Unveiling the Latest Advancements in Artificial Intelligence Concepts
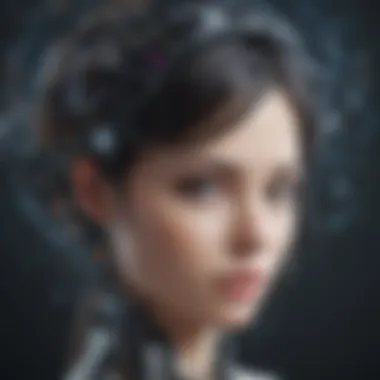
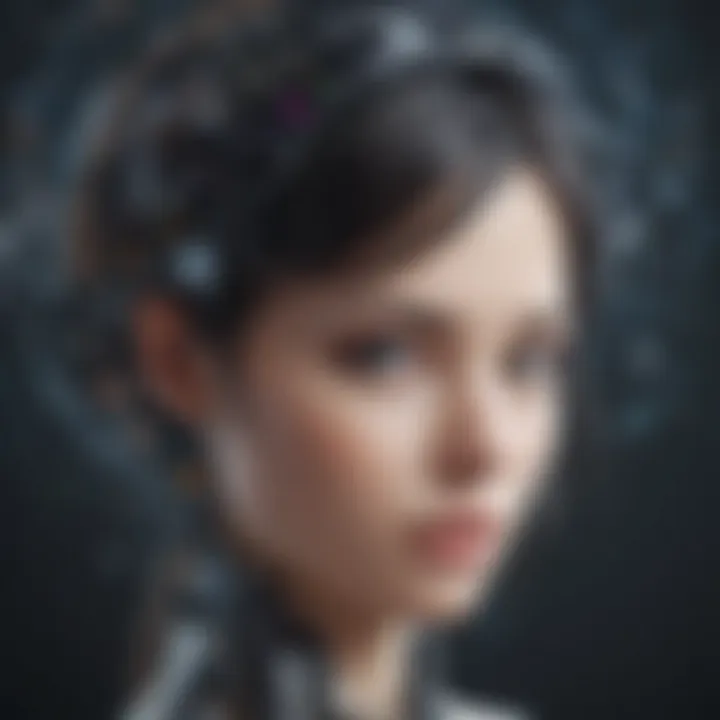
Understanding Advanced AI Concepts in Modern Technology
This section will delve into the complex realm of artificial intelligence (AI), providing a detailed examination of the latest advancements in the field. From machine learning algorithms to neural networks, readers will gain valuable insights into the cutting-edge technologies that are reshaping the digital landscape.
Introduction to AI Concepts
In this sub-section, we will lay the groundwork by introducing fundamental AI concepts. We will explore the basic principles of AI, machine learning, and deep learning. By understanding these core concepts, readers will be better equipped to comprehend the advanced AI topics that will be discussed further in the article.
Key Terms and Definitions
Within the intricacies of AI concepts lie a plethora of technical terms and definitions. This part will highlight key terminology such as artificial neural networks, natural language processing, and computer vision. Clarifying these definitions will facilitate a deeper understanding of the advanced AI concepts that will follow.
Overview of Cutting-Edge Technologies
This segment will provide an overview of the most significant advancements in AI. It will cover topics like reinforcement learning, generative adversarial networks, and explainable AI. With a clear overview of these technologies, readers will be prepared to delve into the specifics of each concept in the subsequent sections.
Stay tuned for the next sections where we will dissect the intricate details of advanced AI concepts and their real-world applications in modern technology environments.
Introduction to Artificial Intelligence
Artificial Intelligence (AI) stands at the forefront of technological advancements, revolutionizing various industries. In this article, we delve into the core elements of AI, exploring its profound implications on modern technology. From machine learning to cognitive computing, AI paves the way for unprecedented innovation and efficiency.
Understanding the Fundamentals of AI
Definition of AI
The Definition of AI encapsulates the essence of simulating human intelligence in machines. It serves as the foundation for developing intelligent systems capable of learning, reasoning, and problem-solving. The key characteristic of the Definition of AI lies in its ability to mimic cognitive functions, enabling machines to perform tasks autonomously. This facet of AI offers an array of benefits, including enhanced productivity and decision-making capabilities, making it a pivotal subject in this article.
Historical Evolution
The Historical Evolution of AI traces the evolutionary path of artificial intelligence from its nascent stages to present-day sophistication. Understanding the historical context provides insights into the progression of AI technologies, marking significant milestones and breakthroughs. Despite its advantages, the Historical Evolution of AI presents challenges such as scalability and ethical dilemmas that need careful consideration in this article.
Key Components
The Key Components of AI encompass the fundamental building blocks that drive intelligent systems. From algorithms to neural networks, each component plays a crucial role in shaping AI capabilities. Highlighting the unique features of Key Components sheds light on the complexity and versatility of AI technologies, paving the way for exploring advanced concepts and applications in modern technology.
Types of AI Systems
Reactive Machines
Reactive Machines operate based on predefined algorithms without storing past data or utilizing memory. Their ability to react to specific inputs in real-time showcases efficiency in tasks that demand quick decision-making. While offering advantages in speed and simplicity, Reactive Machines may face limitations in handling complex scenarios that require context awareness, a critical aspect discussed in this article.
Limited Memory AI
Limited Memory AI extends the capabilities of reactive systems by integrating memory for short-term data retention. This enhancement enables machines to learn from recent experiences and adapt their responses accordingly. The pivotal characteristic of Limited Memory AI lies in its ability to leverage past interactions, opening avenues for enhanced decision-making in dynamic environments crucial for modern technology applications.
Theory of Mind AI
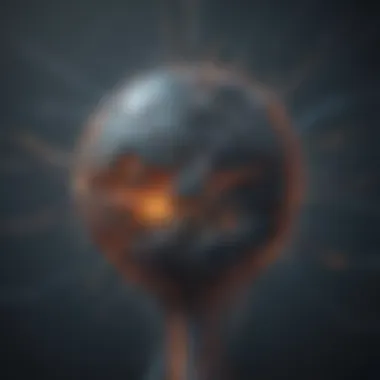
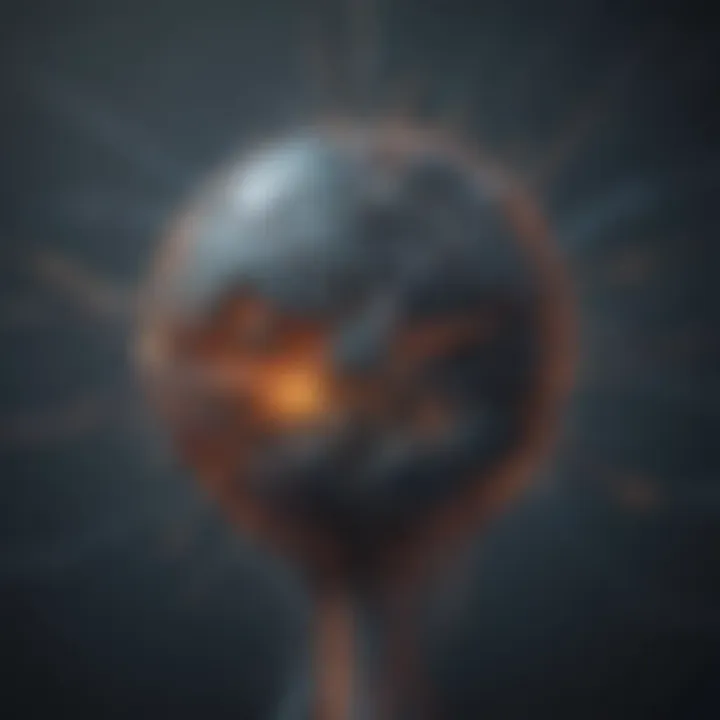
Theory of Mind AI represents a cognitive milestone, enabling machines to comprehend and anticipate human behavior and mental states. By ascribing beliefs, intentions, and emotions to others, these systems exhibit advanced social intelligence. The distinctive feature of Theory of Mind AI lies in its capacity to empathize and interact meaningfully with users, presenting opportunities and challenges discussed in detail within this article.
Significance of AI in Modern Technology
Impact on Various Industries
The Impact on Various Industries showcases AI's transformative influence across diverse sectors, revolutionizing processes and driving innovation. By optimizing operations, enhancing customer experiences, and unlocking new revenue streams, AI fosters growth and competitiveness. Exploring the impact of AI in various industries unveils a spectrum of advantages and challenges, underscoring its critical role in reshaping the technological landscape.
Future Implications
Future Implications of AI probe into the potential ramifications of integrating advanced technologies into society. Anticipating societal shifts, economic disruptions, and ethical considerations is imperative in navigating the evolving AI landscape. Assessing the future implications guides strategic decision-making and policy formulations, addressing the ethical, legal, and social dimensions associated with AI adoption in the digital era.
Machine Learning and Deep Learning
Machine learning and deep learning play a pivotal role in the evolution of artificial intelligence, making them essential topics to explore in this comprehensive article. By delving into the intricacies of machine learning and deep learning, readers can grasp the significance of these concepts in shaping modern technology landscape. These disciplines offer powerful tools for data analysis and pattern recognition, allowing for the development of intelligent systems that can learn from experience and improve over time. Understanding machine learning and deep learning is crucial for staying abreast of the latest advancements in AI.
Core Concepts of and
Algorithms and Models
Algorithms and models are fundamental components of machine learning and deep learning, driving the learning process and decision-making within AI systems. The selection of appropriate algorithms and models is critical to the success of AI applications. Algorithms like decision trees, linear regression, and neural networks form the backbone of machine learning, each with its unique characteristics and capabilities. Knowing how to choose and optimize algorithms and models can significantly impact the outcomes of AI projects. While algorithms and models offer versatile and efficient solutions for processing data and making predictions, they also pose challenges in terms of interpretability and computational complexity.
Training and Testing Data
Training and testing data are essential elements in the machine learning and deep learning pipelines, enabling algorithms to learn and make accurate predictions. The quality and quantity of training data directly influence the performance of AI models, emphasizing the importance of data preprocessing and feature engineering. Testing data helps evaluate the generalizability of AI models and measure their performance under different conditions. Balancing the training and testing datasets is crucial for building robust and reliable AI systems. However, sourcing and preparing high-quality data sets can be labor-intensive and time-consuming, presenting a common bottleneck in AI development.
Neural Networks
Neural networks are at the forefront of deep learning, mimicking the structure and function of the human brain to process complex information and derive meaningful insights. Their ability to extract features from raw data and perform hierarchical learning makes them incredibly powerful in tasks like image recognition, speech synthesis, and natural language processing. Neural networks consist of interconnected layers of neurons that transmit signals and compute outputs based on weighted inputs. While neural networks excel in capturing intricate patterns and relationships in data, they require substantial computational resources for training and inference, posing scalability challenges in large-scale AI applications.
Applications in Real-World Scenarios
Natural Language Processing
Natural language processing (NLP) is revolutionizing communication between humans and machines, enabling systems to understand and generate human language. NLP techniques like sentiment analysis, named entity recognition, and machine translation empower AI systems to interpret text, speech, and context, opening avenues for chatbots, virtual assistants, and content summarization. Despite their transformative potential, NLP algorithms face challenges in handling diverse languages, dialects, and semantic nuances accurately. Overcoming these obstacles is essential for enhancing the effectiveness and accessibility of NLP applications.
Computer Vision
Computer vision is reshaping industries by enabling machines to interpret and analyze visual information like humans. From object detection to image classification, computer vision algorithms underpin various applications such as autonomous vehicles, medical imaging, and augmented reality. Convolutional neural networks are widely used in computer vision tasks for their ability to extract spatial features and recognize patterns in images. However, ensuring robustness and reliability in computer vision systems remains a significant challenge due to factors like lighting conditions, occlusions, and image distortions.
Healthcare
Healthcare stands to benefit greatly from machine learning and deep learning technologies through applications like disease diagnosis, medical image analysis, and personalized treatment planning. By leveraging patient data and medical imaging, AI-powered healthcare systems can enhance diagnostic accuracy, treatment outcomes, and patient care. However, integrating AI into healthcare workflows requires addressing regulatory compliance, data privacy, and ethical considerations to ensure patient safety and data security. Collaborating with healthcare professionals and stakeholders is crucial for deploying AI solutions that align with clinical requirements and ethical standards.
Challenges and Ethical Considerations
Bias and Fairness
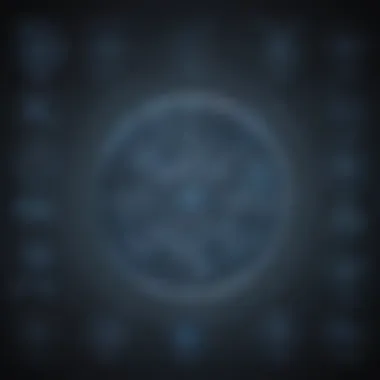
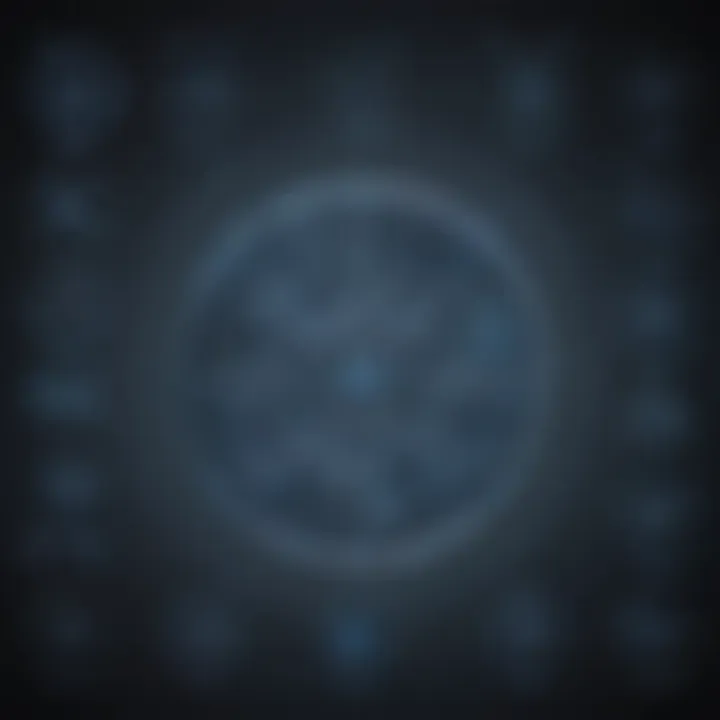
Bias and fairness are critical considerations in AI algorithms and models to ensure equitable decision-making and avoid discriminatory outcomes. Detecting and mitigating bias in training data and algorithm design is essential for building inclusive and unbiased AI systems. However, achieving fairness in AI applications is a complex and ongoing process, as biases can manifest in various forms and contexts. Transparent and responsible AI development practices are necessary to address bias and promote fairness in machine learning and deep learning models.
Privacy Concerns
Privacy concerns arise from the growing collection and analysis of personal data by AI systems, posing risks to individuals' privacy and autonomy. Safeguarding sensitive information and ensuring data confidentiality are paramount in AI applications to maintain trust and compliance with data protection regulations. Implementing encryption, anonymization techniques, and access controls can help mitigate privacy risks and protect user data from unauthorized access or misuse. Transparency and accountability in data processing practices are essential for establishing a privacy-conscious culture in AI-driven environments.
Transparency
Transparency in AI systems involves providing users with visibility into how algorithms make decisions and process data, fostering trust and understanding. Explainable AI techniques like model interpretability and algorithmic transparency enable stakeholders to comprehend AI outputs and identify potential biases or errors. Enhancing transparency in AI design and deployment promotes accountability and ethical use of AI technologies. However, achieving transparency without compromising proprietary information or trade secrets poses challenges for organizations seeking to balance openness with intellectual property protection.
Neural Networks and Cognitive Computing
Neural networks and cognitive computing play a pivotal role in the realm of artificial intelligence, showcasing advanced capabilities that have revolutionized modern technology. By leveraging intricate algorithms inspired by the human brain, neural networks enable machines to learn, adapt, and make decisions autonomously. This article unravels the complexities of neural networks and cognitive computing, shedding light on their significance in pushing the boundaries of AI innovation.
Architectures and Functions
Feedforward Networks
Feedforward networks, a fundamental architecture in neural networks, facilitate the flow of data in one direction without forming any loops. This linear structure allows for streamlined processing of information, making it an efficient choice for tasks requiring straightforward decision-making processes. The key characteristic of feedforward networks lies in their ability to transform input data into meaningful outputs through interconnected layers of nodes. While their simplicity and ease of implementation make them popular for basic classification tasks, their lack of feedback loops may limit their capability to handle complex, dynamic data.
Recurrent Neural Networks
On the contrary, recurrent neural networks (RNNs) introduce a feedback mechanism, enabling them to retain memory of past information while processing current inputs. This recurrent architecture empowers RNNs to exhibit temporal dynamic behavior, making them well-suited for sequential data processing tasks such as language translation, speech recognition, and time series prediction. The unique feature of RNNs lies in their ability to connect previous states to the current computation, facilitating context-aware decision-making. However, challenges such as vanishing gradients and processing long-range dependencies may pose limitations to their effectiveness in certain scenarios.
Convolutional Neural Networks
Convolutional neural networks (CNNs) excel in extracting spatial hierarchies and patterns from input data through convolutional and pooling layers. This specialized architecture is ideal for image and video processing tasks, leveraging shared weights to detect features at different spatial positions. The key characteristic of CNNs lies in their capability to capture local patterns and spatial dependencies, allowing for robust image recognition and object detection. While CNNs are highly successful in computer vision applications, their resource-intensive nature and limited adaptability outside visual data domains may pose challenges in broader AI contexts.
Enhanced Learning Capabilities
Pattern Recognition
Pattern recognition, a core capability of neural networks, enables systems to identify regularities or similarities within data and make informed predictions or classifications. This ability to recognize patterns and trends in complex datasets is crucial for various AI applications, including anomaly detection, predictive maintenance, and fraud detection. The unique feature of pattern recognition lies in its adaptability to diverse data modalities, ranging from images and signals to textual information. While pattern recognition enhances AI systems' ability to derive meaningful insights, challenges related to imbalanced datasets and overfitting necessitate careful optimization and validation.
Adaptability
The adaptive nature of neural networks allows them to adjust their internal parameters based on feedback received during training, thereby improving performance and generalization capabilities. Adaptability enables AI systems to learn from new data instances, refine their predictions, and continuously enhance their decision-making processes over time. The key characteristic of adaptability lies in the network's ability to update its weights and biases through iterative training, fostering resilience against noise and variability in input data. While adaptability confers flexibility and responsiveness to neural network models, risks of overfitting and slow convergence rates highlight the importance of balanced training strategies.
Self-Learning
Self-learning mechanisms embedded within neural networks enable systems to autonomously acquire knowledge, refine their strategies, and improve performance without explicit human intervention. This automated learning process involves self-adjustment of model parameters, exploration of novel solutions, and adaptation to evolving data distributions. The unique feature of self-learning lies in the network's capacity to iterate on its own learning dynamics, promoting continuous improvement and robustness in decision-making. While self-learning empowers AI systems to self-optimize and adapt to changing environments, challenges related to interpretability and ethical considerations may arise, warranting ethical guidelines and monitoring mechanisms.
Inspiration from Human Brain
Biological Analogies
Drawing inspiration from the intricate structures and functions of the human brain, neural networks mimic biological analogies to enhance cognitive computing. By modeling computational processes after neuronal communication pathways, AI systems can simulate complex behaviors such as learning, memory consolidation, and information retrieval. The key characteristic of biological analogies lies in their ability to capture the brain's parallel processing capabilities and hierarchical organization, fostering efficient information processing and decision-making. While biological analogies unlock new possibilities for AI advancements, uncertainties regarding the fidelity of neural network models to biological systems underscore the need for interdisciplinary research and cross-domain collaborations.
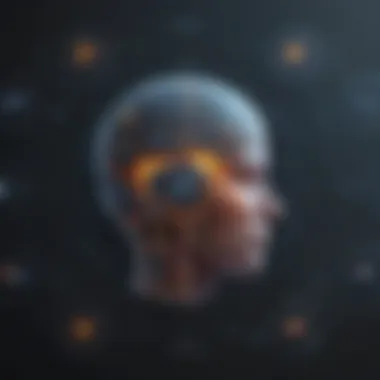
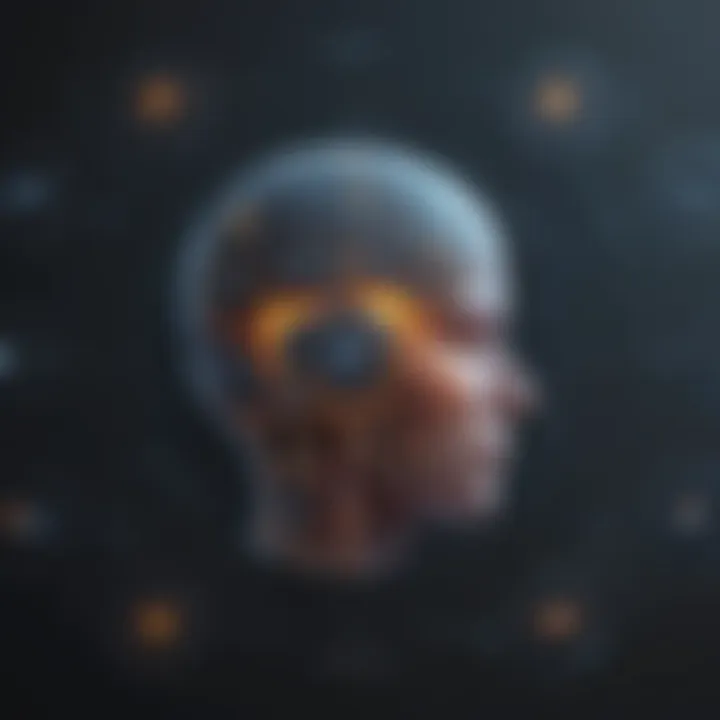
Connection to Neuroscience
The integration of neuroscience principles into AI research enhances our understanding of cognitive processes, brain functionalities, and behavioral dynamics. By establishing connections between neural network operations and neuroscientific findings, researchers can unravel the mechanisms underlying learning, attention, and perception. The unique feature of connection to neuroscience lies in the synergy between computational models and empirical brain studies, enriching AI models with insights from cognitive neuroscience. While this interdisciplinary approach fuels innovations in AI technologies, discrepancies between artificial and biological systems may limit the direct translation of neuroscientific discoveries into practical AI applications.
Future Innovations
Exploring the frontiers of neural networks and cognitive computing, researchers continually strive to push the boundaries of AI innovation and develop novel solutions for complex computational challenges. Future innovations in neural networks may involve hybrid architectures combining different neural network types, neuro-inspired algorithms for improved learning dynamics, and brain-computer interfaces for seamless human-machine interactions. The key characteristic of future innovations lies in their potential to revolutionize diverse sectors ranging from healthcare and transportation to finance and entertainment, shaping the future of AI-enabled technologies. While these advancements hold promise for addressing societal needs and augmenting human intelligence, ethical considerations, and regulatory frameworks must evolve in tandem to ensure responsible AI deployment and mitigate unintended consequences.
Ethical and Societal Implications of AI
In the realm of Artificial Intelligence (AI), the examination of Ethical and Societal Implications holds a critical significance. As AI technologies continue to advance, it becomes imperative to consider the broader consequences they may have on our society and the ethical dilemmas they pose. Understanding the impact of AI on various facets of our lives is essential for navigating the evolving digital landscape. Ethical considerations surrounding AI encompass issues like data privacy, algorithmic bias, and transparency, influencing the trustworthiness of AI systems. Meanwhile, societal implications delve into how AI affects employment patterns, economic structures, and public perception. Delving into these topics sheds light on the responsibilities that come with integrating AI into different domains.
Impact on Employment and Economy
Automation Effects
Within the domain of AI, Automation Effects represent a pivotal aspect with profound implications for industries and economies. The deployment of automated systems, powered by AI technologies, brings about efficiency enhancements and productivity gains. This streamlining of processes leads to cost reductions and accelerates task completion, driving operational effectiveness. However, Automation Effects can also result in workforce displacements as tasks traditionally performed by humans transition to automation. This shift necessitates reskilling and upskilling initiatives to align the workforce with the demands of automation-centric environments.
Job Displacement
Job Displacement, another consequential facet of AI integration, underscores the restructuring of job markets due to automation. The displacement of roles through AI-driven processes prompts the need for job reallocation and the creation of new employment opportunities in emerging sectors. While automation streamlines workflows, it necessitates strategic workforce planning to mitigate societal challenges stemming from job loss.
Economic Shifts
The integration of AI into industries catalyzes Economic Shifts by reshaping production paradigms and market dynamics. AI-driven automation optimizes resource allocation, enhances product quality, and introduces innovative technological solutions. This fosters economic growth, stimulates market competitiveness, and encourages entrepreneurial initiatives. However, economic shifts resulting from AI adoption can trigger disruptions in traditional business models, necessitating adaptive strategies to navigate evolving market landscapes.
Regulatory Frameworks and Governance
Data Privacy Laws
Within the context of AI, Data Privacy Laws play a pivotal role in safeguarding individuals' personal information from unauthorized access or misuse. These regulations establish guidelines for data collection, storage, and usage, ensuring compliance with ethical standards and protecting user privacy. Data Privacy Laws contribute to building trust between consumers and AI systems, fostering transparency and accountability in data handling practices.
Ethical Guidelines
Ethical Guidelines in AI governance serve as frameworks for ethically responsible development and deployment of AI technologies. These guidelines delineate principles for ensuring fairness, accountability, and transparency in AI systems. By adhering to ethical standards, organizations and developers can mitigate potential biases in algorithms, uphold user trust, and align AI advancements with societal values.
International Collaboration
Promoting International Collaboration is essential for establishing cohesive regulatory standards and governance frameworks in the global AI landscape. Collaboration among nations facilitates the exchange of best practices, harmonizes regulatory approaches, and fosters cross-border partnerships in AI research and development. International cooperation enhances the ethical oversight of AI technologies, encourages knowledge sharing, and enables collective efforts to address transnational AI challenges.
Public Perception and Trust in AI
Media Portrayal
The portrayal of AI in the media shapes public perception and influences attitudes towards AI technologies. Media narratives can either instill confidence in AI capabilities or fuel apprehensions regarding its societal impact. Accurate and balanced media representations play a crucial role in enhancing public understanding of AI, dispelling myths, and elucidating the benefits and challenges associated with AI integration.
Education and Awareness
Educational initiatives and Awareness campaigns are instrumental in fostering informed discourse on AI technologies and their implications. By imparting knowledge about AI principles, applications, and ethical considerations, educational programs empower individuals to make informed decisions regarding AI utilization. Increased public awareness cultivates a critical appraisal of AI advancements, promoting digital literacy and ethical engagement in AI discourse.
Building Trust
Establishing Trust between users and AI systems hinges on transparent communication, ethical practices, and reliable performance. Trustworthy AI frameworks prioritize user privacy, data security, and algorithmic accountability. Building trust requires organizations to prioritize ethical standards, engage in transparent AI development processes, and prioritize user-centric design principles to engender confidence in AI systems.