Exploring Certified Artificial Intelligence: Implications & Applications
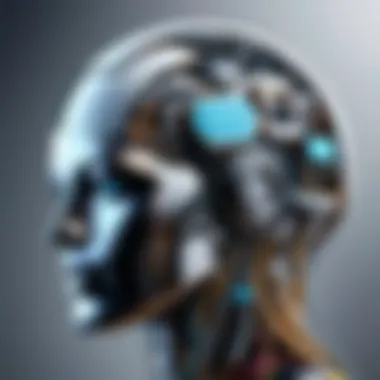
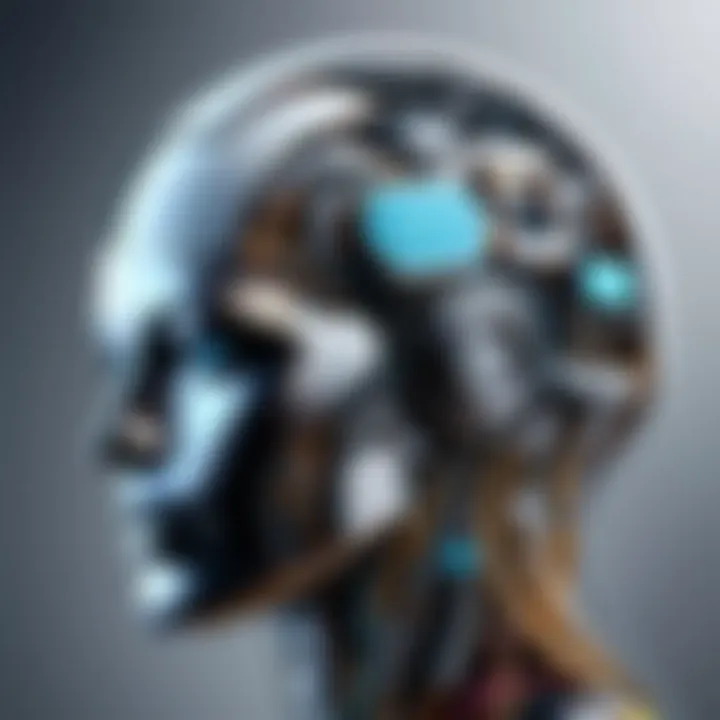
Intro
The intersection of artificial intelligence and certification addresses a pressing need for accountability within advanced computational systems. At its core, certified artificial intelligence ensures both reliability and transparency in algorithms. This is becoming increasingly critical as AI applications proliferate across delicate sectors such as healthcare, finance, and transportation. The article aims to unpack these layers.
Through the collective lens of technological evolution, the exploration will detail certification processes, the varied standards embraced by industries, and the implications of these measures. The analytical depth involved is intended for those entrenched in IT decisions, risk management, or algorithm development. By detailing real-world applications and those end-results, readers align understanding with practical insights.
Moreover, the ongoing evolution within certified AI suggests future trends that warrant attention. The dual emphasis on rooted theory and concrete application contexts provides valuable commingle insight for a discerning audience. Commencing with foundational concepts, the discourse will build towards proficiency in understanding the relevance of these technologies in shaping tomorrow’s AI industry dynamics.
Understanding Storage, Security, or Networking Concepts
Before diving deeper into certified AI systems, understanding the foundational technologies that underpin them is crucial. The quiet harmony among storage, security,text, and networking protocols affects how AI tools operate.
Foreword to the Basics of Storage, Security, or Networking
Storage, security, and networking form the backbone of computing infrastructure. Storage solutions categorize data for extract and utility, while security constrains that access. Networking orchestrates the interactions among devices. Each element represents a spectrum of technologies capable of significant impacts on AI deployment.
Key Terminology and Definitions in the Field
Understanding AI necessitates clear comprehension of key terms:
- Artificial Intelligence (AI): Systems designed to perform tasks typically requiring human intelligence.
- Data storage: Technologies and techniques for preserving information.
- Network: The interconnectedness of devices facilitating data exchange.
- Cybersecurity: Measures and practices for protecting systems from intrusions.
Overview of Important Concepts and Technologies
While the planet entertains complex AI algorithms, understand basic technologies:
- Cloud Storage Solutions like Amazon S3 allow unlimited scaling but often require enhanced security measures.
- Encryption Protocols safeguard data in transit and at rest, assuring privacy among AI conversations.
- API Integration affords traffic between systems, marking resilient back-and-forth data exchanges.
Grasping these foundational pieces is very important for IT professionals venturing into AI framework realities.
The seamless integration of storage, security, and networking becomes a desirable pedigree allowing smooth AI processes.
Let us move on to best practices in securing these elements effectively for reliable AI operations.
Preamble to Certified Artificial Intelligence
In today’s fast-evolving technological landscape, understanding certified artificial intelligence (AI) is becoming paramount. As AI continues to permeate various industries, the significance of certification takes center stage. Certified AI represents a framework ensuring that systems adhere to established standards, promoting safety, reliability, and effective performance. This topic warrants in-depth exploration for multiple reasons. First, certification helps organizations comply with regulations, fostering trust with consumers and stakeholders. Consumers increasingly demand transparency and accountability. With certified AI, users can have more confidence that systems behave as expected.
Another reason for discussing this topic is the role of certification in enhancing reliability. As more AI-driven systems proliferate, maintaining consistency and reliability becomes critical. Certification processes involve rigorous testing and evaluation, assuring users that AI systems function appropriately. This minimizes the risks associated with deploying AI across various applications.
Furthermore, regulatory compliance is critical in today's technological environment. Industries face strict regulations to ensure ethical AI deployment. Understanding certified AI includes implications related to logistics, finance, healthcare, and other vital sectort/her.
Defining Certified Artificial Intelligence
Certified artificial intelligence refers to AI technologies that have undergone formal evaluation and certification by recognized authorities. These authorities set the criteria under which systems are assessed. The certification process validates algorithms and functionalities, demonstrating their compliance with established ethical guidelines and performance standards. This enhances stakeholder trust. Therefore, organizations investing time and resources in certified AI technologies position themselves more favorably within their industries.
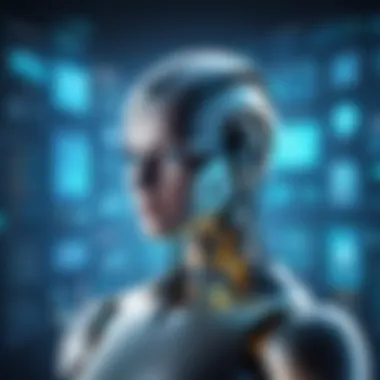
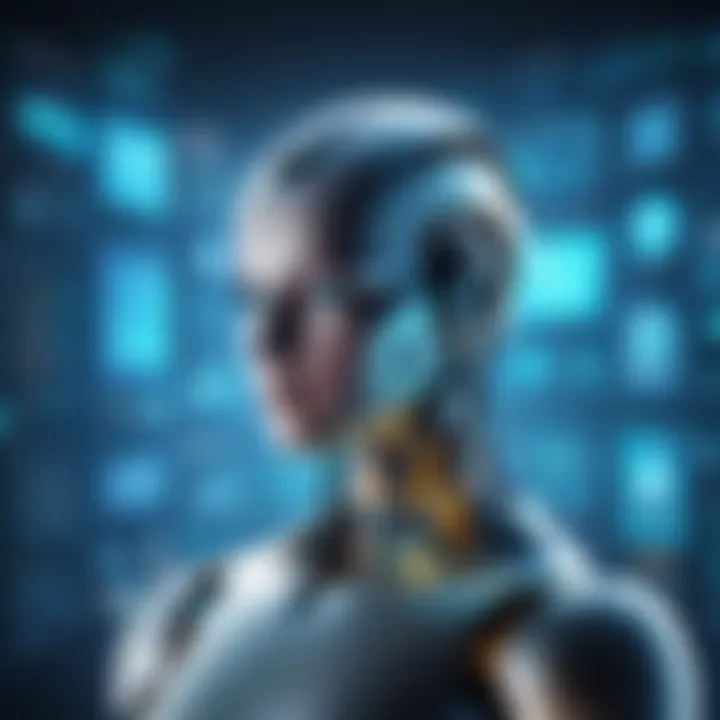
History and Evolution of AI Certification
The concept of certifying AI has evolved significantly since its inception. Early attempts at bestowing some form of validation sought to address safety concerns arising from automated systems. However, with rapid technological advancements, the need and processes for certification began to formalize.
In the early 2000s, a handful of agencies started deliberating on standards; however, changes appeared sluggish. It wasn't until the late 2010s that significant strides were made. Various organizations engaged industry leaders and stakeholders to develop robust frameworks for certifying AI algorithms, tools, and their implementations. Today, recognized entities are working collaboratively to refine criteria further, ensuring continuous improvement within artificial intelligence domains.
The Importance of Certification in AI
AI technologies continue to expand and permeate various sectors. As such, the significance of certification becomes increasingly clear. The importance of certification in AI encompasses three primary aspects. These catagories include building trust with end users, ensuring regulatory compliance, and enhancing the reliability of AI systems. Each of these factors demonstrate how certification can create a safer environment in which AI can thrive.
Building Trust with End Users
In an age marked by rapid technological advancement, establishing trust between AI providers and end users is critical. Certified AI systems provide assurance about the integrity, security, and ethical considerations associated with their use. When organizations can demonstrate they adhere to certification standards, the users feel more confident. This способствует acceptance and generates interest in emerging AI solutions.
Users are often skeptical due to past incidents with unreliability or biases in AI applications. Certification deeply increases transparency, promoting consumer confidence. Often, direct feedback meets persistent data management challenges. Therefore, organisations that invest in certified AI benefits from higher satisfaction rates from their stakeholders. The certification process practically integrates stringent security measures from its inception, fostering protection against breaches and misuse.
Regulatory Compliance and Standards
Another crucial aspect of AI certification is regulatory compliance. Governments and organizations are increasingly striving for effective policies. Using certified AI can help ensure compliance with local and international regulations. Global initiatives necessitate adherence to norms governing privacy, data protection, and AI operability. The lack of compliance could result in legal ramifications or fines that could tarnish reputations.
Companies that seek certification can better align with existing and emerging guidelines. Certified AI systems commonly show their commitment to lawful practices while protecting against exploitation. Moreover, regulators often rely on certification as a tool to benchmark the technology. They use this benchmark to guide evaluations regarding the responsible deployment of AI technologies. In this regard, companies that prioritize certification have enhanced competitiveness over non-certified operatives.
Enhancing AI System Reliability
Reliability remains a major concern within the AI sector. A trustworthy AI system enhances performance metrics. Certification consists of processes that evaluate performance consistency under various circumstances. This procedure assists developers in identifying potential pitfalls through rigorous testing.
Furthermore, certified AI systems create long-term benefits in reliability over time. By undergoing regular assessments, certified technology resonates with end-user expectations. Organizations report improvement in decision-making abilities and prediction accuracies when relying on certified systems. The commitment to lasting performance not only prevents operational chaos but also reduces downtime. As a result, companies investing in certified AI establish a more robust technological foundation moving forward.
Trusted AI systems streamline operations while addressing user doubts through comprehensively adhered methodologies.
Certification Processes for AI Technologies
Certification is fundamental in ensuring that artificial intelligence systems meet defined standards of functionality and safety. It establishes a framework within which AI technologies are trusted by users and applicable across various industries. This section elaborates on the key elements, benefits, and essential considerations related to certification processes.
Key Organizations Involved in Certification
Several organizations play pivotal roles in the certification landscape of AI technologies. Identifying these entities provides clarity on the authority and processes involved. Important bodies include:
- ISO (International Organization for Standardization): Known for developing international standards that influence quality, safety, and efficiency in various sectors, including information technology.
- IEEE (Institute of Electrical and Electronics Engineers): This organization focuses on advancing technology. It has published various standards specifically aimed at ethical considerations and safety in AI.
- NIST (National Institute of Standards and Technology): NIST plays an essential role in AI-related certifications in the U.S., establishing benchmarks and guidelines for performance evaluations.
- ITL (Information Technology Laboratory): This division of NIST offers research and guidance related to IT goals and metrics.
- UL (Underwriters Laboratories): Known for safety testing, UL extends its work to include evaluation and certification of AI products and solutions.
Step-by-Step Certification Process
Navigating the AI certification journey involves several integral steps:
- Preparation and Planning: Understand specific requirements, relevant standards from organizations, and the intended application of the AI technology.
- System Development: Follow documented standards during AI development to ensure compliance.
- Testing Phase: Perform rigorous testing with respect to identified benchmarks, focusing on both performance and safety protocols.
- Documentation: Documentation forms the backbone of the certification process. Clearly outline procedures, test results, and compliance data.
- Submission for Review: Submit documentation to the chosen certifying organization. This may also involve additional testing or evaluation by third-party experts.
- Await Decision: The certifying body will review materials and can request amendments or further test results.
- Receiving Certification: Once approved, the AI technology will receive its certification, formally indicating compliance with established DA standards.
- Ongoing Compliance: Regular audits or assessments may be required to maintain certification amidst evolving technologies.
Common Certifications Available
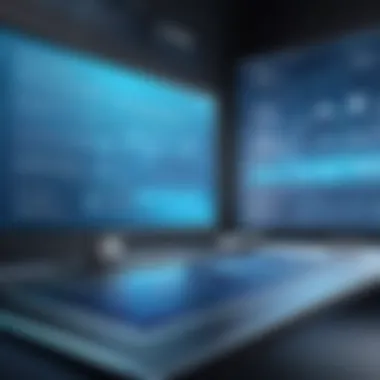
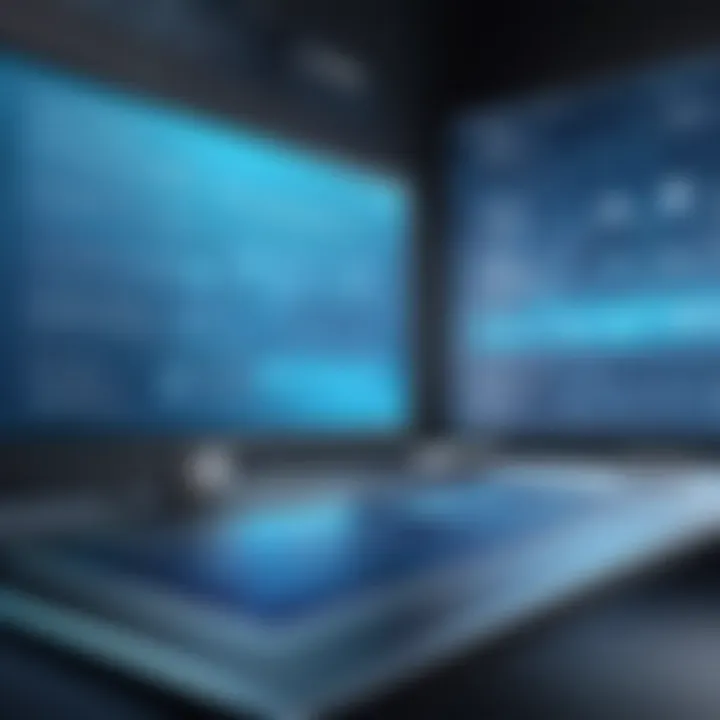
AI technologies can pursue various certification options. Relevant certifications include:
- ISO/IEC 27001: Focused on information security management, this standard assures users that AI systems protect sensitive data.
- IEEE 7000 Standard: Concentrating on ethical considerations in AI, it encourages accountable designs.
- NIST Cybersecurity Framework: While not unique to AI, this framework assists in certifying cybersecurity integration, especially in systems involving data-driven choices.
- UL 2900-1: A standard guiding cybersecurity assurance for software, applicable to AI-driven applications.
Certification solidifies stakeholders’ trust. It represents not just a checklist but an ongoing commitment against failures.
In summary, the certification processes for AI technologies represent a vital interaction between compliance, trust, and the fundamental necessity to adapt to daily challenges. Understanding these processes lays the groundwork for successful implementation and adherence to existing regulations. The certification steps, organizational roles, and various available certifications form a cohesive framework leading to innovation and reliability in AI technologies.
Applications of Certified AI in Various Industries
Certified Artificial Intelligence (AI) has become integral in various industries, providing substantial benefits in efficiency and reliability. Applying certified AI systems ensures compliance with established standards, fostering confidence among end-users and organizations alike. The utilization of certified AI addresses specific needs in different sectors, enhancing service delivery and operational effectiveness.
Healthcare: Improving Diagnosis and Patient Care
The healthcare industry is rapidly adopting certified AI to elevate patient diagnosis and care standards. Systems powered by certified AI assist medical professionals in diagnosing diseases more accurately and swiftly. For example, image recognition technologies can analyze medical images, flagging potential issues like tumors that may be missed by the human eye.
Furthermore, AI-enabled chatbots can streamline patient communication, reducing wait times in hospitals. The reliability of certified AI in providing accurate diagnoses not only improves patient outcomes but also optimizes hospital operations. As such, integrating certified AI enhances both patient trust and overall healthcare effectiveness.
Finance: Risk Management and Fraud Detection
The financial sector leverages certified AI for risk assessment and fraud detection purposes. By analyzing vast amounts of transaction data, certified AI tools help organizations predict potential risks more effectively. For instance, machine learning algorithms can identify unusual patterns in spending behavior, thus flagging potential fraudulent activities.
Utilization of certified AI in finance reduces operational risks by ensuring that predictive models comply with stringent regulatory standards. Moreover, financial institutions that deploy certified AI foster trust with clients, demonstrating their commitment to safeguarding sensitive data.
Manufacturing: Streamlining Operations and Safety
In the realm of manufacturing, certified AI contributes to operational efficiency, safety, and product quality. Advanced AI systems analyze production data to identify areas of inefficiency, making it easier to minimize waste and reduce costs. Predictive maintenance powered by certified AI keeps machinery in optimal condition, thus decreasing downtime and enhancing safety standards.
Moreover, certified AI can assist in quality control processes by consistently monitoring product specifications and detecting deviations from planned targets. Integrating certified AI solutions leads not only to better production outcomes but also to satisfying consumers and adhering to safety regulations.
Transport and Logistics: Enhancing Efficiency and Safety
The transport and logistics industry increasingly relies on certified AI to improve efficiency and security in operations. Certified AI technologies power smart routing systems, ensuring that deliveries reach their destination faster and with fewer resources. This not only reduces costs but also lessens environmental impact, aligning with sustainability goals.
Additionally, AI can optimize inventory management within warehouses. An accurate analysis of stock levels supports better decision-making processes and minimizes wasted resources. Reliable certified AI systems assure stakeholders that transportation and logistics operations are compliant with relevant regulations, enhancing community trust.
With these applications, certified AI not only revolutionizes various industries but also paves the way for future advancements. Through continual integration, organizations can achieve heightened levels of performance and dependability.
Challenges in Obtaining Certification
In the rapidly accelerating landscape of artificial intelligence, certification represents both a substantial benchmark and a significant hurdle. As organizations strive to bring their AI systems up to certified standards, they encounter a multilayered web of challenges. Understanding these obstacles is crucial for comprehending the broader impact of certified AI within the technological spectrum.
Technical Barriers to Implementing Standards
Technical barriers often emerge as the foremost challenges in the certification of AI systems. One significant factor involves interoperability among various AI technologies. Additionally, insufficient or unclear guidelines can lead to disparities in how certification standards are interpreted and applied.
Organizations may differ in their computational architectures, coding languages, and algorithms. This variability hinders a coherent approach toward a uniform certification policy. Addressing these aspects often requires considerable unresolved debate among tech leaders and regulators to establish acceptable use cases. Leading to delays in implementation, a collaboratively defined set of standards would enable a smoother certification landscape. More consistent technical parameters would benefit developers, fostering an environment of innovation when working toward certification.
Costs Associated with Certification
The financial implications of pursuing certification can be daunting. These costs may include operational expenses, consultation fees, and technological upgrades necessary for compliance. Moreover, maintaining adherence to evolving standards can lead to ongoing expenditures that stretch resources for organizations, especially smaller firms.
Considering these costs, frivolous spending might occur when organizations invest resources without aligning their technology roadmap to specific certification requirements. This misalignment adds layers of complexity and can engender disillusionment towards the certification process. Budgeting appropriately, with thorough research on certification types suitable for an organization’s needs, is essential in offsetting these costs significantly.
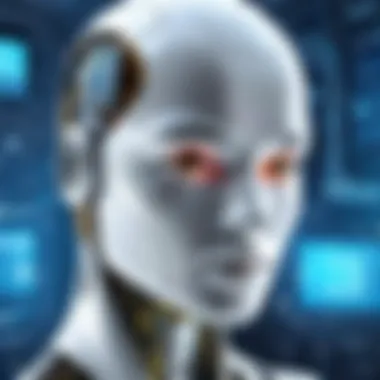
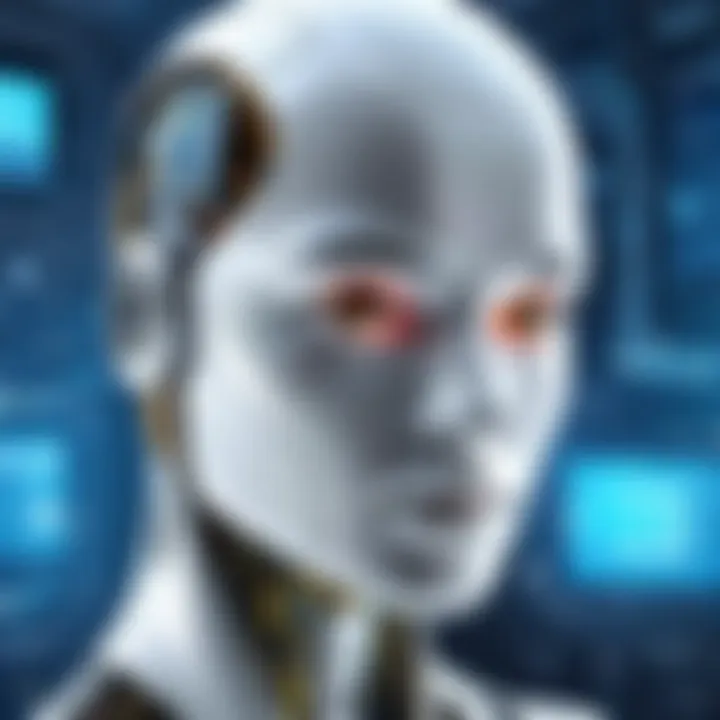
Evolving Nature of AI Technologies
As artificial intelligence technologies continually advance, they present another level of challenges in the certification journey. This rapid evolution often outpaces existing standards, rendering them obsolete almost as soon as they are established. New algorithms, AI methodologies, and frameworks rapidly develop, introducing trends that challenge previous norms.
Consequently, certifying bodies often struggle to keep pace with innovations while drafting regulations that provide due guidance. Not maintaining equilibrium can lead to under-regulation of critical areas such as data privacy and ethical dilemmas linked to AI deployment. Certifying bodies need to constantly update their criteria. This demands an agile approach toward standards development to support responsible AI growth while ensuring this technology can still deliver on reliability and trust.
The journey toward certification in AI is shadowed by a landscape that is itself marked by rapid transformation and uncertainty. Maintaining relevance in the face of technology's unyielding evolution is one of the major challenges.
The hurdles concerning technical implementation, financial investments, and the pace of technological change all pose real threats to the goal of certified AI. Understanding and analyzing these challenges arms stakeholders with the knowledge necessary to navigate the labyrinth of certification more effectively.
Future Trends in Certified AI
Future trends in certified artificial intelligence represent a pivotal juncture for both developers and users alike. As technology continues to march forward at a relentless pace, certified AI is increasingly essential in ensuring systems can be trusted, regulated, and operate seamlessly with unique demands across different sectors. Highlighting the developments in integration, standardization, and the imminent requirements fosters an understanding that is invaluable to all involved in the technology landscape.
Integration with Emerging Technologies
The integration of certified AI with emerging technologies is likely to reshape the contours of industries significantly. As we find ourselves in an age dominated by big data, Internet of Things (IoT), and blockchain technology, coupling these innovations with certified AI can enhance their applicability and reliability. For example, combining AI with blockchain ensures the integrity of datasets by making them tamper-proof. This collaboration permits trust in data fed to AI systems, supporting the results these systems generate.
Several elements come into play here:
- Improved data quality: Certified AI aids in assessing and verifying the sources and accuracy of data generated through IoT.
- Enhanced analytics: Advanced AI algorithms can provide deeper insights when paired with extensive datasets from multiple emerging technologies.
- Standardized frameworks: As interfaces are standardized, the interoperability between various technologies turns more feasible.
Global Standards and Harmonization Efforts
The conversation surrounding certified AI cannot ignore the need for global standards and making sense of varied frameworks across borders. Certification processes can profoundly affect an AI system's reach.
Currently, numerous organizations aim to shell out numerous protocols from different regions, bringing inconsistency into certifications. Initiatives like ISO/IEC JTC 1/SC 42, focusing on artificial intelligence, seek to streamline standards emerging globally. Consistent efforts toward harmonization result in substantial benefits, such as:
- Trust enhancement: Users are more likely to accept AI systems if they meet internationally recognized standards.
- Facilitated market access: Businesses can effectively and rapidly navigate foreign markets with established certification norms globally.
- Better compliance: Standardized regulations tend to simplify the adoption of laws while preventing companies from misguiding stakeholders.
Predictions for the Evolution of Certification
Gazing into the future, the evolution of certification processes for AI appears to increasingly accommodate the rapid technological advancements inherent in the system. Predictions indicate major shifts in several aspects:
- Adaptive regulatory frameworks: Certification processes will likely become more dynamic, evolving alongside AI as systems change, ensuring that they remain adequate.
- Technological-driven benchmarks: New benchmarks, specific to industries and technologies, will form part of the certification to suit different operational contexts, moving beyond conventional testing methods.
- Emphasis on ethical standards: There is probable focus on aligning certification practices with ethical AI considerations, ensuring AI-generated decisions do not inadvertently favor one group over another. This trend accounts for the rising demand for fairness and transparency in AI.
As developments unfurl around certified AI, adapting to identify and prepare for these transformations lays groundwork for all officers involved. Amid developing technologies, clarifying standardized benchmarks sparks effective dialogue among academia, industry, and the public to leverage AI toward newer horizons.
Culmination
The conclusion of this article synthesizes the main points regarding certified artificial intelligence. It emphasizes the relevance of certification about innovation, regulatory compliance, and user assurance.
Summarizing Key Takeaways
- Importance of Certification: Certification fosters trust between users and AI technologies, reinforcing the value of performance metrics and transparency.
- Impact Across Industries: Certified AI solutions have proven indispensable in sectors like healthcare, finance, and manufacturing. Their application aids in improving efficiency and decision-making processes.
- Challenges Remain: Issues such as cost barriers and technical constraints are still prevalent. Understanding these hurdles is necessary for enhancing the adoption of certified AI.
- Future Preparedness: Experts must stay tuned for evolving standards that keep pace with technology advancements. Synthetic solutions require ongoing vigilance and refinement.
The Future Landscape of Certified AI
Looking forward, the trajectory of certified artificial intelligence will be shaped by several factors:
- Emerging Technologies: The integration of AI with quantum computing and blockchain is on the horizon and will redefine Ay certification standards.
- Harmonization of Global Standards: As technology becomes more global, creating a unified set of standards will be critical. Global cooperation can establish more comprehensive compliance and functionalities.
- Adaptation to Change: The fast-paced nature of AI development means that certification standards must adapt continuously. Proactive measures in certification processes will be necessary to tackle new advances effectively.
In summary, an emphasis on certified AI will innovate sectors while ensuring rigorous standards enshrine trust and reliability. As practitioners of these technologies, it becomes essential to advocate for both improvement and adoption of certification across all frontiers.