Understanding Enterprise Data Strategy for Success
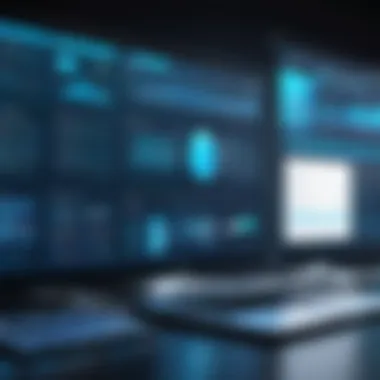
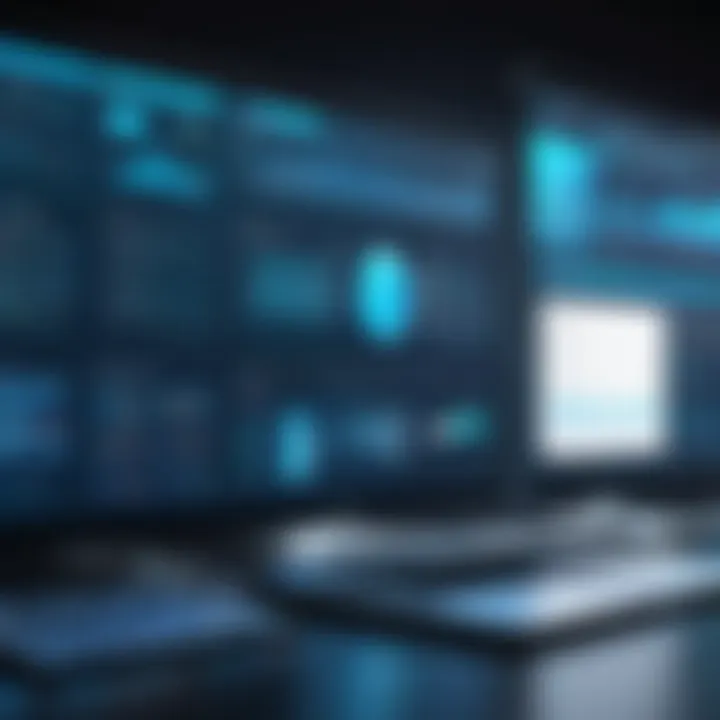
Intro
Exploring data strategy encompasses aspects of data governance, methodology, and technology. Each element plays a role in establishing a solid framework for managing data as a valuable asset. With the swift evolution of technology, staying abreast of industry trends and developments is essential for IT professionals, decision-makers, and anyone interested in nurturing a data-driven environment.
This article dissects the relevant components of enterprise data strategy, highlighting the importance of storage, security, and networking concepts vital to best practices and innovations.
Understanding Storage, Security, or Networking Concepts
Prelims to the basics of storage, security, or networking
Every organization relies on three core pillars: storage, security, and networking. Storage refers to how data is retained and retrieved over time. Effective storage solutions drive efficiency and access. Security ensures that data remains protected from threats. Diverse methods exist to mitigate risks. Finally, networking involves connecting systems and users. This connection is pivotal for seamless data flow.
Key terminology and definitions in the field
Understanding the terminology related to these concepts is vital. Here are some key terms:
- Data Lake: A centralized repository that stores structured and unstructured data.
- Encryption: A method of securing data by converting it into a coded format.
- Bandwidth: The maximum rate of data transfer across a network path.
Overview of important concepts and technologies
With the constant evolution in technology, we see various solutions emerging. Some notable ones include:
- Cloud Storage: This allows for scalable storage solutions, giving access from any location.
- Firewalls: Essential for blocking unauthorized access and protecting internal networks.
- Software-Defined Networking (SDN): Focuses on decoupling control from hardware, offering flexibility in networks.
Best Practices and Tips for Storage, Security, or Networking
Tips for optimizing storage solutions
Organizations should prioritize effective storage solutions by:
- Implementing Tiered Storage: Use a combination of storage types based on data usage patterns.
- Regular Data Audits: Periodically review data for relevance and conformity to compliance requirements.
Security best practices and measures
For enhancing data security, consider:
- Regular Updates and Patching: Keeping systems updated minimizes vulnerabilities.
- User Training: Educating employees on security practices is essential.
Networking strategies for improved performance
To boost networking efficiency:
- Optimize Traffic Flow: Identify and eliminate bottlenecks.
- Utilize Virtual Private Networks (VPNs): Secure remote access to the network.
Industry Trends and Updates
Latest trends in storage technologies
Recent trends include:
- Edge Computing: Bringing computation closer to data sources for quick retrieval and processing.
- AI-Powered Storage Management: Using machines to manage storage allocations intelligently.
Cybersecurity threats and solutions
Emerging threats require constant vigilance. Some notable ones:
- Ransomware: Targeting sensitive data to extort organizations.
- Phishing Attacks: Manipulating users into revealing confidential information.
Solutions to these threats can range from advanced threat detection software to user awareness training programs.
Networking innovations and developments
Innovation in networking includes:
- 5G Technology: Providing faster and more reliable connections.
- Network Functions Virtualization (NFV): Virtualizing network services for agility and efficiency.
Case Studies and Success Stories
Real-life examples of successful storage implementations
Consider a financial organization that adopted cloud storage solutions, which allowed them to manage data better with scalability and cost-effectiveness.
Cybersecurity incidents and lessons learned
Take the example of the Equifax breach. The company learned the importance of timely software updates and robust incident response plans.
Networking case studies showcasing effective strategies
An e-commerce giant optimized its network architecture using SDN. This facilitated seamless transactions during peak periods, enhancing customer satisfaction.
Reviews and Comparison of Tools and Products
In-depth reviews of storage software and hardware
Amazon S3 is known for its scalability and strong security measures. It offers variety for businesses of all sizes.
Comparison of cybersecurity tools and solutions
Compare solutions like Norton and McAfee regarding their features and customer support,
Evaluation of networking equipment and services
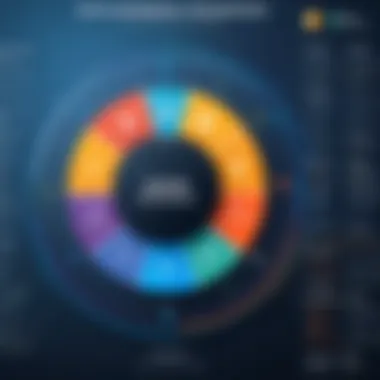
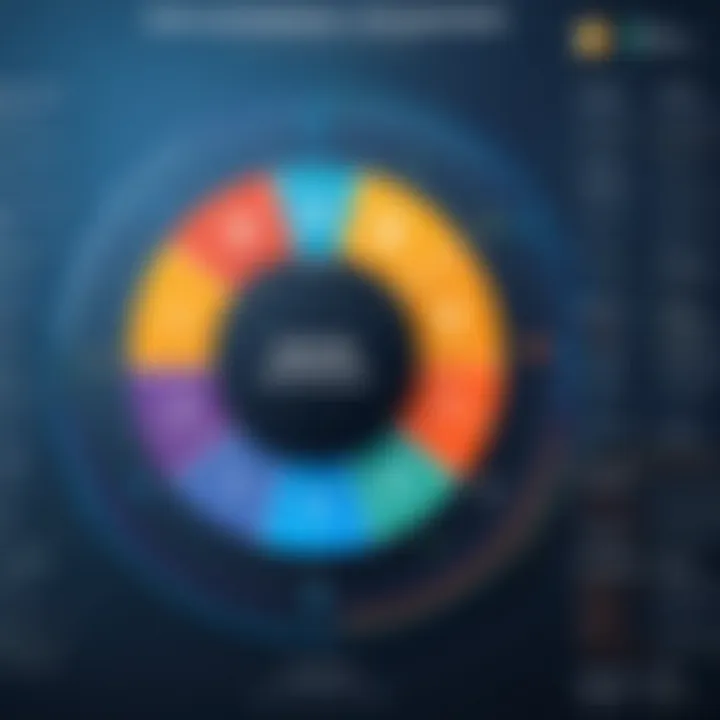
Cisco routers are widely recognized for their reliability and advanced performance in enterprise settings.
By delving into these topics, we aim to provide insights necessary for fostering a robust data strategy aligned with modern initiatives.
Intro to Enterprise Data Strategy
In today’s digital landscape, the term "Enterprise Data Strategy" has gained significant traction among organizations striving for competitive advantage. The importance of establishing a solid data strategy cannot be overstated as it serves as the backbone of informed decision-making, operational efficiency, and long-term growth. As businesses increasingly rely on data-driven insights, a well-defined data strategy becomes not only beneficial but essential for success.
An effective data strategy encompasses several key elements that help organizations manage their data assets systematically. These elements include data governance, data quality management, and data architecture design, among others. Collectively, they form a framework that supports the organization’s objectives while addressing challenges that arise from data fragmentation and inefficiency.
For organizations that seek to leverage the full potential of their data, aligning their data strategy with business goals is vital. This alignment ensures that data practices contribute directly to business outcomes, creating value and fostering innovation. Furthermore, understanding the pivotal role data plays allows companies to navigate the complexities of data management with ease.
In this article, we will explore these themes in-depth, highlighting the relevance of enterprise data strategies, their components, and the methodologies that enhance their effectiveness. Through this comprehensive guide, IT professionals, decision-makers, and other stakeholders will gain insights into creating and sustaining a robust data-driven environment.
Definition and Importance
An enterprise data strategy is a comprehensive approach to managing an organization’s data assets. It encompasses the processes, technologies, and policies required to effectively collect, store, and analyze data. The primary aim of this strategy is to enable organizations to harness data for better decision-making and operational efficiency.
The significance of defining a clear data strategy is multifaceted. Firstly, it helps establish a governance structure that ensures data integrity and compliance with regulations. This governance is crucial, given the increasing scrutiny of data privacy laws and regulations worldwide. Secondly, organizations with a well-implemented data strategy can manage their resources more effectively, identify key performance indicators, and align data-related initiatives with their broader business objectives.
Role in Modern Organizations
In modern organizations, data is often regarded as a strategic asset. This view emphasizes the need for an enterprise data strategy that not only manages data but also leverages it for competitive advantage. The role of data becomes even more pronounced as businesses adopt advanced technologies such as artificial intelligence and machine learning, which rely heavily on high-quality data for analytics and insights.
Organizations today face the challenge of data overload, with vast quantities of information generated daily. Without a clear strategy, this data becomes overwhelming, rendering it less useful. A comprehensive data strategy streamlines data collection and analysis, leading to more informed decisions.
Moreover, it creates a culture of data-driven decision-making. When employees at all levels can access and utilize data, the organization as a whole benefits from heightened innovation and adaptability. Promoting a data-centric culture requires training and clear communication regarding the tools and processes in place.
"Data-driven decisions can lead to better business outcomes, higher efficiency, and new opportunities for growth."
Components of an Effective Data Strategy
An effective data strategy is crucial for organizations seeking to leverage their data assets to drive decision-making and innovation. The components discussed here lay the groundwork for a structured approach to managing data. By focusing on these elements, organizations can enhance their data management capabilities, ensuring that their data serves not only regulatory purposes but also strategic objectives. Proper integration of these components leads to efficiency and improved data-driven outcomes.
Data Governance Framework
A data governance framework establishes the policies, standards, and processes for managing data within an organization. It defines who can make decisions regarding data and outlines the responsibilities related to data handling, protection, and quality. A robust governance framework helps organizations ensure compliance with regulations, such as the General Data Protection Regulation (GDPR) or the Health Insurance Portability and Accountability Act (HIPAA).
Benefits of an effective data governance framework include:
- Clarity in data ownership and accountability.
- Enhanced data quality and integrity through defined standards.
- Improved trust in data as a reliable source for decision-making.
Implementing a systematic approach to data governance means that stakeholders across departments understand their roles in maintaining data quality. This creates a culture of responsibility and respect for data as a valuable asset.
Data Quality Management
Data quality management focuses on ensuring that data is accurate, complete, reliable, and timely. Poor quality data can lead to detrimental decisions, impacting an organization’s operations and reputation. Thus, managing data quality is a vital aspect of any data strategy.
Key practices for maintaining data quality include:
- Regular data assessments and cleaning.
- Establishing data entry standards to reduce human error.
- Implementing automated systems for data validation.
Organizations should emphasize a continuous improvement mindset regarding data quality. Investment in quality management tools can facilitate this process, ensuring that decisions are based on high-quality data.
Data Architecture Design
Data architecture design involves creating a blueprint for how data is to be collected, stored, accessed, and utilized across an organization. This aspect is crucial as it influences how data can be integrated and analyzed effectively. A well-designed data architecture allows seamless flow of data between systems and promotes collaboration among stakeholders.
Considerations in data architecture include:
- Selection of appropriate data storage solutions, such as databases or data lakes.
- Implementation of standardized data models to streamline data integration.
- Ensuring scalability to accommodate future data growth.
Architectural designs should be adaptive, allowing for new technologies or methodologies to be integrated without significant disruption. Clarity in this design promotes smoother operations and better data management.
Data Lifecycle Management
Data lifecycle management refers to the processes that govern how data is managed from its creation to its deletion. Understanding the lifecycle of data helps organizations maximize their data investments while ensuring compliance with data retention regulations.
The key stages in the data lifecycle include:
- Data Creation: How data gets generated and captured.
- Data Storage: Choosing suitable storage solutions.
- Data Usage: Access and sharing protocols.
- Data Archiving: Procedures for archiving unused data.
- Data Deletion: Safe deletion methods conforming to legal requirements.
Each stage must be managed effectively to prevent data loss and ensure security. Establishing clear policies at each stage allows for responsible data management, fostering trust in data's value within the organization.
Aligning Data Strategy with Business Objectives
Aligning data strategy with business objectives is crucial for any modern organization. A well-defined data strategy can harness the potential of data to meet market demands and drive performance. When data management is aligned with business goals, organizations can make informed decisions that fuel growth and enhance competitive advantage.
Understanding Business Needs
To align data strategy effectively, one must first understand the specific needs of the business. This involves identifying key business goals and how data can support them.
- Conducting interviews or workshops with various stakeholders can uncover insights into their unique requirements.
- Assessing market trends is also vital. Businesses operate in a dynamic environment where adaptation is necessary. The data strategy should not only reflect current needs but also anticipate future trends.
- Engaging with customers helps organizations understand external expectations. Customer feedback can directly influence data collection priorities, enabling organizations to align offerings with market demand.
Creating Value through Data Insights
Data insights represent opportunities for creating value within an enterprise. When organizations leverage data effectively, they can uncover patterns, identify risks, and discover new opportunities. This capability is integral to achieving alignment between data strategy and business objectives.
- Implementing advanced analytics can transform raw data into actionable insights. Business leaders can then make data-driven decisions that align with strategic goals.
- Encouraging a culture of data literacy within the organization empowers employees to utilize data in their daily tasks.
- Sharing data insights across departments promotes transparency and facilitates collaborative efforts, ultimately enhancing performance and driving growth.
Performance Metrics and KPIs
To gauge the success of aligning data strategy with business objectives, it is imperative to define clear performance metrics and key performance indicators (KPIs). These metrics serve as benchmarks for assessing progress and effectiveness.
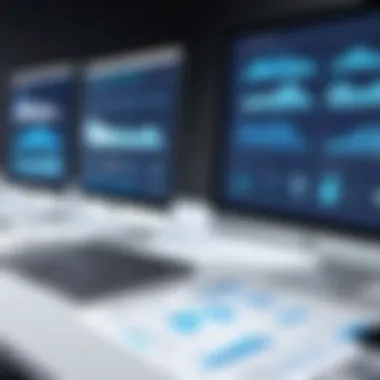
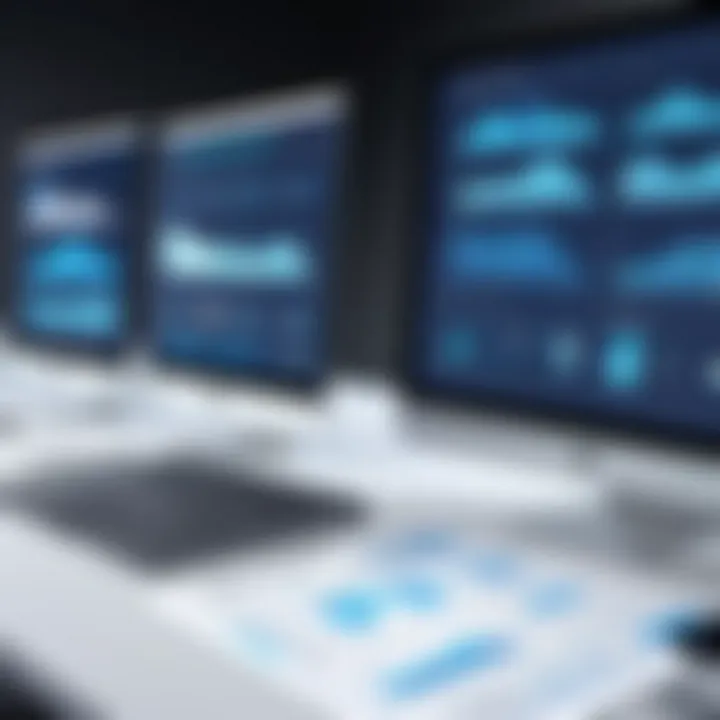
- Establishing SMART goals (Specific, Measurable, Achievable, Relevant, Time-bound) ensures that both data initiatives and business objectives are quantifiable.
- Regularly reviewing performance allows organizations to adjust strategies as needed. This flexibility is crucial in a rapidly changing business landscape.
- Integrating metrics with business outcomes ensures that data strategy remains relevant. Metrics should reflect the broader business context, making it easier to demonstrate value derived from data initiatives.
"Aligning data strategy with business objectives ensures that every data initiative supported brings the organization closer to its goals. It is not just about collecting data; it's about how that data is put to use."
In sum, aligning data strategy with business objectives is not a one-time task but an ongoing process that demands commitment, adaptability, and consistent engagement from all stakeholders. Organizations that navigate this alignment successfully are well-positioned to harness the true power of their data, securing a sustained competitive edge.
Methods for Data Strategy Development
In today’s data-driven environment, developing an effective data strategy is crucial not only for optimal data utilization but also for aligning it with overarching business objectives. There are various methods to approach this development, each providing unique benefits and considerations. A well-structured data strategy can help organizations harness the full potential of their data assets while ensuring data integrity and accessibility.
Conducting a Data Maturity Assessment
A Data Maturity Assessment serves as the foundation for understanding where an organization stands in terms of data management capabilities. This evaluation involves examining current data practices and identifying gaps in processes and technology. The assessment typically involves several key elements:
- Data Collection: Gathering data on existing practices and policies around data management.
- Performance Benchmarking: Comparing current capabilities against industry standards or best practices.
- Gap Analysis: Identifying discrepancies between current practices and desired outcomes.
The benefits of conducting a comprehensive Data Maturity Assessment are significant:
- Clarity of Current State: Organizations gain insights into their present data handling and analytics capabilities.
- Informed Strategy Development: Insights from the assessment inform strategic planning, ensuring that resources are allocated effectively.
As organizations analyze their maturity level, they can set clear objectives for improvement. This step is not just about evaluation but also about laying the groundwork for future data initiatives. Without this critical assessment, organizations risk moving forward without understanding their unique needs and challenges, which can lead to poor investments in data management tools and practices.
Stakeholder Engagement and Buy-In
Stakeholder engagement is essential for successful data strategy development. It involves getting input from various individuals or groups affected by data initiatives, ensuring their needs and concerns are addressed. This can include team members, department heads, and even external partners. Effective engagement has several advantages:
- Increased Visibility: Engaging stakeholders fosters transparency in the strategy development process.
- Improved Collaboration: Stakeholders can provide diverse perspectives for a more rounded strategy.
- Fostering Ownership: When stakeholders feel involved, they are more likely to support and invest in the strategy.
To achieve genuine buy-in from stakeholders, organizations should focus on clear communication. Regular updates, collaborative meetings, and opportunities for feedback can help create an inclusive environment. A strategy developed in isolation is often met with resistance, so it is crucial to involve stakeholders from the beginning. Collaboration at this stage can also ease future implementation efforts, making it smoother and more efficient.
Technology's Role in Data Strategy
Technology serves as a cornerstone in the architecture of an enterprise data strategy. Its integration into data management systems allows organizations to not only store vast amounts of data but also to analyze and draw insights from that data effectively. In a world where data is increasingly recognized as a valuable asset, understanding the intersection of technology and data strategy becomes fundamental for organizations looking to leverage their data assets.
The benefits of combining technology with a solid data strategy are numerous. First, it enhances data accessibility. Advanced data management tools enable employees to access data easily, fostering a culture of data transparency. Second, it can improve decision-making. With the right technology in place, decision-makers can use real-time data analytics to inform their choices, thereby increasing operational efficiency.
Additionally, adopting the latest technologies can lead to significant cost reductions. Companies can minimize operational costs by automating data collection and report generation, decreasing the need for manual input. Moreover, new technologies can enhance data security, ensuring that sensitive information remains protected against breaches.
However, organizations must consider factors such as compatibility and scalability when integrating new technologies into their data strategy. The chosen tools should align with existing systems and future growth plans. Proper training and support for staff are also essential to ensure effective use and maintenance of these technologies.
"Technology is no longer a separate function of the enterprise; it is the enterprise."
Data Management Tools and Platforms
Data management tools and platforms have become integral to successful enterprise data strategies. These tools serve a variety of purposes from data storage, data integration, to data analysis. Popular platforms like Microsoft Azure and Amazon Web Services provide scalable solutions for storing and processing data in the cloud. They allow enterprises to handle large datasets without investing heavily in physical infrastructure.
Besides cloud services, there are specialized tools such as Talend and Informatica that focus on data integration and data quality. These tools help organizations to cleanse, transform, and integrate disparate data sources, providing a unified view of data that is crucial for insightful analysis.
Moreover, Business Intelligence (BI) platforms like Tableau and Power BI transform raw data into interactive visualizations. These tools empower businesses to create reports easily and glean insights without the need for a data science background.
Emerging Technologies in Data Management
The world of data management is evolving rapidly with the advent of new technologies. Artificial intelligence and machine learning have started to play significant roles in how data is handled. These technologies can analyze patterns and predict trends, allowing organizations to be proactive rather than reactive in their data strategies.
Another prominent technology is blockchain, which introduces enhanced data security by providing a decentralized option for data storage. This can be particularly useful in sectors that require high levels of trust and verification, such as finance and healthcare.
Additionally, the Internet of Things (IoT) plays a crucial role in data generation. IoT devices can provide continuous streams of data that organizations can leverage for real-time analytics and operational improvements.
As enterprises navigate the complex landscape of data management, embracing these emerging technologies can provide a competitive edge. Organizations must stay informed about these trends to utilize their data effectively and maintain their position in an increasingly data-driven world.
Governance and Compliance Considerations
Effective governance and compliance are critical in the context of enterprise data strategy. Organizations are tasked not only with managing their data but also ensuring that it is handled in a way that aligns with regulatory requirements and internal policies. This section discusses the necessity of governance and compliance, detailing their benefits and the considerations that organizations must keep in mind.
Regulatory Requirements
The digital landscape is shaped by an array of regulatory frameworks that dictate how organizations must handle their data. Compliance with regulations such as the General Data Protection Regulation (GDPR) and the Health Insurance Portability and Accountability Act (HIPAA) is not optional. Violations can result in hefty fines and significant harm to the organization's reputation.
Organizations must develop a clear understanding of applicable regulations. This involves identifying which regulations are relevant and how they impact data handling practices. For instance, GDPR emphasizes the principles of data minimization and user consent, demanding organizations to be transparent about data usage. This pushes companies to adopt rigorous data management techniques.
In addition to legal obligations, companies can benefit from regulatory compliance in several ways:
- Enhanced Trust: Clients and customers are more likely to engage with businesses that are compliant. It cultivates a culture of trust, which is vital for long-term relationships.
- Reduced Risk: By adhering to regulatory requirements, organizations can mitigate risks related to data breaches and privacy violations.
- Operational Efficiency: Compliance initiatives often lead to streamlined processes, as they require a thorough examination of data usage and practices.
Risk Management in Data Handling
Risk management is an essential aspect of the governance framework. It involves identifying, assessing, and prioritizing risks related to data management, followed by coordinated efforts to minimize their impact. Understanding potential risks allows organizations to proactively implement measures that safeguard data integrity and confidentiality.
The risks can come from various sources, including internal threats such as employee negligence and external threats like cyberattacks. Therefore, organizations must continually evaluate their risk landscape. This evaluation might include:
- Conducting Risk Assessments: Regular assessments help identify vulnerabilities in data processes. This should be reflected in the organization's risk management strategy.
- Implementing Controls: After identifying risks, mitigating strategies need to be put in place. Access controls, data encryption, and regular audits can play a role in reducing risk exposure.
- Training and Awareness: Employees should be trained on data handling procedures and the importance of complying with governance measures. This creates a workforce that is aware and vigilant about data protection.
In summary, governance and compliance considerations form the backbone of a robust enterprise data strategy. Organizations must prioritize the development of frameworks that address regulatory requirements and implement effective risk management strategies. By doing so, they can create an environment where data is managed responsibly, ensuring not only compliance but also the long-term viability of their data practices.
"Effective data governance is not just a matter of compliance—it's a strategic business imperative that enhances organizational performance."
Organizations that take governance and compliance seriously are better positioned to navigate the complexities of the data landscape while extracting maximum value from their data assets.
Metrics for Measuring Data Strategy Success
Metrics play a crucial role in determining the effectiveness of a data strategy. Without clearly defined metrics, organizations risk making decisions based on intuition rather than concrete evidence. Measuring success allows businesses to assess the performance of their data initiatives, evaluate progress over time, and make informed decisions about future strategies.
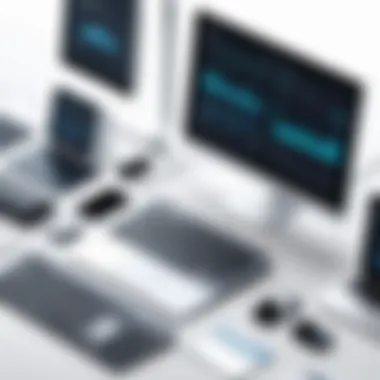
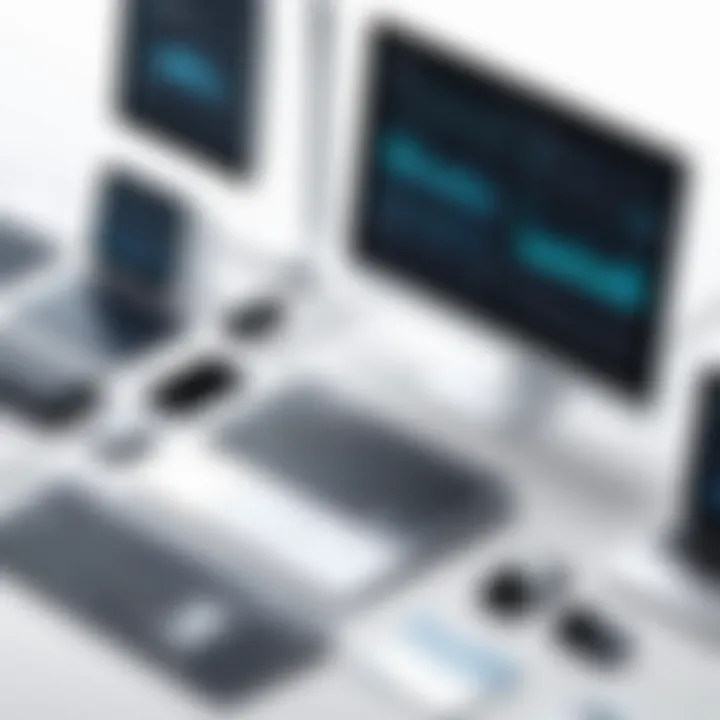
Adopting specific metrics aligned with business goals can provide insights into the quality, availability, and security of data. Moreover, quantifiable metrics create accountability across teams, ensuring that every stakeholder understands their role in achieving data objectives.
Key benefits of implementing these metrics include:
- Improved Decision Making: Metrics help frame the context around data usage and its alignment with business goals. This clarity fosters better decisions.
- Enhanced Data Quality: Establishing metrics for data quality reinforces standards, encourages adherence, and ultimately leads to more reliable datasets.
- Resource Optimization: By tracking performance against metrics, organizations can identify inefficiencies, leading to more strategic resource allocation.
Overall, establishing metrics is foundational for continuous monitoring and evaluation.
Defining Success Metrics
Defining success metrics is not a trivial task. It requires comprehensive understanding of both data objectives and business goals. Success metrics should not only reflect data performance but also align with organizational targets. Here are some elements to consider:
- Relevance: Ensure that each metric is directly related to key objectives, providing genuine insights.
- Quantifiability: Metrics should be measurable. Use numeric indicators, such as percentage increase in data quality or reduction in data retrieval time.
- Timeliness: Metrics need to be evaluated regularly to provide real-time insights.
- Comparison: Establish benchmarks for performance comparison, which can be internal or external.
Moreover, common success metrics in data strategy may include:
- Data Accuracy Rate: Measures how often data is correct against expectations.
- Data Accessibility: Analyzes how easily and quickly data can be retrieved by relevant stakeholders.
- Costs Related to Data Management: Examines expenses associated with data storage, retrieval, and processing.
Continuous Improvement Practices
Continuous improvement should be at the heart of any data strategy. As businesses evolve, so do their data needs. Here are some practices to facilitate ongoing enhancement of data strategies:
- Regular Assessment: Frequently review success metrics and methodologies to adapt to changing business environments.
- Feedback Loops: Continuous input from key stakeholders ensures that data strategies remain relevant and effective.
- Training Programs: Regular training on data management tools and protocols ensures that employees stay informed about best practices.
Importantly, organizations must cultivate a culture of improvement. > "Embracing a mindset of learning and adaptation plays a pivotal role in sustaining effective data strategies."
In summary, measuring success and ensuring continuous improvement creates a dynamic data strategy that aligns with ever-changing business needs. This approach enables organizations to optimize their data assets effectively, ensuring they derive maximum value from their data investments.
Challenges in Implementing Data Strategies
Implementing a data strategy is not just about having a plan; it involves navigating through a complex maze of challenges. Recognizing these obstacles can significantly enhance the chances of a successful implementation. A robust data strategy should be both comprehensive and adaptable, adjusting to internal and external pressures.
Successful data strategies align with business goals, ensuring that data serves as a strategic asset. Misalignment with these objectives can lead to wasted resources, ineffective data governance, and ultimately, failed projects. Understanding the challenges helps organizations preemptively devise solutions to create a more data-driven culture.
Common Pitfalls
When developing a data strategy, there are several common pitfalls to avoid. These include:
- Lack of Clear Objectives: Organizations may embark on data initiatives without clearly defined goals, leading to misaligned efforts.
- Inadequate Stakeholder Engagement: Often, the stakeholders who use data in their daily tasks are not consulted, resulting in a strategy that does not address real-world needs.
- Underestimating Data Complexity: Data environments can be intricate due to different types of data, storage systems, and privacy regulations. Neglecting to plan for these complexities leads to execution failures.
- Neglecting Change Management: Implementation of a data strategy tends to bring about changes in processes which, if not managed well, can face resistance from staff.
"A robust data strategy needs to be seen as an ongoing journey, not a static document."
Addressing Resistance to Change
Resistance to change is a significant barrier when implementing a data strategy. People often prefer the status quo, especially in organizations where workflows become routine. Several strategies can help mitigate this resistance:
- Effective Communication: Clearly explain the reasons behind the change and the benefits it will bring to all employees, not just management.
- Training and Support: Offer continuous training programs to ensure all team members feel equipped to handle new technologies and processes.
- Involve Employees in Planning: Engaging employees in the development of the data strategy can foster a culture of acceptance.
- Address Concerns Promptly: Create an environment where employees feel comfortable voicing their concerns and feedback about the data strategy.
By addressing these common pitfalls and effectively managing resistance, organizations increase the likelihood of implementing a successful data strategy that meets their overall business objectives.
Case Studies in Enterprise Data Strategies
Case studies in enterprise data strategies serve as tangible examples for organizations seeking to refine and optimize their data management practices. These case studies reveal practical applications, challenges faced, and solutions implemented by various organizations. Understanding real-world scenarios allows businesses to assess their own situations against established benchmarks and insights, fostering an environment of continuous learning.
The significance of case studies extends beyond mere academic interest. They provide valuable lessons that can inform strategic decisions. By examining different approaches, organizations can identify best practices and avoid common pitfalls. Engaging with such examples helps substantiate the need for a robust data strategy aligned with business objectives.
Successful Implementations
Successful implementations of enterprise data strategies often demonstrate clear alignment between business goals and data management practices. One relevant example is the case of Netflix. The streaming giant effectively harnesses data analytics to personalize viewer experiences. By leveraging user data, Netflix recommends content tailored to individual preferences, which enhances customer satisfaction and retention. This approach showcases how integrating data insights into a business model can drive user engagement and ultimately revenue.
Another illustrative case is Johnson & Johnson. The company embraced a comprehensive data governance initiative aimed at ensuring data quality and compliance across all business units. By establishing clear data ownership and accountability, Johnson & Johnson enhanced its operational efficiency. Their success highlights the importance of establishing a structured approach to data management that aligns with regulatory requirements while also promoting consistency and reliability in data utilization.
Successful case studies underline key elements of an effective data strategy: strong leadership commitment, an organizational culture open to data-driven decision-making, and the endorsement of cross-departmental collaboration.
Lessons Learned from Failures
Conversely, lessons learned from failures can be just as insightful. A notable example is the case of Target. The company's ambitious data-driven marketing strategy aimed to predict consumer behavior. However, a lack of proper data governance led to a significant misfire. In attempting to predict which customers might be pregnant, Target sent advertisements to households, resulting in fallout and negative publicity. This misstep illustrates the risks of insufficiently understanding customer data privacy and ethical considerations.
Furthermore, Kodak serves as a cautionary tale. Once a leader in photography, Kodak failed to adapt to the digital transformation due to an inadequate data strategy. While the company had access to valuable market insights, its inability to pivot its business model cost it significant market share. This example emphasizes that a stagnating approach to data management can hinder innovation and competitiveness in an evolving landscape.
When examining failures, organizations must focus on areas such as data governance, understanding customer privacy, and maintaining agility in strategy adaptation. Failing to recognize these elements can lead to oversights that may hinder success.
"Learning from both successes and failures in data strategy is a critical component of ongoing improvement and innovation."
Future Trends in Data Strategy
In the rapidly evolving landscape of information technology, the notion of data strategy gains prominence. Organizations are increasingly recognizing the need to adapt to changes, driven by both technological advancements and regulatory demands. Future trends in data strategy are not merely predictions; they form an essential framework for organizations aiming to maintain flexibility, competitiveness, and compliance in a data-centric world.
AI and Automation in Data Management
Artificial Intelligence (AI) and automation are reshaping the way data is managed. These technologies offer enhanced capabilities in data processing, analysis, and decision-making. Automating repetitive tasks frees resources and allows professionals to focus on strategic initiatives. AI can sift through vast datasets to surface insights that are otherwise time-consuming to uncover.
For instance, AI-powered tools can significantly enhance data quality. By automatically identifying anomalies or inconsistencies in datasets, organizations can ensure more reliable data for decision-making. Additionally, predictive analytics can help in forecasting trends, thereby informing future strategies.
The implementation of AI in data management is not without challenges. Organizations must ensure they have the necessary infrastructure, skilled personnel, and a clear understanding of ethical implications. Furthermore, training algorithms on high-quality data is critical; poor data can compound errors effectively turning solutions into problems.
The Role of Data Ethics
As organizations leverage data, the conversation often turns towards data ethics. This aspect becomes pivotal in the development and execution of data strategies. Ethical considerations must guide the collection, management, and usage of data to ensure compliance and build trust with stakeholders.
Organizations should adhere to principles like transparency, accountability, and privacy. For example, obtaining explicit consent before data collection is not only a legal requirement in many jurisdictions but also fosters trust among users.
Moreover, the responsible usage of AI in analyzing data also brings ethical questions to the forefront. Without proper oversight, there is a risk of bias in algorithms, which can amplify existing inequalities.
"Data ethics is not just an add-on to your strategy; it is integral to its success."
Creating a framework for ethical data management can mitigate risks and build a data culture rooted in responsibility. Employing a Data Ethics Officer or establishing an ethics committee can be beneficial in ensuring compliance with ethical standards.
As organizations look forward, integrating AI and maintaining ethical considerations are not just optional; they have become necessities in shaping a successful data strategy.