Exploring Ethical AI and Machine Learning Initiatives

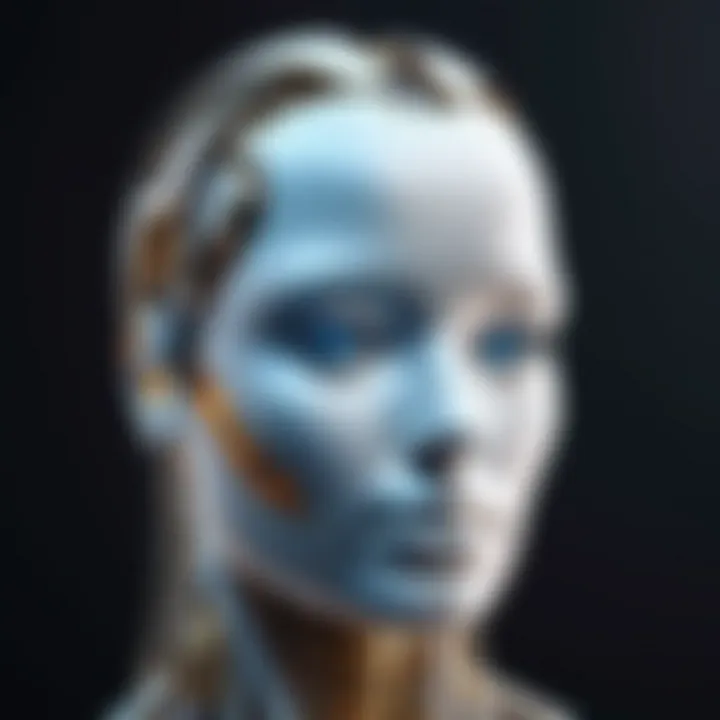
Intro
The Institute's Mission
"Ethical AI is not just about preventing harm; itâs about promoting a future where technology serves humanity's best interests."
Framework for Ethical AI
To tackle the ethical dilemmas that arise in AI and machine learning, the institute has laid out several key guidelines:
- Transparency: Systems should be designed with clarity in mind, allowing users to understand how decisions are made.
- Accountability: Developers and organizations must take responsibility for the outcomes generated by their AI systems.
- Inclusivity: AI solutions should consider diverse perspectives, ensuring that they benefit all sections of society.
- Fairness: Mechanisms must be in place to prevent bias in AI systems, providing equal treatment regardless of user demographics.
These principles serve as a foundation for the instituteâs initiatives and collaborations, shaping the broader AI landscape.
Collaborations and Partnerships
The role of the institute extends beyond just shaping guidelines. They actively engage with various stakeholders to leverage shared knowledge and resources.
- Academic Institutions: Collaborating with universities helps in the creation of cutting-edge research and educational programs.
- Industry Leaders: By partnering with corporations, the institute influences best practices and standards in tech development.
- Government Bodies: Engaging with policymakers ensures that ethical considerations are integrated into legislation affecting AI technologies.
In partnership with these various entities, the institute works tirelessly to embed ethics into the programming of AI.
Challenges Ahead
Despite the institute's efforts, it faces several adversities:
- Rapid Technology Changes: The speed at which AI evolves can outpace the establishment of corresponding ethical guidelines.
- Global Disparities: Different regions of the world have vastly different approaches to AI ethics, complicating collaborative efforts.
- Public Trust: As AI becomes more prevalent, concerns about privacy and misuse continually grow. Restoring trust among the general populace is a significant challenge that has to be addressed.
As these challenges loom, the institute remains committed to fostering a dialogue that prioritizes ethical integrity in AI and machine learning.
End
Preamble to the Institute
AI systems are increasingly embedding themselves in daily life, from job recruitment to law enforcement, and even healthcare diagnostics. Thus, understanding the foundation of this institute sheds light on its fundamental principles and objectives, which are crucial for safeguarding the integrity of AI practices worldwide.
The institute aims to instigate a paradigm shift. Rather than merely following prevailing trends, it seeks to forge pathways where ethics and practicality coalesce seamlessly. Education through workshops and forums is one such avenue; these initiatives enlighten stakeholders about the pressing ethical issues associated with AI deployment. Another vital aspect is fostering collaboration among various sectorsâtech, law, academia, and civil rights organizationsâto ensure that differing perspectives enrich the discourse surrounding ethical AI.
"The development of AI without ethical consideration is like driving a car with no brakes. You may be moving fast, but at what cost?"
Foundational Principles
- Transparency: Transparency in AI systems allows stakeholders to understand how decisions are made, thereby fostering trust in AI technologies.
- Accountability: If an AI system harms an individual or groups, accountability measures ensure that there are recourses for redress. Having mechanisms in place to address these issues is fundamental.
- Fairness: Addressing inequities in AI algorithms is paramount to avoid systemic biases that could exacerbate existing social issues. This principle aspires to equitable treatment across various demographic segments.
- Privacy: With increasing concerns around data privacy, emphasizing stringent privacy protections becomes paramount to secure users' information while fostering innovation.
These principles aren't mere words; they translate into actionable strategies that guide the formulation of AI policies and practices.
Objectives and Goals
- Educating Stakeholders: One of the primary aims is to foster a culture of learning, engaging policymakers, tech developers, and the public in meaningful dialogues about ethical AI.
- Establishing Frameworks: The institute seeks to develop comprehensive frameworks for ethical AI deployment that integrate practical guidelines with ethical imperatives. This may involve collaboration with international bodies to standardize best practices.
- Promoting Research: Initiating and supporting research initiatives that address pressing ethical questions around AI is vital. This research could scrutinize the intersections of technology, ethics, and society, uncovering new insights.
- Encouraging Collaboration: Building partnerships with businesses, government, and non-profits to share resources and knowledge is essential. These coalition efforts are designed to ensure collective responsibility in ethical governance.
Through these objectives, the Institute positions itself not only as a thought leader but also as a facilitator of change, ensuring that ethical considerations are holistically embedded within AI advancements.
Importance of Ethical AI
The landscape of artificial intelligence is rapidly evolving, making the need for ethical considerations more crucial than ever. The Importance of Ethical AI delves into safeguarding societal values while harnessing the power of technology. This section highlights key aspects that underline why ethical AI practices are pivotal not just for developers but for everyone who interacts with these systems.
Understanding Ethical Concerns
Addressing ethical concerns in AI is a multifaceted endeavor. One major area of concern revolves around bias. Algorithms can inadvertently reflect and reinforce historical inequalities if they're not carefully designed. For instance, a hiring algorithm trained on resumes from a predominantly male workforce may favor male candidates, perpetuating gender imbalances. This is a call for designers and developers to pay close attention to the data they use.
Moreover, there's transparency, or the lack thereof, in AI systems. An AI decision-making process can often resemble a black box, making it hard for users to grasp how outcomes are derived. This opacity can lead to distrust among users, especially when decisions have significant consequences.
Furthermore, privacy remains a hot-button issue. With AI technologies collecting vast amounts of personal data, ensuring that individuals are not subjected to unwarranted surveillance is paramount. Striking a balance between innovation and privacy rights is a tightrope walk for tech companies.
Implications for Society
Ethical AI has far-reaching implications for society as a whole. Here are some key aspects:
- Job Displacement: As automation increases, thereâs a growing fear about the future of work. Ethical approaches in AI can help mitigate these concerns by emphasizing retraining and upskilling workers affected by these changes.
- Accountability: When AI systems fail or lead to harm, the question arises: who is responsible? Establishing clear guidelines around accountability ensures that companies remain answerable for their AI's actions. This not only protects the public but also fosters trust in technology.
- Equity: We must ensure that the benefits of AI are distributed fairly. Toward that end, thoughtful implementations can prevent the digital divide from widening further, allowing marginalized groups to benefit from advancements in technology.
Ethical AI isn't just a trend; itâs a necessity. If left unchecked, the consequences can be dire, influencing everything from personal privacy to societal equity.
Current Trends in AI Governance
Keeping pace with the rapid development of artificial intelligence, the landscape of AI governance is shifting significantly. These shifts matter not just to tech enthusiasts or industry insiders, but to society at large. Ethical frameworks and legislative guidelines are getting more pronounced as stakeholders look to harness the power of AI responsibly. A well-defined governance structure is crucial because it determines how the technology is developed and deployed, ultimately impacting users and communities.
Significantly, the efficacy of regulation and collaboration can influence how visible access to AIâs transformative power becomes. The alignment of policy and practice can offer a sense of trust that people need when interacting with AI systems.
Legislative Developments
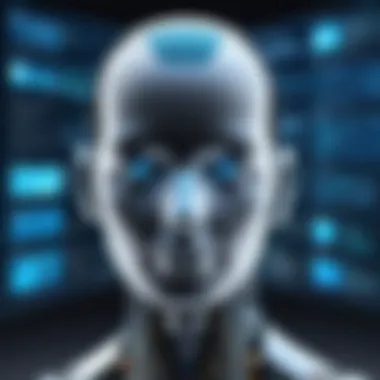
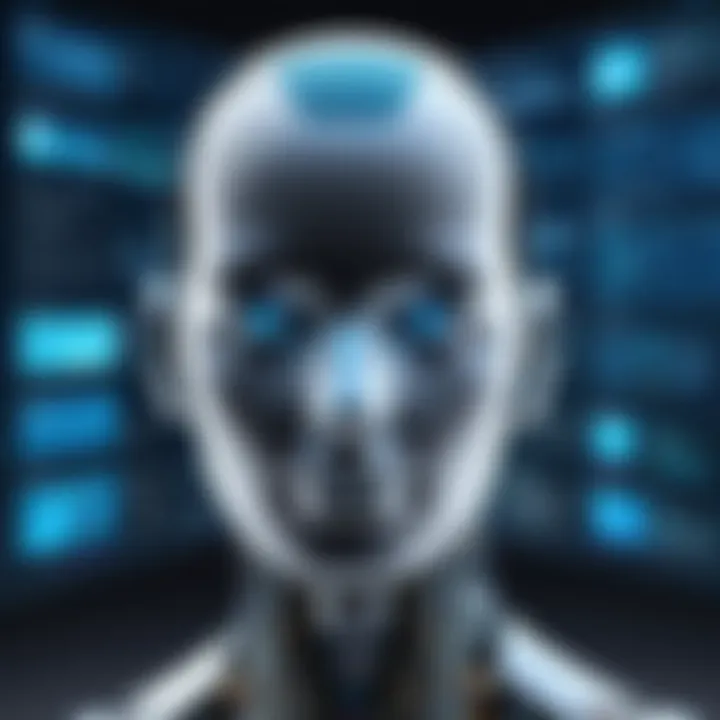
In recent years, there's been a palpable surge in legislative action regarding AI governance. Different countries are beginning to recognize the need for a structured approach. This clarity helps organizations navigate the tangled web of laws while also keeping citizens safe. Governments are proposing and enacting laws to address key issues like data privacy, algorithmic accountability, and transparency.
For instance, the European Union has been spearheading initiatives like the proposed AI Act. This framework attempts to categorize AI applications based on their risk, setting up a tiered approach to regulation. Low-risk categories could experience less stringent oversight, while systems deemed high-risk, such as those used in healthcare or criminal justice, are subject to intensive scrutiny.
"As AI technology grows more complex, legislation must be agile enough to adapt without stifling innovation."
This balancing act is tricky but essential. While legislation is vital for safeguarding public interests, it should not impose unnecessary burdens that deter innovation. Organizations must be aware of these developments, adjusting their strategies to comply without losing their competitive edge.
International Standards
With AI technologies crossing borders at lightning speed, the need for international standards becomes apparent. Various organizations are working towards establishing common frameworks to ensure consistency and safety in AI deployment.
The International Organization for Standardization (ISO) and the Institute of Electrical and Electronics Engineers (IEEE) have been particularly active in this realm. These bodies are developing standards that address the ethical use of AI, focusing on data handling, algorithmic fairness, and accountability measures. Having these international standards provides a benchmark against which organizations can measure their practices, fostering an environment where ethical AI use is not just an option but a norm.
However, achieving consensus on global standards has its challenges. Differences in culture, legal frameworks, and economic priorities can lead to varied interpretations of what constitutes ethical AI usage. Thus, fostering dialogue among stakeholders is paramount for achieving a cohesive global approach.
Frameworks for Ethical AI Implementation
The development and deployment of artificial intelligence technologies cannot exist in a vacuum. As AI continues to permeate various aspects of life, the necessity for a robust framework becomes evidentâone that ensures ethical considerations are woven into the fabric of AI practices. Implementing ethical frameworks is essential for guiding the actions of developers, policymakers, and other stakeholders in the AI landscape. Such frameworks can lend clarity to the often murky waters of ethical dilemmas, secure public trust, and help alleviate fears surrounding potential threats posed by AI.
Guiding principles embedded in these frameworks help balance innovation with responsibility. They ensure that as AI systems evolve, they do so with a conscientious approach towards impacts on individuals, communities, and society at large. This balance is pivotal in harnessing AIâs capabilities while mitigating risks. Frameworks for ethical AI implementation can lead to several notable benefits:
- Standardization of best practices across organizations.
- Increased accountability in how AI systems are designed and utilized.
- Establishing a culture of transparency, improving public trust.
- Encouraging collaborative efforts among various sectors and disciplines.
A carefully crafted framework informs users and creators on navigating the complexities of ethical AI, reinforcing the belief that technology must serve humanity and not the other way around.
Design and Development Principles
At the core of any framework for ethical AI implementation lie design and development principles, critical for ensuring that AI systems are aligned with ethical standards right from inception. The emphasis is on integrating ethical considerations into the design phase, which is often overlooked.
These principles may include:
- User-centric design: Focusing on the needs and rights of users ensures that systems are created with usability, accessibility, and fairness in mind.
- Diversity in design teams: Including individuals from varied backgroundsâbe it culture, gender, or expertiseâaccounts for diverse perspectives and reduces the risk of bias.
- Iterative development: This practice encourages frequent review and adaptation of AI systems based on user feedback and ethical assessments, ensuring the technology remains viable and just.
Moreover, commitment to sustainability and long-term impacts must feature prominently. By considering the ecological and societal implications right from the design phase, AI can be a force for good.
Assessment and Monitoring
Once an AI system is designed and developed, it requires continual oversight. This is where the assessment and monitoring component of ethical frameworks comes into play. Effective assessment ensures that the systems remain within ethical bounds throughout their lifecycle.
Key facets of this process include:
- Regular audits: Independently conducted assessments can illuminate issues like bias, transparency, and accountability.
- Real-time monitoring: Allowing for the tracking of AI systems while they function in society provides immediate insights into how they impact users and communities.
- Stakeholder feedback: Gathering insights from users and affected communities serves as a critical mechanism to adjust systems where necessary.
Taking a proactive stance on assessment and monitoring enables organizations to respond swiftly to ethical dilemmas and societal concerns. Those acting on such frameworks must be prepared to pull the plug or pivot when necessary. In essence, implementing such strategies not only safeguards the integrity of AI technologies but also fortifies public confidence in their adoption and usage.
"AI, if unchecked, can lead to unintended consequences. Ethical frameworks are not just a safety netâthey are essential for guiding AI towards a beneficial relationship with society."
In summation, frameworks for ethical AI implementation serve as foundational pillars guiding the responsible development and usage of AI technologies. Within these frameworks, design principles ensure ethical considerations are present from the very beginning, while ongoing assessment and monitoring safeguard against flaws that could emerge during operation. This structured approach not only benefits developers and organizations but ultimately promotes a society that can harness AI for the greater good.
Research Initiatives
Research initiatives form a cornerstone for the Institute's work in ethical AI and machine learning. These projects not only seek to push the boundaries of technology but also assess its alignment with ethical principles that are essential for responsible deployment. The goal isn't just about advancing technology; rather, it's about ensuring that such advancements lead to societal good without unintended consequences.
The importance of these initiatives lies in several key areas:
- Innovation in Ethical Practices: Research initiatives facilitate the exploration of innovative solutions to ethical dilemmas associated with AI technologies. By focusing on real-world applications, the Institute can test and refine frameworks that govern ethical practices in AI development.
- Evidence-Based Policies: These projects often generate data which can guide effective policymaking. Insights gleaned from research help to shape regulations that not only govern AI's development but also its deployment, ensuring accountability at every stage.
- Interdisciplinary Collaboration: Research initiatives frequently involve collaboration across fields such as sociology, psychology, and computer science. Such partnerships deepen the understanding of how AI technology interacts with societal dynamics, providing a holistic view of the implications of AI.
Collaborative Projects
Collaborative projects are an essential part of the Institute's strategy, facilitating partnerships among various stakeholders in the AI ecosystem. These collaborations often bring together industry leaders, academics, and policymakers, fostering a rich dialogue about ethical standards and practices in AI.
One notable example is the partnership with universities to create ethical benchmarks for AI algorithms. Involving academic researchers not only strengthens the validity of findings but also ensures that emerging voices in the field contribute to the debate on AI ethics. Such collaborative projects often lead to the establishment of new methodologies and best practices that can be integrated into tech development.
Moreover, the diversity of thought in collaborative settings sparks innovation. By harmonizing differing viewpoints, these projects can surface underrepresented ethical concerns that an isolated approach might overlook.
Funding Opportunities
Funding is a critical enabler for research initiatives dedicated to ethical AI. The Institute actively seeks financial support from various sources to fuel its projects. This funding isn't merely about sustaining operations; itâs a lifeline that helps transform innovative ideas into real-world implementations.
- Grants from Government Agencies: Government organizations often allocate funds specifically aimed at ethical AI activities. These grants can support wide-ranging projects, from deploying ethical frameworks to evaluating the societal impacts of AI technologies.
- Private Sector Investments: Increasingly, technology companies understand the importance of ethical considerations in AI. Some are providing funding to initiatives that align with their corporate social responsibilities. For instance, collaborations with companies like IBM or Google can lead to substantial financial support.
- Crowdfunding and Philanthropic Contributions: There is a growing trend of crowdfunding for ethical AI projects. This democratizes the funding process and allows for grassroots participation. Philanthropic organizations, too, are channeling funds into causes aligned with promoting responsible AI use.
In summary, research initiatives play a pivotal role in bridging the gap between technological advancement and ethical responsibility. Through collaborative projects and diversified funding strategies, the Institute fosters a rich ecosystem aimed at addressing the complex ethical dimensions of AI and machine learning.
Engagement with Stakeholders
In the realm of ethical AI and machine learning, the significance of engagement with stakeholders cannot be overstated. Stakeholders include not just corporations and industries, but also a variety of groups such as governmental bodies, academic institutions, and civil society organizations. Engaging these diverse entities allows for a multifaceted approach when addressing ethical concerns and emerging challenges in AI development.
Importance of Engagement
The need for dialogue and collaboration among different stakeholders is paramount. By bringing various voices into the conversation, the Institute can better understand the myriad of perspectives on ethical considerations, which can lead to richer and more effective ethical frameworks and guidelines. When stakeholders share their specific concerns and insights, it enhances transparency and helps cultivate a sense of trust among the public.
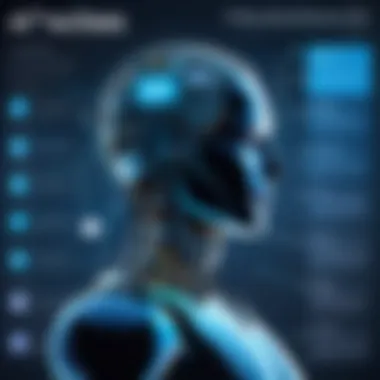
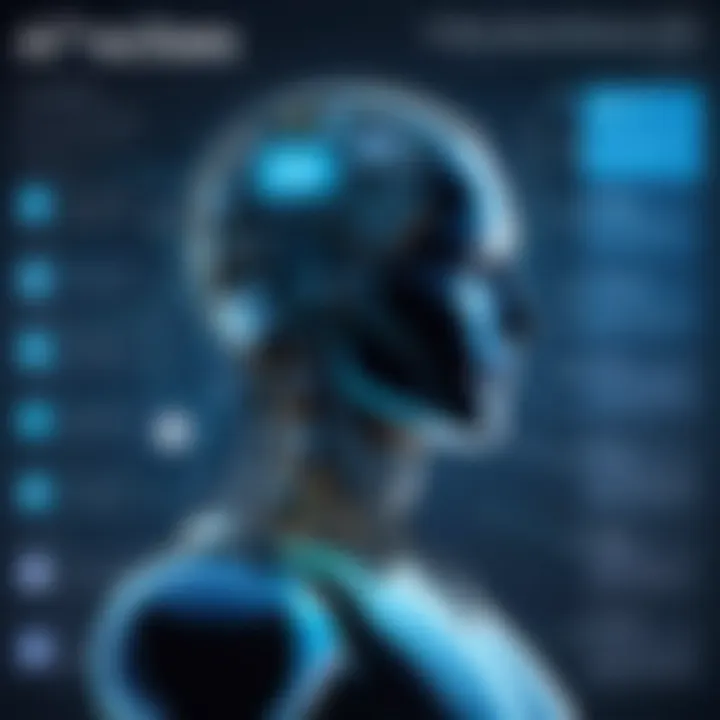
A few specific considerations about the value of stakeholder engagement include:
- Broader Perspectives: Industry leaders might focus on technological advances, while academics may highlight theoretical risks. Such hybrid thinking often leads to innovative solutions.
- Shared Responsibility: Engaging stakeholders ensures that the burden of ethical considerations is distributed, reducing the likelihood that any single group is unfairly targeted for responsibility.
- Informed Decision-Making: Data-driven insights from various sectors can guide better governance decisions.
"When we engage with a wide array of stakeholders, we're not just scratching the surface; we're digging deep into the bedrock of ethical AI. That's where the true insights lie."
Industry Collaborations
A vital area of engagement involves collaborations with industries that are developing AI technologies. These partnerships can lead to impactful projects that address real-world challenges. For instance, the automotive industryâwhich relies heavily on AI for self-driving technologiesâmust work collaboratively with safety regulators and ethics boards to ensure that societal concerns are met head-on.
Moreover, industry collaboration can take various forms:
- Joint Research Initiatives: Enterprises teaming up to research ethical AI practices, which might result in cutting-edge solutions for common problems.
- Workshops and Conferences: Setting up platforms where stakeholders can share insights, guidelines, and strategies.
- Feedback Mechanisms: Creating channels for companies to present their solutions and receive feedback from experts and the community.
Academic Partnerships
Building ties with academic institutions is equally crucial. Universities do not only serve as breeding grounds for theories and studies but also as incubators for innovative ideas. Academic partnerships can bolster ethical AI practices in several ways:
- Research Collaboration: Joint studies can explore the implications of AI technologies, contributing to a stronger base for ethical guidelines.
- Curriculum Development: Collaborating to create educational programs focused on ethical AI principles can prepare the next generation of tech professionals.
- Engaging in Ethics Discourse: Involvement of ethicists in research can help to contextualize AI developments within broader societal values.
Ethical Decision-Making Models
The evolution of artificial intelligence mandates a deep understanding of ethical decision-making models. As AI technology permeates various sectors, ethical frameworks become essential, ensuring that outcomes align with both social values and legal norms. Making these ethical choices isn't just an academic exercise; it has real-world implications that can affect people's lives. Organizations that grasp this aspect gain not only a competitive edge but also establish a positive reputational standing. Therefore, we delve into two core ethical decision-making models: utilitarian frameworks and deontological approaches.
Utilitarian Frameworks
Utilitarianism stresses maximizing overall happiness or utility. This approach can be immensely practical in the realm of AI, as it provides clear metrics for evaluating the impacts of AI technologies. For instance, when designing an AI algorithm for healthcare, developers might consider how best to allocate resources to achieve the greatest well-being for the largest number of patients. This might mean prioritizing algorithms that enhance productivity in hospitals, optimizing treatment plans, or even predicting patient outcomes.
Some key elements of utilitarian frameworks include:
- Cost-Benefit Analysis: A structured assessment of both the positive and negative consequences associated with AI applications.
- Flexibility: These frameworks can adapt to varying scenarios by allowing decision-makers to weigh the benefits against the harms.
Utilitarian frameworks enable AI practitioners to create systems that generate overall societal benefits, thus ensuring that no one individual or group is disproportionately advantaged in the decision-making process.
However, a critical consideration with utilitarianism is the potential to overlook minority rights. For instance, if an AI model sacrifices the needs of a small group to benefit the larger population, ethical dilemmas arise. Striking this balance is crucial to realizing the full potential of ethical frameworks in AI.
Deontological Approaches
Deontological ethics offers a different lens for examining AI decisions. Rather than focusing solely on outcomes, this approach emphasizes duties and rules. It insists on adherence to moral principles, regardless of the consequences. Within AI, this can manifest through policies that restrict certain functionalities, such as ensuring privacy and security of user data.
Important aspects of deontological approaches in AI include:
- Adherence to Rights: Upholding individual rights, such as consent and privacy, even when it could lead to lesser overall utility.
- Moral Obligations: Emphasizing the ethical duties that organizations uphold, leading to frameworks and standards that guide AI development.
For example, consider an AI driving system. A deontological perspective might impose strict safety measures, even if they slightly reduce efficiency, because itâs inherently wrong to endanger human lives.
These ethical decision-making models highlight that developing AI isn't merely a technical endeavorâit requires a thoughtful analysis of ethical implications. This multifaceted discourse enhances our understanding of ethical AI, ultimately paving the way for innovative yet responsible machine learning solutions.
Impact of Bias in Machine Learning
Bias in machine learning has become a significant concern, impacting everything from algorithmic fairness to societal perceptions of technology. A key aspect of ethical AI entails addressing these biases head-on; otherwise, we risk allowing injustices to seep into systems that influence critical areas such as hiring, law enforcement, and healthcare.
Consider the real-world ramifications: algorithms that are skewed can perpetuate stereotypes, leading to systemic discrimination. For instance, recruitment tools trained on biased datasets may favor candidates from certain demographics while overlooking others entirely. This can result in a workforce that is fundamentally unrepresentative, tarnishing the narrative of meritocracy
This underlines why understanding the roots of bias is critical. If creators and stakeholders in AI arenât aware of inherent biases, they can't correct themâand thus, the ethical implications follow suit. The stakes are high when AI decisions increasingly affect our lives, making it essential to recognize the various facets of bias.
"Bias in AI isn't just a technical issue; it's a societal one, and solving it requires a collective effort."
Origins of Bias
Bias in machine learning often stems from several origins. Generally, these can be categorized into three primary sources: dataset bias, algorithm bias, and user bias.
- Dataset Bias: This arises when the data used to train the models is itself biased or skewed. For example, if an AI model is trained predominantly on images of light-skinned individuals, it might struggle to accurately recognize darker skin tones. Essentially, the model reflects the world based on its training data, leading to disparities.
- Algorithm Bias: Sometimes, the algorithms can introduce bias, as certain algorithms may favor patterns that exist in the flawed datasets over others. A decision tree that splits too quickly might ignore minority classes in the data, leading to inaccurate predictions for those groups.
- User Bias: This is often overlooked. When human judgment is involved in the development or deployment of AI systems, decisions can reflect the biases of the individual or team. If those developing AI come from homogenous backgrounds, they may inadvertently create lenses that exclude diversity in perspectives.
Strategies for Mitigation
Mitigating bias in machine learning is not just a needâit's an ethical imperative. Several strategies can be employed to address this challenge effectively:
- Diverse Data Collection: Fostering diversity in data collection ensures that a representation of various demographics is included. This may require redefining data acquisition methods and ensuring that diverse groups are represented, scrutinizing datasets for biases as they are gathered.
- Regular Auditing: Conducting audits of AI and machine learning models can shed light on potential biases. Continuously monitoring output can help identify adverse impacts before they cause significant harm. Itâs vital to use performance metrics to evaluate fairness across different demographic groups.
- Algorithmic Transparency: Ensuring that stakeholders understand the algorithms and the bases for AI decisions can illuminate where biases may stem from. Employing explainable AI techniques allows for greater scrutiny of how decisions are made, making the black-box nature of many models more transparent.
- Inclusive Team Composition: Broadening the talent pool by bringing in people from various backgrounds can significantly help in identifying biases and reflecting diverse perspectives. A more inclusive team could effectively recognize biases and make thoughtful adjustments during both the model's development phase and deployment.
In summary, understanding and addressing bias in machine learning is crucial for ethical implementations and outcomes. By actively combating these issues through awareness and proactive measures, the AI community can ensure that technological advancements bolster equality rather than reinforce existing inequities. The road ahead is not easy, but the journey towards a more equitable AI landscape is certainly worth the effort.
Transparency and Accountability
Transparency and accountability are two cornerstones that underpin the ethical implementation of artificial intelligence and machine learning technologies. These principles not only enhance public trust but also promote responsible innovation. As the capabilities of AI grow, so do the complexities surrounding its use and the ethical dilemmas that surface.
Without clear transparency, AI systems can appear as black boxes, where the decision-making processes are obscure. This lack of visibility can lead to skepticism and could even amplify fears regarding discrimination or unforeseen biases in AI outcomes. Hence, establishing transparent processes is essential for fostering a culture of trust and allowing stakeholders to understand how decisions are made.
Conversely, accountability means that there are frameworks in place to ensure that individuals and organizations are held responsible for their AI-related actions. It creates a system where the consequences of AI decisions can be traced back to their origins, ensuring that the right entities can be scrutinized or corrected when necessary. This accountability not only safeguards against misuse but also encourages ethical considerations in system design and deployment.
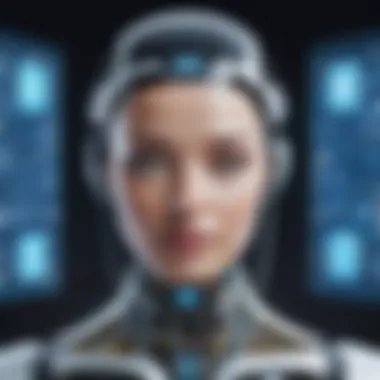
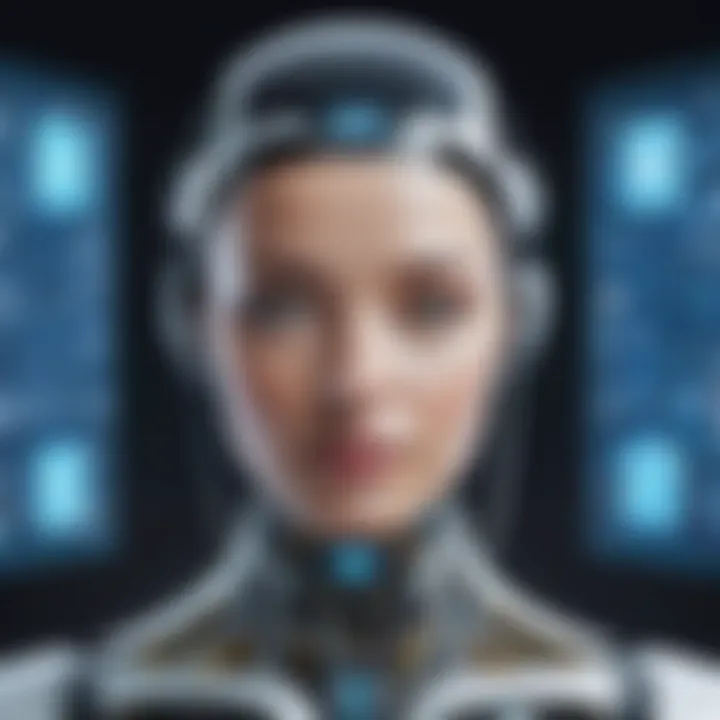
In focusing on these important elements, organizations and regulatory bodies can better navigate the complexities of AI ethics, creating systems that are not only innovative but also responsible and aligned with societal values.
Building Trust through Transparency
Transparency plays a pivotal role in building trust among users, stakeholders, and the broader public. When AI processes and their outcomes are transparent, it empowers individuals to understand and question the systems that influence their lives. For instance:
- Open Communication: Organizations should communicate clearly about how their AI systems function, outlining the algorithms and data sources employed.
- User Involvement: Engaging users in the design phase can cultivate a sense of ownership and trust, as they see their feedback incorporated into the system.
- Regular Reports: Institutions can publish regular reports assessing the performance and fairness of AI systems, making it easier for stakeholders to track progress or flag issues.
Through transparency, AI can shift from being an opaque entity to a collaborative tool that users feel comfortable working with.
Mechanisms for Accountability
Accountability mechanisms are procedures and structures that ensure adherence to ethical standards in AI applications. They serve as a safety net for users and can mitigate risks associated with deploying these technologies. Some important mechanisms include:
- Audit Trails: Keeping detailed records of the decision-making data ensures that organizations can retrace their steps if something goes awry. This includes logging who made decisions and why, which can illuminate areas for improvement.
- Impact Assessments: Conducting thorough evaluations before rolling out AI systems allows organizations to anticipate potential negative effects and adjust practices accordingly.
- Regulatory Compliance: Adherence to national and international regulations can provide guidelines for accountability measures, creating a structured approach to ethical AI implementation.
As AI technologies evolve, so too must the mechanisms we employ to ensure they are used responsibly. Both transparency and accountability must be ingrained in the culture of organizations as they embrace AI, guiding them toward responsible innovation and fostering positive societal impacts.
"Transparency and accountability in AI systems are not just regulatory checkboxes; they are essential practices for fostering trust and ensuring ethical standards are maintained."
Case Studies of Ethical AI Practices
In examining the landscape of artificial intelligence, it's imperative to draw lessons from case studies that highlight ethical implementations of AI. These practical examples provide a reservoir of knowledge regarding how ethical considerations can be woven into the fabric of AI and machine learning projects. The main idea here isn't just to showcase success stories but to also shine a light on the complex realities that arise in these applications.
Successful Implementations
Successful implementations serve as beacons in the often murky waters of artificial intelligence. Take for instance, the efforts made by Google with their AI Principles. This initiative emphasizes fairness, privacy, and accountability in AI development. By setting these standards, Google laid a framework that other companies can aspire to.
Another noteworthy example comes from IBM, which launched its Watson for Oncology. The system utilizes AI to provide treatment recommendations for cancer patients, drawing from vast databases linking medical literature, patient records, and clinical guidelines. It shows how AI can augment human expertise while prioritizing patient safety and bioethical considerations.
These implementations signify not only technological advancement but also a commitment to ethical practices, underscoring the principle that AI should align with societal values.
Lessons Learned
Analyzing these case studies reveals critical lessons that stakeholders in the field of AI should internalize. Firstly, collaboration is key. The partnership between IBM and global healthcare institutions to refine Watson illustrates that input from diverse stakeholders enhances the system's reliability and ethical ground. Without feedback from those on the frontlines of care, AI development risks becoming out of touch with real-world needs.
Additionally, the importance of continuous learning is evident. With AI evolving, itâs essential for organizations to remain agile and responsive. Companies should regularly evaluate and update their ethical frameworks in response to new findings and societal shifts. A relentless focus on transparency emerges as a salient theme; when organizations are open about their processes and algorithms, they cultivate greater trust.
"Building a transparent AI model helps to not only regain public trust but ensures more responsible decisions in the long run."
Lastly, the value of accountability cannot be overstated. Systems like Googleâs should be examined through audits to uphold ethical standards and ensure compliance. Failure to address these factors can lead to severe reputational damage and loss of public confidence in AI technologies. Striking a balance between innovation and ethical responsibility is an ongoing challenge that these case studies bring to the forefront.
Through the lens of these real-world applications, it becomes clear that ethical AI practices are not merely aspirational goals but necessary components for sustainable advancement in technology.
Future Directions of Ethical AI
The future directions of ethical AI are vital not only for the technology itself but also for the society it serves. As artificial intelligence continues to evolve, it raises significant questions about its implications on privacy, autonomy, and accountability. Envisioning the future means anticipating the consequences of these advancements will help steer AI toward a more beneficial path.
In recent years, organizations have realized the need for ethical frameworks that guide AI development. The significance of this topic cannot be overstatedâit's about striking a balance between innovation and moral responsibility. This balance is essential, as the AI we create will not only address todayâs problems but also shape the societal landscape for generations to come. The considerations in this area fall into distinct categories:
- Proactive Regulation: Developing policies that not only respond to current challenges but also preemptively address potential future issues.
- Public Awareness: Increasing transparency and educating society about how AI technologies work and their impacts.
- Collaboration across Disciplines: Engaging experts from various fields, including law, sociology, and beyond, to ensure a multi-faceted view of ethical challenges.
Below is an outline of two critical areas that will define the future of ethical AI:
Innovative Research Areas
Research into ethical AI is becoming a hotbed of exploration. The focus lies on developing systems that are not only technically proficient but also socially responsible. A few innovative research areas include:
- Explainable AI: This focuses on creating algorithms that can transparently explain their decision-making processes. An AI that can justify its actions is better positioned to gain public trust.
- Fairness in Algorithms: Pursuing mathematical approaches to reduce bias in AI systems. Researchers aim to create guidelines that ensure equal treatment across diverse demographics.
- AI in Environmental Sustainability: Exploring how AI can help tackle climate change or optimize resource use in agriculture and manufacturing. This area holds transformative potential when combined with ethical guidelines.
These research areas not only offer practical solutions but also influence the way we perceive AIâs role in society. Theyâre crucial for fostering a more equitable and responsible tech environment.
Emerging Ethical Challenges
With evolving technologies, new ethical considerations inevitably arise. Understanding these challenges is pivotal if we want to navigate the future responsibly. Some prominent concerns include:
- Data Privacy: As AI systems become more integrated, the risk of infringing upon personal data privacy intensifies. What mechanisms can be devised to mitigate this risk while still benefiting from data?
- Autonomy and Control: As machines acquire decision-making capabilities, questions about human autonomy emerge. How do we ensure that humans remain in the driverâs seat?
- Socioeconomic Disparities: AI has the potential to widen the gap between those with access to technology and those without. Addressing this imbalance is essential for a fair progression in society.
"Itâs crucial that ethical considerations in AI evolve alongside the technology itself; without this synergy, we face dire consequences."
The way forward requires a deliberate and inclusive dialogueâone engaging technologists, ethicists, and the public. This is the path towards building a future where AI genuinely serves humanity, respecting ethical boundaries while fostering innovation.
The End
Recap of Findings
The central findings throughout the article reveal a landscape rich in both promise and peril. Here are some significant points that emerge:
- Framework Establishment: The institute has set forth comprehensive guidelines aimed at ensuring that AI development aligns with ethical norms, stressing the importance of transparency and accountability.
- Stakeholder Engagement: It has catalyzed collaborations across industries and academia, fostering a dialogue that includes a diverse array of voices in AI governance.
- Bias Mitigation: An important emphasis was placed on recognizing and addressing bias in machine learning algorithms, which prior research indicated as a pivotal issue in ethical AI frameworks.
- Emerging Challenges: Future directions point to unique ethical challenges introducing complexity into AI applications, necessitating continuous adaptation and learning from past experiences.
These findings serve as a springboard for future investigations and discussions on AI ethics, stressing the shared responsibilities amongst all stakeholders to uphold these values.
Call for Continued Dialogue
While the Institute has laid a robust foundation, the journey doesnât end here. Continued dialogue remains vital in addressing the fast-evolving landscape of AI and machine learning. Stakeholders â from policymakers to tech developers â must acknowledge the collective responsibility towards ensuring that AI technologies serve the broader society positively. To maintain momentum, consider the following avenues for ongoing conversations:
- Public Engagement Initiatives: Creating platforms where public opinions can be shared on ethics in AI opens channels for feedback and enhances transparency.
- Workshops and Seminars: Continued educational efforts can help various stakeholders understand the implications of ethical AI in practical terms, bridging gaps in knowledge and understanding.
- Regular Policy Evaluations: Periodic reviews of existing policies related to AI and machine learning should be conducted to ensure they remain current with technological advancements and societal needs.
- Research Collaborations: Encouraging interdisciplinary research partnerships fosters innovation and ensures diverse perspectives are included in decision-making processes.