Unveiling the Latest Machine Learning Trends in Technology
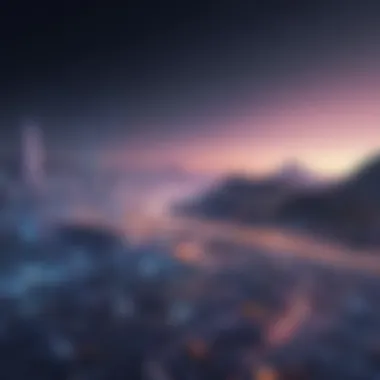
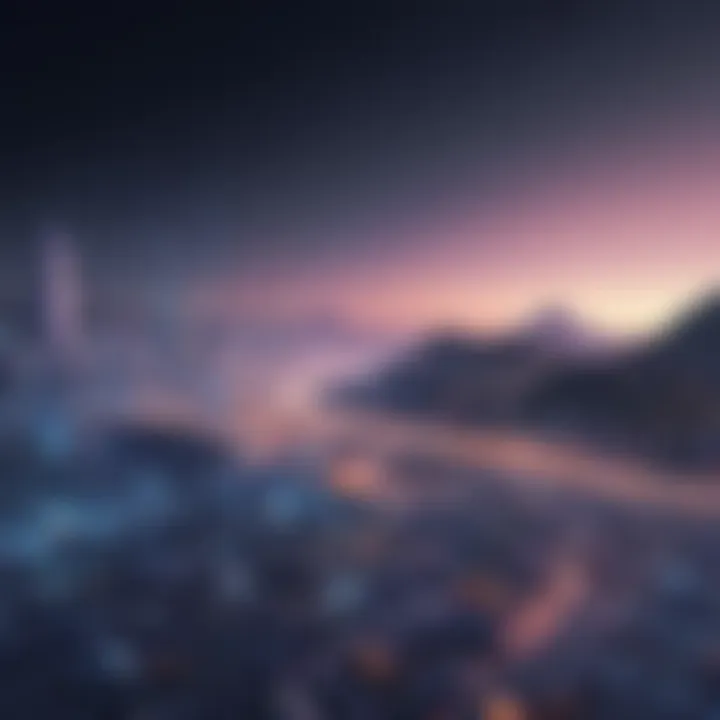
Understanding Machine Learning Trends
Machine learning, a fundamental aspect of artificial intelligence (AI), is witnessing a rapid evolution, impacting various sectors substantially. It plays a pivotal role in transforming how data-driven insights are harnessed by businesses and individuals alike. This section aims to explore the nuanced trends within machine learning and their profound implications across the technological landscape.
Integration of AI in Diverse Industries
One of the paramount trends in machine learning entails the widespread integration of AI applications across diverse industries. From healthcare to finance, AI-driven solutions are revolutionizing operational efficiencies and decision-making processes. The seamless incorporation of machine learning algorithms into industry-specific workflows is propelling innovation and enabling organizations to achieve unprecedented levels of productivity.
Emergence of Explainable AI
Another notable trend is the rise of explainable AI, shedding light on the black box phenomenon prevalent in traditional AI systems. With stakeholders increasingly emphasizing the importance of transparent and interpretable AI models, explainable AI is gaining prominence. By enhancing the interpretability of machine learning algorithms, explainable AI fosters trust among users and facilitates the ethical deployment of AI technologies.
Industry Trends and Updates
In the realm of machine learning, staying abreast of industry trends and updates is paramount to capitalize on emerging opportunities and address evolving challenges. This section will delve into the latest developments in machine learning technologies, cybersecurity threats and solutions, as well as networking innovations and advancements. Understanding these trends is crucial for leveraging the full potential of machine learning in a rapidly evolving technological landscape.
Introduction: Unfolding the Significance of Machine Learning Trends
Machine learning stands at the forefront of technological innovation, heralding a new era of data-driven insights and automation. The realm of machine learning encompasses a spectrum of algorithms, applications, and trends that intersect to redefine how businesses and individuals harness the power of information. In the context of this article, delving into the landscape of machine learning trends holds paramount importance for understanding the evolving dynamics of technology.
Exploring the nuances of machine learning trends serves as a compass for navigating the complex terrain of artificial intelligence and data science. This journey elucidates pivotal transformations underway in industries, ranging from healthcare to finance, revamping traditional processes and unlocking unprecedented efficiency. By dissecting the currents of machine learning trends, we equip ourselves with the foresight to capitalize on emerging opportunities and mitigate potential challenges.
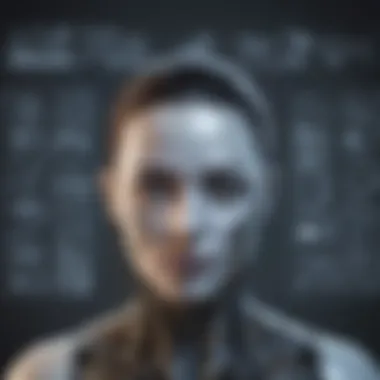
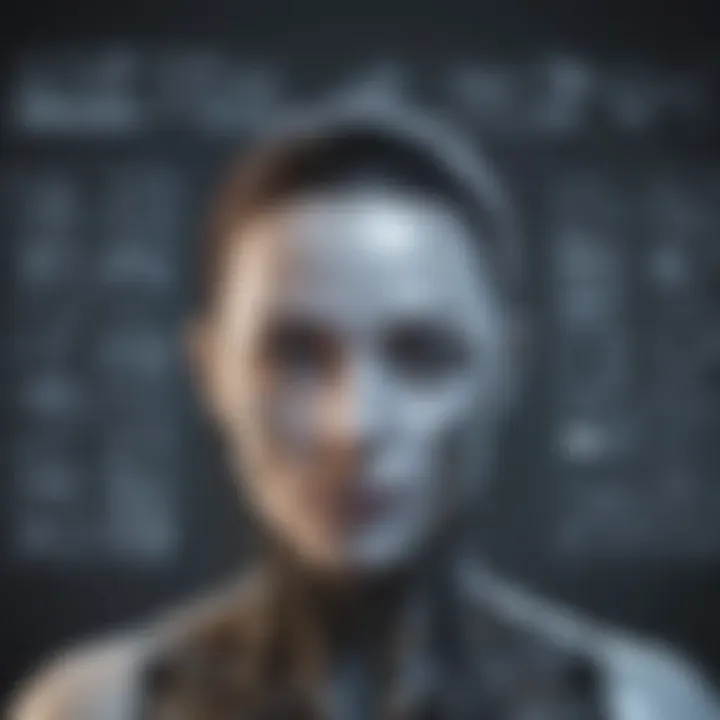
This magnifying glass on machine learning trends not only unravels the current state of affairs but also forecasts the trajectory of technological advancements. By elucidating the intricacies of AI integration in various sectors and the burgeoning prominence of explainable AI, this exploration paves the way for a deeper comprehension of how algorithms shape our daily interactions with technology. Through this article, we aim to elucidate the kaleidoscope of machine learning trends, offering a panoramic view of the fusion between human intelligence and computational prowess in the digital age.
Understanding Machine Learning
In the realm of technological advancements, Understanding Machine Learning holds a paramount position. It is the bedrock upon which intricate algorithms and AI applications are built. Understanding Machine Learning enables professionals to decipher complex patterns within data, fostering accurate predictions and informed decision-making processes. Delving into the intricacies of machine learning empowers IT experts, cybersecurity specialists, and students alike to navigate the evolving landscape of AI technologies with confidence and finesse.
Definition of Machine Learning
Machine Learning serves as the cornerstone of modern AI systems, allowing machines to learn from data patterns and improve over time without explicit programming. This dynamic discipline encompasses algorithms and statistical models that enable machines to perform tasks without explicit instructions. From predictive analytics to image recognition, Machine Learning permeates various facets of our digital ecosystem, promising unparalleled efficiency and accuracy in data-driven processes.
Types of Machine Learning Algorithms
- Supervised Learning: Supervised Learning represents a pivotal aspect of Machine Learning, where models are trained on labeled data to make predictions and decisions. Its ability to learn from historical data and generalize to new instances makes it a valuable tool for various applications in finance, healthcare, and beyond. While supervised learning excels in tasks with clear objectives and well-defined outcomes, it may struggle with unstructured data and complex patterns.
- Unsupervised Learning: Unlike supervised learning, Unsupervised Learning deals with unlabeled data, aiming to uncover inherent patterns and structures within datasets. By exploring data without predefined outputs, unsupervised learning reveals hidden insights and relationships that might evade human perception. While incredibly versatile in clustering and anomaly detection, unsupervised learning often requires extensive preprocessing and interpretation of results.
- Semi-supervised Learning: Situated between supervised and unsupervised learning, Semi-supervised Learning combines labeled and unlabeled data to inform model training. This approach proves beneficial in scenarios with limited labeled data, enabling models to leverage both labeled and unlabeled samples for enhanced performance. By striking a balance between supervised precision and unsupervised scalability, semi-supervised learning showcases adaptability and resource-efficiency in complex learning tasks.
- Reinforcement Learning: Emulating the trial-and-error learning process observed in humans, Reinforcement Learning focuses on sequential decision-making through interactions with an environment. Agents learn through rewards and penalties, refining their strategies to maximize cumulative rewards over time. With applications ranging from game playing to robotics, reinforcement learning addresses dynamic environments where outcomes depend on a sequence of actions and responses.
Current Trends in Machine Learning
In the realm of technological advancements, current trends in machine learning play a pivotal role. These trends are not mere transient fads but are shaping the very fabric of how industries operate and individuals interact with data-driven insights. Understanding these trends is essential for staying abreast of the rapidly evolving landscape of technology.
Integration of AI in Industries
The integration of Artificial Intelligence (AI) in industries marks a significant shift in traditional operational paradigms. AI is no longer confined to the realm of science fiction; it is now a tangible and transformative force within various sectors. Industries across the spectrum, from healthcare to finance and beyond, are harnessing the power of AI to streamline processes, boost efficiency, and drive innovation.
As AI continues to evolve, its integration within industries heralds a new era of productivity and growth. The ability of AI algorithms to sift through vast datasets, identify patterns, and generate actionable insights is revolutionizing decision-making processes within organizations. From predictive analytics in finance to personalized healthcare solutions, the impact of AI integration is multifaceted and profound.
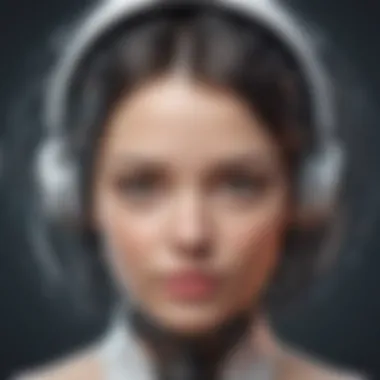
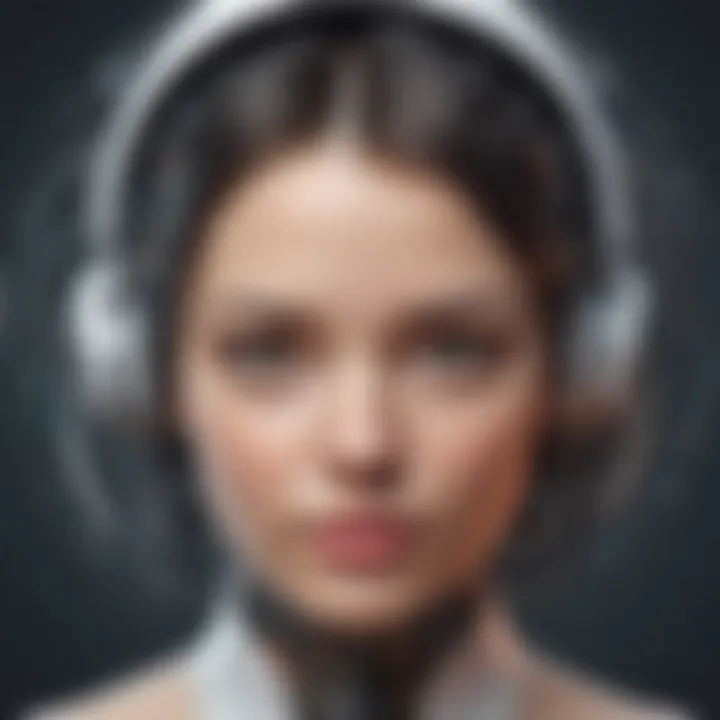
Explainable AI
The emergence of explainable AI addresses a critical limitation of traditional black-box algorithms. While conventional machine learning models could provide accurate predictions, the rationale behind these outputs often remained opaque. Explainable AI seeks to bridge this gap by offering transparency and interpretability in decision-making processes.
In domains where accountability and trust are paramount, such as healthcare and finance, explainable AI provides stakeholders with insights into how decisions are reached. By providing interpretable explanations for its outputs, explainable AI enhances user confidence, facilitates regulatory compliance, and enables meaningful human-AI collaboration.
Personalized Machine Learning Models
Personalized machine learning models epitomize the intersection of data-driven insights and individual preferences. These models leverage consumer data to tailor recommendations, predict user behavior, and deliver customized experiences. In the age of information overload, personalized ML models offer a pathway to targeted and relevant content that resonates with users.
By analyzing user interactions and historical data, personalized ML models can anticipate user needs, anticipate trends, and recommend personalized solutions. Whether in e-commerce, content curation, or digital marketing, the adoption of personalized ML models is transforming how businesses engage with their audience, driving customer satisfaction and loyalty.
AI Ethics and Bias Mitigation
As machine learning algorithms become ubiquitous in decision-making processes, the importance of AI ethics and bias mitigation cannot be overstated. Biases inherent in training data, algorithmic decisions, or implementation strategies can perpetuate societal inequities and amplify discrimination.
Ensuring ethical AI development involves identifying and addressing biases at every stage of the machine learning lifecycle. From dataset collection and preprocessing to model training and deployment, proactive measures must be taken to mitigate biases, promote fairness, and uphold ethical standards. By prioritizing AI ethics and bias mitigation, organizations can cultivate trust, foster inclusivity, and ensure that AI technologies serve the collective good.
Future Prospects of Machine Learning
In the realm of technological evolution, the anticipation of what the future holds for machine learning looms large. The section "Future Prospects of Machine Learning" within this insightful article bears significant weight as it navigates through the unfolding landscape of possibilities and innovations. Understanding the crucial role that future prospects play in driving advancements in machine learning is paramount for IT professionals, cybersecurity experts, and students eager to grasp the trajectory of this dynamic field.
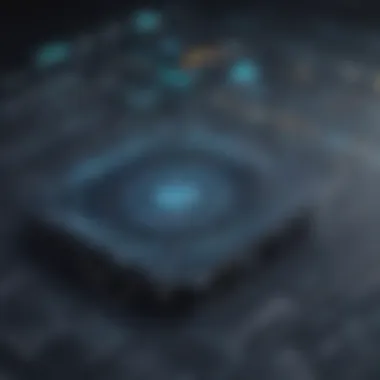
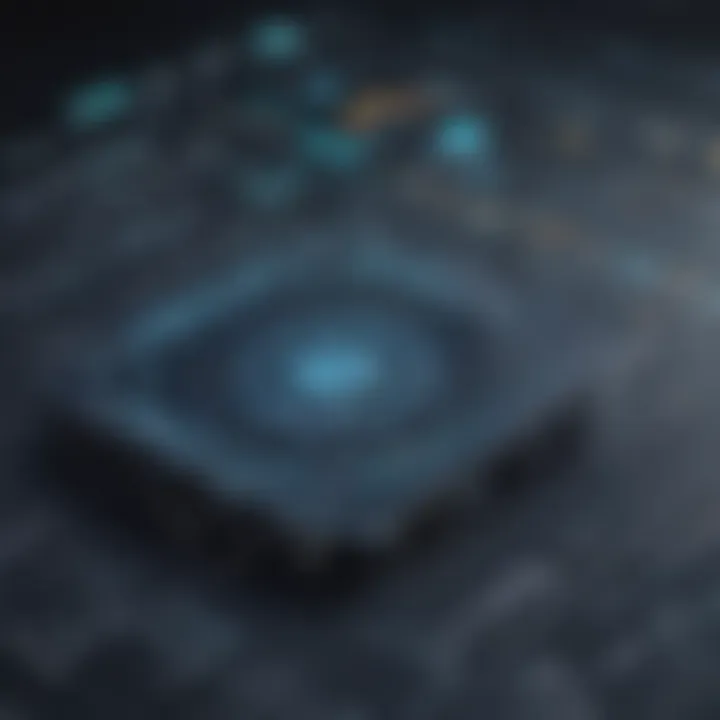
Delving into the core of this topic unveils a myriad of tangible benefits and insights awaiting in the future of machine learning. The incessant advancements in deep learning, automated machine learning (AutoML), quantum machine learning, and the exponential growth of data science collectively constitute the underlying fabric of the forthcoming strides in this domain. These elements serve as pivotal pillars, propelling the field towards broader vistas of functionality, efficiency, and scalability.
Considering the trends within machine learning, the contemplation of future prospects bears a dual emphasis on innovation and adaptability. While innovation fuels the exploration of uncharted territories in AI technologies, adaptability becomes the key to harnessing these innovations effectively within diverse operational contexts. Striking a delicate balance between pushing the boundaries of technological capabilities and ensuring practical implementation resonates deeply within the discussions surrounding future prospects.
Faced with the rapid evolution of technology, stakeholders must remain vigilant towards emerging trends in machine learning to harness the potential benefits while mitigating associated risks effectively. The spotlight on future prospects brings into sharp focus the transformative impact these advancements can have on businesses, societies, and individuals, underscoring the critical importance of staying abreast of the evolving landscape within the realm of machine learning.
Advancements in Deep Learning
Within the expansive domain of machine learning, the realm of deep learning stands as a beacon of cutting-edge advancements and possibilities. The subsection
Impact of Machine Learning Trends
In the vast landscape of technology, the impact of machine learning trends is profound. This article delves into the evolving trends that shape the realm of data-driven insights, bringing to light the transformation these trends instigate in various industries. From revolutionizing decision-making processes to enhancing customer personalization, the implications are far-reaching and vital. Amidst the integration of AI and the emergence of explainable AI, understanding the impact of these trends becomes imperative for businesses and individuals seeking to stay abreast in this dynamic field.
Enhanced Decision-Making Processes
Enhanced decision-making processes are at the core of the machine learning trends pervading the technological sphere today. By harnessing the power of advanced algorithms and predictive analytics, businesses can decipher complex data sets to make informed decisions swiftly and accurately. Such advancements enable organizations to streamline operations, identify profitable opportunities, and mitigate risks effectively. Moreover, the automated insights provided by machine learning algorithms significantly boost efficiency and precision in decision-making processes, setting a new standard for strategic planning and execution.
Improved Customer Personalization
In the era of heightened personalization, machine learning trends play a pivotal role in tailoring customer experiences to individual preferences and behaviors. By leveraging data analytics and predictive modeling, businesses can create hyper-targeted marketing campaigns, recommend personalized products and services, and enhance overall customer satisfaction. Through the seamless integration of machine learning algorithms, companies can foster stronger customer relationships, foster brand loyalty, and drive revenue growth. Thus, the emphasis on improved customer personalization underscores the transformative potential that machine learning trends bring to the forefront.
Addressing Cybersecurity Challenges
With the proliferation of data breaches and cyber threats, addressing cybersecurity challenges is a crucial aspect of contemporary machine learning trends. By utilizing advanced anomaly detection algorithms and proactive threat intelligence, organizations can fortify their defense mechanisms and safeguard sensitive information effectively. Machine learning algorithms can detect irregular patterns, identify potential vulnerabilities, and respond to security incidents in real-time, thereby bolstering overall cybersecurity posture. The integration of machine learning in cybersecurity efforts underscores a proactive approach to risk management and data protection in an interconnected digital landscape.
Efficient Resource Management
Efficient resource management stands as a cornerstone of machine learning trends, enabling organizations to optimize resource allocation, streamline operations, and improve overall productivity. By harnessing predictive analytics and optimization algorithms, businesses can forecast demand, allocate resources efficiently, and enhance cost-effectiveness. Machine learning trends empower organizations to automate repetitive tasks, reduce manual errors, and maximize resource utilization, leading to enhanced operational efficiency and sustainable growth. The strategic deployment of machine learning in resource management exemplifies a data-driven approach to decision-making, promoting agility and resilience in the face of evolving business landscapes.