Data Fabric: A Key Framework for Data Management
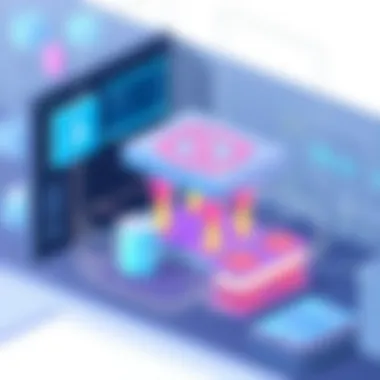
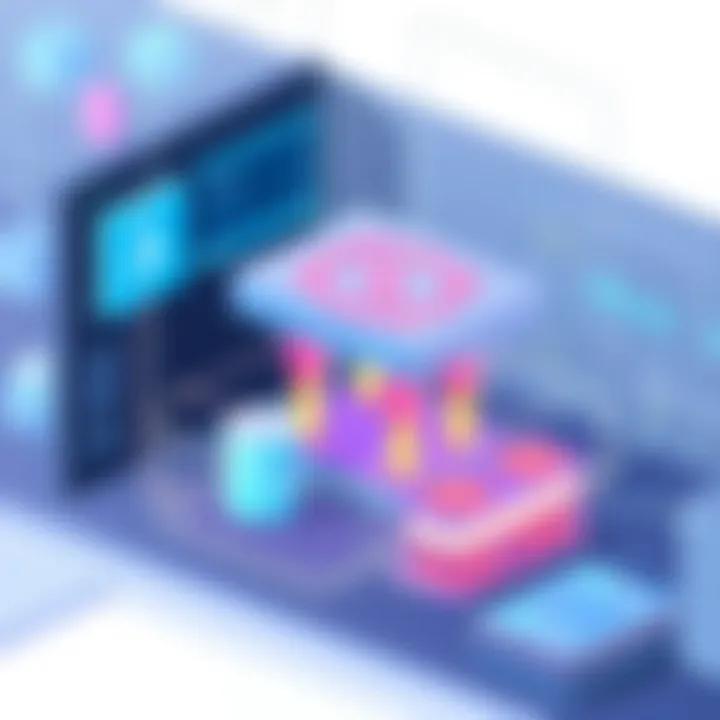
Intro
In today's digital environment, the way organizations manage their data can make or break their operational efficiency. As data continues to explode in volume and complexity, the need for effective management strategies has become more paramount than ever. Data fabric emerges as a crucial framework in this regard, offering a cohesive structure to handle the myriad of challenges organizations face.
This article aims to dissect data fabric in detail, illuminating its significance in the modern data management landscape. Not only does it tackle the hurdles that companies are grappling with today, but it also unravels the essential elements that define data fabric. Moreover, we will examine its pivotal role in bolstering data access and integration across diverse platforms.
The following sections will peel back the layers of this intricate topic, addressing its foundational concepts, industry trends, and practical applications. IT professionals, cybersecurity experts, and students will benefit from the comprehensive insights shared here. With that, letâs dive deeper into the core components that underpin data fabric and how they interact within the broader spectrum of data management.
Understanding Storage, Security, or Networking Concepts
When discussing data fabric, itâs vital to grasp the underlying concepts of storage, security, and networking. Each element interconnects to create an efficient data management system that can adapt to various challenges.
Intro to the Basics of Storage, Security, or Networking
At its core, storage refers to the method in which data is retained, whether thatâs on physical devices or in the cloud. Security encompasses the measures employed to safeguard data from unauthorized access or breaches. Lastly, networking relates to how systems communicate and share data efficiently.
Key Terminology and Definitions in the Field
- Data Fabric: A unified architecture that integrates disparate data sources.
- Cloud Storage: Data stored on remote servers accessed via the internet.
- Encryption: The process of encoding data for security.
- API (Application Programming Interface): A set of protocols for interacting with software components.
Overview of Important Concepts and Technologies
Understanding various technologies is crucial for effectively implementing a data fabric. For instance, technologies like data virtualization allow users to access data across various sources without the need for comprehensive duplication of data. Then there's microservices architecture, enabling the creation of modular software components that can streamline operations. Both are integral to supporting a robust data fabric.
As we continue this exploration, we will look closely at best practices, industry trends, real-world case studies, and more, all crucial for leveraging data fabric successfully.
Understanding Data Fabric
The realm of modern data management can often feel like walking through a labyrinth, where shadows of inefficiency loom large. To cut through the fog, a solid understanding of data fabric is imperative. This framework emerges as an essential instrument that helps organizations manage their data amidst growing complexities. By grasping this concept, IT professionals and cybersecurity experts alike can better navigate the overwhelming web of data integration, access, and governance challenges.
Definition and Core Concepts
At its heart, data fabric refers to an architecture that facilitates seamless data management across diverse locations and systems. Essentially, it connects various data services, integrating them into a single platform that allows for better manipulation and utilization. This cohesive management not just enhances data accessibility but aims to resolve many of the frustrations brought on by traditional data silos.
Moreover, there are a few core concepts to grasp:
- Data Integration: The ability to pull data from various sourcesâbe it cloud, on-premises, or hybrid environmentsâinto a unified view.
- Real-time Access: Offering up-to-the-minute data availability that is crucial for decision-making.
- Data Governance: Ensuring that data security, compliance, and quality standards are being upheld.
These components work harmoniously within the framework, contributing to the overall efficiency and effectiveness of data management practices.
Historical Context
To appreciate the significance of data fabric today, it's essential to look back at its evolution. Initially, organizations relied heavily on monolithic databases that were cumbersome and often isolated. This segregation of data resulted in countless issuesâdisparate systems, incomplete insights, and frustrated users trying to stitch together a cohesive understanding from fragmented information.
As the digital landscape transformed, so did the approaches to data management. The rise of big data brought new challenges, necessitating a shift toward more agile methodologies. In response, tech giants and data strategists began to develop integrated architectures that could handle an array of data sources while providing flexibility and scalability.
The concept of data fabric was formally introduced as a solution to bridge historical gaps in data management practices. By leveraging advanced technologies such as machine learning, organizations could finally overcome previous limitations, fostering environments ripe for collaboration and innovation.
Data fabric encompasses not only the technical layers of data connectivity but also a cultural shift toward data-driven decision-making. In a world where the essence of business strategy lies in data, understanding this framework is no longer optional; it's paramount for success.
The Data Landscape Today
In today's digital ecosystem, the data landscape is more complex than ever. Organizations find themselves grappling with enormous volumes and various types of data, making effective management a top priority. The landscape is not just about storage; itâs about extracting value from data while maintaining security and compliance. By understanding the nuances of the current data environment, businesses can leverage data fabric as a strategic response to contemporary challenges and opportunities.
Challenges in Data Management
Data Silos
Data silos represent a persistent headache for many organizations. This refers to instances where data is stored in isolated systems, leading to limited accessibility and inadequate sharing across departments. Data silos create barriers, preventing organizations from reaching their full potential in data utilization. One key characteristic of data silos is their ability to prevent collaboration, often resulting in duplicated efforts or inconsistent information.
The challenge arises because departments may use different systems and tools to store their data, leading to fragmentation that can affect decision-making processes. Not having a consolidated view of information can be detrimental. To tackle this issue, data fabric plays a significant role in integrating these silos. By fostering a unified approach, organizations can streamline operations and enhance real-time data access, which is crucial for responsive business strategies.
Legacy Systems
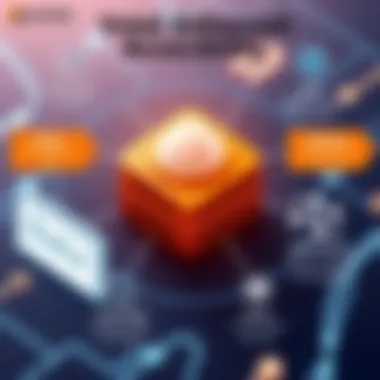
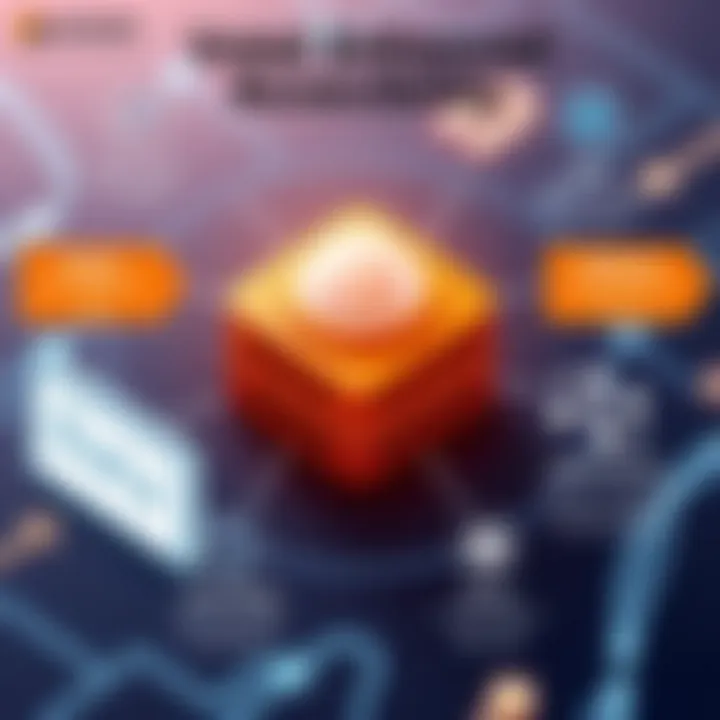
Legacy systems are another roadblock in effective data management. These are older computer systems or applications that may be slower, costly to maintain, and often lacking compatibility with modern solutions. The reliance on legacy systems could stifle innovation. Their key characteristic is that they've often been tailored to specific functions that can limit scalability or adaptability to new technologies.
The unique feature of legacy systems is their experience within an organization; they've been used for years, but this can become a double-edged sword. While they often have a wealth of historical data and established workflows ingrained into company culture, they can hinder integration attempts with newer data solutions. Consequently, moving towards a data fabric architecture that supports such integration can radically improve operational efficiency and agility.
Compliance Issues
Compliance issues are looming over every organization dealing with data management. Regulatory frameworks are tightening, and businesses must ensure that they adhere to laws like GDPR or HIPAA. A key aspect of compliance is accuracy in data reporting and processes that ensure data privacy. This is incredibly critical as non-compliance can lead to severe penalties or reputational damage.
The unique feature of compliance issues lies in the diversity of regulations across different regions and industries. This requires organizations to be adaptable and vigilant in their data governance practices. Data fabric can offer a robust approach to managing compliance by embedding security and governance in every phase of the data lifecycle, thereby facilitating easier tracking and auditing.
Emerging Trends
Cloud Adoption
Cloud adoption is gaining momentum as companies look to streamline data management processes. It allows organizations to store data in virtual environments, offering significant advantages such as scalability and cost-effectiveness. The distinctive characteristic of cloud adoption is the flexibility it provides, enabling businesses to adjust their resources according to demand. This empowers organizations to respond rapidly to changes and maintain ongoing operations without the physical limitations of traditional infrastructure.
An interesting angle about cloud adoption is how it integrates seamlessly with data fabric principles. By applying data fabric within cloud environments, businesses can create dynamic data pipelines that offer improved access to information and support real-time analytics. However, organizations often face challenges with data security and privacy when adopting cloud solutions. Such considerations are vital as they navigate this complex landscape.
Data Democratization
Data democratization is transforming the way teams access and utilize data. It involves empowering all employees, regardless of technical expertise, to leverage data for their work. Key to this concept is the development of user-friendly tools that simplify data analysis and reporting. The unique characteristic of data democratization is its focus on inclusivity, promoting a data-driven culture across the organization.
While innovations have made data access easier, it can lead to chaos if not managed correctly. Organizations must implement governance frameworks to ensure that while data is accessible, it is also secure and used responsibly. This illustrates how data fabricâs core principles align with fostering data democratization, enabling organizations to build trust in their data while maximizing its use.
"In today's fast-paced world, understanding the data landscape is critical for businesses striving to stay ahead and remain competitive."
In summary, the data landscape today is an intricate web of challenges and opportunities. By addressing data silos, legacy systems, and compliance issues while leveraging emerging trends like cloud adoption and data democratization, organizations can effectively navigate this landscape. Data fabric serves as a framework to integrate these elements, enabling businesses to thrive in a data-driven world.
Components of Data Fabric
In the realm of data management, the concept of data fabric serves as a vital framework that emphasizes the integration, governance, and management of data across diverse environments. Each component of this framework not only plays a pivotal role in unifying data sources but also directly addresses the challenges organizations face with data accessibility, quality, and security. Understanding these components is crucial, as they lay the foundation for effective modern data strategies.
Data Integration
Real-Time Data Access
Real-time data access stands out as a cornerstone in effective data integration within a data fabric. This aspect allows organizations to tap into data as it comes in, providing them with the most current insights possible. With businesses moving at the speed of light, relying on up-to-date information is no longer a luxury; itâs a necessity.
One key characteristic of real-time data access is its ability to deliver instantaneous analysis and reporting, greatly enhancing decision-making processes. This immediacy can be particularly beneficial in scenarios such as financial trading or customer support, where timely information can mean the difference between success and failure. However, implementing real-time access does come with its challenges, including the need for robust infrastructure and potential security risks that must be managed.
"Real-time data access is like a high-speed train that keeps businesses on track. Without it, organizations might as well be taking the scenic route, missing crucial opportunities along the way."
Batch Processing
On the other hand, batch processing represents a more traditional approach to data integration. Unlike real-time systems that pull data constantly, batch processing collects and processes data in defined groups at scheduled intervals. This method is particularly valuable for operations that do not demand immediate insights, for instance, monthly reporting or archiving processes.
What makes batch processing appealing is its efficiency in handling large volumes of data without overwhelming systems. Itâs like preparing a big meal: it takes a bit longer, but it allows for fine-tuning and cooking the ingredients just right. Nevertheless, while it minimizes the load on resources, it can lead to delays in accessing the most recent data, which could be a drawback in fast-paced environments.
Data Governance
Data Quality Management
When we talk about data governance, data quality management holds a vital position. It ensures the integrity and accuracy of data, which is essential for any organization aiming to rely on its data for critical decisions. High-quality data is the bedrock upon which analytics and insights are built.
An essential characteristic of data quality management is its focus on establishing consistent standards and processes for data entry and maintenance. This framework not only helps in rectifying inaccuracies but also in preventing future errors. In this modern era, where data-driven decision-making reigns, neglecting data quality can lead to misguided strategies and lost resources. Yet, achieving and maintaining high data quality is an ongoing challenge, often requiring a culture shift within organizations and dedicated resources to constantly oversee and refine data processes.
Access Control
Access control is another critical aspect of data governance that safeguards sensitive information within the data fabric. This control involves defining who has permission to access which data and under what conditions. Building strong access control systems helps mitigate risks, particularly with the increasing emphasis on privacy regulations.
The key feature of access control is its ability to enforce policies that restrict data access based on roles and responsibilities. This practice not only protects sensitive data from unauthorized access but also fosters trust among stakeholders. While this is undoubtly beneficial, crafting effective access control can be complex, often requiring meticulous planning and continuous updates to adapt to new threats and business needs.
Metadata Management
Metadata management serves as the unsung hero within the data fabric, playing a crucial role in organizing and cataloging data assets. This process involves documenting data sources, usage, and lineage, offering a roadmap of data flows and connections across the organization. By doing so, it enhances visibility and understanding of data structures, making it easier to navigate in the vast sea of information.
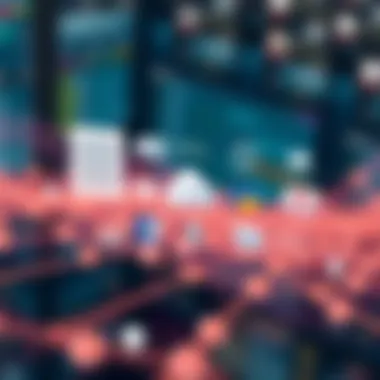
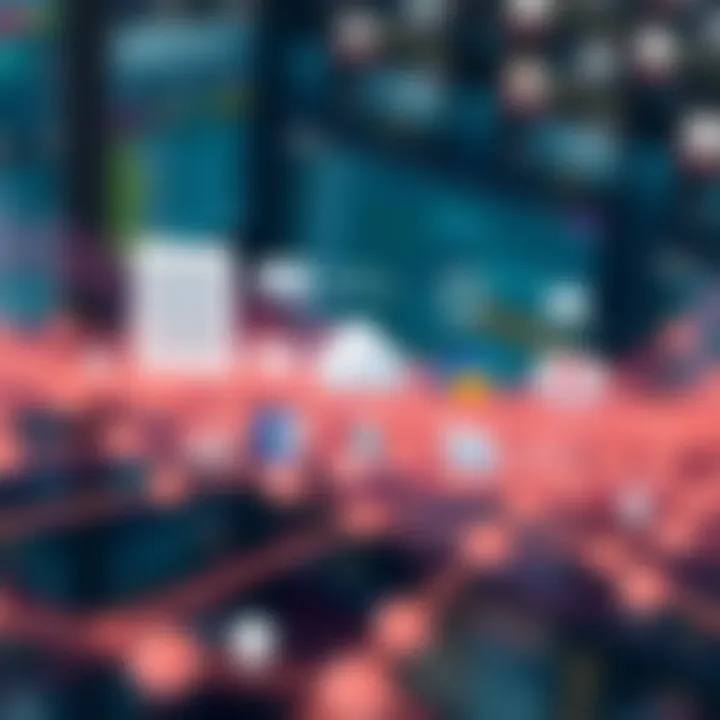
With effective metadata management, organizations can simplify the process of data discovery, enabling users to find and utilize data efficiently. Moreover, it can help drive compliance by ensuring that data lineage is clear, which is increasingly important in a landscape punctuated by regulatory scrutiny. Despite its advantages, the challenge lies in ensuring that metadata remains up-to-date and accurate, particularly as data environments evolve and grow more complex.
Benefits of Implementing Data Fabric
In the rapidly evolving landscape of data management, organizations are pumping significant resources into the quest for better solutions. Itâs here that the concept of data fabric shines, offering various advantages that address the complexities of modern data challenges. The benefits of implementing data fabric stretch beyond mere technological convenience, enhancing aspects of operational efficiency, security, and analytical capabilities.
Enhanced Data Accessibility
One of the standout features of data fabric is its ability to improve accessibility across disparate data sources. Instead of being locked away in silos, accessible data leads to streamlined processes. With a well-implemented data fabric, companies can expect to have data readily availableâallowing employees to find and use information when they need it.
- Cross-Platform Integration: Data fabric can integrate various systemsâbe it on-premise databases or cloud storageâproviding a unified view of information.
- User-Centric Approach: Easy access means that stakeholders from different departments, even those without technical expertise, can work with data efficiently. This democratization promotes collaboration and innovation.
By bridging gaps between different data sources, organizations can make decisions based on a holistic view, rather than piecing together fragmented data points.
Improved Data Security
The phrase "security is paramount" has never rung truer than in todayâs digital age. Security threats loom large, and as data breaches become more sophisticated, having a fortified approach to data management is vital. Data fabric allows organizations to enforce consistent security policies across all data environments, adding an important layer of protection.
- Centralized Governance: With centralized governance capabilities, data fabric enables organizations to establish strict access controls and compliance regulations uniformly across all datasets.
- Automated Threat Detection: Integrating AI and machine learning capabilities allows for real-time monitoring, thus identifying potential threats before they can escalate.
In a landscape where compliance regulations are constantly evolving, maintaining robust security measures is not just beneficialâitâs essential to safeguarding essential data assets.
Boosting Analytics and Insights
Data is only as good as the insights it can generate. Data fabric enhances an organizationâs analytical capabilities, enabling them to glean insights that drive strategic decision-making. With data handled efficiently, organizations can unlock a treasure trove of data insights in real-time, directly supporting data-driven strategies.
- Holistic View for Analytics: With all data linked through a data fabric, analytics become more comprehensive. Companies can analyze data from various perspectives without the hassle of data preparation.
- Facilitating Predictive Analytics: By leveraging machine learning algorithms, businesses can not only look at past data but also predict future trends, enabling proactive decision-making.
Technology and Tools Supporting Data Fabric
In the digital age, the way organizations manage data is paramount. Thriving in a landscape filled with multifaceted challenges demands a robust and agile approach. Expecting data to just flow smoothly without the right technology and tools is like asking for rain in a drought. Data fabric stands as the framework that integrates various data sources, and the tools that support it make all the difference.
Integration Platforms
Integration platforms are the backbone of data fabric. They ensure that disparate data sources communicate effectively, allowing businesses to operate more cohesively. Without these platforms, data can end up trapped in silos or stuck in legacy systems, making it hard to leverage all that valuable information. Tools like MuleSoft and Talend help organizations pull together data from multiple sources, regardless if they're in the cloud or on-site.
- Seamless Connectivity: These platforms make it easier to connect different applications and databases, providing a 360-degree view of the data landscape.
- Data Transformation: They facilitate not only data transfer but also data transformation, ensuring that information is relevant and ready for analysis.
- Scalability: As organizations grow, integration platforms can scale accordingly, capable of handling increased data loads without breaking a sweat.
Data Orchestration Tools
Data orchestration tools take the integration process a step further. They ensure the right data reaches the right place at the right time. Imagine a conductor leading an orchestra; without their guidance, the music would be chaos. Tools like Apache Airflow and Apache NiFi fall into this category, enabling organizations to automate data workflows.
- Automated Workflows: These tools manage complex data pipelines, automating routine tasks like data cleansing and movement.
- Monitoring and Management: They provide dashboards for real-time oversight, allowing teams to respond swiftly to any issues that arise.
- Flexibility: Organizations can adapt workflows on-the-fly, responding to changing business needs without significant downtime.
AI and Machine Learning
Artificial intelligence and machine learning represent the cutting-edge tools within the data fabric framework. They are not merely add-ons but integral components that enhance data usability and insights extraction.
Predictive Analytics
Predictive analytics is a powerful asset in the world of data management. It focuses on using historical data to forecast future trends, allowing businesses to make informed decisions. Tools such as IBM Watson and Microsoft Azure Machine Learning provide robust predictive capabilities.
- Anticipating Outcomes: By analyzing past data patterns, predictive analytics helps organizations foresee potential opportunities or threats.
- Decision Support: This tool aids in strategic planning, offering insights that support long-term goals.
- Limitations: However, the accuracy of predictions relies heavily on data quality; poor data can lead to misguided strategies.
Data Insights Generation
The generation of actionable data insights is arguably one of the most coveted benefits of data fabric. This process translates raw data into valuable insights, driving business intelligence efforts. Tools like Tableau and Power BI are frontrunners in this space, turning data into visual narratives.
- Visual Representation: Data insights generation often emphasizes visualization, making complex data understandable at a glance.
- Informed Decisions: By converting data into insights, organizations can base decisions on hard facts rather than gut feelings.
- Challenges: Still, generating insights consistently can be a struggle, particularly when dealing with vast data sets that require sophisticated analytical methods.
"Technology is not just a tool; it is a vital component that shapes how we manage our data and derive value from it."
Case Studies: Successful Data Fabric Implementations
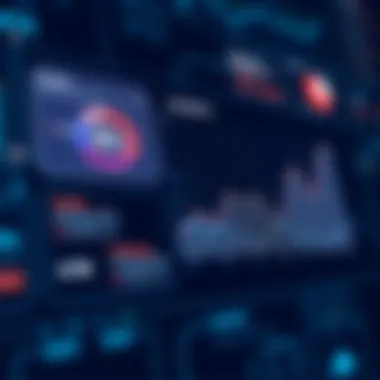
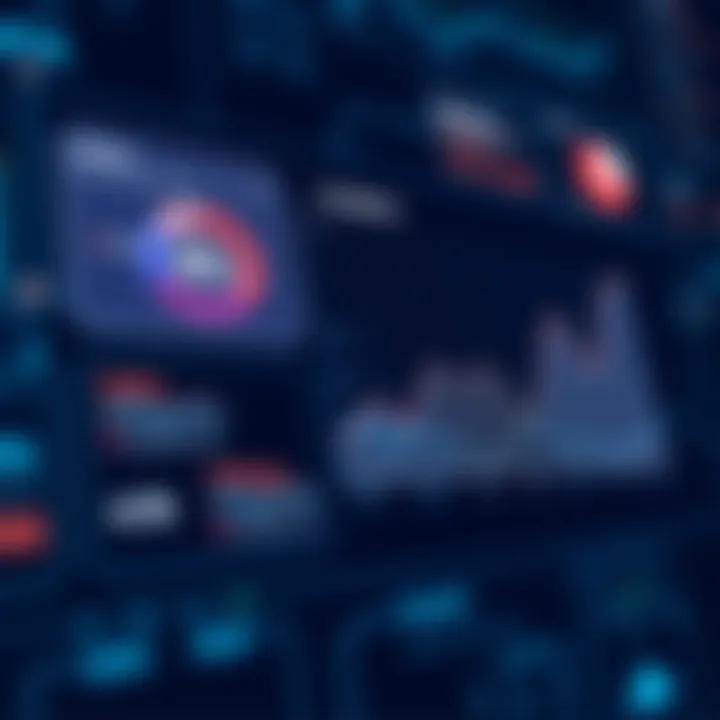
As organizations embrace data fabric as a critical framework for modern data management, real-world applications highlight its transformative impact. Examining case studies offers insights into practical implementations, benefits realized, and lessons learned. These narratives not only validate the theories but also elucidate the nuances of deploying such a complex architecture within a variety of sectors. By scrutinizing the financial and healthcare industries, we can glean valuable information about the successes and challenges faced during these data fabric journeys.
Financial Sector
The financial services industry, renowned for its stringent regulations and vast datasets, has much to gain from implementing data fabric. A notable example is a multinational bank that adopted a data fabric strategy to integrate its disparate data sources. The bank faced challenges with data silos resulting from multiple acquisitions over the years, leading to inefficiencies and compliance risks.
In implementing a data fabric, the bank aimed to achieve a single source of truth for all customer data. This was accomplished through the adoption of robust data integration and governance frameworks, allowing for real-time access to data across branches and functions. Key benefits observed include:
- Enhanced compliance: With a centralized data management system, compliance with regulations took a significant leap forward. The organization could quickly generate reports for regulatory bodies, adapting to ever-changing requirements efficiently.
- Improved customer insights: The integration enabled the bank to analyze customer behavior across various segments. This led to the development of tailored financial products and personalized services, ultimately enhancing customer satisfaction.
- Cost reduction: Streamlining the data processes reduced operational costs attributed to maintaining multiple, fragmented data systems.
"Data fabric fundamentally changed our approach to data management, aligning it with our business goals and compliance needs," said the bank's chief data officer.
These outcomes underscore the potential of data fabric in reshaping the financial landscape, presenting a model worth emulating.
Healthcare Industry
In the healthcare sector, the implementation of data fabric is not just about efficiency; it pertains directly to patient care and outcomes. A healthcare provider network embarked on a data fabric initiative to address challenges associated with disparate health records from various clinics and hospitals.
Prior to the data fabric deployment, patient data was often scattered, leading to delays in care and difficulties in coordination among healthcare providers. The integration of a data fabric allowed this network to consolidate and analyze patient information more effectively. Key achievements included:
- Enhanced patient care: By providing healthcare professionals with access to comprehensive patient histories, the ability to make informed decisions improved significantly. This wasnât just about accessing files faster; it meant better treatment pathways and outcomes for patients.
- Operational efficiency: The integration facilitated streamlined workflows, reducing redundancies and saving time for healthcare workers. Staff could now focus more on patient care rather than administrative tasks related to data retrieval and management.
- Cost-effective solutions: By minimizing duplication of tests and procedures through improved visibility of patient data, the healthcare provider realized substantial cost savings over time.
The fabric not only ensured data availability but fostered an ecosystem where health information is leveraged effectively, underscoring its value in improving healthcare delivery. As such, the case studies in finance and healthcare provide a blueprint for successful implementation of data fabric, critical for organizations looking to optimize their data strategies.
Future of Data Fabric
The inevitability of data evolution is upon us. As organizations strive for greater efficiency and insight, the future of data fabric becomes an essential focus in navigating this complex data landscape. With the rapid pace of change in technology and data management needs, organizations cannot afford to remain stagnant. Embracing data fabric frameworks is not just a strategy; itâs a necessity for survival and success.
Evolving Technologies
A closer look at evolving technologies reveals how interconnected they are with the concept of data fabric. Technologies like edge computing and Internet of Things (IoT) expand the boundaries of data management. As more devices come online, the data flow becomes nearly dizzying. The advent of edge computing means that data processing occurs closer to the source, minimizing latency and enhancing real-time analytics. This shift impacts how data fabric operates, pushing for an increase in integrations that can handle distributed data processing efficiently.
Additionally, developments in quantum computing may catapult data analysis capabilities to unprecedented levels. The ability to perform complex calculations at lightning speeds could redefine data management paradigms entirely. The integration of such advanced technologies into data fabric will inevitably adjust the very fabric of data management, making it more robust yet adaptable to the age of hyper-convergence.
"Data is the new oil, but without the right infrastructure, itâs just sludge."
â Unknown
As technology continues to evolve, organizations should consider how their data fabric can adapt to embrace newer tools and systems. This evolution will likely necessitate ongoing adjustments to governance policies and integrations to safeguard data quality amid exponential growth.
Predictions for Data Management Strategies
Looking ahead, numerous predictions arise regarding data management strategies. First, organizations should expect to see a significant shift toward AI-driven decisions. As artificial intelligence systems improve, the reliance on historical data will give way to predictive analytics. Companies might find themselves pivoting their strategies based on insights generated from advanced algorithms that learn and adapt over time.
Another prediction involves an increased emphasis on data literacy across all levels of an organization. This shift means not just having a one or two data specialists on staff; it requires everyoneâfrom executives to entry-level employeesâto understand data. Data fabric needs to be user-friendly enough to allow individuals to extract insights without a heavy tech background.
To summarize, the future of data fabric hinges on both technological evolution and cultural adaptation in organizations. Adapting to technological advancements and promoting data literacy will be key to creating a dynamic and responsive data management ecosystem. By preparing for these shifts, organizations will be in a prime position to leverage their data assets fully, turning potential challenges into significant opportunities.
Culmination
The conclusion of this article serves as a crucial wrap-up of the various facets of data fabric. It encapsulates the essence of why understanding this framework is vital in todayâs data-driven landscape. As organizations continue to grapple with massive datasets, a robust data management strategy becomes imperative. Here, we will break down the significance of several elements discussed throughout this article, along with the broader implications for businesses demanding efficiency and security in their operations.
In summary, the key elements of data fabric focus on integration, governance, and accessibility across diverse data sources. This framework does not merely offer a theoretical overview; it provides actionable strategies and tools. Below are highlighted benefits that underline the importance of implementing a data fabric strategy:
- Seamless Connectivity: Data fabric promotes essential connectivity between varied data sources, reducing operational silos that have hampered productivity.
- Enhanced Compliance: In an age where data breaches make headlines, a sound data governance framework informs better compliance management and risk mitigation.
- Empowered Analytics: With data democratization at its core, decision-makers at every level gain faster access to insightful analytics that drive informed choices.
"A well-executed data fabric strategy is not just a nice-to-have; itâs becoming increasingly important in maintaining competitive advantage."
As we navigate the future landscape of data management, keeping an eye on evolving technologies and methodologies will be paramount. Organizations willing to adopt and adapt to data fabric principles may find themselves at the forefront of innovation and efficiency.
Summary of Key Points
To encapsulate the narrative we've explored:
- Understanding Data Fabric: A conceptual framework that enhances data management via integration and real-time access.
- The Data Landscape Today: Organizations face challenges like data silos and legacy systems.
- Components of Data Fabric: Key components include data integration, governance, and metadata management.
- Benefits: Organizations can gain better accessibility, security, and insightful analytics through implementation.
- Technologies Supporting Data Fabric: Platforms designed for seamless integration are vital, influenced by advances in AI and machine learning.
- Future Directions: Continuous evolution expected as technologies progress and transform data management.
Final Thoughts on Data Fabric
In wrapping up our examination, it becomes clear that data fabric is not just a passing trend; it is an essential framework that organizations, whether in tech, finance, or healthcare, cannot overlook. The integration of various data sources leads to a holistic understanding of operations, enabling improved strategy formulation.
Whatâs noteworthy is that implementing data fabric goes beyond merely adopting new technologyâit necessitates a cultural shift within organizations. Embracing data as a strategic asset will enable teams to identify patterns, predict outcomes, and ultimately drive higher performance.
The road ahead will demand agility and innovation. Companies that grasp the tenets of data fabric can significantly position themselves for future success in an age where data reigns supreme. As we advance, the agility to adopt new data strategies will set apart the leaders from the laggards in an ever-competitive marketplace.