaviors and trends.
Another source pertains to sensor data derived from smart devices. As smart technology proliferates, data from these sensors can greatly improve spatial and contextual insights. Rich geographical analysis through geospatial locations can bring about superior proximity-based services. The variety and volume require effective management, but the benefits are tangible when processed correctly.
Establishing pipelines that effectively integrate these new sources is crucial. Organizations must focus on improving connectivity and extracting meaningful narratives from heterogeneous data types.
Increased Focus on Data Privacy
The increased focus on data privacy marks a significant shift in data enrichment practices. With growing public concern around personal privacy and extensive use of data, complying with regulations like GDPR and CCPA is no longer optional. Organizations need to design their data enrichment processes ensuring prior consent and transparency to strengthen trust with users.
Privacy techniques such as data anonymization and encryption now play a pivotal role. As companies draw more data from diverse sources, they must balance quality improvements with users' rights. This can involve implementing stringent data governance frameworks.
Forward-thinking organizations recognize the need for a blended approach. Innovations in privacy-preserving machine learning create opportunities for effective enrichment without sacrificing individual privacy. The dialogue about data ethics must continue to elevate as a priority during data product development.
The complexity of future data operations hinges on prioritizing both enrichment and privacy.
Proper focus on these areas not only protects users but also cultivates public confidence, which ultimately boosts operational efficiency. Ultimately, embracing future trends in data enrichment aligns practices with sophisticated expectations set by both audience and regulatory bodies.
Epilogue
In the realm of data management, the conclusion represents a pivotal opportunity for reflection and synthesis. The importance of concluding an exploration of data enrichment techniques is twofold: to encapsulate key insights gained from the preceding sections and to highlight the long-term significance these methods can bring to organizations.
First and foremost, the article presents diverse data enrichment strategies that are essential for enhancing the quality and utility of data. Thus, enriching data not only leads to better accuracy and relevancy but also empowers decision-makers across industries to develop informed strategies. As businesses continue embracing data-driven cultures, the relevance of sophisticated data enrichment cannot be overstated.
Additionally, organizations that improve their data enrichment processes often experience social and operational benefits. These benefits can manifest as deeper customer insights, fine-tuned predictive analytics, and overall success in achieving defined objectives. Moreover, improving data quality amplifies the potential for innovation, especially through the usage of emerging technologies like machine learning and artificial intelligence. This article emphasizes the interconnectedness between comprehensive data understanding and systemic business growth.
Notably, ethical considerations around data privacy and compliance should also feature in discussions around data enrichment. Organizations must navigate these aspects diligently. Ongoing challenges and best practices align to fortify these concerns, promoting integrity within operations. Additionally, continue to evaluate the efficacy of processes, monitoring the impact of enriched data, subsequently adjusting strategies as necessary.