Exploring the Versatile Applications of NLP
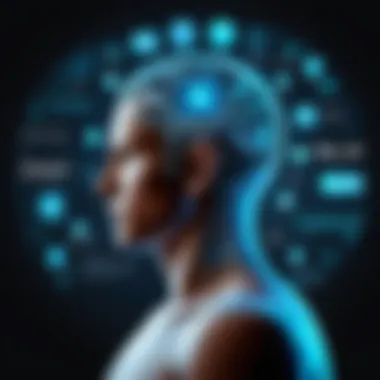
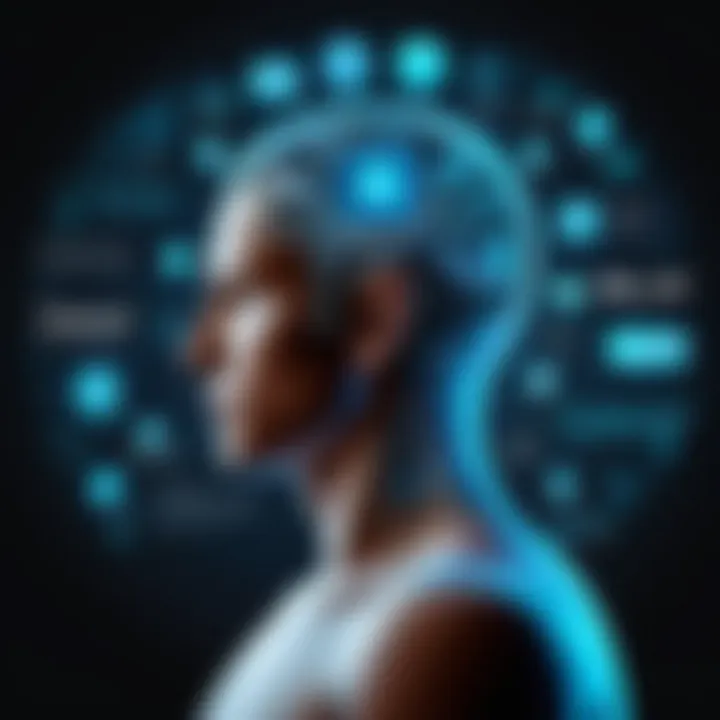
Intro
Natural Language Processing, often abbreviated as NLP, is gaining traction across a variety of sectors, fundamentally altering how organizations engage with data and customers. This technology marries linguistics with artificial intelligence to enable machines to understand, interpret, and generate human language. The implications of its applications are far-reaching, touching everything from customer service to healthcare, and as we dive deeper into this theme, itâs clear that the landscape is rapidly evolving.
Through this article, we will unpack the multifaceted use cases of NLP, shedding light on the specific ways organizations harness its capabilities to enhance productivity and improve communication. Notably, the rise of conversational agents, sentiment analysis, and automated content generation demonstrates the diverse functionalities NLP offers. However, itâs equally imperative to address the associated challenges that accompany its adoption, such as ethical considerations and technical limitations.
Each section aims to deliver clear insights into the realm of NLP, exploring both established and emerging avenues for its application. Letâs embark on this exploration to see how NLP is not just changing the way we process language, but also reshaping entire industries and their operational methodologies.
Preamble to Natural Language Processing
Natural Language Processing (NLP) stands at the intersection of computer science, linguistics, and artificial intelligence, serving as a critical enabler for machines to understand, interpret, and generate human language in a way that is not just computationally effective but also intuitively meaningful. The rise of NLP can be traced back to the increasing demands for technology that can bridge the communication gap between humans and machines, a gap that often seems like a chasm.
One key aspect of NLP is its capability to transform unstructured data into structured insights. This is achieved by employing algorithms that mimic the cognitive functions of human beings, allowing machines to comprehend context, emotion, and nuances within text. For IT professionals, understanding NLP is not just beneficialâitâs essential for modern data-centric strategies.
Definition and Importance
NLP can be defined as a branch of artificial intelligence that focuses on the interaction between computers and humans through natural language. The primary objective is to enable systems to interpret and respond to user inputs in a way that feels natural and intuitive. This broad definition encompasses several techniques, including tokenization, parsing, sentiment analysis, and language generation.
The importance of NLP is underscored by its diverse applications across various sectors. Businesses utilize NLP for enhancing customer service through chatbots, analyzing consumer sentiment on social media, and improving the search functionality on their websites. Health institutions deploy NLP for clinical documentation, enabling faster patient interaction analysis, thus improving healthcare delivery. Beyond professional environments, NLP influences how individuals engage with technology in daily life, through virtual assistants like Siri or Google Assistant, who understand and respond to our commands with increasing accuracy.
Moreover, as organizations continue to delve deeper into data analytics, NLP offers valuable opportunities to extract insightful patterns and trends from vast datasets, which otherwise would be difficult to harness. In a world inundated with information, having the tools to sift through and make sense of it is priceless.
Brief History of NLP
The history of NLP is as dynamic as the technology itself, evolving from rudimentary systems to advanced models that underpin many of todayâs applications. The journey began in the 1950s when researchers experimented with basic natural language translations. A landmark moment was the Georgetown-IBM experiment in 1954, which demonstrated the potential for automated translation from Russian to English.
In the decades that followed, the field saw significant hurdles, mainly due to the limitations of computational power and a lack of robust linguistic theory. However, in the 1980s, the introduction of machine learning algorithms started to shift the landscape. With advancements in computational linguistics and the increasing availability of large datasets, NLP began to flourish.
Fast forward to the present day, machine learning and deep learning have revolutionized how we approach language processing. Technologies like recurrent neural networks (RNNs) and transformers have enabled more complex understandings of context and semantics, thus paving the way for innovations in chatbot technologies, automated content generation, and sentiment analysis. As these advances continue to unfold, the potential for NLP expands, bringing forth new inspirational use cases and reshaping established domains.
"Natural Language Processing is not just about understanding words. Itâs about understanding the human experience behind those words."
In sum, the introduction to NLP encapsulates a vital piece of the puzzle in todayâs data-intensive world, offering innumerable possibilities for optimizing communication between humans and computers, all while challenging us to think critically about the implications of this technology.
NLP in Customer Service
In an ever-evolving digital landscape, the incorporation of Natural Language Processing (NLP) in customer service is no longer just a novel innovation but a foundational element for many organizations looking to enhance customer experiences and streamline operations. The deployment of NLP tools helps companies respond swiftly to customer inquiries, analyze feedback effectively, and ultimately foster deeper connections with their clientele. Each interaction becomes an opportunity to build trust, image, and a sense of reliability, which is essential in todayâs competitive marketplace.
Chatbots and Virtual Assistants
One of the most visible applications of NLP is in the realm of chatbots and virtual assistants. These AI-driven tools can handle a multitude of customer queries, ranging from account inquiries to troubleshooting issues, all day and night. Many businesses have adopted chatbot technology to automate responses. For instance, companies like Zendesk and Drift use advanced NLP techniques to power their chatbots, allowing them to comprehend language nuances and intent behind customer queries.
Through machine learning and continuous training, these chatbots become increasingly proficient. Take, for example, a customer who types, "My order hasnât arrived yet". A well-trained chatbot utilizes NLP to grasp context, sentiment, and urgency, providing a relevant and empathetic response in real-time.
Moreover, integrating these tools into platforms like Slack or Microsoft Teams means they can also facilitate internal communications, making data easily accessible to employees. The beauty of chatbots lies in their ability to learn over timeâcollecting data about frequently asked questions allows them to enhance responses and reduce unnecessary handovers to human agents, therefore saving time and costs for businesses.
Sentiment Analysis for Feedback
Understanding customer emotions is crucial to improving services and products, which is where sentiment analysis comes into play. By employing NLP techniques, organizations can sift through massive amounts of feedback commentaries, reviews, and social media posts to gauge customer sentiment.
Imagine an airline receiving thousands of tweets post-flight; rather than trawling through them manually, NLP algorithms sort these comments into categoriesâpositive, negative, or neutralâallowing companies to monitor brand perception easily. Tools like Lexalytics and MonkeyLearn leverage sentiment analysis to derive meaningful insights from customer data.
This data can then be actionable. If a hotel notices a spike in negative feedback about cleanliness, they can swiftly address the issue before it escalates. A single negative review might seem trivial, but it can snowball into a broader public relations crisis; therefore, proactive sentiment analysis is indispensable. Additionally, in a world where online reputation can make or break a business, continuous monitoring becomes vital.
"In the era of digitization, being receptive to customer sentiment not only enhances satisfaction but also preserves brand integrity."
NLP in customer service is not merely about automation; it's about fostering rich, personal experiences. The conversation is evolving from transactions to relationships, and organizations that leverage NLP tools effectively stand to benefit immensely from improved service delivery, enhanced customer satisfaction, and ultimately, boosted loyalty.
Adopting these technologies means being ahead of the curve in recognizing and addressing customer needs. As the potential of NLP continues to be harnessed, businesses must remain agile, adapting their customer service strategies to remain relevant.
NLP Applications in Healthcare
Natural Language Processing (NLP) is carving out a significant niche in the healthcare sector. Its ability to interpret and analyze human language makes it an invaluable tool for healthcare professionals and organizations seeking to improve patient outcomes and streamline operations. The integration of NLP not only enhances clinical efficiency but also bridges the gap between patient interactions and data management, driving innovations across various healthcare processes.
Clinical Documentation Improvement
Clinical documentation is critical in healthcare, as it ensures that patient information is accurate, accessible, and comprehensive. NLP plays a pivotal role here, automating the transcription of medical records and providing tools for better documentation practices. With NLP, healthcare providers can swiftly convert voice notes into well-structured text, thus saving time and reducing clerical errors. This efficiency allows clinicians to focus more on patient care rather than paperwork.
The adoption of automated documentation systems powered by NLP can significantly enhance the quality of data captured. For instance, systems like M*Modal and Nuance's Dragon Medical leverage NLP to assist physicians by suggesting appropriate medical codes and terminology as they document patient encounters. This capability not only improves the quality of clinical data but also enhances billing accuracy, leading to better revenue cycle management.
Moreover, NLP can analyze existing documentation to identify deficiencies in patient data, prompt necessary updates, and ensure compliance with regulatory standards. The result is a more robust clinical documentation process that supports better healthcare delivery.
Patient Interaction Analysis
Understanding patient interactions is crucial for improving healthcare services. NLP technologies offer healthcare providers a window into the sentiments, concerns, and needs of patients through voice and text analysis. By evaluating patient communicationsâfrom telephonic consultations to written feedbackâhealthcare organizations can extract valuable insights.
For example, organizations can utilize sentiment analysis tools to gauge the emotional tone in patient communications. This analysis helps in identifying common concerns or areas of dissatisfaction, which can then be addressed to enhance patient experiences. Additionally, NLP can track changes in patient sentiment over time, enabling providers to assess the effectiveness of treatments or service improvements.
Furthermore, NLP can facilitate more personalized patient interactions. By analyzing communication patterns, healthcare providers can tailor their responses and follow-ups based on individual patient needs. This is particularly helpful in chronic disease management, where understanding a patientâs emotional and psychological state can significantly influence treatment adherence and outcomes.
NLP in healthcare ultimately allows the provider to shift from reactive to proactive care, enabling timely interventions and a more patient-centric approach.
In summary, NLP applications in healthcare are not merely about enhancing operational efficiency but are also centered around improving the quality of care offered to patients. As these technologies evolve, they promise to open new avenues for better health outcomes and more effective patient engagement.
Educational Uses of NLP
Natural Language Processing (NLP) plays a transformative role in education by personalizing learning experiences and enhancing assessment methodologies. The landscape of education is evolving, thanks largely to the adoption of advanced technologies. With NLP, educational institutions can tailor instruction to individual needs, ultimately fostering better outcomes for learners of all backgrounds.
Personalized Learning Environments
At the heart of promoting effective learning experiences lies the concept of personalized education. NLP facilitates this by enabling adaptive learning systems that analyze student interactions and preferences. For instance, platforms like Khan Academy utilize NLP to assess student responses in real-time, adjusting the difficulty of exercises accordingly. This approach encourages mastery by ensuring that lessons progress at a pace suited to each learner.
âPersonalized learning is like tailoring a suit; it must fit the individual perfectly.â
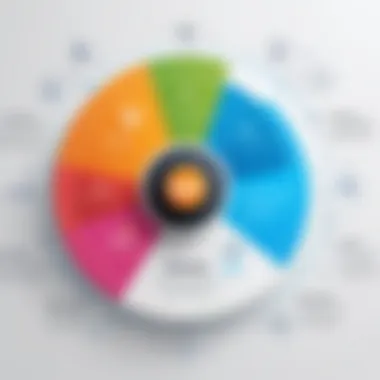
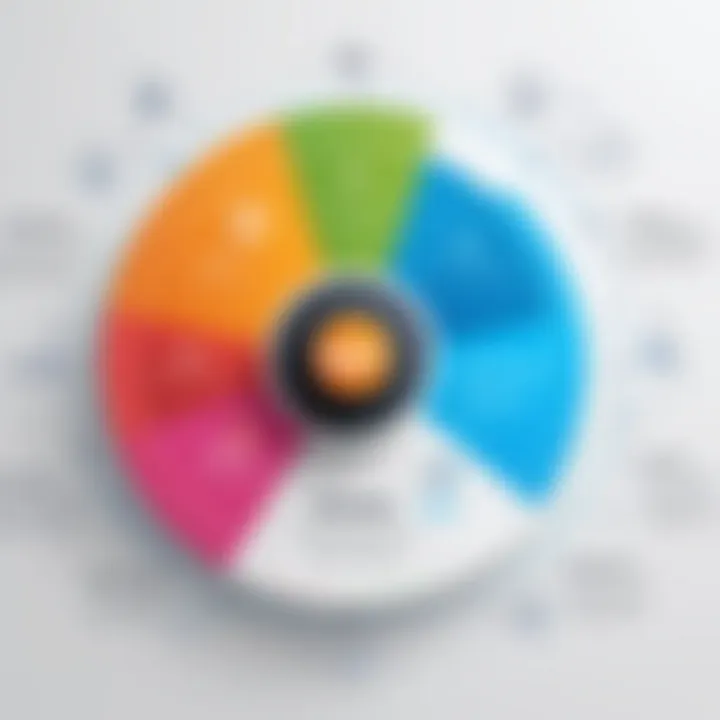
Consider how language models can augment classroom instruction. Gone are the days when one-size-fits-all strategies ruled the classroom. With NLP-enabled systems, educators now have tools that help identify specific areas where a student struggles. From phonics to mathematical concepts, NLP can swiftly pinpoint each studentâs learning gaps. As a result, teachers can design targeted interventions that empower students to learn effectively and independently.
- Personalized learning environments increase engagement by catering to diverse learning styles.
- They provide immediate feedback, making it easier for students to understand their strengths and weaknesses.
- Such environments promote a sense of ownership over learning, fostering a lifelong love for education.
Yet, fostering personalized education isn't without its hurdles. Ensuring data privacy remains a paramount concern. Institutions must navigate these challenges carefully, implementing measures to protect student information while leveraging NLP's capabilities.
Automated Essay Scoring
Another intriguing application of NLP within education is automated essay scoring, a game-changer for assessing student writing in a fair and efficient manner. Traditionally, essay grading has been time-consuming, often leading to inconsistencies. By utilizing algorithms trained on large datasets of written work, platforms can provide rapid feedback while maintaining accuracy in scoring.
Automated scoring systems analyze factors such as grammar, punctuation, coherence, and even creativity. For instance, tools like Grammarly not only assist students in editing their work but also provide insights into their writing tendencies. This results in a win-win: educators spend less time grading and more time focusing on student engagement, while pupils receive immediate feedback that drives improvement.
- Automated essay scoring helps maintain grading consistency across various subjects.
- It empowers students with instantaneous and constructive feedback, often boosting writing quality as they learn in real-time.
As with personalized learning environments, challenges do arise. Critics of automated essay scoring often point towards the potential for mechanical biases in evaluations, raising questions about equity in assessment. Therefore, while these systems offer incredible value, it remains essential for educators to use them as supplementary tools rather than complete replacements for human judgment.
NLP in Data Analysis
Natural Language Processing plays a crucial role in data analysis, bridging the gap between human communication and computer understanding. As businesses accumulate vast amounts of unstructured data, extracting valuable insights can seem like finding a needle in a haystack. NLP provides the tools needed to sift through this data efficiently, enabling organizations to make informed decisions based on clear, actionable insights. The significance of incorporating NLP into data analysis cannot be overstated; it not only optimizes research but also enhances the quality of information derived from textual data.
Trend Identification in Unstructured Data
Trend identification is a fundamental aspect of data analysis, particularly when dealing with unstructured data such as social media posts, emails, or customer feedback. Unstructured data is typically abundant yet challenging to analyze directly due to its random nature. Here, NLP shines brightly by employing various techniques to identify trends and patterns in this raw data.
- Keyword Extraction: Using tools that pinpoint frequently occurring words or phrases helps in highlighting what is capturing attention over time. Businesses can tailor strategies based on these insights.
- Clustering: By grouping similar data points, organizations can discover hidden themes within unstructured content. This could reveal emerging trends in consumer behavior or new market demands.
- Sentiment Analysis: This approach gauges public opinion around specific topics. For instance, analyzing tweets about a recent product launch can help brands assess market reception in real-time.
- Predictive Analytics: NLP allows analysts to create models that not only recognize current trends but also predict future outcomes. This foresight can be immensely powerful for strategic planning.
With these methods, companies can be in the know and seize opportunities before they slip through their fingers. By employing NLP, organizations can drill down into volumes of data with clarity and speed, making them a step ahead in the competitive landscape.
Text Mining Techniques
Text mining is another pillar propping up the capabilities of NLP in data analysis. It involves extracting significant information from text documents and databases, turning raw data into knowledge.
- Information Retrieval: This technique retrieves relevant documents from large datasets based on user queries. Imagine scouring through millions of academic articles to find one specific reference â NLP automates and accelerates this process.
- Named Entity Recognition: By identifying entities such as people, organizations, or locations in text, businesses can obtain a clearer picture of the content theyâre working with. Knowing the key players mentioned in a series of articles about your industry offers strategic advantages.
- Natural Language Understanding: This encompasses the capability of machines to interpret and generate human-like responses to text. It is a complex yet rewarding facet of NLP that empowers data analysis tools to provide in-depth insights based on the semantic meaning behind the words.
"Text mining transforms the seemingly chaotic world of words into structured, quantifiable insights. This capability enhances decision-making processes across various sectors."
As organizations increasingly recognize the power of data, mastering text mining techniques will be paramount. With these tools, each piece of data becomes a potential asset, revealing stories and trends that guide future actions.
In summary, NLP's integration into data analysis delivers robust methodologies for handling unstructured data, including trend identification and text mining. This allows businesses to derive valuable insights that inform decisions, revealing trends and themes that could otherwise remain hidden in the deluge of information.
Enhancing Search Engines with NLP
The landscape of information retrieval has been shaped dramatically by the evolution of natural language processing. In todayâs world, filled with a constant stream of information, the ability of a search engine to sift through mountains of data is crucial. NLP enhances search engines in ways that greatly improve how users find and interact with information. The significance of integrating NLP into these systems cannot be overstated; it leads to better comprehension of user intent and enhances the overall user experience.
One of the primary benefits of using NLP in search engines is its ability to grasp context and semantics. Unlike traditional keyword-based searches that largely depend on exact matches, NLP understands the meaning behind the words. This capability allows search engines to return results that are more relevant to the user's query, thereby saving time and increasing satisfaction.
Moreover, as users become accustomed to conversational interfaces, expect systems that understand complex questions and follow-up inquiries. For instance, if a user asks, "What are the benefits of green tea?" and subsequently inquires, "How about weight loss?", the search engine should seamlessly recognize the context carried over from the first question. This fluidity transforms interactions, leading to a more intuitive experience.
Semantic Search Capabilities
When we talk about semantic search, we refer to the technology that seeks to understand the meanings and relationships behind queries, rather than merely matching keywords. This shift allows search engines to produce results that align closely with user intentions, significantly enriching their search results.
NLP plays a pivotal role in semantic search by leveraging techniques such as Named Entity Recognition (NER) and word embeddings. For example, when a user types "Apple products," a semantic search engine equipped with NLP can differentiate between the fruit and the company, powering more relevant search results related to technology.
Additionally, semantic search utilizes concepts like synonymy and antonymy. Rather than being restricted to the exact words typed, the search can pull in related terms. With algorithms recognizing relationships within vast datasets, users can find information they may not have even thought to search for, thus broadening their horizons.
Natural Language Queries
Natural language queries represent another leap forward in search technology, enabling users to interact in a conversational manner. This change simplifies the user experience, as one need not remember the precise phrasing to yield results. Instead of stringing together keywords, users can pose questions just like they would when talking to a person.
Take the query, "Show me Italian restaurants nearby with good reviews." Here, the search engine processes the nuances of the request, recognizing the context, the user's intent, and the importance of location and quality. This ability to handle nuanced language enhances user engagement and satisfaction.
Additionally, using NLP in this context allows for more advanced search features such as the ability to process voice commands. As virtual assistants gain traction in many households â think of Google Assistant or Amazonâs Alexa â users are increasingly turning to voice for searches. This trend necessitates robust NLP capabilities to understand and process spoken language effectively.
In summary, the integration of natural language processing into search engines is not simply a technical upgrade; it reflects a fundamental shift in how users seek and consume information. By prioritizing the complexities of human language, NLP ensures that search engines do more than just return pages of text. They become interactive tools that empower users to uncover knowledge more organically.
"With NLP, search engines are no longer just tools; they become companions in our quest for information."
This integration drives user satisfaction and engagement, making search engines not only more effective but also more aligned with the natural way people communicate.
NLP in Social Media Analysis
Social media platforms have changed the way people communicate, share information, and express their emotions. Given the vast amount of data produced daily, the role of Natural Language Processing (NLP) in analyzing and interpreting social media content becomes crucial. Understanding the public's thoughts and feelings towards brands, products, or events can offer invaluable insights for companies striving for improvement.
Monitoring Brand Sentiment
One significant application of NLP in social media analysis is monitoring brand sentiment. Companies use NLP algorithms to scrape social media posts, comments, and reviews, allowing them to gauge public opinion in real time. By identifying words and phrases that indicate positive or negative sentiment, businesses can respond quickly to changes in customer perceptions.
In practical terms, hereâs how it works:
- Data Collection: Brands gather data from various sources like Facebook, Twitter, and Reddit. Posts containing the brand name or related keywords are filtered out for analysis.
- Sentiment Analysis: The collected data is processed through sentiment analysis models, which classify the tone of each post. For example, if someone tweets, "I love using Brand X's products!", the algorithm tags this as positive. Conversely, a comment like, "Brand Y let me down today," gets a negative label.
- Reporting: The results are compiled into reports that show trends over time, allowing marketing teams to strategize based on public sentiment.
Companies can also identify potential crises before they escalate. A sudden uptick in negative mentions might signal an emerging issue, enabling timely action.
"Brand sentiment analysis through NLP gives companies the ability to not just react but to anticipate customer needs and sentiments."
Content Recommendation Systems
Another noteworthy use of NLP in social media is in content recommendation systems. These systems leverage user data and preferences to recommend relevant content, significantly improving user engagement and satisfaction. Here's how it functions:
- User Profiles: By analyzing the types of content users engage withâsuch as likes, shares, or commentsâNLP systems can create detailed profiles based on user preferences and interests.
- Natural Language Understanding: By understanding the natural language used in posts, comments, and tags, the systems can determine the themes and topics that resonate most with users. For instance, if a user regularly interacts with travel-related content, the system prioritizes similar posts visually formatted to catch their eye.
- Delivering Recommendations: The final step involves tailoring the social media feed. When a user logs in, the platform curates posts that align with analyzed preferences, enhancing the relevance of seen content.
Through effective use of NLP in content recommendation systems, platforms not only keep users engaged but also drive ads more strategically. Increased interaction translates directly to higher advertisement performance, benefitting both the platform and its advertisers.
NLP for Content Creation
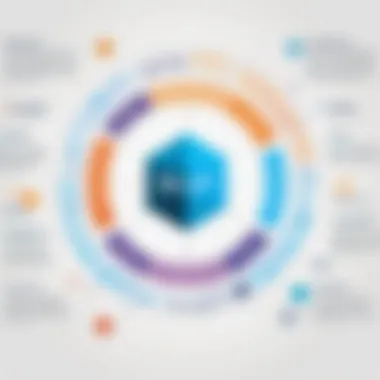
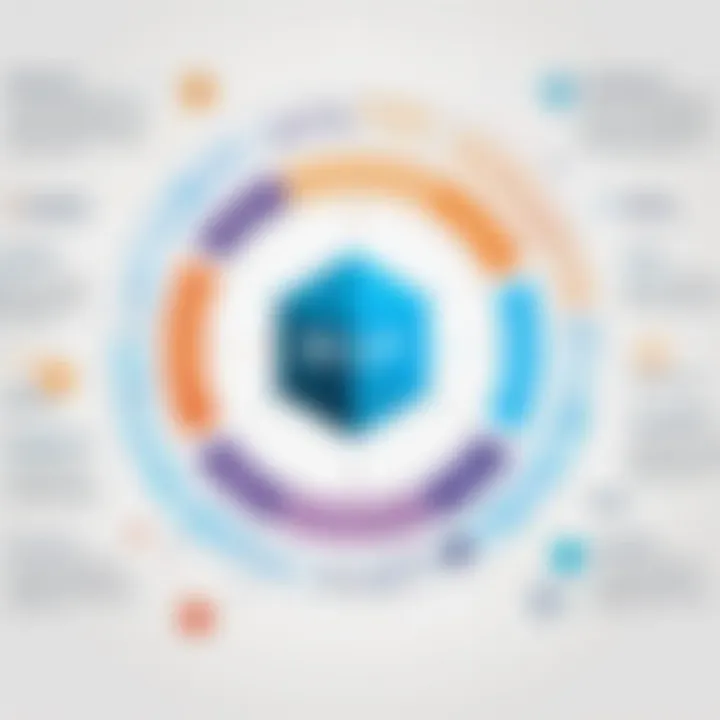
Content creation has become a dynamic field, constantly evolving with advancements in technology. In this landscape, Natural Language Processing (NLP) plays a vital role. It not only automates the generation of text but also enhances the overall creative process for writers and journalists.
One of the standout features of NLP in content creation is its ability to generate news automatically. Automated news generation can keep pace with the rapid flow of information in today's digital world. Media companies can leverage NLP algorithms to produce real-time updates for breaking news stories. This offers several benefits:
- Timeliness: Automated systems can quickly comb through data and generate reports far more swiftly than human writers, ensuring crucial stories are delivered promptly.
- Volume: These systems can handle a high volume of content without the exhaustion and fatigue that humans might experience.
- Consistency: Once programmed, NLP models produce information with a consistent tone and structure, creating a unified brand voice.
However, there are considerations to keep in mind. The challenge of maintaining accuracy and ethical standards remains significant. An algorithm, while efficient, lacks the nuanced understanding of context, tone, and sensitivity that only a human can provide.
Automated News Generation
Automated news generation is a practical use of NLP that empowers media outlets and organizations to diversify their output without significantly ramping up resources. At its core, this involves algorithms that can scan through vast amounts of dataâfrom reports to social media updatesâto find key information that needs to be reported.
Provided that it is implemented with a strategy, automated news generation enables organizations to survive in a landscape where first-to-broadcast often dictates leadership in the news cycle. For example, companies like Bloomberg use NLP to create financial reports. Such a practice can be integral for investors or stakeholders who need quick insights into market conditions or trends.
A major benefit of automated generation is that it can free human writers from the grind of producing routine reports or rehashing existing news updates. This allows them to focus on deeper investigative work or analysis, ultimately enhancing the quality of journalism.
"The ability of AI to generate news stories, though still in its infancy, highlights the ever-blurring line between traditional journalism and technology-driven reportage."
Assisting Writers with Language Tools
NLP doesn't stop at just generating content; it also aids writers in their craft through language tools. By offering suggestions, analyzing text, and providing insights, NLP empowers authors to produce high-quality content. Tools like Grammarly and Hemingway Editor exemplify how NLP can refine writing by suggesting style and grammar improvements.
These tools analyze sentences for clarity, tone, and readability. They help ensure that the final piece resonates with its intended audience without sacrificing individuality or voice. For all writersâwhether seasoned professionals or beginnersâthese assistive technologies can serve as invaluable partners.
Moreover, NLP tools can help authors find inspiration. By analyzing trends and topics within a particular niche, writers can uncover fresh angles to explore that might otherwise go unnoticed. This predictive insight can uplift content creation strategies and tap into audience interests effectively.
In a time where content is king, leveraging NLP in the writing process not only enhances productivity but also drives engagement. As the technology continues to evolve, writers who integrate these tools into their workflow can expect not only to maintain relevancy but thrive in the competitive content landscape.
NLP in Financial Services
The financial sector is no stranger to the rapid growth of technology, and natural language processing (NLP) stands out as a transformative force. With the ability to process vast amounts of text and extract meaningful insights, NLP empowers organizations to make data-driven decisions and improve overall efficiency. As customer interactions become increasingly digitized, the relevance of NLP in financial services cannot be overstated.
By unlocking critical information buried within documents, emails, and social media, NLP aids in multifaceted waysâfrom streamlining operations to enhancing customer service experiences. The efficient handling of languages not only minimizes human error but also helps institutions stay ahead in a competitive landscape. Hereâs a closer look at two pivotal applications that showcase the importance of NLP in this industry.
Fraud Detection Mechanisms
Fraud detection has been a hot topic in the financial world, especially in an age where cyber threats loom large. NLP systems can analyze textual data from various sources, such as transaction records, emails, and customer feedback, to identify patterns indicative of fraudulent activity. For instance, by utilizing anomaly detection methods, NLP can spot unusual phrases or language structures that deviate from the norm, effectively flagging potentially suspicious transactions.
NLP techniques such as supervised learning models and sentiment analysis play a crucial role here. These models can be trained on historical fraud data to recognize characteristics associated with illicit activities. Accumulating insights from various communication forms allows financial institutions to adapt quickly and take preemptive actions.
- Real-time alerts: NLP systems can provide alerts to fraud analysts about suspicious activities, allowing for rapid response and mitigation.
- Cost efficiency: Automating the detection process reduces labor costs and the potential loss from fraud.
- Decision-making support: NLP helps decision-making by presenting data visually, which can aid further analyses.
"Financial institutions investing in NLP for fraud detection could save millions by catching bad actors before they strike."
Market Sentiment Analysis
Understanding market sentiment is vital for financial institutions, as it directly influences investment strategies and forecasting. NLP tools can gauge public sentiment through the analysis of news articles, social media chatter, and earnings reportsâall of which convey valuable information about market trends. By evaluating emotions tied to specific financial instruments or market segments, analysts can derive actionable insights.
Through sentiment analysis, stakeholders can gain clarity on how external factors affect market perception. For example, if a new regulatory framework is introduced, one can track public sentiment around it using NLP. Companies that adapt their strategies based on prevailing sentiments may be better positioned to hedge against potential market swings.
- Trend forecasting: Continuous monitoring of sentiment allows for trend forecasting based on market reactions in real-time.
- Risk assessment: By understanding the sentiment behind significant events, financial institutions can better gauge risks associated with specific investments.
- Competitive edge: Insights drawn from sentiment analyses can provide a competitive advantage when positioning new products or services in the market.
In summary, the utilization of NLP within financial services not only augments traditional methods but also opens doors to innovative strategies, ensuring organizations can navigate the complex landscape effectively.
Legal Applications of NLP
In a world where legal processes can be slow and bureaucratic, NLP brings a scent of modernization. By integrating advanced natural language processing technologies, law firms and legal departments are streamlining their operations, thereby enhancing overall productivity. This section explores how NLP is reshaping the legal landscape, focusing on the vital roles it plays in document review and contract analyses, which stand as cornerstones of legal practice.
Document Review Automation
Document review is a tedious task, one that can keep lawyers burning the midnight oil. These documents are often filled with pages of jargon, lengthy clauses, and minute details that must be scrutinized meticulously. By employing NLP tools, the laborious process of reviewing legal documents is significantly accelerated.
Key benefits include:
- Time Efficiency: Instead of spending hours or even days going through each document, NLP software can scan and flag pertinent sections almost instantaneously.
- Error Reduction: Automation diminishes the likelihood of human error, which can prove costly in legal contexts. Automated systems are more consistent in identifying critical information.
- Cost Savings: Law firms can redirect resources from time-consuming document reviews to more strategic tasks. Clients appreciate this shift, as it translates to lower legal fees.
Some notable tools in this arena include LawGeex and Kira Systems which utilize machine learning algorithms to enrich their processing capabilities. They help extract relevant information from contracts, making the document review phase smoother and more reliable.
"With the help of NLP, lawyers can focus more on strategy rather than sifting through mountains of paper."
Contract Analysis Tools
Contracts are the bread and butter of legal work, intricately drafted documents that define relationships and obligations. A small mistake in understanding or interpreting a contract can lead to grave consequences. Luckily, NLP is enhancing the ease and accuracy of contract analysis through specialized tools.
Noteworthy advantages of employing NLP in contract analysis include:
- Risk Assessment: NLP algorithms can identify potentially risky clauses by analyzing historical data and flagging unusual terms or conditions that deviate from standard agreements.
- Efficient Searches: It allows the quick retrieval of key clauses across multiple contracts, making it easier for lawyers to compile necessary information without hunting through endless files.
- Improved Compliance: By having a more systematic analysis, legal professionals can ensure their contracts adhere to the latest regulations, effectively minimizing legal repercussions.
Popular contract analysis tools such as eBrevia and Luminance are leading the charge in providing advanced solutions for legal professionals handling extensive portfolios of contracts. Their capacity to automate contract summaries and track revisions serves as invaluable resource.
NLP in Marketing Strategies
In today's fast-paced marketing landscape, the integration of Natural Language Processing (NLP) has become indispensable. Companies use NLP to carve out an edge over their competitors, optimizing efforts in understanding consumer behavior and crafting more effective campaigns. Other than just âbuzzword bingo,â NLP in marketing offers real-time insights and automation, helping brands identify what resonates with their target audience. This section dives into two primary aspects of NLP's role in marketing: targeted ad campaign analysis and content optimization techniques.
Targeted Ad Campaign Analysis
Targeted advertising is no longer just about throwing an ad on a platform and hoping for the best. Thanks to NLP, marketers can dig far deeper to understand consumer sentiment and preferences. The advent of algorithmic models enables companies to analyze vast amounts of data, including social media posts and customer reviews, to tailor advertising campaigns that hit home.
NLP can dissect language patterns to reveal the nuances of audience sentiment. By employing sentiment analysis, marketers can gauge not just what customers say, but how they feel about products or brands. This insight is invaluable. Advertisements can be adapted to address specific feelings, whether those are frustrations or joys. For instance, a company selling health supplements can personalize messaging based on sentiment analysis, positioning itself as the 'solution' to the concerns expressed online.
Here are some benefits of using NLP in targeted ad campaign analysis:
- Increased Relevance: Ads become more tailored and directed at specific audiences, enhancing click-through and conversion rates.
- Real-time Adjustments: Marketers can pivot their strategies based on ongoing data analysis instead of relying on pre-set frameworks that might be outdated.
- Cost Efficiency: Fine-tuning ad placements and messaging through analysis helps in better resource allocation, reducing wastage of media spends.
Content Optimization Techniques
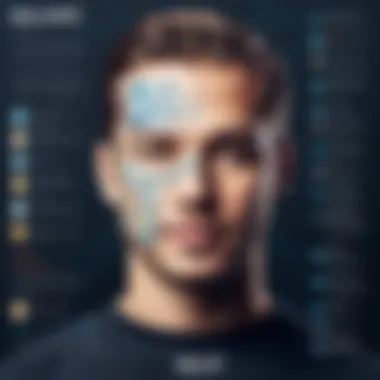
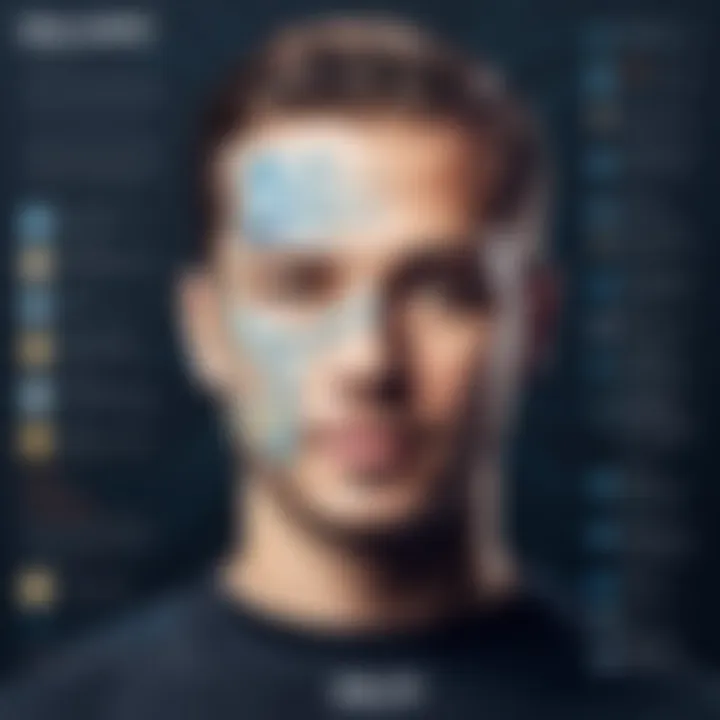
Content is king, but only if it's optimized. With the rise of NLP, optimizing content isn't just about inserting keywords; itâs about understanding what really engages an audience. NLP tools can analyze user interactions and feedback, providing insights into which types of content are best received and why.
For example, using tools like topic modeling and keyword extraction can help in shaping content strategies. By analyzing the language used in popular articles, blogs, or social media, marketers can discover trending topics and phrases that attract engagement. This approach allows them to produce content that is not only well-optimized for search engines but also resonates with readers.
Moreover, NLP enables automated content analysis, ensuring consistency in messaging across different platforms. It can help determine readability scores, sentiment, and even identify potential biases in content. This aids in creating clear, constructive and unbiased messaging that appeals to a wider audience. Here are a few optimization techniques powered by NLP:
- Dynamic Content Personalization: Tailoring content based on individual user preferences improves the user experience and enhances retention rates.
- SEO Enhancements: Using predictive models, marketers can prioritize keywords likely to yield better search visibility.
- Competitor Analysis: NLP can be utilized to ascertain what content performs well for industry peers, providing a basis for strategic planning.
"Incorporating NLP in marketing strategies transforms scattered data into critical insights, allowing companies to engage consumers like never before."
In a nutshell, delving into the applications of NLP in marketing strategies reveals its capability to refine advertising efforts while elevating content. With a structured approach and keen focus on analytics, organizations can not only keep up with the competition but can also set the trends in the industry.
Ethical Considerations in NLP
As the field of Natural Language Processing (NLP) continues to mature, the conversation around its ethical implications has started gaining traction. This is not just for show; it matters deeply. The utilization of NLP technologies in varied industries raises critical questions about data ethics, privacy, and fairness. Examining these ethical dimensions is essential not only for compliance with legal standards but also for fostering trust among users and stakeholders. By addressing these concerns proactively, organizations can leverage NLP while contributing positively to society.
Data Privacy Concerns
Data privacy is rightly at the forefront of any discussion surrounding NLP. When systems absorb and analyze large amounts of textual data, often sourced from user interactions or public platforms, the potential for privacy breaches can become a serious liability.
Firstly, the challenge lies in ensuring that personal information remains confidential. Bots processing customer inquiries, for instance, might inadvertently capture sensitive data embedded in user interactions. If these logs are mishandled or accessed by unauthorized parties, the fallout can be severe. In many jurisdictions, regulations like the GDPR in Europe place stringent obligations on organizations to protect users' data. Failure to comply can result in hefty fines and reputational damage.
Moreover, NLP techniques such as text mining and sentiment analysis often require significant amounts of data to be effective. This creates a dilemma: how do organizations maintain robust data utility while respecting privacy? Following a principle of data minimization, where only necessary information is collected, can provide a pathway. Also, employing methods like differential privacy can enhance user safety by adding noise to datasets, thus obscuring personal data while still allowing for meaningful analysis.
"Navigating the waters of data privacy is like walking a tightrope; it demands balance, care, and foresight."
Addressing Bias in Algorithms
Next up on the ethical checklist is bias in algorithms. Natural Language Processing models learn from vast troves of data, and if that data is skewed, biased, or incomplete, the algorithms will mirror these imperfections. This can lead to troubling applications, where the technology disproportionately benefits or burdens certain groups based on race, gender, or socioeconomic status.
Hidden biases can enter the mix during the data collection phase. For instance, language models trained primarily on online content may reflect the prejudices prevalent in those platforms. This means if communication in user-generated content subverts any group or community, there's a risk NLP systems will adopt that skewed perspective. Not only does this hinder fairness, but it also exacerbates existing inequalities in society.
Thus, it becomes crucial for developers and data scientists to implement strategies that can help mitigate these biases. This includes:
- Diverse Data Sourcing: Actively seeking data that includes various demographics can minimize bias from the get-go.
- Regular Audits: Continuous monitoring of algorithms to identify and rectify any emerging biases.
- Transparent Reporting: Establishing clear communication regarding data sources and model assessments can help build accountability.
In summary, navigating ethical considerations in NLP isn't merely a box to check off; itâs a critical responsibility that requires vigilance, transparency, and a commitment to doing what's right in the face of evolving technology.
Challenges Faced in NLP Implementation
As organizations strive to harness the power of Natural Language Processing, they encounter a maze of complexities which can hinder effective implementation. Acknowledging these challenges becomes pivotal not just for the success of individual projects but also for the broader acceptance of NLP technologies across various sectors. This section sheds light on specific hurdles practitioners face, helping the reader understand the depth of consideration required in deploying NLP solutions.
Limitations of Current Technologies
Current NLP technologies, despite their impressive capabilities, have limitations that often restrict their effectiveness. These limitations often emerge in several key areas:
- Understanding Context: NLP models sometimes fail to grasp the context. While recent advancements have allowed models to analyze vast datasets, their ability to accurately interpret nuanced language remains challenged. For instance, a sarcasm-laden tweet may lead to misinterpretation, affecting sentiment analysis results.
- Multilingual Challenges: Many NLP tools excel in English but falter in other languages. As companies go global, the need for effective multilingual processing becomes essential. The lack of reliable datasets for less commonly spoken languages limits the application of NLP in diverse regions.
- Ambiguity and Complexity: Human language thrives on ambiguity, and resolving these subtle differences can be daunting for machines. Words that have multiple meanings or phrases dependent on regional usage often trip up NLP systems, causing errors in understanding.
"Comprehending the context, nuances, and cultural factors is essential for NLP's evolution and real-world viability"
- Bias in Data: If the training data used to fuel NLP models is biased, the output will also reflect those biases. For instance, training on biased data can mean that the tool may inadvertently perpetuate stereotypes or lead to unfair treatment in applications like hiring tools or loan approvals.
Integration with Existing Systems
Integrating new NLP technologies with existing systems poses another set of challenges. Organizations frequently find themselves in a tug of war, trying to balance innovation with their current operational frameworks. Key integrations issues include:
- Technical Compatibility: Many organizations might have legacy systems that aren't designed to support modern NLP technologies, creating a significant compatibility issue. The ripple effects can also disrupt workflows if the integration isnât handled with careful planning.
- Change Management: Implementing NLP solutions requires change management expertise. Employees are often hesitant to embrace new technologies, fearing job replacement or complicated learning curves. Hence, a systematic approach to managing this change is crucial for successful integration.
- Data Silos: In many organizations, data resides in silos, making it hard for NLP tools to effectively gather and process information from multiple sources. A seamless data flow is crucial for achieving meaningful insights.
- Security Concerns: Finally, any new technology brings along security and privacy concerns. Handling sensitive information with NLP tools necessitates rigorous protocols to ensure data protection, often complicating the implementation process further.
In summary, while the possibilities with NLP are immense, navigating the obstacles posed by technology limitations and integration challenges requires careful thought and planning. Effectively tackling these issues not only enhances the viability of NLP solutions but also reinforces trust within organizations concerning their deployment.
Future Trends in NLP
As industries move quickly into the digital age, the relevance of Natural Language Processing (NLP) canât be overstated. Knowing whatâs on the horizon for NLP is essential for IT professionals and enthusiasts alike. These trends are not just fads; they reflect the growing needs for efficiency, accuracy, and better interaction between systems and humans. Grasping these ideas can help organizations stay ahead of the curve and leverage NLP to its fullest potential.
Advancements in Machine Learning Techniques
Machine learning remains a cornerstone of NLP advancements. With techniques improving by leaps and bounds, the ability to analyze and interpret language is witnessing an upswing that was once thought to be the stuff of dreams. For instance, algorithms have become better equipped to handle intricate subtleties in language, like sarcasm or context-driven meaning.
- Improved Algorithmic Efficiency: New algorithms can process vast datasets more quickly, unlocking valuable insights from heaps of information in minimal time.
- Increased Personalization: By employing advanced algorithms, services can tailor content to individual user preferences, enhancing the overall user experience.
- Robust Data Analysis: These improvements lead to more accurate data analytics, providing businesses with actionable insights that were previously difficult to attain.
As these techniques evolve, the effectiveness of NLP applications will, in turn, become more notable, leading to innovations previously not imaginable.
The Role of Deep Learning in NLP
Deep learning is setting the stage for a new era in NLP. By simulating the workings of the human brain, deep learning can analyze and generate language in ways that simpler models cannot. This technology is helping to enhance several key aspects of NLP.
- Natural Language Understanding (NLU): Advanced models utilize deep learning to achieve superior understanding of context, nuances, and intent behind user queries.
- Natural Language Generation (NLG): With deep learning, generating human-like text has never been easier; this opens doors to automated content creation and tailored customer interactions.
"Deep learning is reshaping how machines understand text comprehensively, building more advanced conversational AI systems that feel human-like in interaction."
- Multilingual Capabilities: Deep learning can also facilitate communication across languages seamlessly, allowing organizations to reach a broader audience.
- Real-time Translation: With continued advancements, real-time translation services have improved, bridging language gaps in a multitude of applications.
The influence of deep learning on NLP is profound and wll certainly pave the way for breakthroughs that could redefine communication as we know it.
Closure
The significance of the conclusion in this article lies in its ability to encapsulate the main themes discussed throughout the exploration of natural language processing (NLP). This section serves not merely as an endnote; rather, it acts as a mirror reflecting the core ideas while providing a cohesive understanding of NLP's diverse applications.
Summarizing Key Insights
NLP has introduced a seismic shift across various industries, fundamentally altering how we interact with technology and data. Key insights extracted from the article include:
- Automation: NLP simplifies tasks, enabling businesses to streamline workflows and reduce human error. Automated chatbots, for example, are not just a gimmick; they're now critical elements in customer service, driving efficiency and improving response times.
- User Interaction Enhancement: Through sentiment analysis and various language models, organizations can understand customer emotions and preferences better. This allows for tailored experiences, leading to increased satisfaction and loyalty.
- Data Transformation: With NLP, vast amounts of unstructured data can be deciphered, allowing companies to identify trends, insights, and actionable strategies that were previously hidden in the noise of raw data.
By encapsulating these insights, the conclusion emphasizes how pivotal NLP is becoming in modern communication and its transformative impact on multiple sectors.
Implications for Future Research
The section on future research implications underscores the ongoing evolution in the field of NLP. As technological advancements continue apace, several areas remain ripe for exploration:
- Enhanced Algorithms: Developing more sophisticated algorithms could vastly improve the ability of machines to understand and generate human-like language, addressing nuances and context more effectively.
- Cross-linguistic NLP: Expanding NLP capabilities to encompass multiple languages and dialects can help ensure technology is inclusive and accessible, accommodating a global audience.
- Accountability in AI: Researching frameworks to make NLP systems more transparent and accountable is essential. Addressing issues such as bias in language models is crucial for ethical usage.