Unveiling the Profound Insights of Big Data: An In-Depth Survey
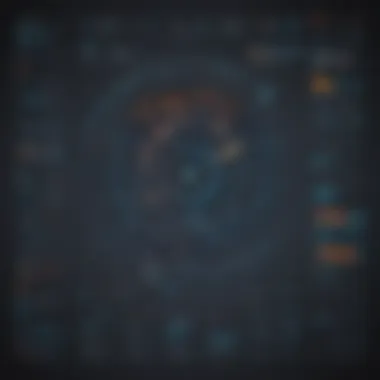
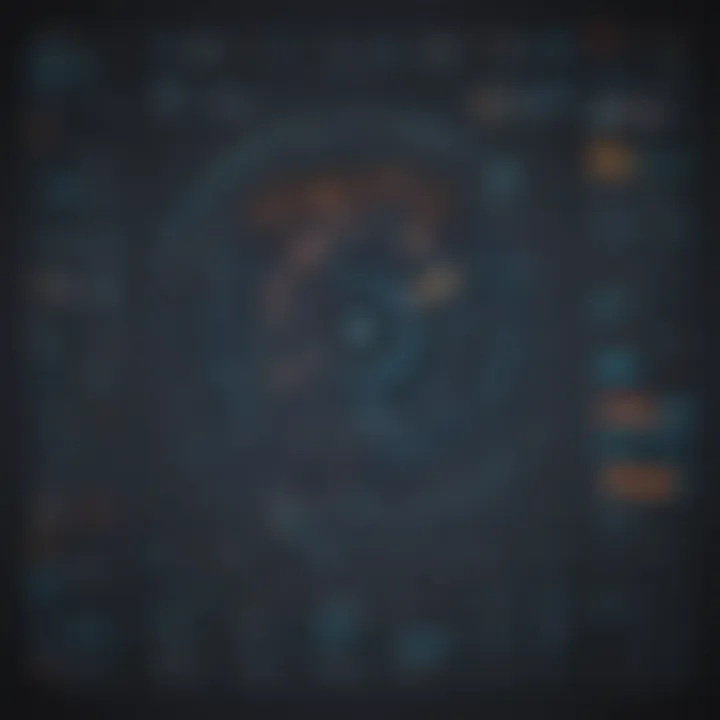
Understanding Big Data Concepts and Implications
Analyzing the Impact of Big Data
In dissecting the impact of big data, one delves into the intricate web of data analytics, decision-making processes, and the transformative power of insights derived from massive datasets. Through a detailed survey, we unravel the profound implications of big data on driving innovation, enhancing operational efficiency, and shaping strategic directions across different sectors. The analysis extends beyond surface-level observations, offering a profound understanding of how big data catalyzes change and progress in today's data-driven world.
Unraveling the Challenges of Big Data Management
Within the realm of big data lie formidable challenges that organizations encounter in capturing, storing, managing, and analyzing data at scale. This section explores the hurdles faced in ensuring data security, maintaining data integrity, and deriving meaningful insights from the deluge of information available. Through a comprehensive survey lens, we examine the strategies and technologies employed to mitigate these challenges, providing insights into best practices and innovative solutions that address the complexities of big data management.
Harnessing the Benefits of Big Data Insights
While challenges abound in managing big data, so too do the benefits await those who can effectively harness its insights. This section sheds light on the competitive advantages and transformative potential that big data offers to organizations across sectors. From predictive analytics driving strategic decision-making to AI-powered innovations revolutionizing customer experiences, the benefits of leveraging big data insights are manifold. Through a detailed survey, we uncover practical applications of big data insights and illuminate the path to deriving tangible value from data-driven strategies.
Synthesizing the Insights
In synthesizing the information gleaned from our comprehensive survey, a holistic view of big data emerges. The convergence of impact, challenges, and benefits paints a nuanced picture of the evolving data landscape and the strategic imperatives for organizations looking to capitalize on the power of big data. By distilling key takeaways and synthesizing actionable insights, this survey equips tech enthusiasts, professionals, and researchers with a roadmap for navigating the complexities and possibilities presented by big data analytics and insights.
Introduction
Welcome to this comprehensive exploration of the insights extracted from the realm of Big Data. In this intricate puzzle of data abundance, we aim to carve out a detailed map that navigates through the very essence of Big Data and its multifaceted dimensions. Understanding the complexities and nuances of Big Data is pivotal in today's data-driven landscape, where organizations harness this treasure trove to gain competitive advantages and insights that pave the way for strategic decision-making and innovation.
Defining Big Data
Characteristics of Big Data
Diving into the ocean of Big Data, we encounter its defining characteristics that distinguish it from conventional data sets. The sheer volume, velocity, variety, and veracity of data encapsulate the essence of Big Data. Its mammoth size challenges traditional data processing methods, necessitating advanced analytics tools and techniques. This characteristic trait of Big Data fuels the revolution in data science, enabling organizations to extract valuable insights, detect patterns, and predict trends with unparalleled accuracy and depth.
Importance in the Digital Age
Amidst the digital deluge, the significance of Big Data in the digital age cannot be overstated. Organizations across industries harness the power of Big Data to gain a competitive edge, drive innovation, enhance customer experiences, and optimize operational efficiencies. In the era where data is hailed as the new currency, leveraging Big Data has become imperative for staying ahead in the dynamic business landscape. Its role in transforming raw data into actionable insights and driving informed decision-making propels organizations towards success in a data-centric world.
Scope of the Survey
Objectives of the Survey
The survey sets out with the primary objective of unraveling the intricate tapestry of Big Data, shedding light on its impact, challenges, and benefits across various industry verticals. By delving deep into the nuances of Big Data, the survey aims to provide a comprehensive overview that resonates with tech enthusiasts, professionals, and researchers alike. Unearthing the underlying principles and applications of Big Data, the survey strives to arm its audience with a profound understanding of this transformative force shaping the digital landscape.
Focus Areas
Within the expansive realm of Big Data, the survey zooms in on key focus areas that define its applications and implications. From data analytics and predictive modeling to machine learning algorithms and data visualization techniques, the survey accentuates the core pillars that uphold the domain of Big Data. By spotlighting these focal points, the survey aims to offer a holistic view of the diverse facets of Big Data and its relevance in driving innovation, informed decision-making, and operational excellence.
Significance of Big Data Analysis
Role in Business Intelligence
Enabling organizations to unravel valuable insights from vast datasets, Big Data plays a pivotal role in shaping business intelligence strategies. By harnessing advanced analytics and machine learning algorithms, businesses can uncover hidden patterns, trends, and correlations that empower strategic decision-making and operational optimizations. The integration of Big Data analytics in business intelligence frameworks equips organizations with the tools to forecast market trends, enhance customer experiences, and drive innovation with data-driven precision.
Applications across Industries
The versatile applications of Big Data span across a multitude of industries, from healthcare and finance to retail and manufacturing. By leveraging Big Data analytics, industries can streamline operations, optimize supply chains, personalize customer interactions, and innovate product offerings. The ubiquitous nature of Big Data transcends sector boundaries, offering a transformative lens through which industries can enhance efficiencies, mitigate risks, and seize opportunities for growth and differentiation.
Understanding Big Data
Big data is a pivotal aspect of this article, dictating the essence of data analytics in the modern digital landscape. Understanding Big Data encompasses the ability to harness vast volumes of information to extract valuable insights. It serves as the cornerstone for making informed decisions, facilitating innovation, and enhancing operational efficiency. By delving into the nuances of Big Data, readers gain a deep understanding of its significance across industries and the implications it holds for data-driven strategies.
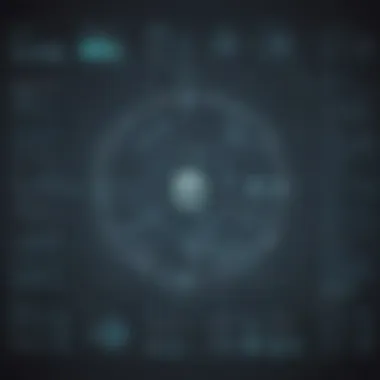
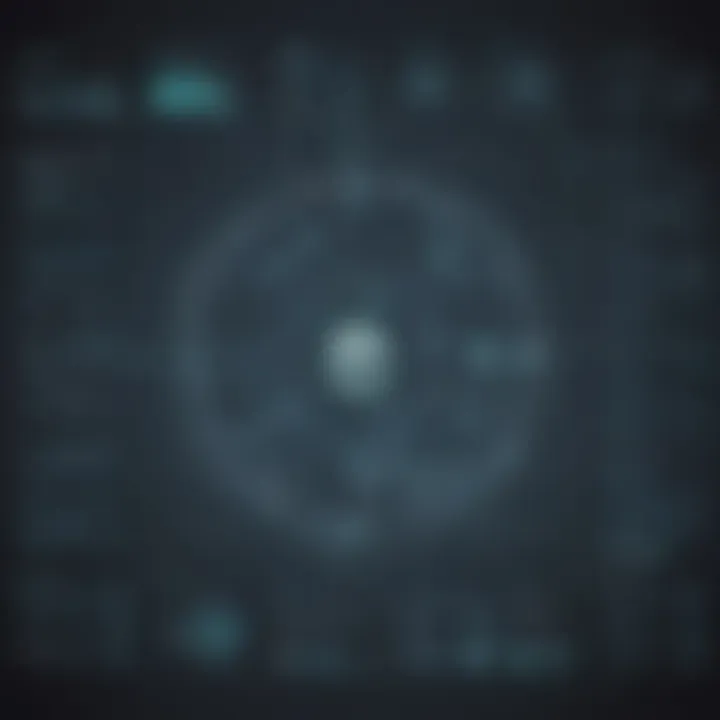
Volume
Generation and Storage of Data
Generation and storage of data play a crucial role in fueling Big Data initiatives. The process involves collecting and preserving massive quantities of information from diverse sources. This aspect not only ensures a continuous influx of data but also necessitates advanced storage solutions to handle the exponential growth. The key characteristic of this element lies in its ability to capture various data types efficiently, ranging from structured to unstructured information. While the generation and storage of data enable extensive data mining and analysis, they also present challenges in terms of scalability and maintenance.
Data Explosion
Data explosion denotes the rapid increase in data volume, a phenomenon directly linked to modern digitization. The explosion is fueled by the ubiquitous presence of digital platforms, IoT devices, and online activities. This surge in data creation amplifies the need for robust infrastructure to accommodate and process the inflow. The key characteristic here is the exponential nature of data growth, which underscores the necessity for scalable and adaptable data management systems. Although data explosion brings unrivaled opportunities for businesses, it also poses challenges related to data organization, security, and processing bandwidth.
Velocity
Real-time Data Processing
Real-time data processing stands as a critical element in the Big Data realm, facilitating instantaneous analysis and decision-making. This practice involves handling data continuously and promptly, ensuring that insights are current and actionable. The key characteristic of real-time processing lies in its ability to provide immediate feedback and response to incoming data streams. This real-time capability is essential for applications requiring time-sensitive responses, such as financial transactions and IoT implementations. While real-time data processing offers unparalleled agility, it demands robust infrastructure and adept data processing algorithms.
Internet of Things (IoT) Impact
The IoT impact on data velocity is substantial, revolutionizing the speed at which data is generated and consumed. IoT devices, interconnected through networks, generate copious amounts of real-time data, necessitating rapid processing mechanisms. The key characteristic of IoT connectivity is its continuous data transmission and reception, requiring swift processing to derive insights efficiently. This impact creates new possibilities in monitoring, automation, and decision-making processes. However, managing the velocity of IoT data poses challenges in terms of scalability, security, and network latency.
Variety
Structured vs. Unstructured Data
The structured vs. unstructured data dichotomy underscores the diversity of data formats encountered in Big Data environments. Structured data adheres to predefined schemas and is organized in tabular forms, enabling easy categorization and analysis. In contrast, unstructured data lacks a fixed format and includes textual, multimedia, and sensor data. The key characteristic here is the flexibility and richness offered by unstructured data sources, presenting opportunities for deriving novel insights. While structured data allows for conventional analysis methods, integrating unstructured data requires advanced techniques like natural language processing and image recognition.
Data Sources Diversity
The diversity of data sources represents the eclectic mix of information channels contributing to Big Data repositories. These sources encompass social media platforms, IoT devices, enterprise systems, and external data feeds. The key characteristic of data source diversity lies in the broad spectrum of data types and origins, enriching the analytical landscape. Leveraging diverse data sources enables comprehensive trend analysis, anomaly detection, and predictive modeling. However, managing data from disparate sources entails data integration challenges, necessitating robust data governance frameworks and interoperability solutions.
Veracity
Data Quality Challenges
Data quality challenges are inherent in Big Data environments, given the sheer volume and variety of information being processed. Ensuring data accuracy, consistency, and relevance poses continual challenges for organizations. The key characteristic of data quality challenges is the need for data validation, cleansing, and normalization processes to maintain accuracy and integrity. Addressing these challenges requires robust data quality frameworks, automated validation tools, and cross-functional collaboration. While maintaining high data quality enhances decision-making and operational outcomes, overlooking data veracity can lead to skewed insights and misguided actions.
Trustworthiness and Reliability
The trustworthiness and reliability of data are paramount for deriving actionable insights and building dependable analytical models. Establishing trust in data sources and ensuring data reliability underpins the credibility of analytical outcomes. The key characteristic here is the emphasis on data provenance, consistency, and transparency throughout the data lifecycle. Trustworthy and reliable data instills confidence in decision-makers, fostering a data-driven culture within organizations. However, maintaining data trustworthiness requires continuous monitoring, governance frameworks, and adherence to data quality standards.
Impact of Big Data
Big Data's significance within the realm of technology is immense, playing a pivotal role across various industries. In the context of this article, exploring the impact of Big Data unveils the layers of data-driven insights and predictive analytics that empower decision-making processes and drive innovation. Understanding how Big Data influences business operations and strategic planning is crucial for navigating the complexities of the digital landscape. With the increasing reliance on data-driven strategies, embracing the insights derived from Big Data becomes a cornerstone for success.
Enhanced Decision-making
Data-driven Insights:
Data-driven insights stand as a beacon of informed decision-making within the vast sea of data. By harnessing analytics tools and techniques, organizations can extract valuable information from complex datasets, enabling them to make strategic choices backed by empirical evidence. The unique advantage of data-driven insights lies in their ability to unravel patterns and trends that might otherwise remain hidden, providing a competitive edge in today's data-centric environment. Though empowering decision-making, this approach demands a meticulous analysis to ensure accuracy and relevance to the context of the discussion.
Predictive Analytics:
Predictive analytics revolutionizes decision-making by forecasting future trends and outcomes based on historical data trends. By leveraging machine learning algorithms and statistical models, predictive analytics empowers organizations to anticipate market shifts, customer behaviors, and operational challenges proactively. The distinctive feature of predictive analytics lies in its capacity to mitigate risks and identify opportunities for growth, thereby optimizing strategic planning and resource allocation. However, the effectiveness of predictive analytics hinges on the quality and relevance of the data utilized, underscoring the importance of data veracity and integrity in this analytical process.
Business Innovation

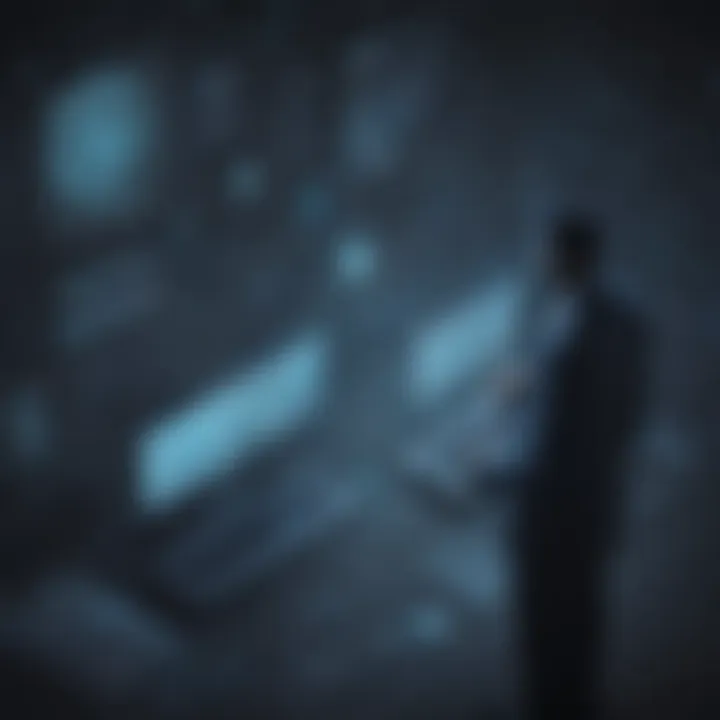
Product Development:
Product development serves as a cornerstone for driving innovation and meeting evolving consumer demands in the marketplace. By leveraging Big Data insights, organizations can tailor their product offerings to align with consumer preferences and market trends effectively. The key characteristic of product development lies in its iterative nature, fostering continuous improvement and adaptation to changing market dynamics. While accelerating time-to-market and enhancing product quality, organizations must balance the innovation potential with cost-effective strategies to ensure sustainable growth and competitive positioning.
Market Trends Analysis
Market trends analysis equips organizations with the ability to decipher consumer behaviors, competitor strategies, and industry shifts in real-time. By employing sophisticated analytical tools, businesses can extract actionable insights from market data, enabling them to make informed decisions and capitalize on emerging opportunities swiftly. The distinct feature of market trends analysis lies in its capacity to guide strategic planning and resource allocation, facilitating proactive responses to market fluctuations and competitive pressures. However, organizations must exercise caution in interpreting market trends, considering the dynamic nature of consumer preferences and industry regulations that could impact the accuracy of their analysis.
Operational Efficiency
Process Optimization:
Process optimization focuses on streamlining workflows, eliminating bottlenecks, and enhancing operational efficiency to maximize productivity and reduce costs. By applying data-driven approaches, organizations can identify inefficiencies, automate repetitive tasks, and improve overall process effectiveness. The key characteristic of process optimization lies in its ability to enhance resource utilization, minimize errors, and accelerate time-to-delivery, thereby enhancing customer satisfaction and organizational performance. While optimizing processes, organizations must strike a balance between agility and stability to adapt to changing market demands without sacrificing quality or compliance.
Cost Reduction Strategies
Cost reduction strategies revolve around identifying cost-saving opportunities, optimizing resource allocation, and minimizing operational expenses to improve profitability. By leveraging data analytics and performance metrics, organizations can pinpoint areas of expenditure, negotiate better vendor contracts, and implement budgeting controls effectively. The unique feature of cost reduction strategies lies in their capacity to drive financial sustainability and allocate resources efficiently, fostering long-term growth and resilience. However, organizations must monitor the impact of cost-cutting measures on product quality and customer experience to avoid compromising value proposition and market competitiveness.
Challenges in Harnessing Big Data
In a tech landscape dominated by massive amounts of data, the challenges in harnessing big data emerge as crucial focal points. The complexity of managing and extracting insights from copious data sets poses significant hurdles for organizations across industries. These challenges encompass a range of specific elements, including data privacy, security measures, data integration, skill gap, and more. Understanding and addressing these challenges are paramount for businesses aiming to leverage big data effectively.
Data Privacy and Security
Compliance Issues
Compliance issues represent a critical aspect in the realm of big data management. Ensuring adherence to regulatory standards and industry guidelines is imperative for safeguarding sensitive information. The key characteristic of compliance issues lies in their ability to dictate data handling protocols and enforce strict regulations to protect data integrity. Compliance issues serve as a vital framework within this article, highlighting the essential role of adherence to data governance standards. While compliance may entail time and resource investments, its advantages in mitigating risks and ensuring data security cannot be overstated.
Cyber Threats
Cyber threats pose a looming concern in the landscape of big data, posing risks to data confidentiality and system integrity. The key characteristic of cyber threats resides in their potential to compromise organizational data through malicious activities such as hacking, phishing, or malware attacks. Within this article, cyber threats underscore the importance of robust cybersecurity measures to counter evolving digital risks. Despite the challenges they pose, addressing cyber threats proactively equips organizations with the resilience needed to safeguard their data from unauthorized access or exploitation.
Data Integration
Silos Breakdown
Silos breakdown represents a pivotal aspect in the successful utilization of big data. Breaking down data silos involves integrating disparate data sources and systems to enable seamless information flow and accessibility. The key characteristic of silos breakdown is its capacity to unify data silos, fostering a holistic view of organizational data for informed decision-making. Within this article, silos breakdown underscores the necessity of cohesive data integration strategies to harness the full potential of big data. While challenges may arise in consolidating diverse data repositories, the benefits of enhanced data connectivity and synergy outweigh the complexities.
Interoperability Challenges
Interoperability challenges underscore the importance of seamless communication and interaction between different systems and platforms. The key characteristic of interoperability challenges lies in their impact on data exchange efficiency and consistency. Within this article, interoperability challenges emphasize the significance of standardizing data formats and protocols to facilitate interoperable data systems. Despite the complexities associated with ensuring interoperability, the advantages of enhanced data sharing and system compatibility are pivotal for leveraging big data effectively.
Skill Gap
Need for Data Scientists
The need for data scientists is a cornerstone in addressing the evolving landscape of big data analytics. Data scientists possess the expertise to interpret complex data sets, derive meaningful insights, and drive data-driven strategies. The key characteristic of the need for data scientists lies in their role as data interpreters and analysts, bridging the gap between raw data and actionable intelligence. Within this article, the demand for data scientists underscores the critical need for specialized skills in data analytics and machine learning. While recruiting and retaining data science talent may pose challenges, the advantages of leveraging expert data analysis skills for decision-making and innovation are invaluable.
Training and Development
Training and development initiatives play a pivotal role in nurturing talent and upskilling professionals in the field of big data. Providing training programs and educational resources enables individuals to enhance their data analysis capabilities and stay abreast of technological advancements. The key characteristic of training and development initiatives lies in their capacity to empower individuals with the knowledge and skills required to navigate the complexities of big data management. Within this article, training and development efforts underscore the importance of continuous learning and skill enhancement to address the skill gap in the big data landscape. While investing in training programs may involve resource allocations, the benefits of a well-equipped workforce proficient in handling big data tasks outweigh the initial investments.
Future Trends in Big Data
In the realm of big data, staying abreast of future trends is paramount to navigating the ever-evolving landscape of data analytics and processing. The section on Future Trends in Big Data within this comprehensive survey sheds light on upcoming developments that will shape the way businesses operate and utilize data. It delves into innovative technologies and methodologies that are projected to revolutionize data management across various industries. Understanding these trends is crucial for IT professionals, cybersecurity experts, and students aiming to make informed decisions and leverage data effectively. This segment offers a visionary outlook into what the future holds for big data analytics, painting a vivid picture of the potential advancements and challenges that lie ahead.
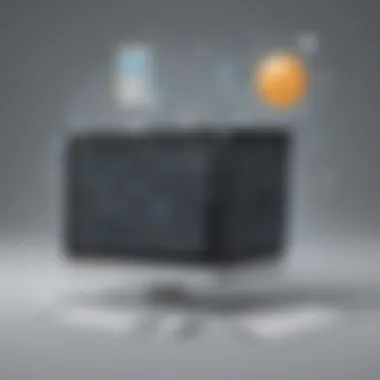
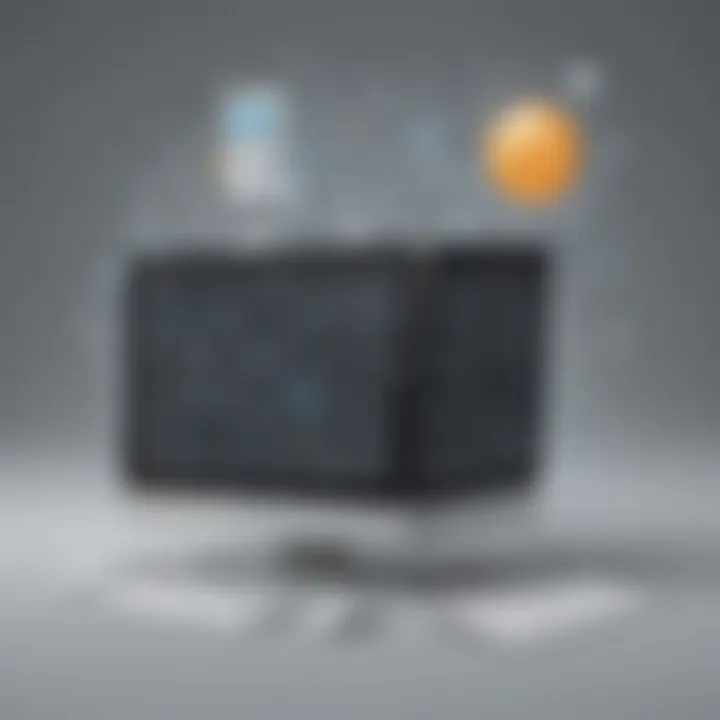
AI and Machine Learning
Automated Insights Generation
Automated Insights Generation plays a pivotal role in expediting data analysis processes by automating the extraction of valuable insights from vast datasets. In the context of this article, Automated Insights Generation enables organizations to garner actionable information swiftly, enhancing decision-making capabilities. Its key characteristic lies in its ability to streamline data interpretation, saving time and resources. The unique feature of Automated Insights Generation is its capability to process immense data volumes efficiently, uncovering patterns and trends that might otherwise go unnoticed. While its advantages include increased productivity and accuracy in data analysis, potential disadvantages may arise from reliance on predefined algorithms, which could overlook nuanced insights.
Pattern Recognition
Pattern Recognition is instrumental in identifying recurring patterns and structures within datasets, facilitating predictive modeling and trend analysis. Within the framework of this article, Pattern Recognition empowers businesses to forecast future trends and make data-driven decisions. Its key characteristic revolves around its capacity to recognize similarities and anomalies in data sets, enabling organizations to derive meaningful conclusions. The unique feature of Pattern Recognition lies in its adaptability across diverse data types and sources, ensuring comprehensive analysis. While its advantages encompass enhanced forecasting accuracy and trend identification, challenges may emerge from interpretation errors or biased data inputs.
Edge Computing
Decentralized Data Processing
Decentralized Data Processing transforms the traditional data processing paradigm by distributing computing resources closer to data sources. In the context of this article, Decentralized Data Processing enhances data processing efficiency and reduces latency by minimizing the distance data travels for analysis. Its key characteristic is the ability to improve real-time data processing and analytics, boosting operational agility. The unique feature of Decentralized Data Processing lies in its capacity to support IoT devices and applications, enabling rapid decision-making at the network edge. While its advantages include improved scalability and responsiveness, challenges may arise from data security risks and network instability.
Reduced Latency
Reduced Latency addresses the critical need for swift data processing and communication by minimizing delays in data transmission. Within the context of this article, Reduced Latency optimizes data transfer speeds, enabling near-instantaneous access to critical information. Its key characteristic lies in its ability to enhance user experiences and support time-sensitive applications such as real-time analytics. The unique feature of Reduced Latency is its capability to boost overall system performance, reducing bottlenecks and inefficiencies. While its advantages encompass improved data accessibility and responsiveness, potential disadvantages may stem from increased network bandwidth requirements and infrastructure costs.
Blockchain Integration
Enhanced Data Security
Enhanced Data Security leverages blockchain technology to safeguard sensitive data against unauthorized access and tampering. In the context of this article, Enhanced Data Security fortifies data integrity and confidentiality, instilling trust in the data exchange process. Its key characteristic lies in its immutable and transparent nature, ensuring data remains unaltered and traceable. The unique feature of Enhanced Data Security is its decentralized architecture, eliminating single points of failure and enhancing resilience against cyber threats. While its advantages include heightened data protection and compliance adherence, challenges may arise from scalability limitations and regulatory complexities.
Immutable Data Records
Immutable Data Records establish a permanent and tamper-evident record of data transactions and interactions using blockchain technology. Within the scope of this article, Immutable Data Records provide a verifiable and auditable trail of data activities, enhancing transparency and accountability. Their key characteristic revolves around the inability to alter or erase historical data entries, ensuring data integrity and authenticity. The unique feature of Immutable Data Records lies in their distributed ledger architecture, enabling secure and trustworthy data sharing. While their advantages encompass data immutability and auditability, potential disadvantages may stem from high computational requirements and limited scalability.
Conclusion
In this concluding section of our exploration on the insights of big data through a comprehensive survey, we encapsulate the essence of our findings and discussions. The significance of reaching a conclusion is paramount as it consolidates the key takeaways and provides a holistic view of the topic. By synthesizing the information presented throughout this survey, we can distill the complexities of big data into actionable insights and valuable learnings for tech enthusiasts, professionals, and researchers alike.
Key Takeaways
Importance of Big Data
Exploring the importance of big data unveils a critical aspect of our survey. Big data plays a pivotal role in driving decision-making processes and fostering innovation across industries. The key characteristic of big data lies in its ability to extract valuable insights from vast datasets, enabling organizations to make informed choices and predict future trends with precision.
The unique feature of big data is its scalability and adaptability to varying data sources, providing a competitive edge in today's data-driven landscape. While the advantages of big data are vast, including improved operational efficiency and enhanced customer experiences, it is crucial to mitigate risks such as data privacy concerns and information security threats to maximize its benefits.
Future Implications
Delving into the future implications of big data forecasts the transformative impact it will have on the technological landscape. The key characteristic of future implications lies in the integration of AI and Machine Learning, ushering in an era of automated insights generation and advanced pattern recognition capabilities. This forward-looking approach positions organizations to leverage cutting-edge technologies for streamlined operations and strategic decision-making.
The unique feature of future implications is the adoption of edge computing, enabling decentralized data processing and reduced latency for real-time analytics. Despite its advantages in enhancing data security through blockchain integration, organizations must navigate challenges associated with data governance and scalability to fully harness its potential.
Closing Thoughts
Continuous Data Evolution
Reflecting on the continuous data evolution underscores the dynamic nature of big data and the evolving trends shaping its utilization. The key characteristic of continuous data evolution is the ongoing growth of data volumes and the emergence of new data sources, necessitating adaptability and innovation in data management practices.
The unique feature of continuous data evolution lies in its ability to drive agility and responsiveness in decision-making processes, enabling organizations to stay ahead in a competitive environment. While the advantages of embracing data evolution are evident in improved business outcomes and market competitiveness, organizations need to address challenges related to data quality maintenance and regulatory compliance to sustain their growth.
Adaptation and Innovation
Exploring adaptation and innovation underscores the transformative potential of leveraging big data for organizational success. The key characteristic of adaptation and innovation is the ability to leverage data insights for strategic business initiatives and product development, fostering a culture of continuous improvement and customer-centricity.
The unique feature of adaptation and innovation lies in its capacity to drive sustainable growth and differentiation in a rapidly changing marketplace. While the advantages of prioritizing adaptation and innovation are manifold, organizations must navigate complexities such as talent acquisition and technology integration to realize long-term success in harnessing the power of big data for innovation and growth.