Exploring Accenture's Data Analytics for Transformation
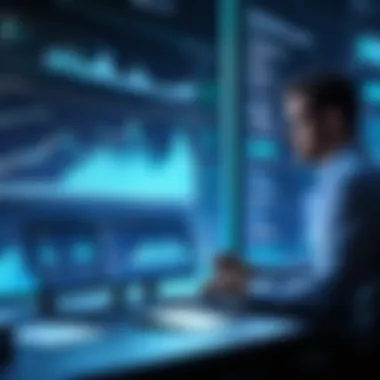
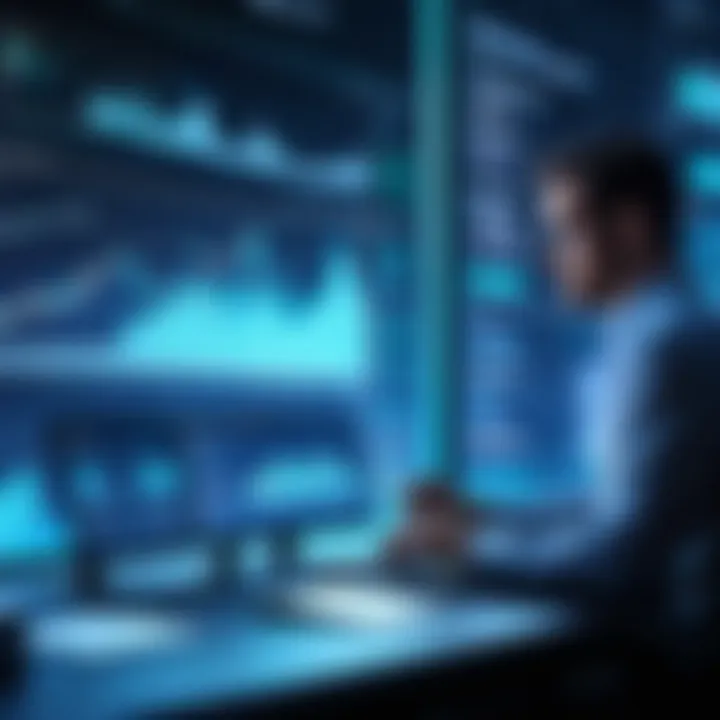
Intro
In today’s landscape, businesses must harness the power of data analytics to remain competitive. Accenture has established itself as a leader in this field, employing various innovative methodologies and technologies to transform businesses. This article aims to explore the capabilities of Accenture's data analytics, giving insight into its substantial role in business transformation.
Understanding how Accenture integrates data analytics into its operations is critical. By focusing on case studies and applications across various industries, we can appreciate the depth of insight that analytics provides. Moreover, we will discuss the relevance of data analytics to modern business challenges, illustrating how it aids in fostering innovation and making strategic decisions.
Throughout this article, professionals will gain valuable knowledge about how to leverage data analytics effectively, driving business efficiencies and achieving better outcomes. By dissecting relevant terminologies and concepts, as well as recent trends and practices, this can serve as a comprehensive guide for IT professionals and business leaders alike.
Understanding Accenture Data Analytics
Accenture Data Analytics represents a pivotal aspect of modern business transformation. In an era where data is generated at an unprecedented pace, organizations are increasingly recognizing the necessity of leveraging this data for strategic advantage. Understanding Accenture’s approach to data analytics is essential for IT professionals, cybersecurity experts, and students alike. It provides insights into how businesses can optimize operations, enhance decision-making processes, and ultimately drive growth.
Defining Data Analytics
Data analytics refers to the systematic computational analysis of data. It is a process that involves collecting and inspecting raw data to uncover patterns, correlations, and trends that can inform decision-making. Data analytics is not just about number crunching; it encompasses a variety of techniques including statistical analysis, predictive modeling, and machine learning.
Accenture's commitment to data analytics hinges on its belief that data-driven insights can drastically improve operational efficiency and business agility. Each organization has unique data assets, and understanding how to harness these properly is crucial.
Accenture's Role in Data Analytics
Accenture plays a significant role in the data analytics landscape by providing services that span the entire analytics lifecycle. This includes data collection, cleaning, processing, analyzing, and visualizing the data. Accenture aims to empower organizations to use their data more effectively, helping them to derive actionable insights that lead to informed decisions.
Accenture leverages industry-specific expertise, advanced technologies, and a robust analytics strategy to support clients in their data initiatives. The firm’s ability to integrate data analytics into its business transformation projects means that clients can expect not only better insights but also improved implementations. Through its dedicated teams and extensive resources, Accenture helps organizations navigate the complexities involved in managing and analyzing data.
"Data is the new oil. It’s valuable, but if unrefined it cannot really be used. Data needs to be refined to extract value."
Key Components of Accenture Data Analytics
Understanding the key components of Accenture's data analytics is essential for grasping how organizations can effectively transform their operations. These components play a vital role in creating meaningful insights that drive business performance. Data analytics involves a systematic approach to collecting and interpreting data, and Accenture has honed its processes to achieve maximum efficiency in this domain. Each component is interconnected, creating a holistic framework that organizations can leverage for strategic advantage. By examining these components, businesses can appreciate the benefits of implementing data analytics more thoroughly.
Data Collection Techniques
Data collection is the first and possibly one of the most critical steps in data analytics. Accenture employs several techniques for gathering data from diverse sources. These sources often include primary data collection methods such as surveys and interviews, as well as secondary sources like existing databases and public records. The integration of these varied data sources enhances the richness of the analysis.
Effective data collection techniques ensure that the gathered data is robust and relevant. There are three essential categories of data collection methods:
- Surveys: Used to gather specific information directly from a target audience.
- Web Scraping: Involves extracting data from websites, providing real-time insights into market trends.
- IoT Devices: These tools collect data autonomously, offering continuous streams of information from various environments.
By refining these techniques, Accenture ensures higher accuracy and relevance in data analytics. Quality data collection is fundamental for achieving insightful outcomes.
Data Processing and Analysis
Once data has been collected, the next step involves processing and analyzing it to extract useful information. Accenture utilizes various methodologies to transform raw data into actionable insights. This phase is crucial as it allows organizations to identify patterns, trends, and correlations hidden within the data.
The processing stage involves cleaning and organizing data, followed by applying statistical models and algorithms to analyze it. Some common analytical techniques used by Accenture include:
- Descriptive Analytics: Summarizes past data to understand trends and patterns.
- Diagnostic Analytics: Investigates the cause of certain trends by comparing variables.
- Predictive Analytics: Uses historical data to forecast future outcomes, enabling better decision-making.
It is at this point that the power of data analytics shines, permitting companies to make informed choices based on empirical evidence rather than intuition.
Data Visualization Tools
Visualization tools are an integral aspect of data analytics, as they help in the representation of data findings in a more digestible format. Accenture employs a variety of data visualization tools to communicate insights effectively to stakeholders. A clear visualization allows for quick comprehension and facilitates better decision-making.
Some popular visualization tools include:
- Tableau: Known for its ability to create interactive dashboards.
- Power BI: Offers real-time data analysis and visualization capabilities.
- D3.js: A JavaScript library that produces dynamic and interactive visualizations.
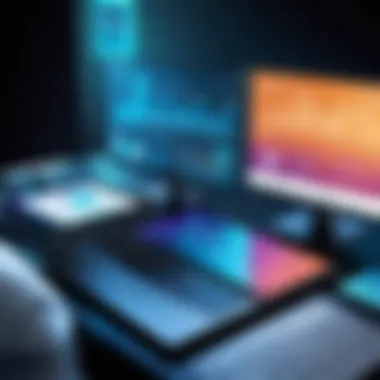
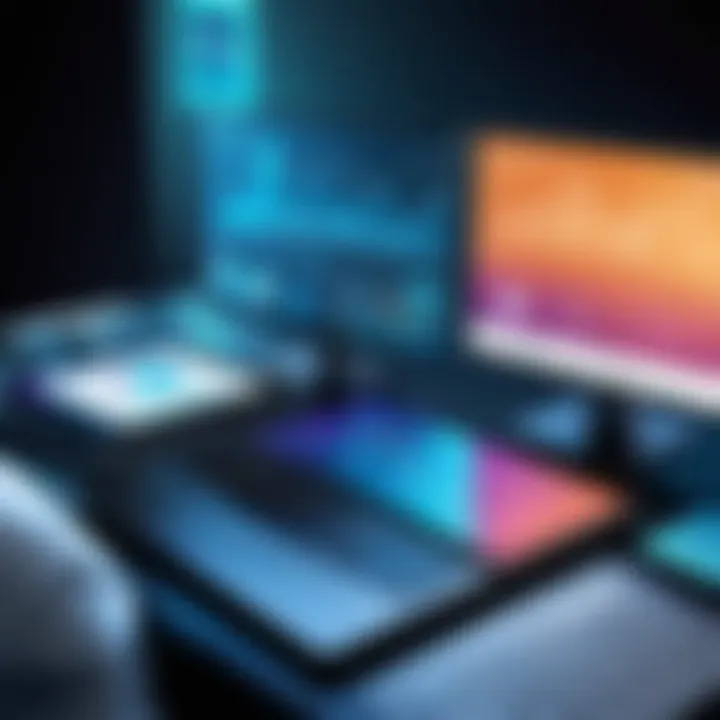
Appropriate visualization of data can highlight key insights that might otherwise go unnoticed in complex datasets. Hence, effective use of these tools is crucial in engaging stakeholders and driving action based on the analytics.
In summary, the key components of Accenture's data analytics process—data collection techniques, data processing and analysis, and data visualization tools—form the foundation for successful business transformation. Each component plays its role in transforming raw data into strategic insights.
As businesses continue to navigate an increasingly data-driven world, understanding these key elements becomes essential for fostering innovation and enhancing decision-making.
Methodologies Employed by Accenture
The methodologies employed by Accenture play a critical role in its ability to harness data analytics for effective business transformation. These structured approaches enable comprehensive insights that drive strategic decisions and foster innovative practices. By using specific methodologies, Accenture can enhance its data-driven decision-making capabilities, increase operational efficiency, and deliver tailored solutions to its clients. Understanding these methodologies is pertinent, especially for IT professionals, cybersecurity experts, and students seeking to delve into the dynamics of data analytics.
Agile Analytics Framework
The Agile Analytics Framework represents a pivotal methodology within Accenture. It underscores the importance of adaptability and responsiveness in analytics projects. This approach is characterized by iterative development, which allows teams to quickly adapt to changing business needs and evolving data landscapes.
Key elements of the Agile Analytics Framework include:
- Iterative Process: Projects are broken down into manageable increments, enhancing flexibility.
- Cross-functional Teams: Experts from different domains collaborate, ensuring a holistic view of analytics challenges.
- Feedback Loops: Regular feedback from stakeholders allows for continuous improvement and alignment with business goals.
These features collectively enhance speed and efficiency. Furthermore, organizations that implement Agile methodologies report higher satisfaction levels as they can address business needs more effectively. The embrace of Agile practices allows Accenture to provide clients with timely insights that are critical for competitive advantage in a rapidly changing landscape.
Predictive Analytics Approaches
Within the domain of methodologies, Predictive Analytics Approaches are increasingly vital. Accenture employs these techniques to leverage historical data in forecasting future trends and behaviors. This methodology is invaluable in various contexts, allowing businesses to make informed decisions based on evidence rather than assumptions.
Some considerations regarding Predictive Analytics include:
- Data-Driven Decision Making: Decisions rooted in analytics tend to reduce risks associated with uncertainty.
- Enhanced Customer Insights: Businesses receive deeper understanding of customer behaviors, assisting in personalizing services.
- Proactive Strategy: Organizations can anticipate market shifts, allowing them to pivot strategies proactively rather than reactively.
"Incorporating predictive analytics not only informs business strategies but also fosters innovation and resilience."
Utilization of Predictive Analytics can manifest in various industries, from finance to healthcare, enhancing service delivery and operational performance. The results often lead to optimizing resources and achieving a significant return on investment.
In summary, Accenture’s methodologies—specifically the Agile Analytics Framework and Predictive Analytics Approaches—are instrumental in refining the process of data analytics. By implementing these structured methodologies, businesses can achieve enhanced efficiency, responsiveness, and strategic insight, critical for navigating today’s complex business environment.
Technologies Underpinning Accenture's Analytics
Data analytics requires strong technical foundations to thrive in a competitive environment. Accenture focuses on various cutting-edge technologies that drive its data analytics capabilities. Understanding these technologies is crucial for organizations that wish to transform their operations through data analysis. This section highlights several key technologies.
Artificial Intelligence Applications
Artificial intelligence is not just a buzzword; it’s integral to how Accenture processes and analyzes data. AI enables systems to learn from data patterns and make predictions without explicit programming. The application ranges from natural language processing to image recognition. This adaptability allows businesses to understand consumer behavior and operational inefficiencies more accurately.
Benefits of AI in Data Analytics:
- Enhanced Decision Making: By analyzing data faster than humans can, AI can provide insights that lead to better decisions.
- Automation: Routine tasks can be automated, allowing teams to focus on complex challenges.
- Predictive Insights: AI helps forecast trends and outcomes, enabling proactive strategies.
Implementing AI applications requires thoughtful integration into existing systems. Organizations must ensure adequate data sets for training models. This might be a challenge in data quality and sourcing.
Machine Learning Frameworks
Machine learning is a subset of AI focused on building systems that learn from data. Accenture employs a variety of machine learning frameworks to improve business operations. These frameworks allow businesses to analyze vast data sets efficiently. Frameworks like TensorFlow or PyTorch shape how models are built and optimized.
Key Considerations for Machine Learning in Analytics:
- Model Selection: Choosing the right model based on the data and desired outcome.
- Training Data: Acquiring high-quality data for training is essential. Poor data can lead to flawed outcomes.
- Continuous Learning: As new data is collected, models must be recalibrated for accuracy.
Machine learning provides the ability to uncover insights that may not be visible through traditional analysis. Businesses can identify patterns that help fine-tune strategies and operations.
Cloud Computing Innovations
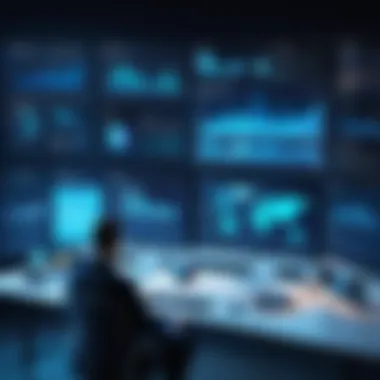
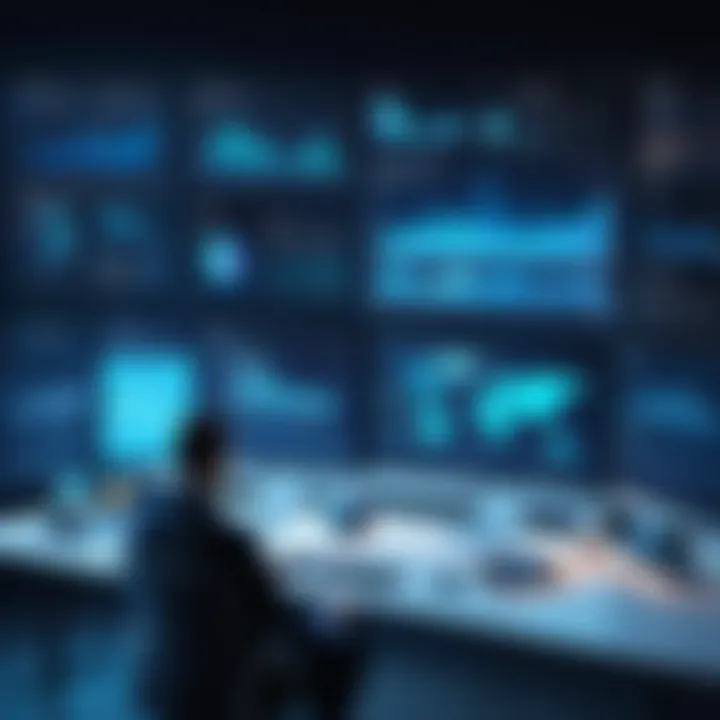
Cloud computing plays a significant role in hosting data analytics platforms and tools. Accenture capitalizes on cloud innovations to ensure its data solutions are scalable and efficient. By using platforms like Amazon Web Services, Microsoft Azure, and Google Cloud, organizations can store and process data at unprecedented speeds.
Advantages of Cloud Computing for Data Analytics:
- Scalability: Resources can be expanded as needs grow, without heavy investment in physical infrastructure.
- Accessibility: Data and analytics tools are accessible from anywhere, promoting collaboration across teams.
- Cost Efficiency: Reduces the need for on-premises hardware, leading to lower operational costs.
While cloud computing offers numerous benefits, companies must address security and compliance concerns. It is crucial to establish robust strategies to protect sensitive data while utilizing these technologies.
Accenture’s use of AI, machine learning, and cloud computing plays a transformative role in how businesses approach data analytics. The integration of these technologies not only enhances analysis but also fosters permanent innovation in business processes.
Case Studies in Diverse Industries
The examination of case studies across various industries serves as a cornerstone for understanding how Accenture applies data analytics to drive business transformation. Analyzing real-world applications provides valuable insights into practical implementations that lead to notable outcomes. Each industry presents unique challenges and opportunities, allowing for a diverse set of examples that highlight the versatility of Accenture's approaches.
Healthcare Sector Applications
In the healthcare sector, data analytics plays a critical role in improving patient outcomes and optimizing operational efficiencies. Accenture has successfully partnered with healthcare organizations to harness vast amounts of data for informed decision-making.
By implementing predictive analytics, healthcare providers can anticipate patient admissions, manage resources better, and reduce wait times. For instance, a health network utilized Accenture's data solutions to analyze electronic health records and operational data. This enabled them to identify patterns in patient flow and adjust staffing strategies accordingly. As a result, they achieved significant reductions in patient wait times and improved care delivery while minimizing costs.
Financial Services Insights
Within the financial services industry, data analytics helps firms gain a competitive edge through improved risk management and customer insights. Accenture's data-driven strategies enable financial institutions to analyze transaction patterns, detect fraudulent activities, and enhance customer satisfaction.
A notable example involved a global bank working with Accenture to refine its risk assessment processes. By employing advanced machine learning algorithms, the bank was able to better predict credit risk and set more accurate interest rates for loans. This not only improved their bottom line but also created more tailored financial products for their customers, thereby improving overall customer experience.
Retail Industry Transformations
The retail industry has experienced transformative benefits from data analytics. Accenture aids retailers in understanding consumer behavior, optimizing inventory, and personalizing marketing efforts. This sector's dynamic nature necessitates agile data strategies to remain competitive.
For instance, an online retailer partnered with Accenture to enhance its customer segmentation. By analyzing shopping patterns and customer demographics, they implemented targeted marketing campaigns that significantly boosted sales conversions. Additionally, data analytics helped the retailer manage inventory effectively, reducing overstock situations and minimizing waste.
"Data analytics is not just about collecting information, but rather about making strategic decisions that lead to tangible business outcomes."
Overall, the case studies within diverse industries underscore the practical applications and effectiveness of Accenture's data analytics solutions. Each example illustrates how tailored analytical strategies can drive significant improvements across sectors, showcasing the essential role of data analytics in modern business practices.
Impact of Data Analytics on Decision-Making
In the contemporary business environment, data analytics stands as a fundamental pillar in guiding decision-making processes. The significance of this aspect cannot be understated. Organizations, both large and small, increasingly rely on data-driven insights to shape their strategies and enhance operational efficiency. Accenture's role in this arena is pivotal, serving as a catalyst that transforms raw data into actionable intelligence that impacts various facets of business operations.
Enhancing Strategic Planning
Strategic planning is vital for long-term organizational success. Accenture leverages data analytics to provide profound insights that inform strategic decisions. By utilizing advanced analytical techniques, businesses can identify market trends, customer preferences, and competitive landscapes.
The incorporation of data analytics into strategic planning has several benefits:
- Precision in Forecasting: Accurate data forecasts can significantly enhance the ability to anticipate market shifts.
- Informed Risk Management: Businesses can identify potential risks through predictive analytics, allowing proactive measures to be put in place.
- Resource Allocation: Data-driven insights help organizations allocate resources more efficiently, ensuring optimal use of finances and manpower.
"Data analytics enables organizations to make informed decisions that are based on evidence rather than intuition."
Furthermore, integrating data analytics into strategic planning fosters innovation. Teams can explore new business models and product offerings based on analyzed consumer behavior and trends. Accenture's comprehensive approach ensures that these insights are relevant, timely, and tailored to specific business objectives.
Optimizing Operational Efficiencies
In addition to enhancing strategic planning, data analytics plays a crucial role in optimizing operational efficiencies. The process involves analyzing workflows and identifying bottlenecks in operations. Accenture applies various analytical methods to empower businesses in streamlining their operations, resulting in cost reduction and time savings.
Key elements that show how data analytics optimizes operations include:
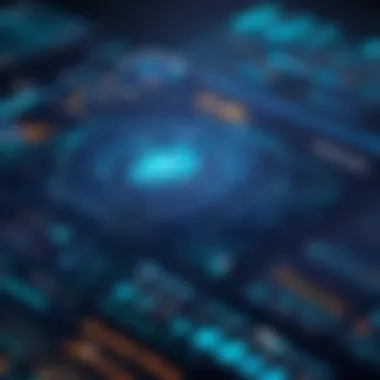
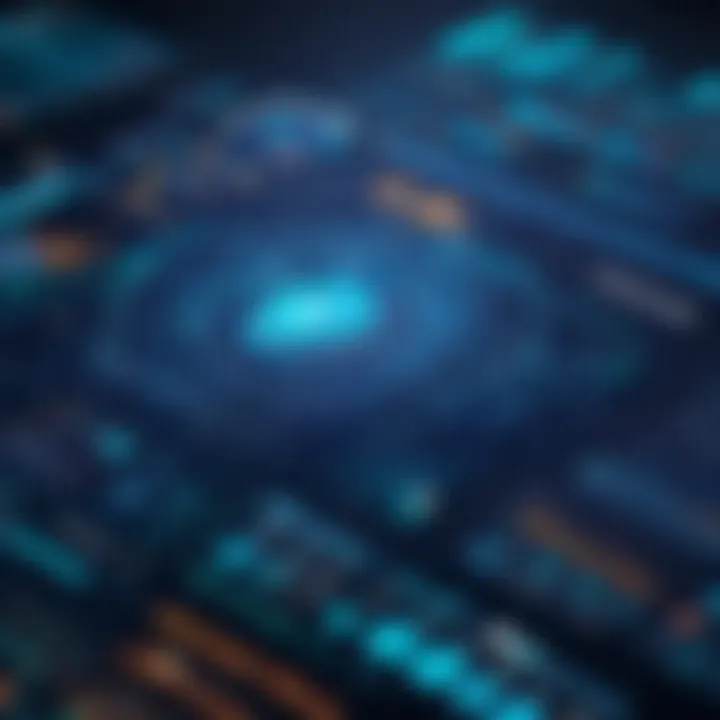
- Real-Time Monitoring: Organizations can monitor their operations in real-time, making necessary adjustments on-the-fly.
- Performance Metrics: By evaluating key performance indicators (KPIs), businesses can identify areas of improvement quickly.
- Supplier Performance Analysis: Data analytics can help assess supplier effectiveness, which directly impacts production timelines and costs.
The implementation of data analytics leads to significant improvements in productivity and efficiency, reinforcing an organization's capacity to adapt to dynamic market conditions. Therefore, understanding these impacts is crucial for IT professionals, cybersecurity experts, and students alike, as they explore the intersection of data and business strategy.
Challenges in Data Analytics Implementation
In today’s data-driven business environment, understanding the challenges associated with data analytics implementation is crucial for organizations looking to leverage insights for competitive advantage. Organizations like Accenture face various complexities that can hinder the effectiveness of their analytics programs. Acknowledging these challenges allows businesses to develop targeted strategies for mitigation, ultimately improving their data analytics capabilities.
Data Quality Issues
Data quality is a cornerstone for any successful data analytics initiative. Poor quality data can significantly skew analytics results, leading to misguided decisions. Common data quality issues include inaccuracy, incompleteness, and inconsistency. Accenture places emphasis on ensuring data integrity through robust data governance practices.
Organizations must frequently validate the data they collect to maintain high standards. This includes checking for missing values and ensuring that data inputs come from reliable sources. The cost of poor data quality can manifest in various ways, including wasted resources, tarnished customer relationships, and missed growth opportunities. Accenture's approach often integrates automated data cleaning solutions, enhancing data reliability and streamlining analytics workflows.
"Data quality is not just about correctness; it's about trustworthiness, ensuring that data informs decisions effectively."
Integration Problems
Integration problems occur when disparate systems fail to communicate effectively, resulting in silos of information. This challenge can impede comprehensive analysis and limit insights that could be gained from a holistic view of data. Accenture emphasizes that seamless data integration is critical to achieving impactful analytics outcomes.
Organizations need to adopt flexible systems capable of integrating various data sources including databases, applications, and cloud services. When systems do not effectively integrate, it leads to duplicated efforts and inconsistent datasets across departments. Aggregating data from multiple platforms often requires investments in integration tools and technologies. Accenture often utilizes middleware solutions that facilitate better data flow between systems, thereby maximizing the potential of collected data.
Future Trends in Data Analytics
The landscape of data analytics is increasingly dynamic, shaped by new technologies and evolving business needs. Understanding the future trends in data analytics is critical for organizations aiming to thrive in a competitive environment. These trends not only enhance operational efficiencies but also influence strategic directions. As businesses integrate advanced analytical capabilities, they unlock potential insights that were previously unattainable.
Emerging Technologies
Emerging technologies are at the core of future trends in data analytics. Innovations such as natural language processing, edge computing, and blockchain are changing how businesses gather and process data. For instance, natural language processing allows companies to analyze unstructured data, such as customer feedback, in real-time. This technology can reveal sentiments and preferences, providing valuable insights for product development and marketing strategies.
Edge computing reduces latency and bandwidth use by processing data closer to the source. This is especially important for IoT applications, where data is generated continuously. Faster processing ensures timely decision-making.
Blockchain technology enhances data integrity and security. As data breaches become more common, assurance that data has not been tampered with is crucial. By using blockchain, companies can create immutable data records, building trust with their customers. With these technologies, businesses can improve their data analytics capabilities, paving the way for deeper insights and strategic advantages.
Shifting Business Paradigms
As data analytics evolves, it drives significant shifts in business paradigms. Companies are moving from a reactive approach to a proactive stance in decision-making. This change involves anticipating market trends and consumer behavior, which can be achieved through advanced predictive analytics.
Data-driven decision-making is no longer an option; it has become a necessity. Organizations must foster a culture that encourages data literacy among all employees. This approach enables teams to harness analytics in their daily operations, leading to optimized performance.
Moreover, sustainability is gaining prominence in business strategies. Organizations are leveraging analytics to measure environmental impacts and improve resource management. Data analytics informs companies about energy consumption patterns and waste generation, helping them make more sustainable choices.
Ultimately, understanding these emerging technologies and shifting paradigms offers organizations the capacity to adapt. By effectively deploying data analytics, companies can transform their operations and enhance their competitive edge.
Closure
In this article, we have explored the multifaceted role of Accenture's data analytics capabilities in driving business transformation. By employing robust methodologies and advanced technologies, Accenture enables organizations to derive meaningful insights from complex data sets.
Summarizing Insights
The analysis presented throughout this article highlights several key insights:
- Comprehensive Data Utilization: Accenture leverages innovative data collection and processing techniques to ensure that organizations make data-driven decisions that cut across various operational levels.
- Impact Across Industries: Case studies from sectors such as healthcare, financial services, and retail demonstrate how data analytics can lead to significant improvements in efficiency and customer satisfaction.
- Decision-Making Enhancement: Data analytics frameworks not only optimize operational efficiency but also elevate strategic planning initiatives, making them more adaptive to market changes.
“Data is the new oil; it’s valuable, but if unrefined it cannot really be used.”
This statement reflects the essence of data analytics within business contexts. Without the right tools and methodologies, organizations cannot unlock their data's full potential.
The Path Forward
Looking ahead, businesses must recognize the transformative power of data analytics as a crucial aspect of their growth strategies. As trends like artificial intelligence and machine learning shape the future landscape, integrating these technologies into business processes will be essential. Organizations should consider the following:
- Continuous Learning: Embracing a culture of data-driven decision-making requires ongoing training and skill development for employees at all levels.
- Investment in Technologies: Companies must invest in cutting-edge tools to facilitate real-time data analysis and visualization.
- Strategic Partnerships: Collaborating with experts in data analytics, such as Accenture, enables businesses to gain access to specialized knowledge and resources that can expedite their transformation.
By addressing these considerations, organizations will not only adapt to current technological advancements but also prepare themselves for future challenges, ensuring longevity and success in an increasingly data-centric world.
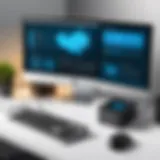
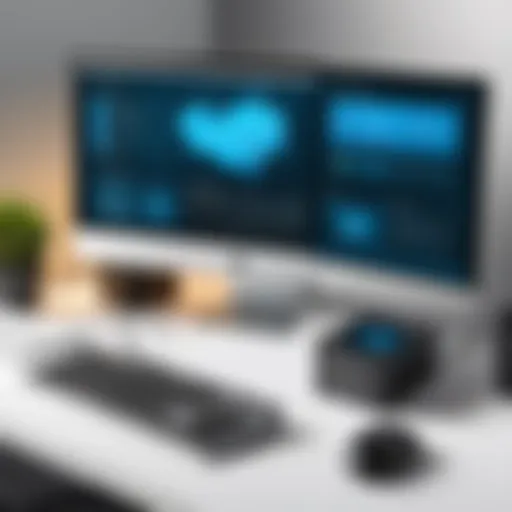