Estimating Azure Data Factory Costs: A Complete Guide
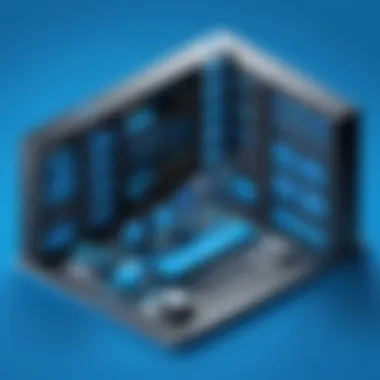
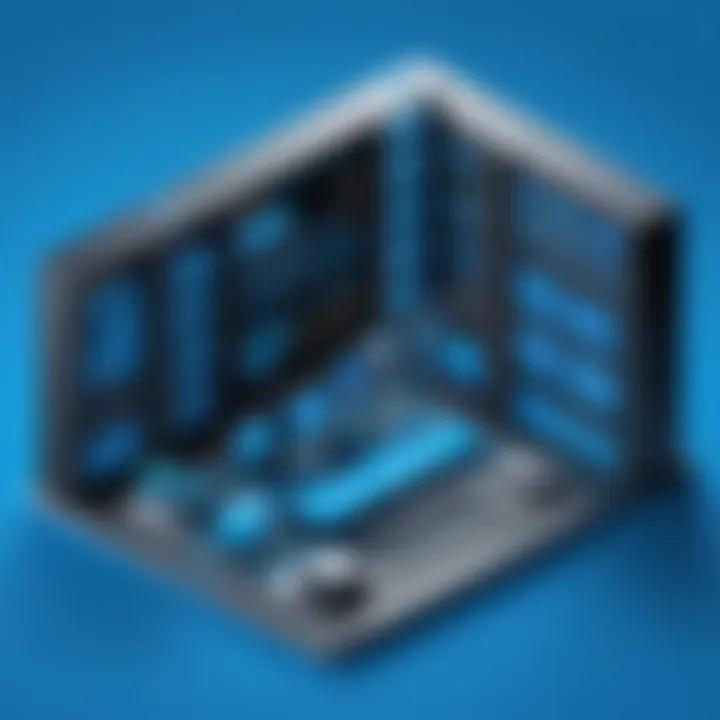
Intro
Navigating the landscape of data integration and analytics, Azure Data Factory emerges as an essential tool for many organizations. Whether you're moving datasets, transforming data, or orchestrating complex workflows, understanding the costs associated with Azure Data Factory is a key factor in maximizing your investment. The intricacies of its pricing model can often feel like navigating a minefield, especially for IT professionals and decision-makers who need to make informed budgetary choices.
This guide aims to dig deeper into estimating costs within Azure Data Factory, breaking down various pricing elements and the factors that drive them. From data movement to data flow and other hidden charges, we'll shed light on all the pieces of the puzzle. Moreover, we'll provide practical strategies for optimizing costs, making sure your organization can effectively utilize this robust service without breaking the bank. As we embark on this detailed exploration, you'll gain a sound understanding of how to curate accurate estimates that align with your specific projects.
Let's get started.
Understanding Azure Data Factory
When it comes to cloud-based data integration and analytics, Azure Data Factory certainly deserves your attention. It's not just another tool in the toolbox; it's a comprehensive solution that facilitates the movement of vast amounts of data across various services and platforms. This section aims to dissect what Azure Data Factory is, highlighting why understanding it is essential for anyone who deals with data management or cost estimation.
What is Azure Data Factory?
In simple terms, Azure Data Factory is a cloud-based data integration service provided by Microsoft Azure. It allows you to create, schedule, and orchestrate data workflows at scale. Imagine needing to pull data from multiple sources—like SQL databases, Excel sheets, or even web services—and transform it into a unified format suitable for analysis or reporting. Well, Azure Data Factory handles that with finesse.
This tool supports various data movement activities, such as copying or transforming data, which can then be stored in a data lake or transformed into an analytical model. Upon its staggering capabilities, significance emerges, particularly for organizations striving to adapt to data-driven decision-making.
Key Features and Benefits
Understanding Azure Data Factory also involves knowing its critical features and the benefits they offer.
- Hybrid Data Integration: Azure Data Factory supports not just cloud-based integration but also on-premise sources. This makes it suitable for businesses operating in a hybrid environment, allowing for seamless data operations without boundaries.
- Code-Free Data Flow: Users can design data flows using a visual interface, enabling even those with limited coding experience to create complex data transformations. This can save time and reduce errors that often accompany manual coding.
- Scalability: The platform handles massive workloads efficiently. Whether you are processing gigabytes or petabytes of data, Azure Data Factory scales according to the needs of your organization, letting you focus on your projects rather than infrastructure limitations.
- Integration with Other Azure Services: It's not just a stand-alone tool; it seamlessly integrates with various Azure services, including Azure Machine Learning and Azure Databricks. Such versatility magnifies its value proposition, making it a central piece in the Azure ecosystem.
- Cost Efficiency: Even though we're diving deep into costs in later sections, having a solid understanding of how Azure Data Factory can optimize data workflows can result in significant cost savings.
Understanding Azure Data Factory isn’t just about knowing its functionalities; it’s about grasping how it can transform complex data pipelines into efficient operations, thereby impacting your bottom line directly.
In summary, Azure Data Factory serves not just as a conduit for data but as a powerhouse for transforming how data is managed, processed, and analyzed. Once you have a firm grasp of what it entails, dovetailing this understanding with accurate cost estimations becomes much more straightforward, ultimately leading to more effective data strategies.
The Significance of Cost Estimation
Estimating costs accurately is paramount when operating within Azure Data Factory. It’s the bedrock that enables organizations to make informed financial decisions and optimize their resources. Understanding how various components contribute to the overall spend helps avoid unwelcome surprises and aligns the technological capabilities with financial strategies.
Moreover, accurate cost estimation fosters a culture of accountability. Teams are better equipped to manage and allocate budgets efficiently, ensuring that resources are utilized for maximum return on investment. In a nutshell, it’s not just about counting pennies but rather about weaving cost-awareness into the fabric of decision-making processes.
Why Accurate Cost Estimates Matter
Getting your cost estimates right can feel like trying to hit a moving target — it’s an ongoing balancing act. But here’s a truth bomb: when organizations fail to predict costs accurately, they may find themselves caught in a financial tight spot.
For instance, consider a company that underestimates the expenses associated with data movement and transformation in Azure Data Factory. Suddenly, costs can spiral out of control, leading to either budget cuts in other essential areas or overspending that jeopardizes the organization’s financial health.
Accurate estimates are like a roadmap. They provide a clear direction for budget allocation, allowing for necessary investments in data initiatives without straining other departments. Moreover, they help to anticipate future expenditures based on historical data, enabling businesses to plan for growth or unexpected developments effectively.
Implications for Budget Planning
Crafting a solid budget plan is akin to piecing together a jigsaw puzzle. Each piece, representing a different cost component in Azure Data Factory, needs to fit seamlessly with the others to create a complete picture. If even one piece is misestimated, the integrity of the entire budget is compromised.
- Visibility: Cost estimation gives stakeholders insight into financial needs across the board. Visibility into each component—from data movement to pipeline costs—means less guesswork and more strategic planning.
- Flexibility: By accurately estimating costs, organizations can create a flexible budget that can adapt to changing circumstances without hampering operational capabilities. This adaptability is crucial in the fast-paced tech environment.
- Strategic Investments: A well-informed budget allows companies to prioritize which projects to invest in. For example, if the cost estimates indicate that certain data workflows may lead to significant savings or enhanced capabilities, it can justify focusing resources in that area.
- Risk Mitigation: For many organizations, surprises are synonymous with risk. Just as an athlete studies their opponents, understanding all elements of their cost structure enables companies to anticipate and minimize risks related to budget overruns.
Accurate cost estimates aren’t merely figures on a spreadsheet; they are the foundation for creating a sustainable and actionable financial framework that organizations can rely on for growth.
In essence, the significance of cost estimation in Azure Data Factory extends beyond immediate costs. It shapes overall business strategy, influences operational efficiency, and ultimately defines how well an organization can leverage data in an increasingly data-driven world.
Understanding Pricing Components
Understanding the pricing components of Azure Data Factory is crucial for anyone looking to manage their budget effectively while leveraging this powerful tool for data integration and analytics. Each pricing element has implications not just on total expenses but also on the strategic decisions made regarding data operations. It's like piecing together a puzzle—recognizing how each component interlocks helps you form a clearer picture of your costs and assists in optimizing your financial investment.
When considering Azure Data Factory, knowing where the money goes is half the battle in managing expenses. Therefore, understanding pricing components allows organizations to anticipate upcoming costs based on usage patterns, helping finance teams to align their budgets accurately with operational needs.
Data Movement Costs
Data movement costs are at the heart of Azure Data Factory's pricing structure. This cost category relates to the process of transferring data across various services or locations within the Azure ecosystem and beyond. By understanding this component, businesses can make informed decisions on how and where to execute data movement. A few key points include:
- Geographic Considerations: Moving data across different Azure regions or from on-premises to cloud incurs varying fees. Each transfer to a new locale has its price tag.
- Volume Matters: The amount of data being moved significantly influences costs. You may want to review patterns of data generation to ensure you're only relocating what's necessary.
- Transfer Rates: Keeping an eye on transfer rates can help identify peak times for bulk data movements, potentially allowing for more strategic scheduling.
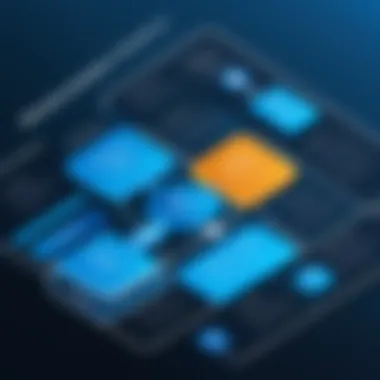
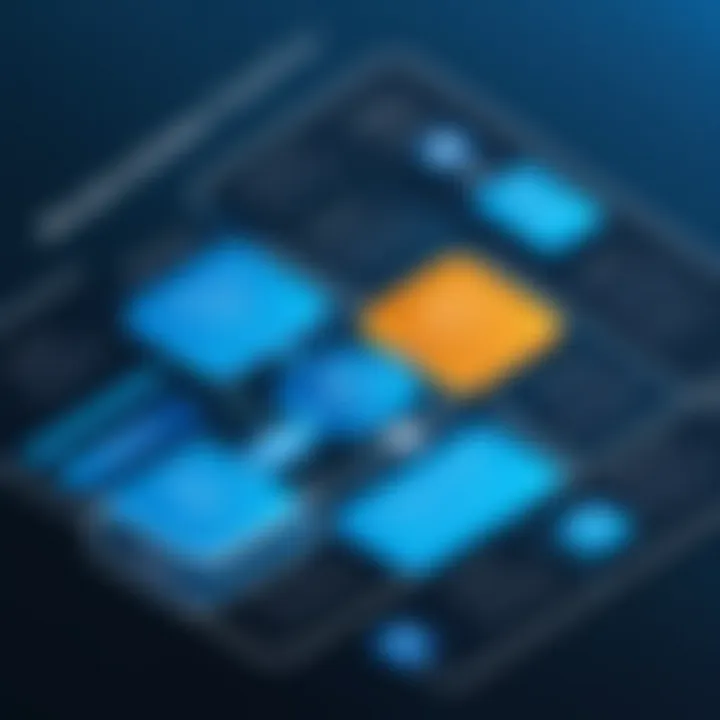
This cost is not just another line item; it can be substantial, especially for organizations handling vast datasets frequently.
Data Pipeline Costs
Data pipeline costs reflect the charges associated with processing data through various stages in Azure Data Factory. Each pipeline can involve intricate tasks ranging from data preparation to transformation, and understanding these costs is essential for controlling overall expenditures. Significant elements include:
- Number of Activities: Each pipeline can include multiple activities, such as data copy, transformations, and integrations, each with its cost. A complex pipeline requires careful consideration to avoid unexpected expenses.
- Execution Time: The duration of pipeline execution plays a role in billing. Longer-running pipelines might not just take valuable CPU time but also wallop your budget.
- Triggers: Using triggers to automate pipelines can reduce manual intervention, but it's vital to gauge how frequently they execute to estimate costs accurately.
Data Integration Costs
Finally, data integration costs encompass the expenses tied to integrating disparate data sources within Azure Data Factory. This integration is essential for creating a coherent data landscape, but knowing the cost structure is equally important. Key considerations include:
- Source Variety: Each source has potentially different fees associated with integration. Be mindful of the types of sources you integrate and how those relationships might impact your budget.
- Service Features: Azure Data Factory provides multiple integration services, each differing in cost. Choosing the right blend of services can create efficiencies, but it requires a solid grasp of pricing mechanisms.
- Data Transformation: Transforming data during the integration can rack up additional costs, depending on the complexity and tools used. If transformations are routine, consider reviewing them for optimization opportunities.
Understanding these components arms IT professionals, data engineers, and budget planners with essential insights, enabling them to make smarter, cost-effective decisions. By mapping out costs, you can better align your data strategies with business goals, ensuring your journey with Azure Data Factory is both successful and financially sound.
"A clear understanding of pricing components turns financial uncertainty into strategic alignment."
Factors Influencing Azure Data Factory Costs
Understanding the costs linked with Azure Data Factory involves analyzing several critical factors. These elements not only affect overall expenditures but also highlight opportunities for optimization. When organizations navigate the world of data integration and workflows, it’s crucial they become well-acquainted with these influences to avoid any financial surprises down the road.
Data Volume
The sheer amount of data being processed stands as one of the leading factors affecting costs in Azure Data Factory. Companies working with massive datasets will incur higher charges because Azure prices services on a pay-per-use model. This means that while efficient data handling can lead to significant insights, it can also stretch budgets thinner than usual. For example, let’s say a business handles 10 terabytes of data as opposed to 1 terabyte; the difference in data movement charges can be staggering.
Long story short, organizations need to assess their data volume carefully. It’s not just about what comes in and what goes out; it’s about how often that data needs to flow. Identifying peak usage times for data can help manage these costs more effectively.
"In the age of big data, less can often be more. Optimize what you have instead of just piling on more."
Frequency of Data Refresh
Another important consideration is how often the data requires refreshing. Organizations that need real-time analytics will find themselves refreshing data constantly, thus incurring ongoing costs. Conversely, businesses with less pressing data refresh needs may find that batching data updates can lead to significant savings. The costs associated with triggering and executing pipelines increase with frequency. For instance, a company updating its sales data hourly will face much different cost dynamics than one doing it weekly.
In addition to considering refresh rates, organizations should also think about using API calls wisely. An established frequency could lead to predictable costs, helping to avoid the sudden surges in monthly billing that many companies face unexpectedly.
Complexity of Data Workflows
Complexity plays a significant role, too. The more intricate a data workflow, the more resources and time it will demand. Workflows involving multiple data sources, transformations, and integrations are naturally going to incur higher costs. For instance, a workflow that pulls data from three different systems, cleanses it, and then integrates it into a data warehouse is going to cost significantly more to run than a simpler setup that only handles basic data movements.
Thus, it's about finding the balance: complexity can be justified when the insights gained lead to greater revenue or other benefits, but unnecessary convolutions should be avoided. Emphasizing clarity in workflow design not only aids in effective operations but can also keep costs in check.
In summary, watching these three factors—data volume, frequency of data refresh, and complexity of workflows—is essential for any organization looking to utilize Azure Data Factory without breaking the bank. Careful planning and monitoring can go a long way in ensuring that spending aligns with business goals.
Cost Estimation Methodologies
Estimating costs in Azure Data Factory isn't merely a cursory glance at numbers on a conveyor belt. Rather, it’s a finely tuned practice that can save your organization a significant chunk of change. When dealing with extensive data flows and intricate pipelines, understanding the methodologies behind cost estimation becomes vital. This section encapsulates two prominent methodologies: Top-Down and Bottom-Up. Each approach has its nuances, benefits, and considerations that can help tailor a cost estimation strategy that fits the unique demands of any project.
Top-Down Cost Estimation
In the realm of Azure Data Factory, the Top-Down Cost Estimation method starts from the macro level. It’s akin to gazing at an expansive map before setting out on a journey. This approach pulls in historical data, enterprise-wide projections, or overall budgetary allocations, allowing you to estimate costs by breaking them down across various departments or projects.
The beauty of this method lies in its simplicity. Organizations can assess their entire spend on Azure services and allocate resources accordingly. Think of it as piecing together a jigsaw puzzle — starting with the bigger picture before zooming into the finer details.
Key elements to consider with this methodology include:
- Historical Spending Trends: Understanding past expenditures can guide future estimations, making your budgeting more reliable.
- Project Scope and Scale: Larger projects often demand more resources and incur higher costs, so reflect on the scope before making assumptions.
- Overall Budget Constraints: Ensure whatever estimate you devise aligns with the organization's financial health.
This method can be a solid choice for large organizations with established budgeting practices, as it uses the bird’s eye view to anticipate costs rather than getting bogged down by minutiae. However, the major downside lies in its potential lack of detail, especially for specific projects or agile initiatives.
Bottom-Up Cost Estimation
Conversely, the Bottom-Up method digs deep. This approach emphasizes meticulous analysis of each component of the project, from start to finish. Imagine constructing a building; you wouldn't just throw a budget together without knowing the cost of bricks or labor.
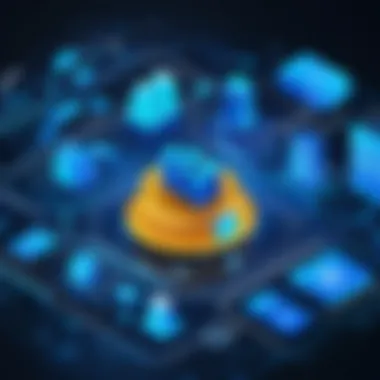
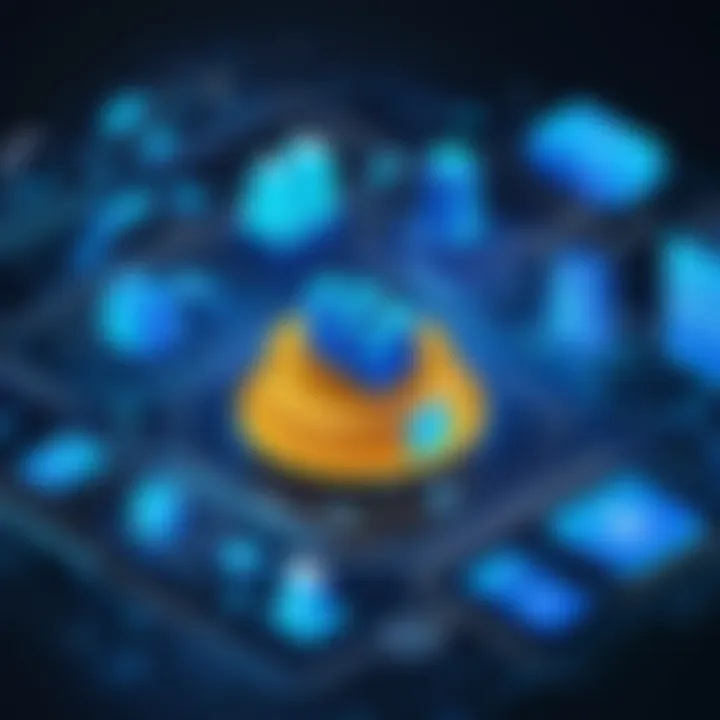
In the Bottom-Up approach, team members decompose tasks, estimating costs on a granular level. This laser-focused style provides a detailed insight into all aspects of the Data Factory ecosystem, allowing for more precise budgeting.
Fundamental aspects include:
- Detailed Task Assessment: Every task or pipeline should have its cost assigned, covering everything from data ingestion to compliance measures.
- Resource Utilization: Each estimated cost is linked to resources being utilized, making it clearer to pinpoint potential wastage.
- Feedback Loop: By collecting input from various teams, the estimates become more accurate, highlighting any overlooked factors.
Utilizing Bottom-Up can lead to precise cost projections that are especially crucial for projects in the early phases or those with uncertain requirements. However, the drawback is often the time required to gather and analyze this information, which can be quite taxing.
Accurate cost estimation, regardless of the method, helps your organization navigate the sea of data efficiently.
Both methodologies offer distinct advantages and potential pitfalls. Ultimately, the choice may depend on the organization’s size, project scope, and the level of detail required. Integrating aspects of both could create a hybrid model that brings a depth of insight coupled with a broader understanding of costs.
Using Azure Pricing Calculator
Understanding the costs associated with Azure Data Factory goes hand-in-hand with leveraging the Azure Pricing Calculator effectively. This tool acts as a compass, guiding organizations through the murky waters of budget estimations. With it, one can forecast expenses with notable precision, making it a cornerstone in financial planning for data integration tasks. The importance of using this calculator cannot be overstated, especially for IT professionals who must navigate complex pricing structures that can change with every evolving requirement.
The Azure Pricing Calculator enables users to customize estimates based on several specific parameters unique to their data processes. This customization can greatly affect the overall financial picture. Budget stakeholders need to be aware that various elements—like data movement frequency and the complexity of workflows—can drastically alter cost projections. By familiarizing themselves with the tool, teams can avoid unexpected financial surprises down the line.
Overview of the Pricing Calculator
The Azure Pricing Calculator is a user-friendly web-based tool designed to assist individuals and organizations in estimating the costs associated with various Azure services, including Azure Data Factory. The interface is straightforward, offering an easy way for users to select the services they plan to utilize.
Key features include:
- Multiple pricing tiers: Users can select service levels based on their needs, such as standard or premium options, which allow for better budgeting.
- Detailed breakdown of costs: Each component's cost is listed clearly—this helps in understanding how each factor contributes to the total estimate.
- Real-time updates: As Azure services evolve and pricing changes, the calculator updates dynamically, ensuring users have the most accurate information.
The tool allows organizations to plan for both current and future data projects. With the costs itemized, budgeting becomes less like a shot in the dark and more of a strategic endeavor.
Step-by-Step Cost Estimation Process
Utilizing the Azure Pricing Calculator can seem daunting at first, but breaking it down into manageable steps simplifies the process significantly. Here’s how to effectively estimate costs:
- Access the Calculator: Navigate to the Azure Pricing Calculator via the Azure website.
- Select Azure Data Factory: Search for Azure Data Factory from the list of services available. Click on it to add it to your estimation.
- Define the parameters: Assign specific variables like the volume of data to be processed, frequency of operations, and data movement considerations:
- Review cost options: After setting your parameters, review the calculated costs. The tool will show total estimated costs as well as breakdowns for each component.
- Adjust and refine: If initial estimates are not aligning with budget expectations, adjust parameters. This iterative approach can lead to insightful adjustments that improve budget alignment.
- Export your estimation: Utilize options to export your cost estimation so it can be shared with budget stakeholders for review.
- Data Volume: Input how much data you expect to move.
- Frequency: Choose how often you will run data jobs.
- Workflow Complexity: Specify whether you will use simple or complex data pipelines.
By following these steps, organizations can develop a clearer financial model, aiding in better decision-making for their data initiatives.
"Budgeting is not just about numbers; it’s about understanding the implications behind those numbers and planning effectively".
Practical Tips for Cost Management
Managing the costs associated with Azure Data Factory is not merely a matter of luck or hope. It requires a valid strategy, careful planning, and ongoing monitoring. The right practices can lead to savings that add up over time. This section discusses practical approaches aimed at ensuring that organizations not only understand their spending but also actively manage it. The benefits of proactive cost management extend beyond immediate savings; they pave the way for optimized performance and smarter resource allocation.
Monitoring and Analyzing Costs
To truly get a grip on expenditures, you need to keep an eagle eye on costs. Monitoring and analyzing costs isn't just a checkbox on a task list—it's an ongoing process. Start by implementing Azure’s built-in monitoring tools. These tools allow users to visualize cost data over time, helping to identify trends. It’s vital to establish baseline costs to compare against future months or projects.
- Integrate Azure Cost Management + Billing to track spending.
- Set up alerts for threshold spending to catch potential budget overruns early.
Analyzing historical data can offer insights into patterns that future projects may follow. For example, a spike in data movement costs during a specific month might correlate with a major project launch. Understanding these patterns helps organizations make more informed decisions about resource allocation.
"If you don’t know where your money’s going, you can’t figure out how to keep it in your pocket."
Conducting Regular Reviews of Expenses
Just like regular check-ups at the doctor’s office, reviewing expenses regularly helps catch any issues before they balloon into larger problems. This practice involves looking into both fixed and variable costs associated with Azure Data Factory. Consider setting a schedule for reviews, perhaps quarterly or biannually, to assess how costs align with initial estimates and budget expectations.
- Consider using a checklist during reviews to cover essential points:
- Were any unexpected costs incurred?
- Are there ongoing projects that have exceeded their predicted budgets?
- Is data movement happening more often than initially planned?
Having a dedicated team or individual responsible for conducting reviews fosters accountability. It's not simply about observing; it's about asking questions and digging deeper into the why behind the numbers. By doing this, businesses can adapt strategies and stay ahead of potential financial issues.
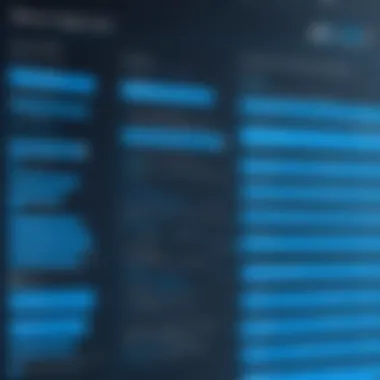
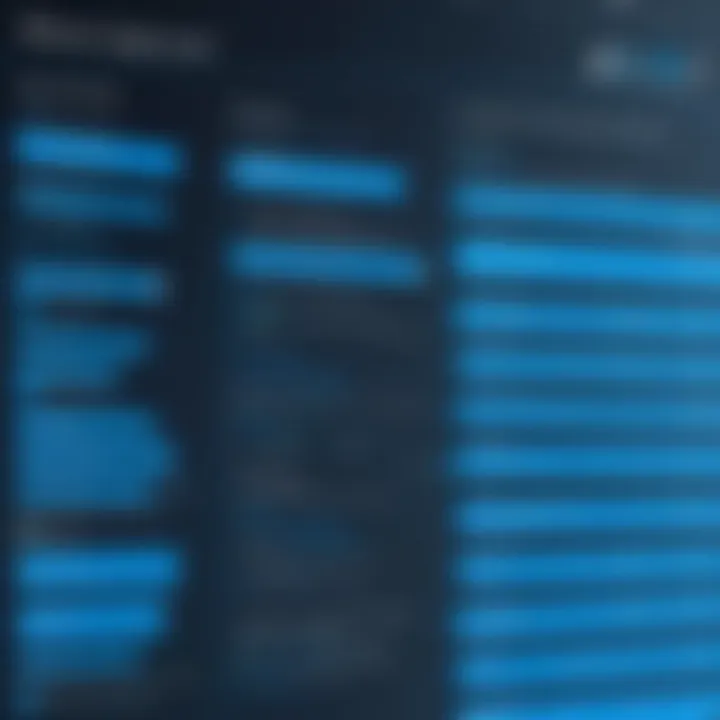
Case Studies of Cost Estimation
When talking about cost estimation, real-world examples offer invaluable insights. It’s one thing to discuss theories, but seeing how different industries apply Azure Data Factory to manage costs can paint a clearer picture. Case studies present tangible evidence of strategies that can work—or don’t work—when estimating costs. They shed light on specific elements like cost control, data efficiency, and integration challenges while allowing readers to glean lessons from successes and failures. This section reveals not just the outcomes but also the thinking behind the decisions made by these industries.
Industry A: Successful Cost Management
In the retail sector, one major chain faced hefty data movement costs that threatened to leave a significant dent in their operations budget. By implementing Azure Data Factory, they streamlined their data flows from various sources—inventory, sales, and customer interactions. They began leveraging data integration pipelines to automate data movement to analytics environments.
They closely monitored their data usage via Azure's built-in monitoring tools. As a result, they reduced their monthly data transfer costs by 30%.
This success didn’t come overnight. The team first crafted a hard-nosed estimation of potential expenses before rolling out Azure. They consulted the Azure Pricing Calculator to assess the likely costs linked to their data volume and refresh rates. What followed was an iterative process where they fine-tuned their strategies based on real time monitoring.
"Being proactive about our cost estimates turned out to be our best decision. We didn’t just pinch pennies; we found ways to improve data strategy, ultimately boosting our efficiency and reducing wastage," reported the IT manager of the chain.
Industry B: Overcoming Budget Challenges
Contrasting the retail example, the healthcare sector paints a different image regarding cost estimation and Azure Data Factory. One healthcare provider found itself juggling between various data sources, including electronic health records and patient management systems. Their costs skyrocketed due to an influx of data, and their previous estimation methods fell flat.
To navigate budgetary concerns, they employed a bottom-up cost estimation approach. The IT team documented every task involved in data movement, integration, and processing with Azure, creating a granular understanding of where expenses lay.
They also established a governance framework to regularly evaluate all associated costs, bringing more visibility to their spending. As a result, they managed to cut unnecessary expenditures by up to 25%.
Through consistent reviews and by engaging business stakeholders regularly, they aligned their strategies towards more effective data management that adhered to budget constraints without sacrificing quality.
Together, these cases illustrate the dual-edged sword of cost estimation—while one can succeed through effective planning, missteps can lead to overspending. Knowing the path taken by others could inspire new thinking for those in the trenches of Azure Data Factory implementations.
The Role of Azure Data Factory in Digital Transformation
Azure Data Factory is not just a tool for data integration; it serves as a crucial component in the broader scope of digital transformation for organizations. In today’s fast-paced business landscape, leveraging data effectively can set a company apart from its competitors. By integrating Azure Data Factory into their infrastructure, organizations can enhance their data strategy, streamline workflows, and ultimately create more value from their data resources.
Integrating Azure Data Factory into Business Strategy
Integrating Azure Data Factory into a business strategy begins with recognizing that it’s not merely about data movement but about fostering a data-driven culture. Companies must align their data pipelines with business objectives. This means outlining a clear plan regarding how data will be pulled, transformed, and utilized to meet strategic goals.
- Building Data Pipelines: Organizations should focus on creating robust and flexible data pipelines using Azure Data Factory. This allows seamless movement of data from various sources, ensuring timely access to critical information.
- Analytics and Insights: Utilizing the integration capabilities of Azure Data Factory allows businesses to analyze data in real-time. By connecting to advanced analytics tools, companies can derive insights that are actionable and directly related to their business needs.
- Collaboration: With multiple stakeholders involved in data management, Azure Data Factory fosters collaboration. Teams can work together on data workflows, ensuring that everyone is on the same page regarding data usage and strategy.
By making Azure Data Factory a pillar of their data strategy, organizations can enhance efficiency, reduce errors, and improve overall agility in responding to market changes.
Evaluating Long-Term Value versus Short-Term Costs
When considering the implementation of Azure Data Factory, financial factors should not be overlooked. Companies often grapple with the tension between immediate expenses and long-term benefits. While the initial investment might seem steep, the focus should shift to evaluating the overall value that data integration can bring to the business.
- Cost-Benefit Analysis: It’s essential to weigh the costs of using Azure Data Factory against the potential savings from improved efficiencies. Questions to ponder include: How much time will automating data workflows save? What revenue opportunities could arise from better data insights?
- Scalability: One of the significant advantages of Azure Data Factory is its scalability. As the business grows, so too can the data operations. This can minimize long-term costs as adjustments are made on-the-go rather than incurring large expenses upfront.
- Risk Reduction: Inaccurate data can lead to poor decision-making, and Azure Data Factory helps mitigate that risk. Investing in accurate and timely data ultimately leads to better strategic decisions, making the long-term financial outlook brighter.
Ultimately, investing in Azure Data Factory represents a strategic move towards setting a company up for future success, weighing the initial costs against the lasting value it can provide in terms of operational efficiency and enhanced decision-making capabilities.
By understanding the role that Azure Data Factory plays in digital transformation, businesses are better positioned to make informed decisions that align technological investments with overarching business strategies.
Future Considerations for Azure Data Factory Costs
In the landscape of data integration and analytics, keeping an eye towards future trends and potential shifts in pricing models is crucial for organizations utilizing Azure Data Factory. As businesses increasingly rely on data-driven decision-making, understanding these dynamics can help in forecasting expenses and optimizing their fiscal strategies.
Emerging Trends in Data Technologies
The field of data technologies is constantly evolving, with fresh innovations and methodologies surfacing regularly. Among these trends, the rise of artificial intelligence and machine learning is making waves, automating many processes that were once manually intensive. When integrated with Azure Data Factory, these technologies can enhance data processing capabilities and significantly reduce operational costs.
Moreover, cloud-native architectures are gaining traction, allowing data solutions to be more flexible and scalable than traditional setups. This shift often leads to changes in how costs are structured, as new pricing models emerge to accommodate these advancements. For instance, pay-as-you-go pricing is becoming a common approach, giving organizations greater control over their expenditures while scaling up or down based on their data needs.
Another notable trend is the increasing focus on data governance and compliance. With regulations growing stricter, investing in compliance-focused features can mitigate risks, albeit at an increased cost. Organizations must weigh these benefits against their budget when considering implementations of Azure Data Factory that align with regulatory standards.
In summary, staying abreast of these trends ensures organizations can proactively adapt their cost estimations for Azure Data Factory, and thus maintain a competitive edge.
Anticipating Changes in Pricing Models
Pricing models for cloud services, including Azure Data Factory, are not static; they evolve in response to market demands and technological advancements. As stakeholders evaluate their options, it’s important to consider anticipated shifts in pricing.
For one, bundled services might become more prevalent, as providers aim to enhance value offerings. This could lead to a restructuring of how individual service costs are calculated. An understanding of these changes can prevent budgeting surprises as organizations might spend more or less based on the packages they choose.
Another factor to consider is the potential introduction of tiered pricing structures. If Azure Data Factory adopts this approach, businesses will need to assess their usage patterns closely to avoid incurring excess costs associated with tiers that exceed their data processing needs.
In addition, external market forces, like changing energy costs and cloud competition, may compel Azure to rethink its pricing strategies. Companies must keep an ear to the ground, as these external factors can significantly affect their operational budget.
"Adaptability to market shifts isn't just a strategy—it's a survival skill in today’s data-centric world."
To conclude, anticipating these changes is more than just prudent; it's foundational for effective financial planning. Businesses that stay ahead of the curve can harness Azure Data Factory's capabilities without getting bogged down by unexpected expenses.