Maximizing Data Quality in Your Pipeline: A Comprehensive Guide to Data Optimization
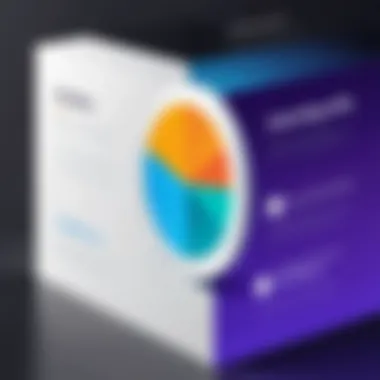
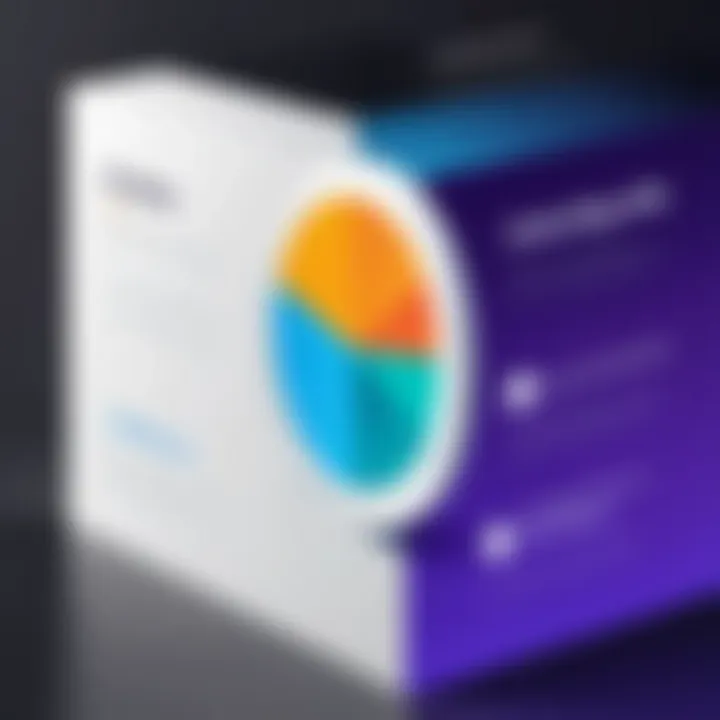
Understanding Data Quality Concepts
In the realm of data quality within pipeline operations, a foundational understanding is crucial for ensuring optimal performance and reliability. By delving into the key components that compose data quality and their relevance in the broader context of data processing, individuals can lay a robust groundwork for enhancing their pipeline operations. Concepts such as data integrity, accuracy, consistency, and completeness form the fundamental pillars of data quality, influencing the reliability and usability of the information within the pipeline.
Strategies for Enhancing Data Quality
To maximize data quality within pipelines, implementing effective strategies is paramount. From data cleansing techniques to establishing data governance frameworks, there are various approaches to elevate data quality standards. Leveraging tools for data profiling, monitoring, and validation can streamline processes and ensure that high-quality data is consistently injected into the pipeline. Additionally, cultivating a data-centric culture within the organization fosters a mindset where data quality is prioritized throughout all stages of the pipeline workflow.
Importance of Data Quality in Pipelines
Recognizing the significance of data quality is essential for driving efficiencies and enabling informed decision-making in pipeline operations. Poor data quality can lead to costly errors, inaccurate analysis, and impaired business outcomes. By upholding stringent data quality standards, organizations can enhance operational performance, gain a competitive edge, and build a foundation of trust with stakeholders. Prioritizing data quality within pipelines is not merely a best practice but a strategic imperative in today's data-driven landscape.
Integrating Data Quality into Pipeline Workflows
The seamless integration of data quality practices into pipeline workflows is imperative for sustained success. This involves embedding data quality checks at critical junctures, automating data profiling processes, and establishing proactive monitoring mechanisms. By weaving data quality considerations into the fabric of pipeline operations, organizations can mitigate risks, optimize performance, and harness the full potential of their data assets. Embracing a holistic approach to data quality integration cultivates a culture of continuous improvement and ensures that quality remains at the forefront of all data-related endeavors.
Evaluating Data Quality Solutions and Tools
When exploring data quality solutions and tools for pipeline optimization, it is vital to conduct a thorough evaluation to align with specific business needs. From data quality platforms offering comprehensive data cleansing capabilities to tools specializing in data deduplication and enrichment, the market provides a wide array of options. By conducting rigorous assessments, organizations can select tools that seamlessly integrate with existing systems, are scalable to future requirements, and align with budget considerations. Moreover, seeking vendor recommendations, analyzing user reviews, and conducting proof-of-concept trials can offer valuable insights for making informed decisions in selecting the most suitable data quality solutions for pipeline operations.
Conclusion
Introduction
In the realm of data management, the quest for optimal data quality remains a critical pursuit. Maximized data quality within pipeline operations paves the way for enhanced decision-making processes and improved business outcomes. Recognizing the pivotal role of data quality in shaping the foundation of organizational strategies, this comprehensive guide delves deep into the intricacies of ensuring data quality along the pipeline journey. By exploring the significance and impact of data quality, this guide provides invaluable insights for stakeholders aiming to bolster their data management processes. Let us embark on a journey towards unraveling the layers of maximizing data quality within your pipeline.
Understanding the Importance of Data Quality
The role of data quality in decision-making
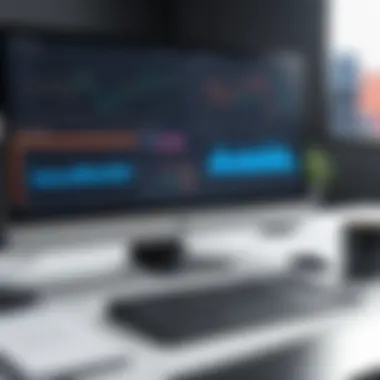
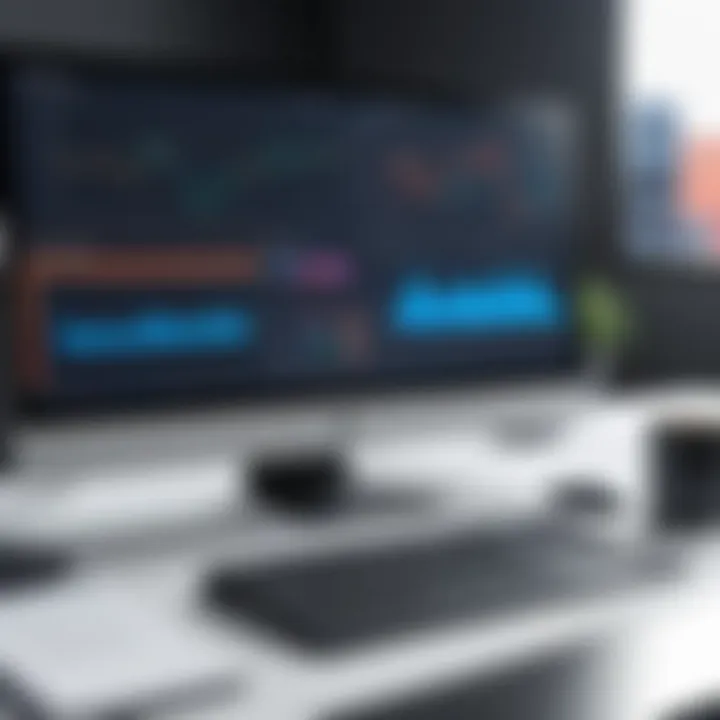
Data quality serves as the bedrock of informed decision-making processes within organizations. By ensuring that data is accurate, reliable, and consistent, stakeholders can derive meaningful insights to support strategic initiatives. The presence of high-quality data empowers decision-makers with the confidence to act upon insights, thereby mitigating risks and capitalizing on opportunities efficiently. Amidst a landscape inundated with data, the essence of data quality in decision-making cannot be overstated. It acts as a guiding light, illuminating the path towards informed and judicious choices, thereby enhancing the efficacy of organizational operations.
Impact of poor data quality on business operations
Conversely, poor data quality can serve as a hindrance to organizational efficiency and efficacy. Inaccurate, inconsistent, or incomplete data can lead to misguided decisions, operational inefficiencies, and compromised outcomes. The ramifications of deficient data quality reverberate across various facets of business operations, potentially resulting in financial losses, reputational damage, and missed opportunities. Recognizing the detrimental impact of poor data quality underscores the importance of vigilance and proactiveness in maintaining a high standard of data integrity within organizational frameworks.
Overview of Data Quality Pipeline
Definition and components of a data quality pipeline
The data quality pipeline encompasses a series of processes and mechanisms designed to ensure the reliability and accuracy of data throughout its lifecycle. From data collection to transformation and validation, each component of the data quality pipeline plays a crucial role in upholding the integrity of organizational data assets. By defining clear protocols and standards for data quality maintenance, organizations can streamline their data management practices and cultivate an environment conducive to data-driven decision-making. Embracing the definition and components of a data quality pipeline signifies a commitment to data excellence and operational efficiency.
Key benefits of implementing a data quality pipeline
The implementation of a data quality pipeline brings forth a multitude of benefits for organizations seeking to optimize their data processes. From improved data accuracy and consistency to enhanced regulatory compliance and stakeholder trust, the advantages of a robust data quality pipeline are multifaceted. By embedding stringent quality control measures and protocols within the data pipeline, organizations stand to gain a competitive edge in a data-driven landscape. Embracing the key benefits of a data quality pipeline sets the stage for organizational success and resilience amidst dynamic market conditions.
Building a Robust Data Quality Framework
In the realm of data management, constructing a robust data quality framework holds paramount importance. This segment is pivotal in ensuring that data within the pipeline meets the highest standards of accuracy and reliability, essential for informed decision-making processes and operational efficiency. By focusing on elements such as data collection and ingestion, data cleansing techniques, and data transformation strategies, organizations can streamline their data processes and enhance overall performance. The benefits of establishing a strong data quality framework extend beyond mere data integrity, encompassing improved organizational outcomes, enhanced customer satisfaction, and heightened competitiveness in the market.
Data Collection and Ingestion
Ensuring data accuracy during collection
Addressing the accuracy of data during the collection phase is a critical aspect of building a reliable data quality framework. It involves implementing rigorous measures to verify and validate incoming data, ensuring that it is free from errors and inconsistencies. By adhering to best practices such as data validation checks, data profiling, and data governance protocols, organizations can significantly enhance the trustworthiness and relevance of their data assets. The meticulous attention to data accuracy during collection serves as the foundation for downstream data processes, ultimately impacting the quality and efficacy of analytical outputs within the pipeline.
Best practices for data ingestion
Efficient data ingestion practices play a pivotal role in the seamless flow of data within the pipeline. Emphasizing the adoption of standardized data formats, data enrichment techniques, and data normalization processes can streamline the integration of diverse data sources. By adhering to best practices for data ingestion, organizations can reduce data silos, improve data consistency, and accelerate the speed at which data is processed and analyzed. Moreover, employing scalable data ingestion frameworks enables organizations to adapt to evolving data requirements and handle increasing data volumes with agility and precision.
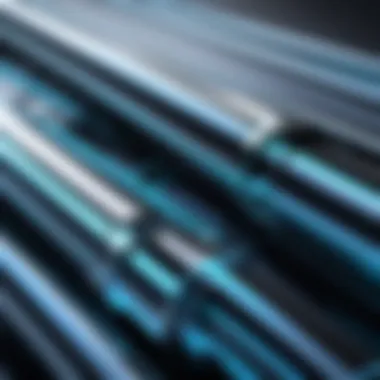
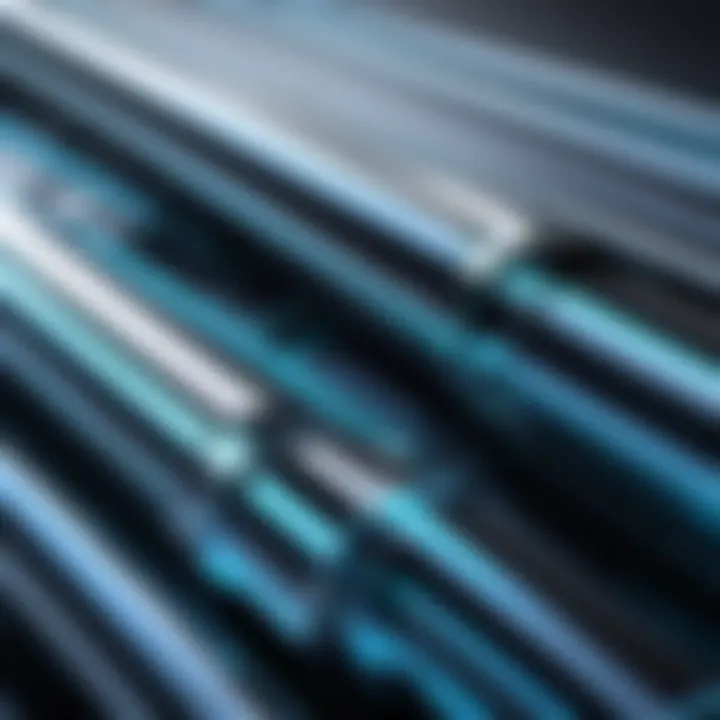
Data Cleansing Techniques
Identifying and correcting data errors
The process of identifying and rectifying data errors is a fundamental component of data cleansing techniques, aimed at improving the overall quality and reliability of data assets. By leveraging advanced data profiling tools, anomaly detection algorithms, and data cleansing algorithms, organizations can effectively pinpoint errors and anomalies within their datasets. The systematic elimination of inaccuracies and inconsistencies ensures that data remains pristine and fit for purpose, instilling confidence in decision-making processes and downstream analytics.
Implementing automated data cleansing processes
Automating data cleansing processes significantly enhances the efficiency and effectiveness of data quality initiatives. By utilizing intelligent algorithms for data deduplication, error detection, and outlier removal, organizations can expedite the cleansing process and reduce manual intervention. Automated data cleansing not only accelerates data preparation tasks but also minimizes the risk of human errors and oversight, leading to higher productivity and data quality standards within the pipeline.
Data Transformation Strategies
Converting data into usable formats
The conversion of raw data into actionable insights requires robust data transformation strategies that focus on standardization, normalization, and structuring of data formats. By standardizing data attributes, applying data enrichment techniques, and aligning data structures across disparate sources, organizations can derive meaningful insights and facilitate data-driven decision-making. Converting data into usable formats not only enhances data accessibility and interoperability but also empowers organizations to leverage the full potential of their data assets for strategic and operational purposes.
Integration of data from multiple sources
Integrating data from multiple sources presents unique challenges and opportunities for organizations seeking to maximize data quality and relevance. By employing data integration tools, schema mapping techniques, and data consolidation strategies, organizations can harmonize disparate data sets and derive cohesive insights from diverse sources. The seamless integration of data fosters a holistic view of organizational data assets, enabling more informed decision-making, trend analysis, and predictive modeling capabilities. Moreover, integrating data from multiple sources enhances data completeness and accuracy, laying the foundation for robust analytics and actionable intelligence within the data pipeline.
Ensuring Data Quality Assurance
Ensuring Data Quality Assurance is a critical aspect within the realm of data management and pipeline operations. In this article, we delve into the intricate details of establishing and maintaining high standards of data quality assurance. By implementing robust data quality assurance measures, organizations can enhance decision-making processes, streamline operations, and gain a competitive edge in the data-driven landscape. Through a comprehensive exploration of Ensuring Data Quality Assurance, readers will gain valuable insights into the significance, benefits, and considerations associated with this pivotal component of data optimization.
Implementing Data Quality Metrics
Defining measurable data quality parameters
Defining measurable data quality parameters plays a pivotal role in assessing and ensuring the accuracy, completeness, and consistency of data within the pipeline. By establishing clear and quantifiable criteria to evaluate data quality, organizations can effectively measure and track the performance of their data processes. This aspect of data quality metrics contributes significantly to the overarching goal of optimizing data quality, allowing stakeholders to identify areas for improvement and implement targeted enhancements. The key characteristic of defining measurable data quality parameters lies in its capacity to provide tangible and actionable insights into the quality of data being processed. By defining specific metrics and benchmarks for data quality, organizations can systematically enhance their data management practices and make informed decisions based on reliable data. The unique feature of defining measurable data quality parameters lies in its ability to bridge the gap between data quality goals and operational realities, enabling organizations to align their objectives with concrete and measurable outcomes.
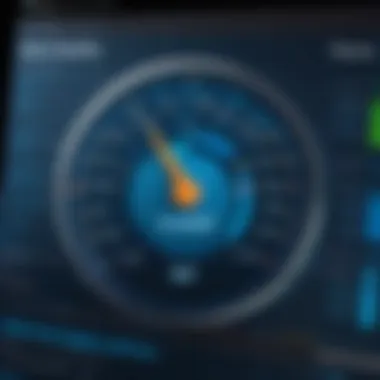
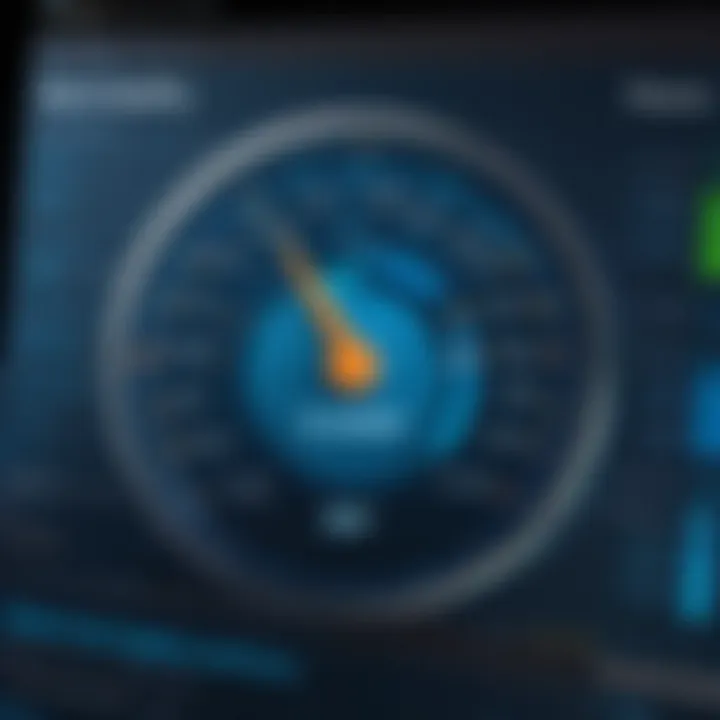
Monitoring and evaluating data quality metrics
Monitoring and evaluating data quality metrics are essential components of a comprehensive data quality assurance framework. By continuously tracking and analyzing data quality metrics, organizations can proactively identify inconsistencies, anomalies, or areas of improvement within their data pipeline. This proactive approach to data quality monitoring enables stakeholders to address potential issues in real-time, mitigate risks, and uphold the integrity of their data assets. The key characteristic of monitoring and evaluating data quality metrics lies in its ability to provide continuous feedback on the performance and reliability of data processes. By monitoring key metrics such as data accuracy, completeness, and timeliness, organizations can stay ahead of potential challenges and maintain a high standard of data quality. The unique feature of monitoring and evaluating data quality metrics lies in its iterative and data-driven approach to quality assurance, empowering organizations to make data-driven decisions and optimizations based on real-time insights.
Continuous Improvement Strategies
Feedback loop for enhancing data quality
The feedback loop for enhancing data quality serves as a fundamental mechanism for iterative refinement and enhancement within the data quality assurance process. By establishing a feedback loop, organizations can systematically capture insights, suggestions, and learnings from data operations, stakeholders, and external sources to enhance their data quality practices. This continuous feedback mechanism enables organizations to adapt to changing requirements, address emerging challenges, and drive continuous improvement in data quality. The key characteristic of the feedback loop for enhancing data quality lies in its capacity to foster a culture of learning, adaptation, and innovation within the data management ecosystem. By soliciting and integrating feedback from various sources, organizations can enhance their data quality processes, anticipate future needs, and align their strategies with evolving data requirements. The unique feature of the feedback loop for enhancing data quality lies in its ability to create a closed-loop system of refinement and optimization, where every insight and input contributes to the overarching goal of improving data quality.
Adapting to evolving data quality standards
Adapting to evolving data quality standards is a dynamic and proactive approach to maintaining data quality excellence in rapidly changing environments. In the era of big data and digital transformation, organizations must continuously adapt their data quality standards to meet evolving regulatory, technological, and operational demands. By staying abreast of emerging trends, best practices, and industry benchmarks, organizations can ensure that their data quality processes remain agile, relevant, and effective. The key characteristic of adapting to evolving data quality standards lies in its responsiveness to external influences and internal feedback loops. By embracing change and innovation in data quality management, organizations can future-proof their data processes, mitigate risks, and capitalize on new opportunities for advancement. The unique feature of adapting to evolving data quality standards lies in its strategic and forward-thinking approach to data quality optimization, allowing organizations to stay ahead of the curve and drive continuous innovation in data management.
Optimizing Data Quality Processes
In this section, we will delve into the critical importance of optimizing data quality processes within your pipeline operations. Maximizing data quality is not just a goal but a necessity in today's data-driven world. By optimizing data quality processes, organizations can ensure the accuracy, reliability, and effectiveness of their data analytics. Whether it is data cleansing, transformation, or integration, every step plays a vital role in enhancing the overall quality of the data ecosystem. Effective data quality processes lead to better decision-making, improved operational efficiency, and increased trust in data-driven insights.
Utilizing Data Quality Tools
Overview of popular data quality tools
When it comes to optimizing data quality processes, leveraging the right tools is paramount. Popular data quality tools offer a wide range of functionalities, from data profiling to anomaly detection, to ensure that data is cleansed and transformed effectively. These tools provide insights into data quality issues, automate repetitive tasks, and enable data quality monitoring throughout the pipeline. One notable characteristic of popular data quality tools is their user-friendly interfaces and robust functionalities that cater to various data processing needs. Choosing the right tool can streamline data quality processes, reduce manual efforts, and enhance the overall efficiency of data operations.
Selecting the right tool for your pipeline
Selecting the right data quality tool for your pipeline requires a careful evaluation of your organization's specific needs and requirements. The chosen tool must align with your data quality objectives, integrate seamlessly with existing systems, and offer scalability for future growth. Whether you opt for open-source solutions or commercial platforms, the key is to choose a tool that not only meets your current data quality challenges but also adapts to evolving data requirements. The unique feature of selecting the right tool lies in its ability to customize data quality checks, automate validation processes, and provide actionable insights for continuous improvement. However, it's essential to weigh the advantages and disadvantages of each tool to make an informed decision that aligns with your data quality strategy.
Automation of Data Quality Checks
Implementing automated data validation processes
Automation plays a pivotal role in enhancing data quality checks by reducing errors, improving efficiency, and accelerating decision-making processes. Implementing automated data validation processes enables real-time data monitoring, instant error detection, and proactive issue resolution. The key characteristic of automated data validation is its ability to enforce data quality standards consistently across all data touchpoints, ensuring data integrity and reliability. By automating data validation, organizations can minimize human intervention, optimize resource utilization, and streamline data quality assessments for better operational outcomes.
Streamlining data quality assessments
Streamlining data quality assessments involves creating a structured framework for evaluating data quality metrics, identifying anomalies, and implementing corrective actions swiftly. This process enhances the overall effectiveness of data quality management by standardizing quality metrics, establishing performance benchmarks, and facilitating continuous improvement. The unique feature of streamlining data quality assessments lies in its capacity to align data quality objectives with business goals, foster collaboration among cross-functional teams, and drive data quality excellence. While streamlining data quality assessments offers numerous advantages in terms of efficiency and accuracy, organizations must also be mindful of potential challenges such as tool compatibility, resource constraints, and data governance issues.

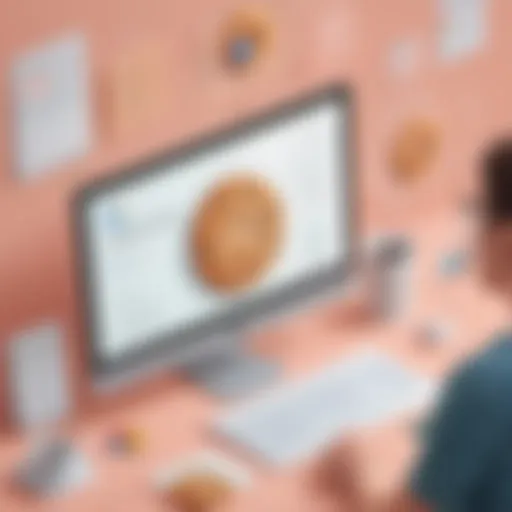