Unveiling the Intricate Relationship between Big Data and AI
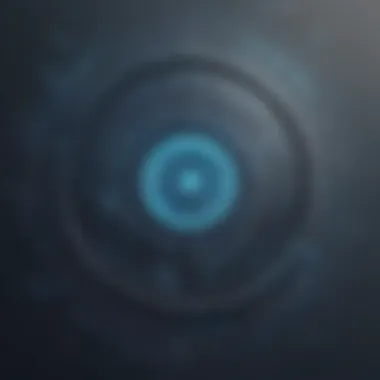
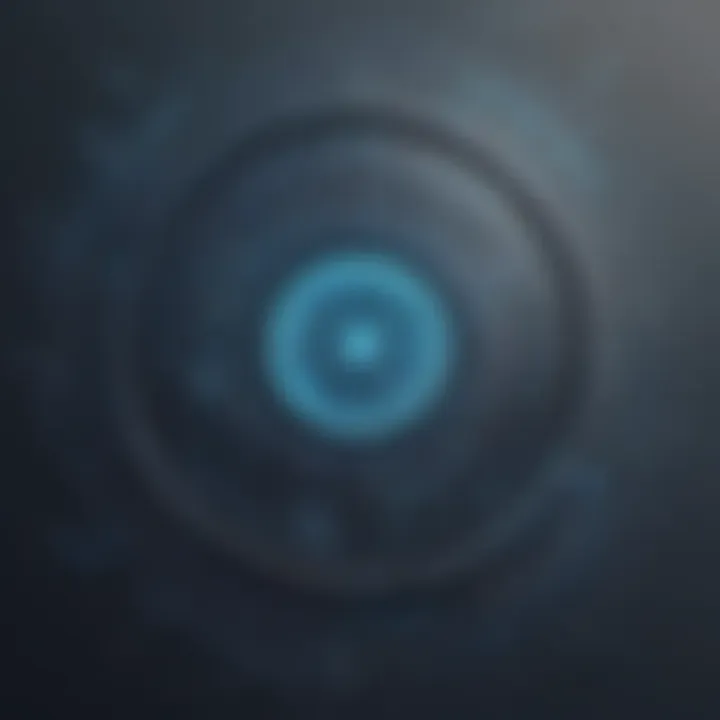
Understanding Storage, Security, or Networking Concepts
In delving into the intricacies of Big Data and Artificial Intelligence (AI), it is imperative to grasp the fundamental concepts of storage, security, and networking that form the backbone of these technologies. Storage refers to the process of storing and managing data in various forms, ensuring accessibility and reliability. Security encompasses measures to protect data from unauthorized access or breaches, safeguarding sensitive information. Networking involves the interconnected systems that enable data exchange and communication. Understanding these core elements is crucial in comprehending the symbiotic relationship between Big Data and AI.
Industry Trends and Updates
When examining the dynamic landscape of Big Data and AI, staying abreast of the latest industry trends is paramount. Continual advancements in storage technologies, cybersecurity protocols, and networking innovations shape the trajectory of these fields. From the evolution of cloud storage solutions to emerging cybersecurity threats and novel networking strategies, being attuned to these developments is essential for professionals in IT and cybersecurity. By monitoring industry trends and updates, organizations can proactively adapt to changes and remain competitive in an ever-evolving technological landscape.
Case Studies and Success Stories
Real-world case studies serve as valuable insights into the practical applications of Big Data and AI in diverse settings. Through examining successful storage implementations, cybersecurity incidents, and effective networking strategies, professionals can glean valuable lessons and best practices. By analyzing these real-life scenarios, individuals can expand their knowledge base, anticipate challenges, and develop resilient strategies for harnessing the power of Big Data and AI effectively. Case studies and success stories offer a window into the practical implications of theoretical concepts, offering tangible examples of how these technologies can drive innovation and transformation.
Reviews and Comparison of Tools and Products
In the realm of Big Data and AI, the landscape is replete with a myriad of tools and products designed to optimize storage, enhance security, and bolster networking capabilities. Through in-depth reviews of storage software and hardware, comparative analyses of cybersecurity tools, and evaluations of networking equipment and services, professionals can make informed decisions about selecting the most suitable solutions for their specific needs. By critically evaluating different tools and products in the market, individuals can streamline their operational processes, fortify their cybersecurity defenses, and maximize the performance of their networks. Reviews and comparisons provide valuable insights into the array of available options, empowering professionals to make strategic choices that align with their organizational objectives.
Introduction to Big Data and AI
In the realm of modern technology, the Introduction to Big Data and AI serves as a critical foundation for understanding the intricate interplay between vast data repositories and artificial intelligence methodologies. This section acts as a gateway into the complex world of data-driven decision-making and innovation brought about by the fusion of big data and AI. By delving into the core principles and applications of both Big Data and AI, readers are primed to grasp the transformative potential they hold across various industries.
Defining Big Data
Volume, Velocity, Variety
When it comes to exploring Big Data, the dimensions of Volume, Velocity, and Variety stand out as fundamental pillars shaping the landscape of information processing. The Volume aspect refers to the sheer magnitude of data being generated daily, demanding advanced tools and algorithms for analysis. Velocity highlights the speed at which data is produced and must be processed, emphasizing the need for real-time capabilities in data handling. Meanwhile, Variety underscores the diverse forms and sources of data, ranging from structured to unstructured formats, enriching the depth of insights gleaned. Understanding and harnessing these characteristics are crucial for leveraging Big Data effectively in AI applications, ensuring a robust foundation for informed decision-making.
Characteristics of Big Data
As we navigate through the Characteristics of Big Data, its defining traits come to the forefront, steering the course of data processing and utilization. Scalability, a key characteristic, caters to the seamless expansion of data storage and analytics systems, accommodating the burgeoning volume of information influx. Complexity underscores the multifaceted nature of data sources and relationships, challenging traditional analytics approaches and prompting the adoption of advanced techniques. Additionally, Veracity emphasizes the reliability and accuracy of data, a critical consideration for maintaining data integrity and driving informed decision-making processes within AI frameworks. Recognizing the nuances of these characteristics is paramount for businesses seeking to extract value from their data assets and empower AI-driven functionalities.
Understanding AI
Machine Learning
In the domain of AI, Machine Learning emerges as a pivotal cog driving predictive analytics and actionable insights within data-rich environments. The essence of Machine Learning lies in its ability to enable systems to learn from data iteratively, without explicit programming, fostering autonomous decision-making capabilities. This capacity makes Machine Learning a sought-after paradigm for organizations looking to harness the predictive power of algorithms and unlock hidden patterns within voluminous datasets. The versatility and adaptability of Machine Learning algorithms empower businesses across sectors to optimize processes, enhance productivity, and drive innovation by leveraging the predictive capabilities ingrained within AI systems.
Deep Learning
Deep Learning, a subset of Machine Learning, ushers in a new era of AI prowess by mimicking the neural network structure of the human brain, facilitating complex pattern recognition and decision-making processes. Its deep neural networks enable the extraction of high-level abstractions from raw data, paving the way for sophisticated analysis and interpretation of intricate datasets. By delving into diverse layers of data representation, Deep Learning algorithms excel at uncovering subtle patterns and dependencies, steering AI applications towards enhanced accuracy and efficiency. The innate capability of Deep Learning to decipher intricate data structures positions it as a cornerstone for achieving groundbreaking advancements in AI research and development.
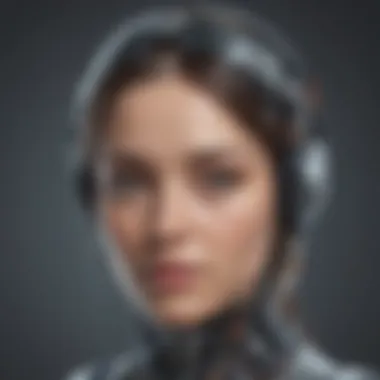
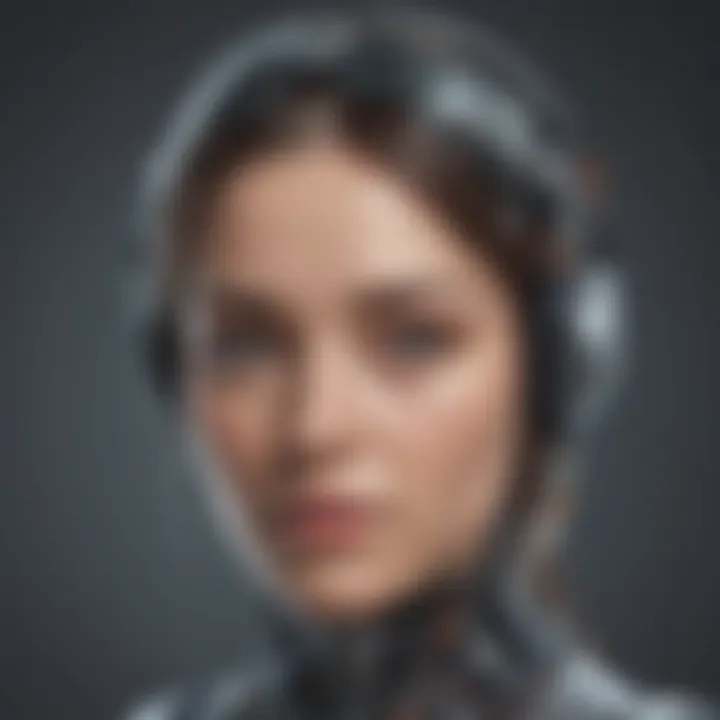
Natural Language Processing
Natural Language Processing (NLP) epitomizes the fusion of linguistic insights with computational intelligence, enabling machines to comprehend, interpret, and generate human language proficiently. Through NLP algorithms, machines can decipher the nuances of human communication, facilitating tasks such as sentiment analysis, language translation, and information extraction. By incorporating context and semantics into language understanding, NLP algorithms transcend mere word-based processing, delving into the realm of contextual understanding and cognitive reasoning. The application of NLP in AI frameworks empowers systems to interact seamlessly with users, streamline information retrieval processes, and augment decision-making through comprehensive language comprehension capabilities.
This extraordinary guide plunges deep into the convergence of Big Data and AI, unraveling the intricate threads that bind information abundance with intelligence augmentation. By scrutinizing each section and subsection meticulously, readers embark on a journey through the nuances of data processing, algorithmic mastery, and transformative applications reshaping the technological landscape. Dive into the profound synergy between data-driven insights and artificial intelligence innovations, unlocking a realm of possibilities that promise to redefine the future of industries and digital evolution.
The Role of Big Data in AI Development
In this section, we unravel the pivotal role that Big Data plays in the development of Artificial Intelligence (AI). Big Data, characterized by massive volumes, high velocity, and diverse varieties, serves as the backbone for AI algorithms to derive significant insights. Without substantial data sources, AI systems lack the foundational support needed to make accurate decisions and predictions. Data Collection and Processing are crucial elements in this context as they ensure that the raw data gathered from various sources is curated, cleaned, and structured in a way that is conducive to AI analysis and utilization. Feature Engineering further refines the data by focusing on aspects like Dimensionality Reduction and Feature Selection, which streamline the dataset, making it more manageable for AI models to process efficiently. Model Training and Optimization steps, including Supervised and Unsupervised Learning techniques, fine-tune the AI algorithms to improve accuracy and performance.
Data Collection and Processing
Data Sources
Delving into the realm of Data Sources, we encounter the fundamental building blocks of Big Data in the realm of AI development. These sources refer to the origins of data, ranging from social media platforms and IoT devices to enterprise databases and sensor networks. Understanding the nature of Data Sources allows AI systems to tap into a wide array of information, capturing diverse perspectives and insights. The key characteristic of Data Sources lies in their ability to provide real-time and historical data that feeds AI algorithms, enabling responsive and informed decision-making. However, one must be cautious about the quality and reliability of data emanating from these sources, as inconsistencies or inaccuracies can significantly impact the outcomes of AI processes. Despite potential challenges, Data Sources remain a popular choice for AI applications due to the sheer magnitude of information they offer.
Data Preprocessing
Transitioning to Data Preprocessing, we witness the crucial phase where raw data undergoes transformation and refinement for AI consumption. This preparatory stage involves cleaning, normalization, and feature extraction, ensuring that data is uniform and structured for seamless AI integration. The key characteristic of Data Preprocessing lies in its ability to enhance data quality, eliminate redundancies, and address missing values, making the dataset more robust and reliable for AI analysis. While Data Preprocessing is essential for optimizing AI performance, it can be a resource-intensive process that requires careful attention to detail. Despite its time-consuming nature, Data Preprocessing remains a beneficial choice for enhancing the efficacy of AI algorithms in extracting valuable insights from complex datasets.
Feature Engineering
Dimensionality Reduction
Embarking on the dimensionality reduction journey, we navigate through the process of streamlining datasets to focus on essential information while discarding irrelevant aspects. Dimensionality Reduction aims to simplify the dataset by decreasing the number of input variables without losing critical information, thereby enhancing computational efficiency and model performance. The key characteristic of Dimensionality Reduction lies in its ability to minimize noise and redundancy in the data, enabling AI models to operate more swiftly and accurately. While this technique can improve processing speed and reduce overfitting risks, it may lead to information loss if not implemented thoughtfully. Despite potential trade-offs, Dimensionality Reduction stands as a popular choice for optimizing AI workflows by simplifying complex data structures.
Feature Selection
Transitioning to Feature Selection, we encounter the process of identifying pertinent features that contribute significantly to AI model outcomes. This selective approach aims to retain relevant attributes while discarding irrelevant or redundant variables, enhancing model interpretability and performance. The key characteristic of Feature Selection lies in its ability to improve algorithm efficiency by focusing on essential data components, reducing model complexity, and minimizing overfitting risks. By selecting only the most impactful features, AI systems can learn more effectively and generalize better to new datasets. Despite its benefits, Feature Selection may pose challenges in determining the most relevant features and balancing computational costs. Nevertheless, its advantages in enhancing AI model accuracy and interpretability make it a valuable choice for optimizing data-driven decision-making.
Model Training and Optimization
Supervised Learning
Delving into Supervised Learning, we explore the method where AI models are trained on labeled data to predict outcomes or classify inputs based on known examples. This approach involves mapping input data to correct output labels, enabling the model to learn from historical patterns and make accurate predictions on new data. The key characteristic of Supervised Learning lies in its ability to learn from labeled datasets, refining predictions over time and adapting to changing inputs. By providing clear guidance through labeled examples, Supervised Learning enhances model accuracy and generalization capabilities. However, challenges such as overfitting and scarceness of labeled data must be addressed to ensure optimal performance. Despite potential obstacles, Supervised Learning remains a popular choice for training AI models in various applications due to its interpretability and efficiency.
Unsupervised Learning
Shifting our focus to Unsupervised Learning, we uncover the realm where AI models explore unlabeled data to discover hidden patterns, structures, or groupings without explicit guidance. This approach involves clustering data points based on similarities or associations, revealing intrinsic relationships and insights within the dataset. The key characteristic of Unsupervised Learning lies in its ability to identify hidden patterns and anomalies in data, enabling AI systems to uncover valuable insights without predefined labels. By leveraging self-organizing mechanisms, Unsupervised Learning enhances data exploration and pattern recognition, albeit with challenges such as scalability and interpretability. Despite potential complexities, Unsupervised Learning stands as a powerful choice for uncovering latent structures and trends within vast datasets, augmenting the depth and breadth of AI analysis and decision-making.
Applications of Big Data and AI Integration
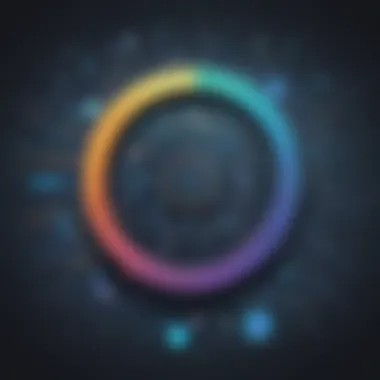
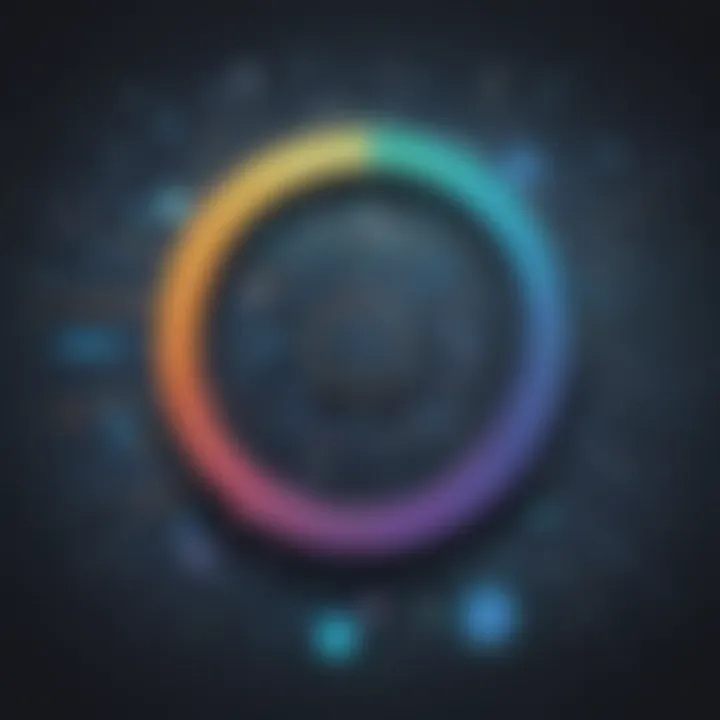
In the expansive landscape of data analytics, the fusion of Big Data and AI represents a groundbreaking paradigm shift, elevating insights, innovations, and decision-making processes to unprecedented levels. The synergy between these two technological powerhouses has unlocked a trove of potential applications across various industries, heralding a new era of intelligent data utilization. By integrating Big Data and AI, organizations can harness predictive analytics, recommendation systems, and natural language processing to optimize operations, enhance customer experiences, and drive strategic growth initiatives.
Predictive Analytics
Forecasting
Forecasting stands as a pivotal component within the realm of predictive analytics, offering organizations the ability to extrapolate future trends, behaviors, and outcomes based on historical data patterns. The essence of forecasting lies in its capability to empower decision-makers with foresight, enabling proactive measures to be taken in response to anticipated developments. Its primary characteristic revolves around the utilization of statistical models and machine learning algorithms to generate accurate predictions, aiding businesses in mitigating risks, seizing opportunities, and maintaining a competitive edge within dynamic markets. However, the accuracy of forecasting models can be influenced by data quality, model complexity, and external factors, necessitating robust validation mechanisms to ensure reliability and relevance in decision-making processes.
Trend Analysis
Trend analysis serves as a seminal element in predictive analytics, facilitating the identification, interpretation, and visualization of patterns within datasets to uncover underlying dynamics and relationships. This analytical approach underscores the significance of recognizing evolving trends and phenomena, empowering organizations to adapt strategies, capitalize on emerging opportunities, and mitigate risks effectively. The key characteristic of trend analysis lies in its ability to discern meaningful insights from data streams, guiding stakeholders in making informed decisions and formulating evidence-based strategies for sustainable growth. While trend analysis can provide valuable strategic foresight, inherent challenges such as data noise, outlier detection, and interpretation biases demand a meticulous approach to data prepossessing and filtering to enhance the accuracy and reliability of trend predictions.
Recommendation Systems
Personalization
Personalization stands at the forefront of recommendation systems, tailoring user experiences, product recommendations, and content delivery based on individual preferences, behaviors, and feedback. The essence of personalization lies in its capacity to enhance customer engagement, loyalty, and satisfaction through curated offerings that align with user expectations and demands. This personalized approach not only enriches user interactions but also drives conversion rates, customer retention, and lifetime value, fostering sustainable relationships between businesses and consumers.
Content Filtering
Content filtering plays a crucial role in recommendation systems, streamlining information retrieval processes by screening and categorizing content based on relevance, quality, and user preferences. The primary characteristic of content filtering revolves around the automated classification and prioritization of data streams to deliver personalized recommendations that resonate with user interests and requirements. By implementing content filtering algorithms, organizations can optimize content delivery, enhance user engagement, and streamline decision-making processes, ensuring that users receive tailored information that aligns with their needs and expectations.
Natural Language Processing Applications
Chatbots
Chatbots represent a transformative application of natural language processing, enabling automated conversational interactions between users and intelligent virtual agents. The key characteristic of chatbots lies in their ability to interpret, process, and generate human-like responses to user queries, requests, and commands, enhancing customer service, support, and engagement. By leveraging chatbot technology, businesses can automate routine tasks, streamline communication channels, and deliver personalized assistance, thereby enhancing operational efficiency and customer satisfaction.
Sentiment Analysis
Sentiment analysis emerges as a valuable application of natural language processing, allowing organizations to extract, quantify, and interpret emotions, opinions, and attitudes expressed within textual data. The fundamental characteristic of sentiment analysis revolves around its capacity to gauge public sentiment towards products, services, brands, or events, enabling businesses to evaluate market perceptions, sentiment trends, and brand sentiment. By harnessing sentiment analysis, organizations can gain valuable insights into customer feedback, market trends, and competitive landscapes, empowering strategic decision-making, reputation management, and customer relationship management initiatives.
Challenges and Considerations in Leveraging Big Data for AI
When navigating the complex realm of big data and artificial intelligence (AI) intersection, it is imperative to address the crucial theme of challenges and considerations in leveraging big data for AI development. With the exponential growth of data volumes and the rapid advancements in AI technologies, organizations encounter a myriad of obstacles and strategic decisions in harnessing the potential of big data effectively for AI applications. This section delves deep into the intricate landscape of balancing data accessibility with stringent data privacy and security measures, ensuring compliance with regulations and mitigating ethical implications.
Data Privacy and Security
In the realm of big data and AI, data privacy and security stand as paramount concerns, shaping the ethical and legal foundations of data-driven decision-making processes. Regulatory compliance serves as a pivotal aspect in safeguarding sensitive data assets and ensuring transparency and accountability in AI systems. By aligning with stringent regulations and data protection laws, organizations can uphold the integrity of data usage, fostering trust with stakeholders and customers.
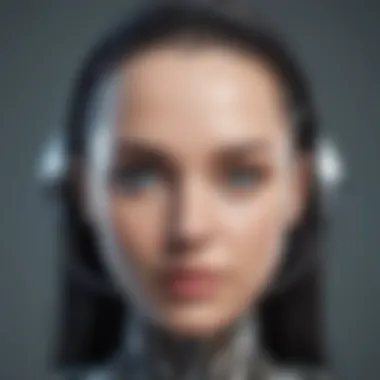
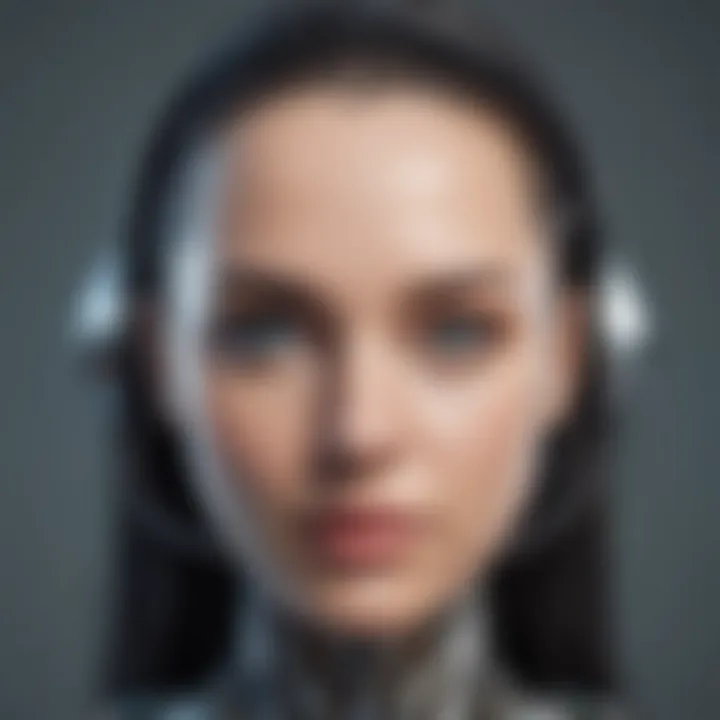
Regulatory Compliance
Regulatory compliance embodies the adherence to laws and standards governing data privacy and security practices within organizations. Its significance lies in aligning business operations with legal requirements and industry standards to mitigate risks associated with data breaches and misuse. By implementing robust compliance frameworks, businesses can enhance data governance practices, minimize legal liabilities, and fortify data security protocols, ultimately fostering a culture of responsible data stewardship.
Ethical Implications
Ethical implications in big data and AI underscore the moral considerations and societal impacts of algorithmic decision-making processes. As AI algorithms influence various aspects of human life, prioritizing ethical practices becomes fundamental in mitigating biases and ensuring fairness in AI-driven decisions. By proactively addressing ethical dilemmas and promoting transparency in AI algorithms, organizations can cultivate an ethical AI culture that prioritizes integrity, equity, and accountability.
Future Trends in Big Data and AI Innovation
As we journey into the realm of Future Trends in Big Data and AI Innovation, we are confronted with a landscape defined by remarkable advancements and potential disruptions. The evolving nature of technology necessitates a keen awareness of the trends shaping our digital future. In this section, we will delve into pivotal aspects of this intricate tapestry, illuminating the significance of embracing innovation to stay ahead in a dynamic tech ecosystem. Our exploration will unravel nuanced insights, strategic considerations, and implications for industries at large.
Edge Computing and AI
In the rapidly evolving digital ecosystem, the integration of Edge Computing and AI emerges as a cornerstone of technological progress. The synergy between these domains ushers in a new era of efficiency, scalability, and real-time decision-making capabilities.
IoT Integration
Within the domain of IoT Integration lies a profound narrative of interconnected devices and data streams. This integration bolsters the efficiency of AI systems by facilitating seamless communication and data exchange across a myriad of interconnected devices. The prowess of IoT Integration lies in its ability to bridge the gap between physical and digital realms, enabling organizations to harness the power of real-time data for informed decision-making. While offering unmatched connectivity and data access, IoT Integration also presents challenges in terms of data security and scalability.
Real-Time Processing
Real-Time Processing stands at the forefront of transformative technological innovation, empowering organizations to process vast data streams instantly. Its pivotal role in enabling real-time decision-making and analytics cultivates a competitive edge for businesses striving for agility and responsiveness. The key characteristic of Real-Time Processing lies in its ability to process data without delays, ensuring timely insights for crucial decision-making processes. However, the complexity and resource-intensive nature of real-time processing pose challenges in implementation and scalability, demanding strategic considerations for optimal utilization.
Explainable AI
The evolution of AI algorithms has brought forth the imperative need for transparency and interpretability in decision-making processes. In this exploration of Explainable AI, we dissect the importance of fostering trust and understanding in AI systems to enhance their societal impact and ethical integrity.
Interpretable Models
At the core of Explainable AI lies the concept of Interpretable Models, where transparency and comprehensibility reign supreme. These models prioritize clarity by enabling stakeholders to decipher the inner workings of AI algorithms, fostering trust and accountability in decision-making processes. The recognizable attribute of Interpretable Models lies in their ability to provide clear and concise explanations for AI outcomes, allowing for informed understanding and validation. Despite their advantages in promoting trust and reliability, Interpretable Models may encounter challenges in handling complex datasets and compromising on model accuracy.
Transparency in AI Decisions
Transparency in AI Decisions acts as a guiding light in navigating the intricate landscape of AI ethics and accountability. By promoting openness and visibility in the decision-making processes of AI systems, transparency instills trust and confidence among users and stakeholders. The quintessential feature of Transparency in AI Decisions lies in its ability to demystify the black box of AI algorithms, enabling stakeholders to comprehend the rationale behind AI-driven decisions. While it fosters ethical AI practices and user acceptance, transparency may also raise concerns regarding data privacy and intellectual property protection.
Ethical AI Development
As technology pervades every facet of our lives, the ethical implications of AI development take center stage. This section delves into the ethical considerations that underpin responsible AI practices and the critical importance of building accountability mechanisms within AI systems.
Responsible AI Practices
Responsible AI Practices embody the ethical compass guiding the development and deployment of AI technologies. These practices underscore the significance of integrating ethical principles and societal values into AI algorithms, ensuring fairness and transparency in their operation. The distinctive feature of Responsible AI Practices lies in their emphasis on advocating for unbiased decision-making and mitigating the impact of algorithmic biases. While promoting ethical norms and societal well-being, responsible AI practices may encounter challenges in balancing ethical standards with business objectives and operational constraints.
Accountability in AI Systems
At the crux of Ethical AI Development lies the cornerstone of Accountability in AI Systems, where transparency meets liability in the realm of AI governance. Accountability mechanisms hold AI systems to task, ensuring that decisions are made accountably and ethically. The pivotal characteristic of Accountability in AI Systems lies in its ability to attribute responsibility for AI-generated outcomes, fostering trust and reliability among users and stakeholders. While accountability mechanisms strengthen ethical frameworks and compliance standards, they may also pose challenges in defining clear accountability boundaries and determining liability in complex AI ecosystems.