Key Competencies Every Data Analyst Must Master
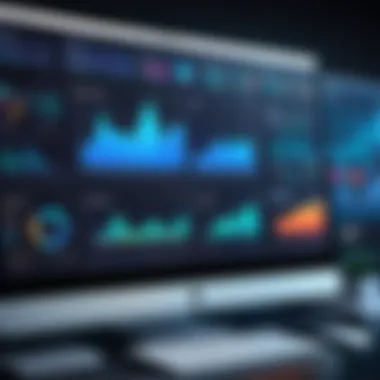
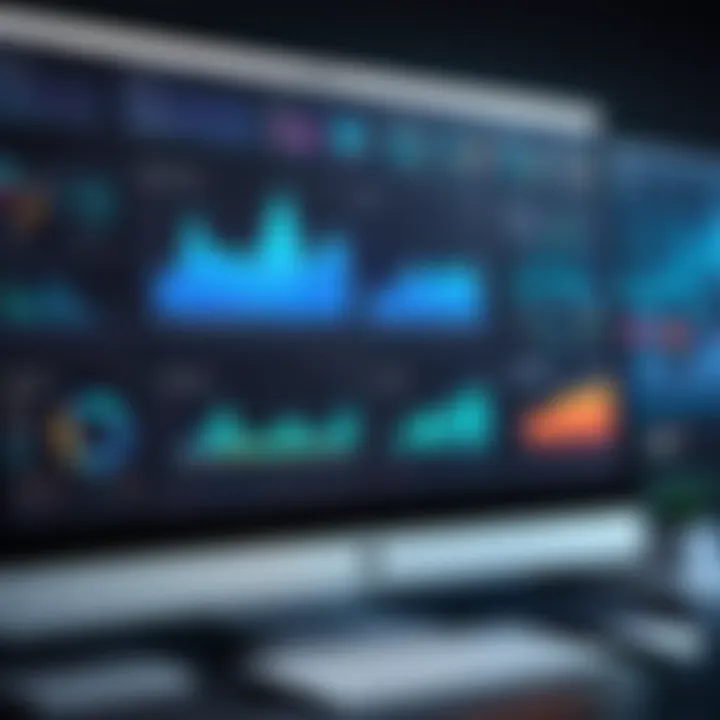
Intro
In today's dynamic and data-centric landscape, the role of a data analyst has transformed considerably. The skills required have expanded beyond mere data collection and processing. Understanding the underlying storage, security, and networking concepts has become vital. A data analyst not only needs to manipulate and derive insights from complex datasets but also to be versed in how these datasets are stored, secured, and transmitted.
Let's venture into the pivotal skills that form the bedrock of a successful data analyst.
Understanding Storage, Security, or Networking Concepts
Prelims to the Basics of Storage, Security, or Networking
At the core of any data-driven operation lies a triad of interdependent elements: storage, security, and networking. Knowledge in these areas is not just a technical necessity but a prerequisite for effective analysis. A data analyst should comprehend how data storage operates—both in traditional databases and modern cloud solutions. Security, too, cannot be an afterthought as the stakes of data breaches continue to rise. Plus, networking plays an integral role, ensuring that data flows efficiently and remains accessible.
Key Terminology and Definitions in the Field
Understanding the vocabulary of data management is crucial. Terms such as data lake, encryption, and bandwidth often float around the industry. The term data lake refers to a centralized repository that allows you to store all your structured and unstructured data at any scale. In contrast, encryption is about securing data, making it unreadable without the right key, which is crucial for protecting sensitive information. Lastly, bandwidth signifies data transfer capacity and significantly affects how swiftly insights can be extracted from data resources.
Overview of Important Concepts and Technologies
A strong grasp of cloud storage solutions like Amazon S3 or Google Cloud Storage is essential. These platforms offer scalability and flexibility, enabling analysts to handle increasing amounts of data with ease. On the security side, familiarity with protocols like SSL/TLS helps ensure secure data transfers across networks. Moreover, understanding virtual private networks (VPNs) is critical to safeguarding data in transit.
"Knowledge is the key to unlocking the potential of data analysis, influencing how businesses make decisions."
Best Practices and Tips for Storage, Security, or Networking
Tips for Optimizing Storage Solutions
When dealing with data storage, maximizing efficiency is paramount. Here are a few pointers:
- Regularly purge unnecessary data to enhance performance.
- Adopt data compression techniques wherever feasible.
- Utilize indexing methods to speed up data retrieval.
Security Best Practices and Measures
Security remains a focal point in data management. Consider these practices:
- Implement two-factor authentication to add an extra layer of security.
- Conduct regular security audits to identify vulnerabilities.
- Keep software and hardware updated to mitigate potential threats.
Networking Strategies for Improved Performance
In terms of networking, having effective strategies can lead to faster data communication. Look into the following:
- Utilize quality of service (QoS) settings to prioritize critical data traffic.
- Ensure proper bandwidth allocation based on data usage trends.
- Regularly test and optimize network performance.
Industry Trends and Updates
Latest Trends in Storage Technologies
With the rapid evolution of technology, keeping a pulse on storage trends is crucial. Cloud-based storage continues to dominate due to its cost-effectiveness and flexibility for businesses.
Cybersecurity Threats and Solutions
As for security, new threats emerge constantly. Phishing attacks have become more sophisticated, and businesses must remain vigilant by training employees in recognizing these threats.
Networking Innovations and Developments
Advancements such as Software-Defined Networking (SDN) are redefining how networks are managed, making them more agile and adaptable.
Case Studies and Success Stories
Real-Life Examples of Successful Storage Implementations
Consider a retail company that transformed its sales data management by migrating to a cloud storage solution, which led to a noticeable reduction in operational costs and improved data access.
Cybersecurity Incidents and Lessons Learned
The infamous Equifax breach serves as a critical reminder that robust data security measures are not optional. The breach exposed sensitive information of countless individuals, hammering home the need for better practices.
Networking Case Studies Showcasing Effective Strategies
A major tech startup improved its network redundancy by incorporating diverse pathways for data transmission, which significantly enhanced its overall service reliability.
Reviews and Comparison of Tools and Products
In-Depth Reviews of Storage Software and Hardware
Analyzing tools like Microsoft Azure and IBM Cloud Storage can provide insight into which platform best fits a company's needs.
Comparison of Cybersecurity Tools and Solutions
Tools such as Norton and McAfee might seem similar, but they cater to different security needs, which a savvy analyst should consider.
Evaluation of Networking Equipment and Services
Reviewing products like Cisco routers can shed light on the performance differences that may influence networking choices.
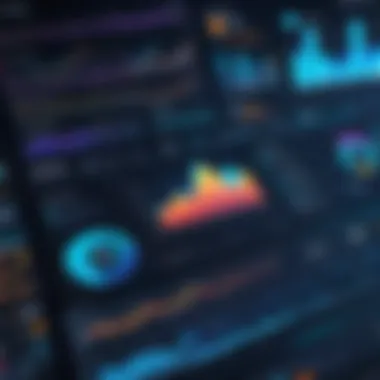
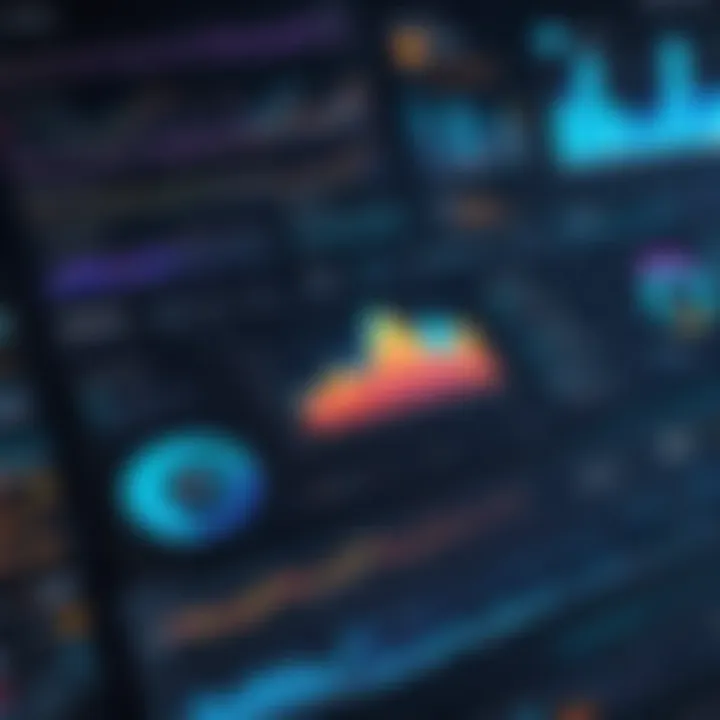
Understanding the Role of a Data Analyst
In the fast-paced world of data, the line between data and actionable insights often blurs. The role of a Data Analyst has gained significant traction as organizations increasingly lean on data-driven decisions. Understanding this role is not merely an academic exercise; it's a crucial step toward appreciating how analysts contribute to achieving business goals.
A Data Analyst serves as the bridge between data and decision-making. They sift through extensive datasets, looking for patterns while turning numbers into narratives. This role is vital, as organizations can lose opportunities and miss market trends without proper analysis.
Investing time to understand the responsibilities and significance of a data analyst sets a solid foundation for both current professionals and aspiring entrants in the field. This knowledge underpins the essential skills that will be discussed further in this article.
Defining a Data Analyst's Responsibilities
The responsibilities of a Data Analyst encompass a wide range of activities, all aimed at transforming raw data into meaningful insights. Analysts commonly engage in the following tasks:
- Data Collection: Gathering relevant data from various sources, whether internal databases or external platforms.
- Data Cleaning: Identifying and rectifying errors and inaccuracies in the datasets to ensure integrity.
- Data Exploration: Conducting exploratory data analysis (EDA) to get a feel for data, visualizing trends, and finding patterns.
- Statistical Analysis: Applying statistical tests to validate findings and ensure they are not due to chance.
- Reporting: Preparing comprehensive reports that highlight key findings, complete with visual aids, so stakeholders can understand the insights quickly.
- Collaboration with Teams: Working alongside other departments, like marketing or finance, to tailor analyses that meet specific business needs.
The multifaceted nature of these responsibilities signifies the versatility expected from a Data Analyst. Their ability to juggle various tasks while retaining focus on the ultimate goal of deriving insights makes them a precious asset in any organization.
Importance of Data Analysis in Organizations
Data analysis holds critical importance in modern organizations, and its impact cannot be overstated. Here are some benefits of robust data analysis practices:
- Informed Decision-Making: With accurate data-driven insights, businesses can make strategic decisions that are not merely based on gut feelings but on factual evidence.
- Identifying Opportunities: Companies equipped with comprehensive data analysis can effectively spot market trends and potential areas for growth. This knowledge allows them to stay ahead of competitors.
- Cost Reduction: By analyzing operational data, organizations can uncover inefficiencies and reduce costs, improving their bottom line.
- Customer Insights: Larger datasets also lead to better understanding of customer preferences, enabling companies to tailor their products or services accordingly.
"In an era where time is money, data analysis is that lens through which businesses can navigate the complex market terrain with precision."
This emphasis on data analysis illustrates just how integral the role of a Data Analyst is to the smooth functioning and success of modern organizations.
Core Technical Skills
In the fast-paced realm of data analysis, core technical skills serve as the backbone for professionals aiming to turn raw data into actionable insights. These skills encompass a blend of statistical knowledge, tools for visualization, and proficiency in programming languages—every piece a vital cog in the data analysis machine. The significance of these competencies cannot be overstated; they influence not just the depth and quality of analysis, but also how data findings are communicated and implemented within organizations. In other words, without a solid foundation in these areas, one might struggle to extract the meaningful patterns that lie buried in heaps of numbers and datasets.
Proficiency in Statistical Analysis
Statistical analysis forms the bedrock of effective data interpretation, helping analysts to sift through data and detect trends, correlations, and outliers. This often requires knowledge of probabilistic models and statistical tests, such as t-tests, chi-squared tests, and regression analysis. The thorough understanding of these tools enables analysts to make data-driven decisions rather than gut-based ones.
Moreover, possessing a strong grasp of statistics not only bolsters credibility but also enhances the ability to forecast future trends. In practical terms, consider this: if an e-commerce company notices an uptick in sales during a particular period, it's not merely the seasonal change at play. Through statistical analysis, a data analyst can explore various factors—like marketing campaigns or competitor actions—to unveil the underlying reasons.
Mastery of Data Visualization Tools
The importance of clear communication cannot be overstated in data analysis. This is where data visualization tools come into play. With the capacity to transform complex data sets into intuitive graphics, these tools serve as vital instruments for conveying insights. Think about it—if you present an intricate data table to a group of stakeholders, they might struggle to grasp the key messages. However, by employing visualization techniques such as charts, graphs, or dashboards, these same data points can come alive, painting a clearer picture of trends and anomalies.
Popular tools like Tableau and Power BI allow analysts to build interactive visualizations, making it easier for stakeholders to engage with the data. This not only fosters a better understanding but also facilitates quicker decision-making. In an era where time is money, the ability to visualize data effectively can mean the difference between success and stagnation.
Expertise in Programming Languages
Programming is another cornerstone of data analysis. A thorough understanding of at least one programming language is essential for data manipulation and analysis. Analysts can efficiently perform repetitive tasks, automate data handling, and create complex analyses that wouldn't be feasible manually.
Python
Python stands tall in the realm of programming for data analysis. Its simplicity and versatility make it a go-to choice for many professionals. With libraries like Pandas for data manipulation and Matplotlib for data visualization, Python allows analysts to focus more on solving problems than wrestling with the code. For instance, when analyzing customer purchase behavior, using Python can streamline the extraction and cleaning of data, making the process less time-consuming.
Moreover, Python’s community support is robust, ensuring that help is just a forum post away. However, its dynamic typing can lead to subtle bugs that might catch a novice analyst off guard, so it's important to approach it with an understanding of best practices.
R
R is another powerhouse in the data analysis landscape, especially favored for statistical analysis and visualization. Its syntax is oriented towards statisticians, offering a wealth of packages specifically designed for complex analyses. When working on projects that require advanced statistical functions or detailed plots, R shines remarkably well. Its graphical capabilities allow analysts to create custom visualizations that may be difficult to achieve with other tools.
That said, R does have a steeper learning curve, which can be intimidating for those just starting out. If one is willing to invest the time, however, the depth it offers can become a strong asset.
SQL
Structured Query Language, or SQL, is integral to managing and querying data stored in relational databases. Analysts proficient in SQL can extract specific data sets from large databases efficiently, a skill invaluable in any data-driven environment. It allows for the crafting of sophisticated queries that can pull targeted information, which is essential for informed decision-making.
SQL's straightforward syntax and wide adoption across industries make it particularly beneficial. With its focus on data manipulation, analysts can create, read, update, and delete records swiftly. The downside, however, is that SQL might fall short in performing advanced analytical tasks compared to programming languages like Python or R, which is something analysts must keep in mind when choosing their tools.
Data Management Proficiencies
In the vast landscape of data analytics, possessing data management proficiencies is crucial. Analysts are like navigators on a ship, steering through a seemingly endless ocean of information. Understanding how to properly manage data can determine the success of a project and, ultimately, the organization. Without solid data management skills, even the most talented analysts might find their efforts going down the drain. Therefore, any aspiring data analyst must hone these essential abilities to harness the true power of data.
Understanding Data Warehousing Concepts
Data warehousing is not just a buzzword in conversations among data enthusiasts; it forms the backbone of effective data analysis. A data warehouse is essentially a central repository that consolidates various data sources. When analysts understand this concept, they gain insight into how to retrieve and leverage the data pivotal for their analyses.
The importance of knowing data warehousing extends beyond mere storage solutions. It offers a structured way to handle large volumes of data, ensuring it is accessible and ready for analysis. An analyst who grasps the principles of data warehousing can recommend more efficient architectures, improving query performance and reducing retrieval times. For instance, utilizing star or snowflake schemas in a data warehouse can significantly ease how data is analyzed.
Moreover, knowledge of data warehousing facilitates better communications with IT departments, ensuring seamless integration processes. In time, this competency becomes a bridge to understanding more complex Data Mining and Business Intelligence concepts, solidifying the analyst's role as a valuable asset in the organization.
Data Cleaning and Preparation Techniques
Before diving into data analysis, one must grapple with the often tedious yet vital task of data cleaning and preparation. If data is the oil that fuels analysis, then data cleaning is akin to refining that oil. Poor data quality can lead to misguided insights, so it's absolutely paramount that analysts adopt robust cleaning techniques.
Essentially, data cleaning involves identifying and correcting errors or inconsistencies. This process might require checking for duplicates, addressing missing values, or standardizing data formats. For instance, if some dates are in MM/DD/YYYY format while others are in DD/MM/YYYY, the analyst must standardize them to maintain uniformity.
Furthermore, preparation techniques, like normalization and denormalization, play a crucial role. Normalization helps in partitioning the data to reduce redundancy, while denormalization can improve data retrieval speeds during analysis.
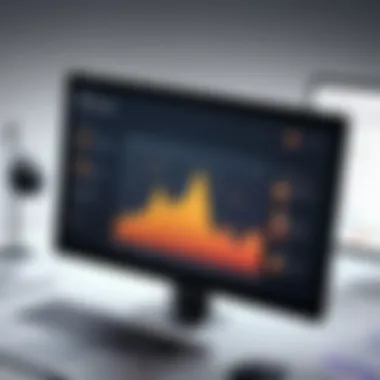
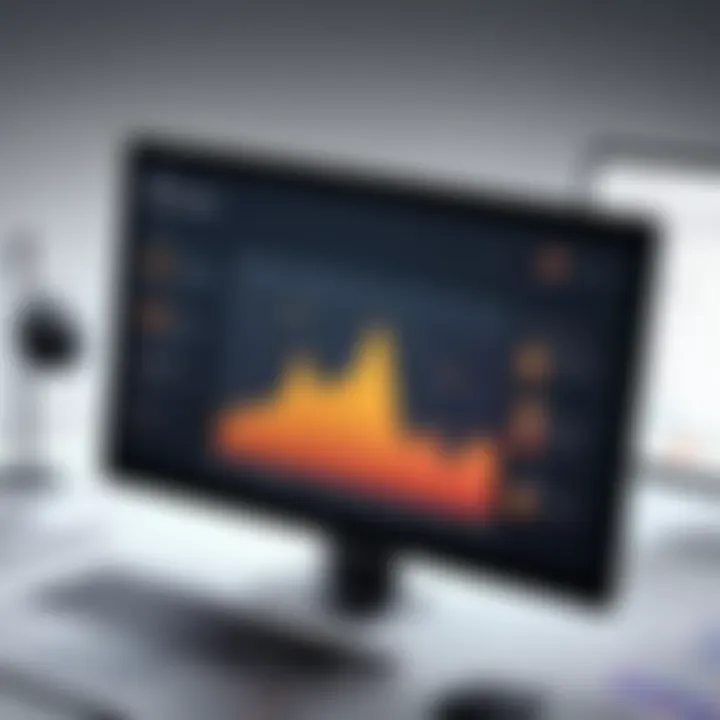
Here’s a brief overview of common data preparation steps:
- Data Auditing: Assess the quality and condition of the data before processing.
- Handling Missing Data: Decide whether to fill in missing values or exclude that data entirely.
- Outlier Detection: Identify and deal with outliers that can skew analysis results.
- Data Transformation: Convert data into the right format or structure for analysis.
In a nutshell, mastering data cleaning and preparation ensures not only the integrity of the data analysis but also enhances its reliability and ultimately informs better decision-making processes.
"The right dataset is like a compass; it’ll guide your analysis to the right conclusions. But a cleaned dataset is the map, showing you where to go without getting lost in the chaos of raw data."
Overall, analysts who excel at data management, particularly in warehousing and cleaning techniques, set themselves apart as indispensable contributors to their organizations. With these proficiencies, they can unlock the full potential of data—transforming it into actionable insights that drive strategic decision-making.
Analytical Thinking and Problem Solving
Analytical thinking and problem solving are at the heart of a data analyst's role. Mastering these skills allows professionals to sift through vast datasets, identify meaningful insights, and solve complex issues. In an environment where data shapes strategic decisions, being able to interpret and analyze that data is not just helpful—it’s essential. Data analysts often face intricate scenarios requiring a nuanced understanding of patterns, relationships, and anomalies within data. This requires a sharp analytical mind and a proactive approach to problem resolution.
Understanding how to recognize patterns and trends can greatly enhance one's ability to make data-driven decisions. Analysts who can see beyond the numbers often find themselves better equipped to propose actionable recommendations that resonate with stakeholders. Moreover, this facet of analytical thinking encourages a deeper engagement with the data, prompting analysts to ask the right questions and assess the validity of their interpretations.
Identifying Patterns and Trends
The ability to spot patterns and trends in data is paramount. It’s akin to being a detective sifting through clues to solve a case. A data analyst studies historical data and observes how changes influence outcomes. For example, examining customer purchase behaviors over time requires discerning whether seasonal fluctuations affect sales or if a specific marketing campaign is driving engagement.
- Look for anomalies: Sometimes, the most insightful trends come from exceptions. An unexpected dip or surge can reveal underlying issues or opportunities that merit further exploration.
- Use visual aids: Data visualization tools, like Tableau or Power BI, can elevate this process. Visualizing data can often lead to quicker and clearer identification of trends than simply hunting through raw datasets.
Overall, identifying trends is not solely about recognizing what has happened but also about predicting what might occur. Doing so positions analysts to contribute meaningfully to strategic planning.
Formulating Hypotheses and Testing
Once trends are identified, formulating hypotheses serves as the next logical step. It’s about making educated guesses that can be tested against data. Analysts should ask themselves questions: What factors could influence this trend? or Is there a correlation between these two variables?
Testing hypotheses involves collecting additional data and conducting various analyses to validate or discard assumptions. This iterative process is critical because:
- It promotes critical thinking: Being able to support or refute a hypothesis fosters a greater understanding of the data and its implications.
- It informs strategy: When hypotheses are tested successfully, they can lead to data-backed decisions that might enhance business performance.
- It cultivates resilience: Not every hypothesis will be proven right. Learning from failures to refine future hypotheses is a crucial part of analytical thinking.
"The most successful analysts are those who embrace uncertainty and derive insights through rigorous testing."
Importance of Domain Knowledge
In the realm of data analysis, possessing technical skills is just the tip of the iceberg. Understanding the specific industry context in which a data analyst operates—often referred to as domain knowledge—is critical for driving impactful insights. Whether in healthcare, finance, or marketing, domain knowledge shapes the perspective of data interpretation and decision-making processes.
Understanding Business Needs and Objectives
A data analyst equipped with robust domain knowledge can pinpoint the crucial business needs and objectives specific to their industry. This understanding goes beyond mere familiarity with data; it means grasping the underlying factors that influence the metrics being analyzed. For instance, in the retail industry, knowing seasonal trends and customer behaviors significantly informs how analysts interpret sales data. They can see not just numbers but the story behind those numbers.
The ability to connect business objectives with data insights ensures that analyses are not just factual but relevant and actionable. When a data analyst understands, for example, the marketing strategies a company employs, they are better positioned to assess the performance of those strategies. They can dive deeper into data that reflects customer engagement and satisfaction, thereby offering recommendations that directly align with the company's goals.
"Data analysis without understanding the business context is like trying to solve a puzzle with half the pieces missing."
Adapting Skills to Various Industries
The adaptability of a data analyst’s skills across various industries hinges largely on their domain knowledge. Data interpretation requirements vary significantly—what is essential in a tech startup might not hold in a governmental organization. The nuances in regulatory standards, customer expectations, and competitive landscapes demand that analysts adjust their tools and approaches accordingly.
For example, analyzing consumer behavior in e-commerce hinges heavily on online shopping trends, while a healthcare analyst focuses more on patient outcomes and compliance with healthcare laws. An astute data analyst is not just crunching numbers; they're tailoring their analytical methods to suit the peculiarities of each industry.
In summary, domain knowledge is an essential component of a data analyst's toolkit. It enriches their ability to translate raw data into insightful, strategic recommendations that resonate with stakeholders, regardless of the industry context.
Thus, skipping over domain knowledge can lead to misinterpretation of data, wasted resources, and missed opportunities—all outcomes that businesses can ill afford.
Communication Skills and Stakeholder Engagement
In the intricate labyrinth of data analysis, communication stands as a beacon that guides the insights derived from numbers to tangible actions. The importance of communication skills in the realm of data analytics cannot be overstated. These skills ensure that data analysts effectively bridge the gap between complex statistical findings and a broader audience that may not have a technical background. When data speaks, it is the responsibility of the analyst to translate this language into meaningful narratives that resonate with stakeholders across different levels. It's not just about presenting facts; it is about telling a compelling story that drives decisions and influences change.
One of the primary benefits of strong communication skills is the ability to foster trust and collaboration among team members and stakeholders. When data analysts can articulate their findings clearly and confidently, it creates an environment where others feel empowered to engage with the data. This collaborative spirit leads not only to richer discussions but also to innovative solutions that might have otherwise gone unnoticed. It’s this trust that can transform mere data presentation into strategic advising.
Translating Data Insights into Recommendations
Translating insights into actionable recommendations is an art form of its own. Data analysts sift through vast arrays of information, uncovering trends and patterns that can inform business decisions. However, the nuances of these insights often get lost in technical jargon. For instance, an analyst might discover a significant uptick in customer engagement when specific marketing strategies are employed. This would necessitate not just the identification of this pattern, but also the framing of it within a context that resonates with stakeholders.
To do this well, analysts must put themselves in the shoes of their audience. What do they care about? What challenges are they facing? By understanding the audience’s perspective, analysts can tailor their recommendations to directly address these concerns. Using straightforward language, grounded examples, and clear visuals can aid in painting a vivid picture. For instance, when presentation tools like Tableau or Power BI are employed effectively, the data can be transformed into easy-to-understand graphs that lay bare the story behind the numbers.
Here's a simple approach to translating insights:
- Identify key findings: What is the most significant insight derived from the data?
- Link to objectives: How does this insight align with organizational goals?
- Propose actions: What specific steps can be taken based on these insights?
Presenting Data to Non-Technical Audiences
When it comes to communicating data to a non-technical audience, simplicity is key. The challenge lies in ensuring that the essence of the data is communicated without overwhelming listeners with technical details. While industry insiders might appreciate a deep dive into the methodologies, non-technical stakeholders are usually more focused on the implications of these findings.
Analysts can employ various techniques to simplify their presentation. If we consider the visuals again, charts and infographics can act as quick reference points and can distill complex information into digestible bits. The color scheme, layout, and annotation should draw the eye and lead the audience toward the most critical data points.
Practical Tips for Presenting Data:
- Use metaphors or analogies to relate complex ideas to familiar concepts.
- Maintain a narrative arc, guiding your audience through the data journey.
- Encourage questions and discussions; this involvement helps to clarify and reinforce understanding.
Ultimately, proficient communication within the context of data analysis is about making connections and fostering understanding. The clearer the message, the more impactful the insights. With finely honed communication skills, data analysts can ensure that their work does not merely live in a vacuum but instead contributes to informed decision-making across their organizations.
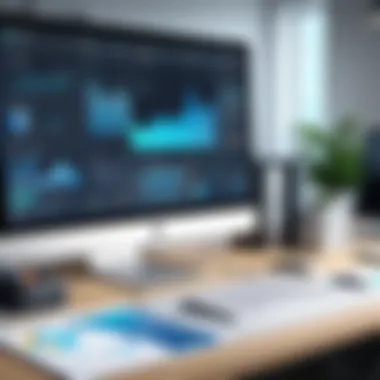
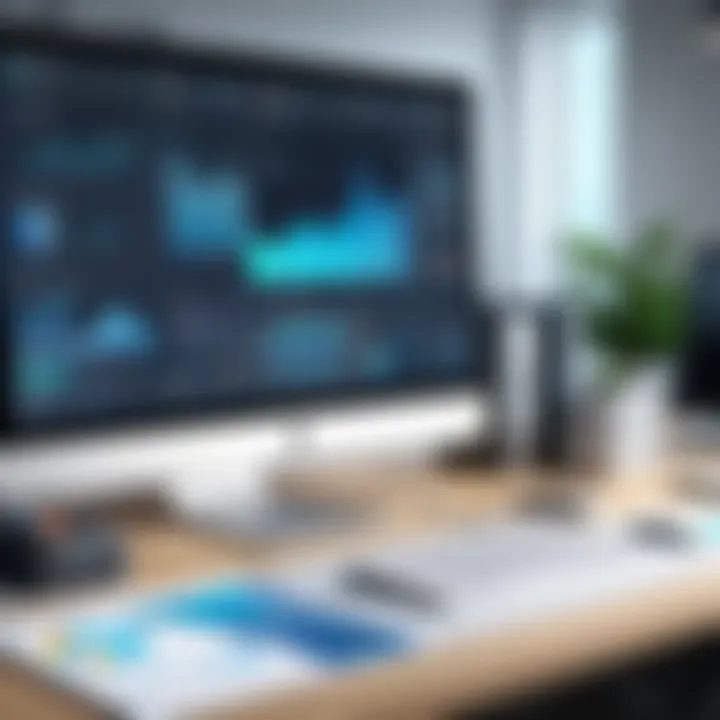
"A great analyst is not just a number cruncher, but a storyteller of the data world. Striking the balance between clarity and depth can make all the difference."
By mastering these communication skills, data analysts position themselves as invaluable assets within their organizations, capable of turning raw data into strategic insights that lead to tangible results.
Collaboration and Teamwork
Collaboration and teamwork stand at the very heart of effective data analysis. In today’s interconnected work environment, no data analyst works in a vacuum. The prowess of a data analyst often shines brightest when they engage with other professionals, pooling diverse areas of expertise to address challenges and derive insights. The fusion of perspectives can lead to more robust findings and innovative solutions, which are critical for driving organizational success.
Understanding how to collaborate effectively is not merely a nice-to-have; it is an essential component of the data analyst’s arsenal. The ability to work seamlessly with others can facilitate clear communication, speed up problem-solving, and foster a sense of shared purpose. Whether it’s liaising with marketing departments to interpret customer data or teaming up with IT to ensure systems can handle large datasets, a good data analyst must learn how to navigate these interactions with finesse.
"Great things in business are never done by one person. They're done by a team of people."
Working with Cross-Functional Teams
Effective collaboration often involves engaging with cross-functional teams. A data analyst must embrace this dynamic, as they will frequently find themselves working alongside professionals from various departments, each with unique insights and objectives. For instance, an analyst may collaborate with sales teams to identify trends influencing purchases or partner with logistics to optimize operations based on supply chain data.
Benefits of cross-functional teamwork include:
- Diverse Perspectives: Different departments bring varied viewpoints, allowing analysts to enrich their analyses with insights from other fields.
- Enhanced Problem-Solving: By blending knowledge across disciplines, teams can develop innovative solutions to complex issues that a single department may struggle to address effectively.
- Improved Communication: Regular interaction with various stakeholders helps analysts hone their communication skills, allowing them to better tailor their messages for different audiences.
It's crucial, however, to recognize that working with cross-functional teams also presents challenges. Conflicting priorities can arise among departments, and varying levels of data literacy might lead to misunderstandings. Navigating these hurdles requires patience, empathy, and strong interpersonal skills from the analyst.
Fostering a Collaborative Environment
Creating a culture of collaboration is pivotal for any organization striving for success in data analysis. A collaborative environment nurtures connection among team members, promotes knowledge-sharing, and leads to collective growth. When analysts feel comfortable reaching out for help or brainstorming ideas, the environment naturally becomes more conducive to innovation.
Ways to foster such an environment include:
- Encouraging Open Dialogue: Establishing norms where team members feel safe sharing ideas and challenges can break down barriers.
- Utilizing Collaborative Tools: Implementing tools like Slack, Asana, or Trello can facilitate communication and help track projects effectively.
- Building Trust: Relationships built on trust encourage collaborators to rely on one another, leading to stronger alliances across teams.
When everyone aligns towards a common goal, the result is increased creativity and productivity. This synergy can elevate data analysis efforts, allowing for deeper insights that ultimately drive actionable strategies, improving overall organizational efficiency.
In short, mastering collaboration and teamwork isn’t just about enhancing one’s analytical skills; it’s about weaving the very fabric of successful data-driven decision-making within an organization.
Continuous Learning and Adaptability
In the rapidly shifting landscape of data analysis, the concept of continuous learning and adaptability becomes paramount. Essentially, data analysts must cultivate a mindset that fosters a commitment to lifelong learning. As new tools, technologies, and methodologies develop at an astonishing pace, staying relevant means engaging with new knowledge frequently. The benefits of adopting this approach are multifold, impacting not only personal career trajectories but also organizational success. Analysts who are able to swiftly adjust to new information can harness data's full potential, ensuring their insights remain cutting-edge and relevant.
Staying Updated with Emerging Technologies
The data realm is teeming with innovations—be it artificial intelligence, machine learning, or big data tools. Keeping one’s finger on the pulse of these advancements is not merely beneficial; it’s essential. Regularly updating technical skills provides a competitive edge. For example, an analyst well-versed in the latest machine learning algorithms can analyze vast datasets far more effectively than one who relies on outdated techniques. Moreover, engaging with professional communities and resources is a practical strategy. Forums like Reddit and specialized articles on Wikipedia or Britannica can offer valuable insights into breakthroughs that could shape data methodologies.
To facilitate this ongoing education, analysts should consider:
- Participating in workshops and webinars: Interact with industry leaders who share their latest findings.
- Online courses: Platforms like Coursera or LinkedIn Learning provide extensive training on new tools.
- Networking: Engaging with peers can lead to shared experiences and knowledge.
By embedding these practices into their routines, analysts ensure that they’re not just spectators in the ever-evolving landscape but active participants.
Embracing Feedback for Improvement
In a field that thrives on quantitative metrics, it's easy to overlook the qualitative. Receiving constructive feedback is an invaluable part of the learning process. Analysts must create an environment where feedback—both giving and receiving—becomes second nature. This culture of open dialogue helps refine analytical methods and enhance the precision of insights.
For instance, an analyst may present a data report and receive input on how to interpret certain trends more accurately or how to visualize data more effectively. Embracing this feedback, rather than shying away from it, leads to noticeable improvements in work quality over time. Here are several strategies to foster an environment rich in constructive feedback:
- Regular peer reviews: Collaborative evaluations can illuminate areas for improvement.
- Establishing mentorship: Learning from seasoned analysts can provide guidance and insights that one might not always foresee.
- Soliciting input from stakeholders: Understanding end-user perspectives can greatly enhance the relevance of analyses.
"Feedback is the breakfast of champions."
In summary, the duo of continuous learning and embracing feedback is not just a passing trend; it forms the cornerstone of a proficient data analyst’s career. By staying curious and receptive to improvement, analysts can assure they remain effective players in the data-driven world.
Ethical Considerations in Data Analysis
In an era where data is hailed as the new oil, ethical considerations in data analysis cannot be brushed under the rug. For data analysts, it is crucial to be not only proficient in interpreting data but also in handling it responsibly. Organizations today are increasingly aware of the implications that can arise from mishandling data. As guardians of data insights, analysts have a responsibility to uphold the principles of ethics and integrity.
Ethical considerations serve multiple purposes. They protect the rights of individuals, ensure transparency in operations, and foster trust between stakeholders. An ethical approach helps prevent data breaches and misuse, ensuring compliance with regulations such as the General Data Protection Regulation (GDPR). Adhering to these principles also promotes a culture of accountability where the data-driven decisions made within the organization are based on sound practices.
Understanding Data Privacy and Security
When delving into data analysis, privacy and security stand as paramount concerns. Data privacy refers to the proper handling of sensitive information, whereas data security emphasizes protecting that information from unauthorized access. Data analysts must be aware of the different types of data they handle.
- Personal Identifiable Information (PII): Information that can be used to identify an individual, such as names, addresses, and social security numbers.
- Health Information: Any data concerning a person’s health status, including medical records.
- Financial Information: Data that relates to an individual’s financial standing, such as bank details and credit scores.
To ensure data privacy and security, analysts should implement measures such as:
- Encrypting sensitive data to safeguard it from unauthorized access.
- Anonymizing data where applicable to remove identifiable information.
- Regularly auditing data access logs to identify potential breaches.
Overlooking these precautions can lead to significant repercussions, not just for individuals but for organizations as a whole. In essence, understanding and implementing robust safety measures not only protect the data itself but also sustain the organization’s reputation.
Navigating Ethical Dilemmas in Analysis
In the intricate realm of data analysis, ethical dilemmas often surface, creating a moral maze for analysts to navigate. These dilemmas manifest in several forms, particularly in the context of data interpretation and usage.
A notable dilemma involves data biases. Data does not exist in a vacuum; it can reflect societal biases. This means analysts must tread carefully when drawing conclusions from data, ensuring that their interpretations do not perpetuate stereotypes or mislead stakeholders. For instance, if a hiring algorithm favors candidates from certain demographic backgrounds, it could lead to discriminatory practices.
"Data can tell a powerful story, but it’s the responsibility of analysts to ensure that story is told ethically."
Moreover, there’s the challenge of balancing business objectives with ethical considerations. Organizations often aim for aggressive growth strategies that may tempt analysts to adopt questionable practices, such as exploiting loopholes or using data without consent. This is where a strong ethical compass plays a pivotal role.
- Transparency: Always communicate the methodologies used in data analysis to stakeholders.
- Accountability: Acknowledge unethical practices when they arise and put measures in place to rectify them.
- Informed Consent: Ensure that data collected from users is done so with their knowledge and agreement.
Navigating these situations requires not just analytical skill but also a solid grounding in ethical principles. Analysts must be advocates for ethical practices within their organizations, pushing back against pressures that might encourage unethical behavior. This safeguards the integrity of the findings and ultimately ensures that data serves its intended purpose without compromising individual rights.