Exploring the Essentials of Data Quality Engineering
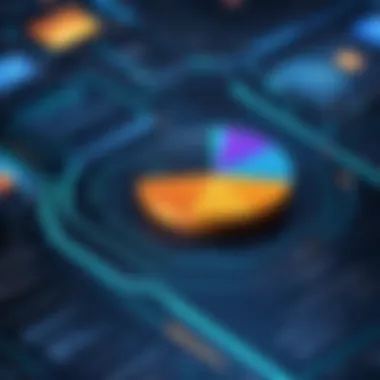
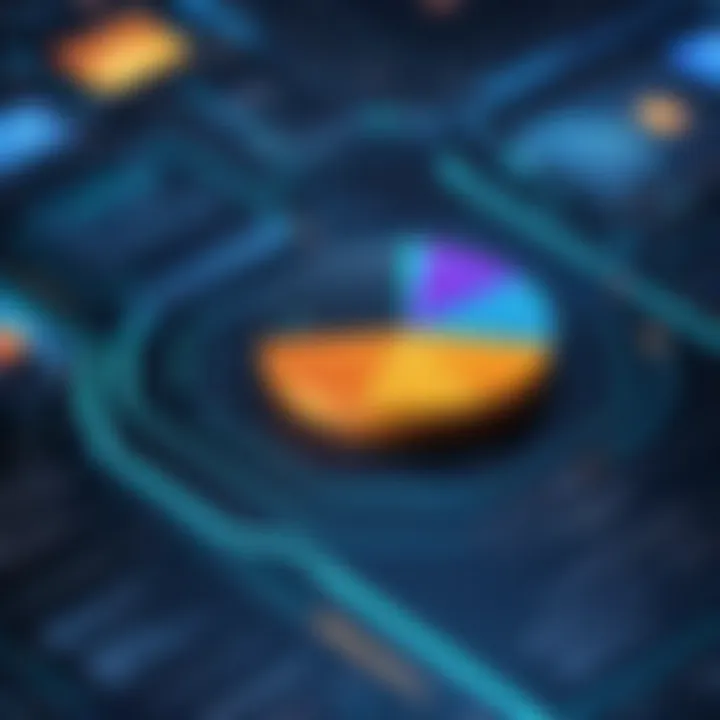
Intro
Data quality engineering isnât just a buzzword in todayâs data-centric world; itâs a linchpin for success in various sectors. As organizations lean increasingly on data to inform their decisions, ensuring that this data is accurate, consistent, and reliable cannot be overstated. When data quality falters, companies might find themselves making choices based on flawed information, leading to misguided strategies and potential losses.
In this article, weâll dissect the core principles of data quality engineering. Weâll also spotlight essential methodologies and tools that anchor these principles while candidly exploring the hurdles that professionals face in maintaining data integrity.
Understand the Basics of Data Quality Engineering
Before diving deep, letâs take a step back and outline what data quality really means. At its heart, it deals with verifying, validating, and refining data to meet the needs of its users. Think of it like ensuring youâve got the right ingredients in your pantry before whipping up that five-star meal.
Here are some core benchmarks that define solid data quality:
- Accuracy: Data must correctly reflect the real-world conditions it represents.
- Consistency: The information should remain stable across various databases.
- Completeness: No important chunks of data should be missing.
- Timeliness: Data should be up-to-date and relevant.
- Relevance: The data must serve its purpose in context.
Importance of Data Quality Engineering
Why should IT professionals and businesses care? Flawed data can lead to poor business decisions. When looking back at historical data, organizations may miss trends or misinterpret patterns because of mere inaccuracies. Imagine relying on data that shows declining sales, only to discover later it was due to a known error in reporting. The fallout from such missteps can be costly, both financially and in terms of reputation.
Letâs be frank here: in an era where data is often considered the new oil, treating it like gold seems only fitting. When done right, data quality engineering streamlines operations, improves decision-making, and even fosters trust among stakeholders.
Best Practices and Methodologies
To fortify data quality, organizations can adopt certain practices and methodologies that sharpen their focus. Itâs like tightening screws on a well-built machine to enhance performance:
- Data Profiling: Regularly analyze existing data to assess its quality level.
- Data Cleaning: Employ techniques to identify and rectify errors within datasets.
- Standardization: Implement uniform formats and standards across all data inputs.
- Automated Quality Assurance: Use software tools designed for constant monitoring of data quality.
These methodologies, when merged thoughtfully, burgeon into a robust data quality framework.
Industry Challenges
It's not all smooth sailing, though. Maintaining data integrity often collides with various challenges. Having multiple data sources can create discrepancies, and manual data entry remains a breeding ground for errors. Moreover, adapting to evolving regulations around data protection, like the GDPR, adds another layer of complexity.
The balanceâbetween innovating data-driven strategies and ensuring qualityârequires ongoing attention.
"Quality is not an act, it is a habit." â Aristotle
Epilogue
As we navigate through this unfolding narrative of data quality engineering, remember this: solid data quality is not merely about avoiding pitfalls; it's about positioning yourself at the forefront of decision-making capabilities. By understanding and applying the principles of data quality engineering, IT professionals and business leaders can elevate their data management strategies, driving better outcomes in their organizations.
The Essence of Data Quality Engineering
Data quality engineering sits at the crossroads where information integrity meets operational efficiency. In todayâs data-driven landscape, the validity of insights hinges on the quality of the underlying data. In essence, data quality is not just an afterthought; itâs a crucial element of any analytical process.
Defining Data Quality
When we talk about data quality, weâre not merely discussing cleanliness or organization. Instead, weâre diving into a multi-faceted concept that encompasses several dimensions. Accuracy, consistency, completeness, and timeliness are just a few key aspects that come into play.
- Accuracy refers to how closely data reflects the real-world constructs it represents. For example, if a company's sales data shows that 200 units were sold but the actual figure is only 150, that data lacks accuracy.
- Completeness implies that all required information is present. An incomplete address in a customer database can lead to missed sales opportunities and dissatisfied clients.
- Timeliness conveys the relevance of data within its necessary timeframes. If data is outdated, it may lose its value in decision-making contexts.
Understanding these dimensions helps not just in assessing data quality but also in crafting strategies for its enhancement.
The Role of Data Quality Engineering
The role of data quality engineering canât be understated; it serves as a bedrock for successful data operations. This discipline focuses on establishing frameworks and methodologies that ensure data maintains its quality throughout its lifecycle.
By integrating quality checks into every stageâdata collection, processing, analysis, and reportingâdata quality engineering ensures that issues are identified early and rectified efficiently.
In practical terms, this might look like:
- Implementing automated data profiling techniques that sift through databases to flag inconsistencies.
- Developing standards and protocols for data entry to ensure everyone within the organization adheres to the same guidelines.
- Enabling regular audits that promote continual assessment and validation of data.
Ultimately, a robust approach to data quality engineering can save organizations time, resources, and reputational damage. By prioritizing data integrity from the outset, companies can leverage their data assets more effectively, driving smarter decisions and fostering trust in their analytical outputs.
"Data quality engineering is not merely a technical challenge; itâs a strategic imperative that influences the entire organizational ecosystem."
In summary, the essence of data quality engineering isnât simply focused on maintaining standards within datasets. It's about embedding a culture of quality that resonates throughout an organization, fostering a mindset where every decision-makers consider the importance of reliable data.
Key Principles of Data Quality Engineering
In the landscape of data management, the concept of data quality engineering stands as a cornerstone of effective strategies. Understanding the key principles is essential not just for data professionals, but also for those keen on advancing their organizational objectives through accurate and reliable data. These principles guide the methodologies and tools employed to assess data integrity, and without them, organizations risk operating on faulty data, which can lead to misguided decisions.
From the onset, it's important to recognize tht data quality is not a one-time affair but rather an ongoing commitment. The three main principlesâaccuracy and precision, consistency and reliability, and completeness and timelinessâform a triad that ensures data serves its purpose well. Let's dig deeper into these principles.
Accuracy and Precision
In the realm of data quality engineering, accuracy and precision often get tangled up, like two peas in a pod, but they hold distinct meanings. Accuracy denotes how close the data is to the true value. For instance, if a company's sales data reports an income of $10,000 but the true figure is $12,000, then that data is not accurate. On the other hand, precision measures the degree of variation within a dataset. If multiple entries of sales data show repeats of $10,000, itâs precise but not necessarily accurate if the true figure is different.
Organizations that prioritize accuracy and precision find themselves better equipped for decision-making. A healthcare institution, for instance, relies on patient data accuracy to provide the correct treatment. If it's not precise, it could lead to severe repercussions, or worse, misdiagnoses.
"Data alone is not the answer; it is accurate data that leads to informed decisions."
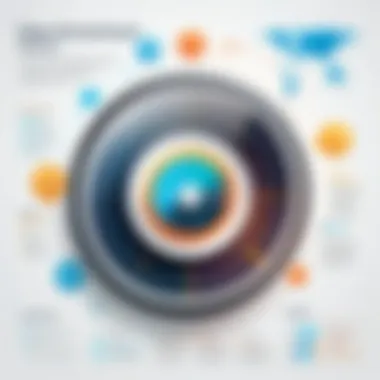
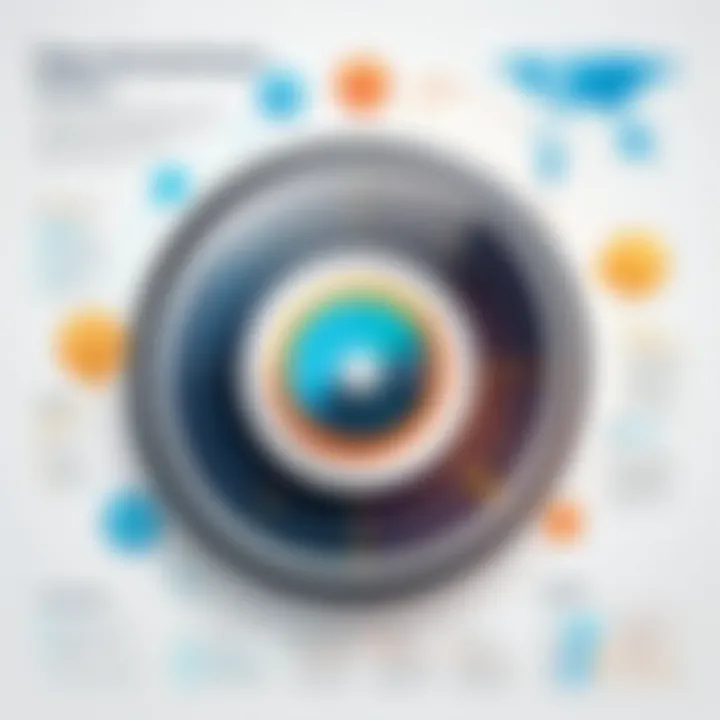
Consistency and Reliability
Letâs face it, data can sometimes be as unpredictable as the weather. Consistency comes into play to address this very issue. It means that over time, across various datasets, data remains uniform. Reliability ensures that the data consistently produces the same results under the same circumstances. For instance, if you're analyzing customer preferences over different periods and find that the results change drastically without any logical reason, that's a red flag.
Imagine a widget factory that tracks production metrics. If a report shows varying output numbers from the same machines in similar conditions, that inconsistency could lead to chaos within operational efficiency. Maintaining consistency ensures that every stakeholder draws from the same well of information.
Completeness and Timeliness
Finally, we arrive at completeness and timeliness, which are like bread and butter in the world of data. Completeness is about having all necessary data points available for analysis. Itâs fundamentally about filling in the gaps. Timeliness, meanwhile, gauges whether the data is up-to-date and relevant to current scenarios. A classic example is a marketing campaignâif the data on consumer trends is outdated, the campaign might miss the mark by a mile.
The two concepts complement one another. For example, if a business database lacks data entries about recent product launches, it may hinder the effectiveness of its customer outreach efforts. Thus, having a complete and timely dataset not only enriches the quality of analysis but also contributes significantly to more rapid and relevant insights.
In summary, the key principles of data quality engineering serve as essential benchmarks. They establish a clear framework for assessing and enhancing data integrity while ensuring that businesses remain agile and informed in their decision-making processes. By honing in on accuracy and precision, consistency and reliability, along with completeness and timeliness, organizations are better positioned to navigate the complexities of todayâs data-driven landscapes.
The Data Quality Lifecycle
The Data Quality Lifecycle is a crucial component in managing the quality of data at every stage of its life, from inception to disposal. This lifecycle typically encompasses four key stages: data collection, data processing, data analysis, and data reporting. Each phase has its own set of challenges and opportunities, driving organizations to focus on meticulous data management practices.
Fostering awareness about this lifecycle is key because a misstep in any stage can ripple through the finalized data products, undermining the integrity of critical business decisions. The importance of data quality cannot be overstatedâit shapes the very foundation for informed decision-making and enhances operational efficiency.
Hereâs why each step matters:
- Ensures relevant and accurate information is captured.
- Allows for verification and validation to catch errors early.
- Empowers stakeholders to rely on data for insights.
- Provides a framework for audits and compliance.
"Data is a precious thing and will last longer than the systems themselves." - Tim Berners-Lee
Data Collection
Data collection is often the first hurdle in the data quality lifecycle, where the robustness of gathered information sets the stage for everything that follows. Effective data collection practices involve thoughtful design of surveys, databases, and data entry processes. This phase is where organizations must prioritize the capturing of accurate and relevant information.
Considerations in data collection include:
- Source Credibility: Always evaluate the trustworthiness of data sources. Whether it's internal databases or third-party platforms, a rigorous vetting process pays off.
- Methodology: Choose between qualitative and quantitative methods based on the intended use of the data. Both have their pros and cons in capturing different aspects of reality.
- Standardization: Implement standard coding protocols or tagging systems to reduce inconsistencies across datasets.
When data collection is done right, it significantly reduces the noise that can pollute subsequent stages of the lifecycle.
Data Processing
Once data has been collected, the next phaseâdata processingâtransforms that raw data into a usable format. This step involves cleaning, transforming, and storing data in standard formats. Given the complexities involved, it's essential to establish clear protocols to ensure data remains both accessible and consistent.
In the data processing stage, important factors to consider include:
- Data Cleaning: Removing duplicates, correcting inaccuracies, and managing missing data are key actions taken during this phase.
- Transformation: Applying functions to convert data into required formats enhances usability. This might include normalizing values or aggregating data from various sources.
- Storage Solutions: Choosing the right storage solution, whether cloud-based or on-premises, can influence how quickly data can be accessed and processed later on.
A well-organized processing system sets the groundwork for achieving meaningful analysis in the next step.
Data Analysis
Data analysis is the stage where the magic happens. Here, insights are drawn from cleaned and organized data. IT professionals and analysts utilize various statistical techniques and tools to decipher patterns, trends, and relationships.
This aspect of the cycle bears significance because it directly impacts decision-making. The importance of data visualization cannot be neglected; it allows complex data interpretations to be comprehensible for stakeholders. In this stage, practitioners should take into account:
- Statistical Methods: Knowing which method to apply can yield the most pertinent insights. Options include regression analysis, clustering, and hypothesis testing.
- Visualization Tools: Utilize dashboards and tools like Tableau or Power BI to present data in an easily digestible manner.
- Collaborative Input: Engaging with team members from different domains can widen perspectives and facilitate better interpretation of results.
Well-executed analysis expands the organizationâs ability to operate from a position of intelligence.
Data Reporting
Lastly, data reporting wraps up the lifecycle by creating a narrative around the findings from data analysis. Reports must convey not only the data insights but also their implications for the business. The essence of good reporting lies in clarity and targeted communication.
Key aspects to keep in mind during this last stage include:
- Clear Communication: Structure reports to separate key findings from supporting data, ensuring the main points stand out.
- Relevance: Tailor reports to the audience, whether they are technical departments, executive teams, or external stakeholders, to optimize impact.
- Feedback Loop: Reports should invite feedback to refine future endeavors, integrating continuous improvement into the lifecycle.
In summary, the Data Quality Lifecycle isn't just a sequence of stages; it is an interconnected framework that demands a holistic approach to ensure data quality across the board.
Methodologies in Data Quality Engineering
Data quality engineering encompasses a range of vital methodologies that serve to enhance the integrity, consistency, and accuracy of data throughout its lifecycle. Knowing the methodologies is pivotal for IT professionals and cybersecurity experts, as these strategies help navigate the complex landscape of data management. Each methodology offers distinctive benefits, considerations, and tools which can be adapted to the specific needs of an organization. The importance of these methodologies cannot be overstated; without them, data runs the risk of becoming unreliable and inaccurate, leading to poor business decisions.
Understanding these methodologies allows stakeholders to pinpoint specific weaknesses in their data processes and establish a roadmap for robust improvements. A strong foundation in these areas promotes a culture that values data integrity, which is increasingly essential in todayâs data-driven world.
Data Profiling Techniques
Data profiling is the first line of defense in assessing data quality. It involves examining data from various sources to identify patterns, inconsistencies, and anomalies. By employing a suite of tools and techniques, IT professionals can create a comprehensive picture of the existing data landscape, which informs both data cleansing and enrichment activities.
Key aspects of data profiling include:
- Structure analysis: Understanding data types and formats, which highlights inconsistencies.
- Content examination: Examining values to find outliers or unusual entries, potentially revealing deeper issues in data collection or entry.
- Relationship discovery: Analyzing how different datasets interrelate can surface hidden connections and enhance the insights drawn from data.
By profiling data before any other processes, companies can prevent downstream errors that would otherwise be costly to fix. In the end, good data profiling lays the groundwork for more effective data management.
Data Cleansing Approaches
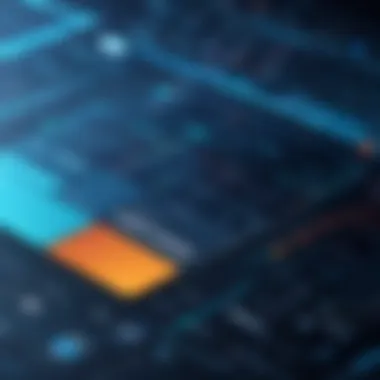
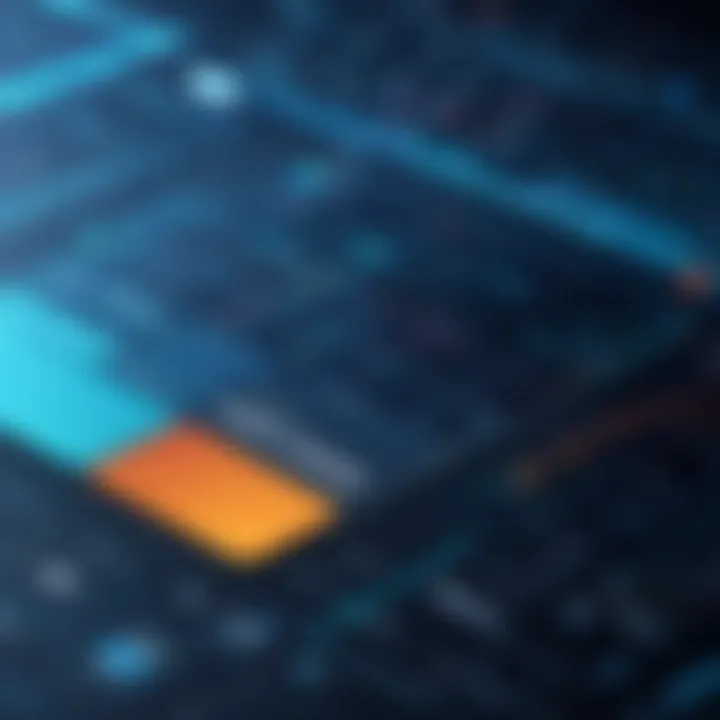
Data cleansing is an essential methodology for ensuring that data remains reliable and accurate. Often seen as a labor-intensive task, itâs fundamental to the maintenance of high-quality datasets. Various approaches exist for data cleansing, each with its strengths.
Common data cleansing methods include:
- Standardization: Harmonizing data formats across large datasets, ensuring that information is consistent and comparable.
- Deduplication: Identifying and removing duplicate records, thus improving database efficiency and accuracy.
- Error correction: This involves flagging, reviewing, and correcting errors identified during data profiling.
The benefits of a structured data cleansing approach cannot be understated. It not only improves the accuracy of reports but also enhances trust in the analytics derived from the data. Moreover, organizations that invest in cleansing methodologies often find enhanced compliance with regulations concerning data integrity.
Data Enrichment Strategies
Data enrichment adds value to existing datasets, making them more useful and actionable. It fills gaps, improves context, and corrects biases by integrating external data sources with the internal database. This practice is crucial in creating a holistic view of the information that an organization holds.
Some effective data enrichment strategies are:
- Third-party data integration: Leveraging external databases such as demographic information or market trends can enhance the utility of existing datasets significantly.
- Real-time data updates: Implementing continuous data updates ensures that the information remains timely, addressing any issues of obsolescence.
- Feedback loops: Using customer feedback to refine datasets, thus ensuring that the data aligns with real-world dynamics.
Incorporating effective data enrichment strategies can radically change how data is perceived and used within an organization. It becomes not just a repository of numbers, but a critical resource for decision-making.
âEnhancing data quality is not just about fixing errorsâitâs about enabling informed decisions.â
In summary, methodologies in data quality engineering serve as the backbone of a firmâs data strategies. Each technique, from profiling and cleansing to enrichment, contributes significantly to maintaining a high standard of data quality. Embracing these methodologies will empower organizations to navigate their data landscapes with confidence and precision.
Tools for Data Quality Management
Data quality management is vital for maintaining cohesive, trustworthy data systems. Utilizing the right tools enhances efficiency, clarity, and reliability at every stage of the data lifecycle. Without appropriate tools, organizations may find themselves wading through errors, misinterpretations, and ultimately poor decision-making.
Importance of Tools in Data Quality Management
The significance of tools in data quality management cannot be overstated. They provide a backbone, supporting the entire framework that ensures data integrity and usability. Integrating these tools not only improves accuracy but streamlines processes, ultimately fostering a data-driven culture within businesses.
Key Elements of Data Quality Tools:
- Automation: Tools automate repetitive tasks, reducing human error and increasing operational speed.
- Scalability: As data grows, tools that easily scale with business needs can handle larger volumes without sacrificing quality.
- Integration: Effective tools can seamlessly integrate with existing systems, promoting a unified data environment.
Benefits of Implementing Quality Management Tools
- Increased Efficiency: Processes that once took hours can often be completed in minutes once tools are properly utilized.
- Cost Reduction: Quality tools eliminate unnecessary data cleansing costs, which can spiral out of control if manual processes are relied upon.
- Enhanced Decision-Making: Higher quality data leads to better-informed decisions, reducing risks and improving outcomes.
Considerations Regarding Tools
Before implementing any data quality tools, it is essential to consider:
- Organizational Needs: Assess what challenges the organization faces and ensure that selected tools align with specific needs.
- User Experience: Tools should be user-friendly and not require excessive training or resources to deploy.
- Support and Updates: Ensure that the tool provider offers adequate support and regularly updates the software to keep pace with changing data environments.
"The efficiency of data management tools lies not just in their features but in how well they align with business objectives."
Automated Data Quality Tools
Automated data quality tools play a crucial role in modern data ecosystems. They perform tasks such as data profiling, cleansing, and validation without requiring continuous human intervention. By employing these tools, businesses can achieve higher accuracy and consistency while freeing up team members to focus on more strategic tasks.
Features of Automated Data Quality Tools:
- Real-Time Monitoring: Quickly identify data quality issues as they arise.
- Batch Processing: Handle large data sets swiftly, making it easier to maintain updated data quality metrics.
- Custom Alerts: Set parameters on what constitutes a quality issue, receiving immediate notification when thresholds are breached.
Data Quality Dashboards
Data quality dashboards are indispensable for visualizing and reporting data quality metrics. They consolidate crucial data points into an easily digestible format that aids teams in understanding data health at a glance.
Advantages of Utilizing Dashboards:
- Quick Insights: Dashboards present metrics that reveal trends and anomalies, allowing rapid response.
- Customizable Views: Users can tailor dashboards to display the most relevant data to their roles, improving engagement and decision-making.
- Collaborative Features: Teams can share real-time insights and metrics, enhancing collaboration across departments.
Data Validation Frameworks
Data validation frameworks are essential for ensuring the integrity of data before it is utilized for decision-making. These frameworks implement enforced rules that data must meet to be considered valid. They can automate checks, enhancing the quality and reliability of data used in reporting and analysis.
Key Components of Validation Frameworks:
- Rule-based Validations: Define and apply rules that data must adhere to based on organizational standards.
- Cross-Check Mechanisms: Implement processes that allow the validation of data against external or historical datasets.
- Feedback Loops: Establish systems that allow ongoing refinement of validation rules based on historical performance and user feedback.
In short, the deployment of the right tools in data quality management is not just a recommendation; it is a necessity in the current landscape. Embracing automation, visualization, and validation together creates a robust framework, allowing organizations to put their best foot forward in their data journey.
Challenges in Data Quality Engineering
In the realm of data quality engineering, the hurdles can be significant and varied. Every organization relies on data to inform decisions, drive growth, and enhance operations. Itâs not just a matter of generating vast amounts of data; the critical focus lies in ensuring that this data is reliable, accurate, and useful. Addressing the challenges associated with data quality is essential for effective data management. Failure to do so can lead to misguided strategies, wasted resources, and an erosion of stakeholder trust. Therefore, tackling these challenges head-on is not merely beneficial, it is an imperative for organizations aiming to thrive in the information age.
Identifying Data Quality Issues
One of the first steps in addressing data quality is identifying the issues lurking within datasets. This isnât as straightforward as it might sound. Problems can be subtle and might creep in during various stages of the data lifecycle. Common issues include missing values, duplication of records, or incorrect entries. Identifying these anomalies often requires a keen eye and the right set of tools.
Spotting data quality issues can take different forms:
- Automated Data Profiling: Using specialized software tools can help automate the discovery of inconsistencies and inaccuracies in data. These tools analyze data patterns and flag anomalies for review.
- Manual Audits: Sometimes, the best way to find issues is to go through the data manually. This is time-consuming but can yield insights that algorithms may overlook.
- Stakeholder Input: Engaging with users who rely on the data can also bring to light issues that may not be visible through automated means. Their feedback can help recognize discrepancies from actual usage.

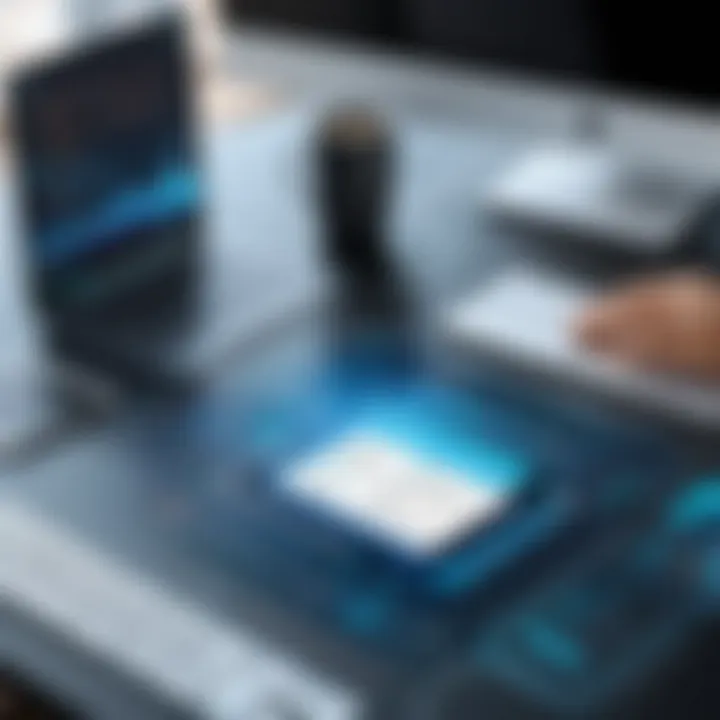
By harmonizing these approaches, organizations can develop a robust identification strategy that serves as the foundation for subsequent data quality initiatives.
Overcoming Resistance to Change
Change is often met with resistance, especially in organizations where data processes have remained stagnant for many years. When it comes to data quality engineering, the implementation of new standards or technologies can be seen as an unwelcome shake-up to routine operations.
To effectively navigate this challenge, communication is key. Here are several strategies that might facilitate smoother transitions:
- Education and Training: Providing staff with tools and training not only empowers them but also diminishes fear of the unknown. Understanding the why and how behind changes can foster acceptance.
- Clear Vision and Leadership: Having clear, confident direction from leadership can guide teams through the shifting landscape. This helps in articulating the importance of data quality initiatives, showing how these changes contribute to broader organizational goals.
- User Involvement: Engaging users in the decision-making process can help in understanding their concerns and incorporating their feedback into new initiatives, making them more likely to accept change.
Overcoming resistance to change is not about force but instead about fostering an environment that values collaboration and open dialogue.
Integrating Data Quality into Business Processes
Integrating data quality initiatives into existing business processes can seem daunting. However, realizing that data quality is not just a one-off project but an ongoing necessity is crucial. Companies that treat data quality as part of their operational fabric reap considerable rewards.
Key considerations for integrating data quality into business processes include:
- Mapping Data Workflows: By understanding current data flow and usage across the organization, teams can identify where quality controls can be integrated without damaging efficiency.
- Establishing Governance Frameworks: Instituting data governance policies will ensure ongoing attention to data quality. This framework delineates roles, responsibilities, and processes for maintaining high data standards.
- Continuous Feedback Loops: Creating mechanisms for continuous feedback helps in fine-tuning processes over time. Regular assessments of data quality can lead to agile adjustments, ensuring that processes are always aligned with organizational goals.
"Incorporating data quality into everyday practices transforms it into the backbone of business intelligence rather than a mere afterthought."
Best Practices for Data Quality Improvement
Ensuring high quality data isnât just a nice-to-have; it's essential for robust decision-making in any organization. Best practices in data quality improvement serve as the framework needed to maintain high standards. They guide organizations in aligning their data strategies with overarching business goals, which can ultimately enhance operational efficiency, reduce costs, and improve stakeholder satisfaction.
Establishing Data Governance
Data governance is akin to setting the rules of the road for data-related processes. It involves defining who can access what data and under which circumstances. In essence, it's about accountability. The importance of establishing clear data governance structures cannot be overstated. Without it, data can easily become unmanageable, leading to issues that can snowball and affect entire teams or departments.
- Key elements of data governance include:
- Roles and responsibilities: Clearly detailing who owns different data sets. This promotes accountability.
- Policies and procedures: Establishing guidelines for data management practices.
- Data stewardship: Appointing individuals to oversee data quality.
An effective data governance framework not only improves data quality but also ensures compliance with regulations like GDPR or HIPAA. In practice, this looks like regular audits, maintaining data lineage, and even utilizing data catalogs for visibility.
Implementing Continuous Monitoring
You can't fix what you don't measure. Continuous monitoring of data quality provides organizations with ongoing insights into their data landscape. It allows for quickly identifying and addressing quality issues before they escalate. By establishing a culture that prioritizes constant vigilance over data, organizations can effectively ensure that data remains useful and accurate.
Some benefits of continuous monitoring include:
- Early Detection: Spot data quality issues in real-time rather than after a major incident.
- Proactive Adjustments: Modify processes based on data trends before they become problematic.
- Stakeholder Trust: Consistent data quality enhances credibility with everyone who relies on this data.
A practical tool for implementing continuous monitoring could be a dashboard that tracks key data quality metrics. This allows for instant visibility and can facilitate timely action when issues arise.
Training and Awareness Programs
Just having the best technology or processes is still not enough. People are at the heart of data quality improvement. Training and awareness programs elevate the importance of data quality throughout the organization. When team members understand the impact of poor data quality, they'll be more likely to take ownership of the data they work with.
Consider the following:
- Custom Training Modules: Tailor training to fit specific roles and responsibilities. Not every team needs the same education.
- Real-World Case Studies: Show how poor data quality has led to negative consequences in the past, illustrating the tangible impacts.
- Career Development: Tie data quality training into employee performance reviews and growth opportunities.
By fostering an environment where continuous learning related to data quality is valued, organizations can create a culture that prioritizes excellence in data management.
"Quality data is not just an IT issue; it's a core business issue that needs everyone's commitment."
Implementing best practices for data quality improvement lays the foundation for lasting success. Through effective governance, persistent monitoring, and focused training, organizations can elevate their data quality efforts. This will, in turn, lead to more informed decision-making and ultimately set the stage for better outcomes.
Future Trends in Data Quality Engineering
In an era where data reigns supreme, the future trends in data quality engineering are not only timely but essential. Organizations are increasingly relying on accurate data to drive their decision-making. These trends signal shifts that could redefine how data quality processes are implemented, managed, and optimized. Letâs explore some pivotal aspects related to these trends, particularly focusing on advancements in technology and cultural changes in organizations.
The Impact of Machine Learning
Machine learning has begun to weave itself into the very fabric of data quality engineering. Instead of just relying on manual checks or traditional algorithms, systems now begin to predict potential data quality issues before they escalate. For instance, algorithms can analyze vast data sets to identify patterns or anomalies that may go unnoticed by the human eye. This predictive capability can act as an early warning system, enabling organizations to tackle data inaccuracies proactively.
Moreover, machine learning models can be trained to recognize quality issues across different datasets, making them adaptable. This built-in flexibility caters well to the constant evolution of data sources. All this together drastically reduces the time spent on reactive data cleaning processes.
"Machine learning is not just a tool; it's the driving engine behind the transformation of data quality management."
Advancements in AI and Automation
The integration of artificial intelligence and automation into data quality engineering presents monumental benefits.
- Efficiency: Automation of routine tasks, like data profiling and cleansing, is not just a convenience; it streamlines processes, resulting in faster turnaround times for analytics.
- Scalability: With growing data volumes, AI-driven tools help organizations scale their data quality efforts without needing proportional increases in manpower.
- Enhanced Accuracy: AI tools can continuously learn from previous data quality issues. This continuous improvement loop ensures that data quality measures become more robust and reliable over time.
However, deploying these technologies is not without challenges. Training staff on new tools and ensuring seamless integration with existing systems can pose hurdles, yet the long-term advantages often outweigh these initial hurdles.
The Shift Towards Data-Driven Cultures
Building a data-driven culture is an ongoing journey for many organizations, and it starts by understanding the value of high-quality data. In this context, data quality engineering is no longer the sole responsibility of a specialized team; instead, it becomes a fundamental part of everyoneâs role.
This dramatic shift encourages:
- Collaboration Across Departments: With a focus on quality, teams must communicate more frequently about their data usage, leading to improved standards and practices.
- Elevated Awareness: Staff at all levels begin recognizing the importance of data integrity, motivating them to improve data input and management practices at ground level.
- Leadership Buy-In: As executives recognize that better quality data translates to better business outcomes, they make it a priority across the organization.
Emphasizing data governance and inclusivity is key to fostering this culture. Organizations will likely invest in tools, training, and processes that create an environment where quality is paramount.