Unlocking the Power of Predictive Analytics: Types for Strategic Decision-Making
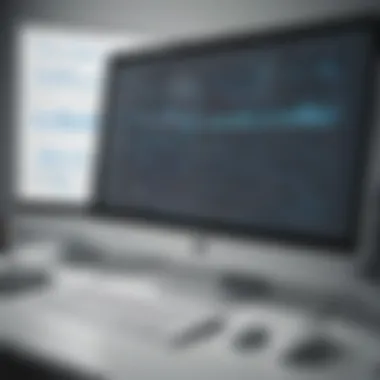
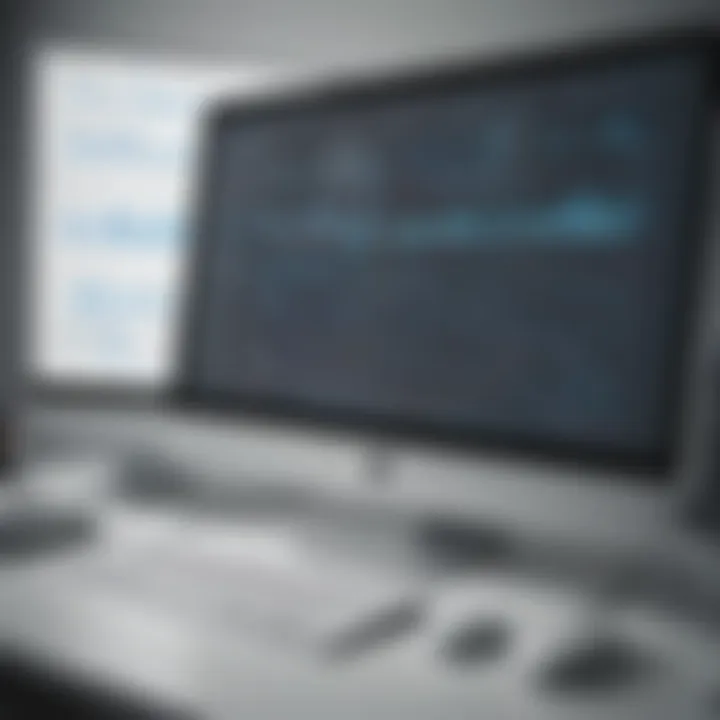
Understanding Predictive Analytics Types
Predictive analytics delves into various types that are pivotal in transforming data into actionable insights. Each type, from descriptive to prescriptive analytics, plays a unique role in driving informed decision-making to gain a competitive edge in the data-driven landscape.
Descriptive Predictive Analytics
Descriptive predictive analytics focuses on understanding past data to gain insights into what has happened. By utilizing historical information, organizations can identify patterns and trends, laying a solid foundation for future predictive modeling.
Diagnostic Predictive Analytics
Diagnostic predictive analytics goes beyond describing historical data and aims to identify the reasons behind certain outcomes. This type helps organizations pinpoint the root causes of specific events or trends, enabling them to make strategic decisions based on a deeper understanding of the underlying factors.
Predictive Modeling
Predictive modeling involves using statistical algorithms and machine learning techniques to forecast future outcomes based on historical data. By creating predictive models, organizations can anticipate trends, behavior, and potential risks, allowing for proactive decision-making and risk mitigation strategies. This analytical approach empowers businesses to make data-driven decisions with a higher degree of accuracy and confidence.
Prescriptive Analytics
Prescriptive analytics focuses on providing recommendations for action rather than just predicting outcomes. By leveraging advanced algorithms and decision-making frameworks, prescriptive analytics offers organizations actionable insights to optimize processes, enhance performance, and drive innovation. This type of analytics empowers decision-makers with informed choices, enabling them to take proactive steps to improve business outcomes.
Conclusion
Exploring predictive analytics types unveils a diverse range of tools and methodologies that organizations can leverage to extract actionable insights from their data. Understanding the nuances of each type - descriptive, diagnostic, predictive modeling, and prescriptive analytics - is crucial for making informed decisions, mitigating risks, and gaining a competitive edge in today's dynamic and data-centric business environment.
Introduction to Predictive Analytics
Predictive analytics forms the backbone of decision-making processes in modern business landscapes, offering the promise of deciphering data to unveil actionable insights. In this article, we unravel the significance of embracing predictive analytics, shedding light on its pivotal role in enhancing decision-making capabilities. By dissecting key elements such as predictive models and algorithms, we aim to equip readers with a profound understanding of leveraging data for strategic advantage.
Understanding Predictive Analytics
Definition and Purpose
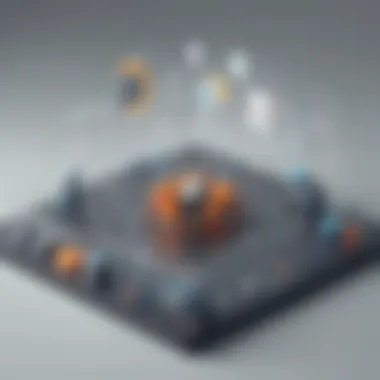
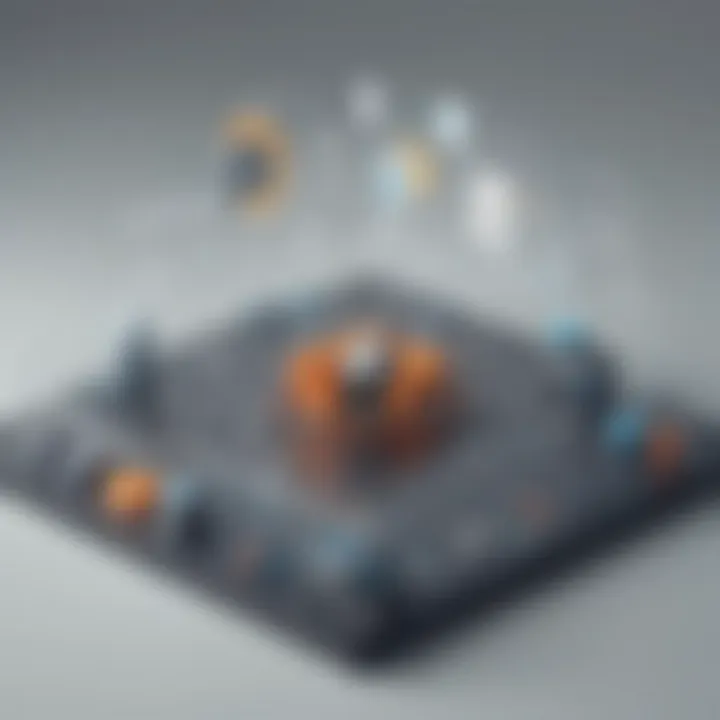
Delving into the core of predictive analytics, its definition, and purpose prove to be instrumental in grasping its essence in this article. The sheer essence of predictive analytics lies in its ability to forecast future trends based on historical data patterns. This methodology serves as a compass for organizations, guiding them towards informed decisions and strategic maneuvers. The inherent capability of predictive analytics to predict outcomes with a notable degree of accuracy stands out as a significant advantage in modern data-driven settings.
Role in Business Operations
The role of predictive analytics within business operations extends beyond mere data analysis; it serves as a catalyst for organizational growth and efficiency. By harnessing the power of predictive analytics, businesses can streamline operations, identify trends, and anticipate customer demands, thereby gaining a competitive edge in the market. However, inherent limitations, such as data complexities and model accuracy, pose challenges that necessitate meticulous consideration.
Benefits of Predictive Analytics
Unveiling the benefits of predictive analytics unlocks a world of opportunities for organizations striving to stay ahead of the curve. From enhanced decision-making processes to optimized resource allocation, the advantages of predictive analytics are multifaceted. Embracing predictive analytics empowers enterprises to leverage data effectively, fostering innovation, and driving sustainable growth in a dynamic business environment.
Key Concepts
Data Mining
Data mining serves as the cornerstone of predictive analytics, enabling organizations to extract valuable insights from large datasets. The essence of data mining lies in its ability to uncover hidden patterns and relationships, laying the foundation for predictive modeling. Leveraging data mining techniques equips businesses with the tools necessary to make data-driven decisions and gain a competitive advantage in the market.
Machine Learning
At the heart of predictive analytics, machine learning algorithms play a pivotal role in processing data and deriving meaningful insights. Machine learning algorithms empower predictive models to adapt to new data inputs, enhancing their predictive accuracy over time. While the scalability and efficiency of machine learning algorithms bolster decision-making processes, ensuring their interpretability remains a challenge worth addressing.
Regression Analysis
Regression analysis emerges as a quintessential tool within predictive analytics, offering a systematic approach to understanding and predicting relationships between variables. By modeling these relationships, regression analysis enables organizations to make informed decisions based on historical data trends. Despite its efficacy in forecasting future outcomes, the complexity of regression analysis warrants a nuanced approach to ensure accurate predictions.
Applications of Predictive Analytics
Marketing and Sales
In the realm of marketing and sales, predictive analytics revolutionizes customer engagement strategies and revenue optimization. By segmenting target audiences, predicting consumer behavior, and optimizing marketing campaigns, organizations can cultivate lasting customer relationships and drive business growth. However, ethical considerations surrounding data privacy and algorithm bias underscore the importance of responsible predictive analytics implementation.
Financial Forecasting
Financial forecasting stands as a cornerstone application of predictive analytics, enabling financial institutions to mitigate risks and seize opportunities proactively. By analyzing historical financial data and market trends, organizations can forecast future financial scenarios, optimize investment strategies, and enhance fiscal resilience. Nevertheless, the interpretability of financial forecasting models remains a critical concern, necessitating transparency and accountability in decision-making processes.
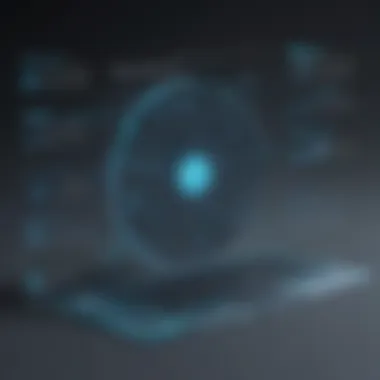
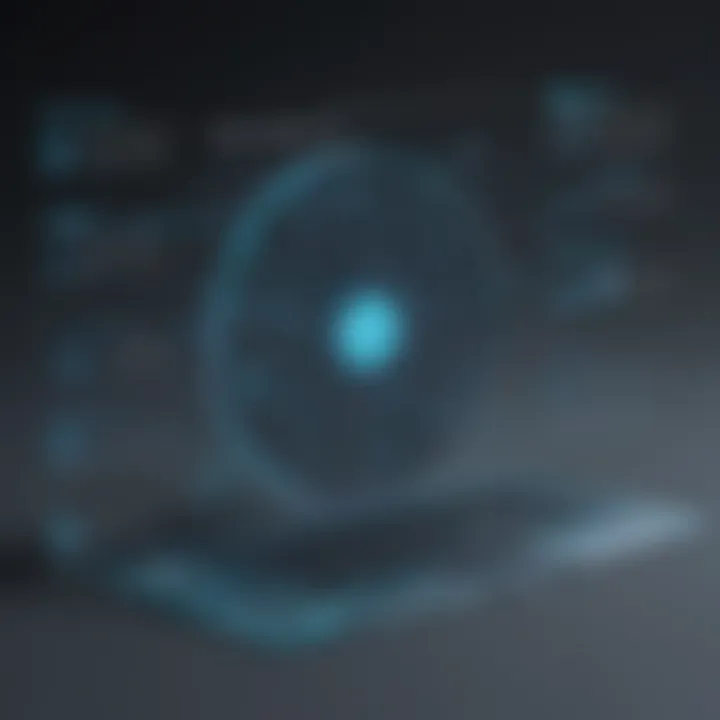
Risk Management
Assessing and mitigating risks lies at the heart of effective risk management, a domain where predictive analytics shines. By identifying potential risks, quantifying their impact, and developing mitigation strategies, organizations can safeguard their operations and investments from unforeseen uncertainties. While predictive analytics empowers risk managers with data-driven insights, addressing algorithmic biases and ensuring model accuracy are paramount to effective risk management practices.
Types of Predictive Analytics
Predictive analytics serves as a critical component in transforming raw data into actionable insights, empowering organizations to make informed decisions essential for gaining a competitive advantage in today's data-centric environment. Understanding the various types of predictive analytics is crucial for maximizing the potential of data-driven decision-making processes. Each type, ranging from descriptive to prescriptive analytics, offers distinct capabilities that organizations can leverage to extract valuable insights from their data. By exploring the different types of predictive analytics, businesses can unlock new perspectives, optimize operations, and enhance strategic planning.
Descriptive Analytics
Overview and Function
Descriptive analytics plays a pivotal role in providing a snapshot of past data trends and performance, enabling organizations to understand historical patterns and behaviors. By summarizing and aggregating data, descriptive analytics offers valuable insights into what has happened within a specific timeframe. Its primary function is to describe past occurrences, allowing stakeholders to identify trends, outliers, and anomalies that may influence future decision-making. Despite its retrospective nature, descriptive analytics serves as a foundational element for more advanced analytical processes by establishing baseline performance metrics and highlighting areas of interest for further investigation.
Incorporating descriptive analytics into the decision-making process provides organizations with a comprehensive understanding of historical data, facilitating trend analysis, performance evaluation, and benchmarking against key performance indicators. By leveraging descriptive analytics, businesses can gain valuable insights into past behaviors, patterns, and outcomes, enabling them to make data-driven decisions informed by historical context and trends. While descriptive analytics excels in providing historical summaries and performance indicators, its main limitation lies in its inability to offer predictive or prescriptive insights, focusing solely on past data observations.
Tools and Techniques
Various tools and techniques are available for implementing descriptive analytics, ranging from simple data visualization platforms to more advanced statistical software. Data visualization tools such as Tableau, Power BI, and Qlik Sense enable organizations to create interactive dashboards, charts, and graphs that illustrate historical trends and patterns in a visually engaging format. These tools allow users to explore data intuitively, identify correlations, and interpret complex relationships within datasets.
Statistical software packages like R, Python, and SAS empower data scientists and analysts to perform in-depth statistical analysis, descriptive statistics, and data mining operations. These tools facilitate the extraction of key insights from large datasets, enable trend analysis, and support decision-making processes based on historical data patterns. Leveraging a combination of data visualization and statistical tools, organizations can optimize their descriptive analytics processes, uncover actionable insights, and enhance data-driven decision-making capabilities.
Challenges and Considerations in Predictive Analytics
Predictive analytics is a complex field that requires a deep understanding of various challenges and considerations to maximize its benefits. In the context of this article, exploring the challenges and considerations in predictive analytics is crucial for IT professionals, cybersecurity experts, and students seeking to leverage data for informed decision-making. By delving into the obstacles and opportunities present in the realm of predictive analytics, individuals can gain a nuanced perspective on how to navigate the data landscape effectively and ethically.
Data Quality and Integration
Data Cleaning Processes:
Data cleaning processes are fundamental in ensuring the accuracy and reliability of the datasets used in predictive analytics. Within the scope of this article, focusing on data cleaning processes sheds light on the meticulous steps involved in detecting and correcting errors, inconsistencies, and missing values in the data. The key characteristic of data cleaning lies in its ability to enhance data integrity and eliminate any discrepancies that could skew analytical outcomes. While time-consuming, data cleaning processes are a necessary investment to ensure the credibility and trustworthiness of predictive analytics results.

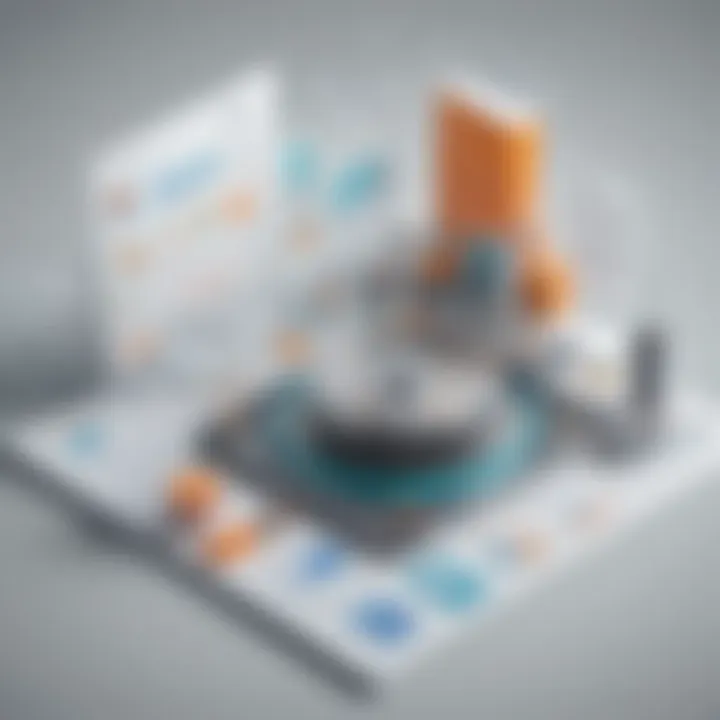
Data Sources Verification:
Data sources verification plays a pivotal role in corroborating the authenticity and relevance of the data used for predictive analytics. By scrutinizing the sources of data and evaluating their reliability, stakeholders can mitigate the risk of basing decisions on erroneous or outdated information. The unique feature of data sources verification is its capacity to enhance the transparency and traceability of data inputs, allowing for greater confidence in the analytical outputs. Despite the additional effort required, data sources verification acts as a safeguard against data inaccuracies and reinforces the credibility of predictive analytics findings.
Model Accuracy and Interpretability
Validation Techniques:
Validation techniques are essential tools for assessing the accuracy and robustness of predictive models in real-world scenarios. In the context of this article, emphasizing validation techniques illuminates the methods used to test and verify the predictive capabilities of algorithms against historical or unseen data. The key characteristic of validation techniques is their ability to validate model performance and generalize results to new datasets, ensuring reliability and applicability across different contexts. While demanding in terms of computational resources, validation techniques are imperative for establishing the credibility and effectiveness of predictive analytics models.
Explainable AI:
Explainable AI refers to the capability of artificial intelligence systems to provide transparent and understandable rationale behind their decisions. Within the framework of this article, highlighting explainable AI underscores the importance of interpretability and accountability in predictive analytics. The unique feature of explainable AI lies in its capacity to elucidate complex machine learning processes and foster trust among users and decision-makers. Despite the interpretive challenges involved, explainable AI enhances the interpretability and acceptance of predictive analytics models, fostering greater user confidence and adoption.
Ethical and Privacy Concerns
Algorithm Bias:
Algorithm bias signifies the partiality or discrimination inherent in algorithms that can lead to skewed or unfair outcomes. By addressing algorithm bias in this article, we recognize the ethical implications of biased algorithms and their impact on decision-making processes. The key characteristic of algorithm bias is its potential to perpetuate discrimination and reinforce existing inequalities if left unchecked. Mitigating algorithm bias is essential for promoting fairness and equity in predictive analytics applications, safeguarding against adverse consequences and ensuring ethical integrity.
User Data Protection:
User data protection encompasses the measures and practices aimed at safeguarding individual privacy and confidentiality in data-driven processes. In the context of this article, focusing on user data protection underscores the importance of upholding data privacy regulations and ethical standards. The unique feature of user data protection lies in its emphasis on transparency, consent, and data security to prevent unauthorized access or misuse of personal information. While demanding stringent compliance measures, user data protection is indispensable for mitigating risks, building consumer trust, and upholding ethical principles in predictive analytics initiatives.
Future Trends in Predictive Analytics
Predictive analytics is a dynamic field that continuously evolves, making it crucial to grasp the future trends. Anticipating changes in the landscape helps stakeholders stay ahead. The importance of future trends in predictive analytics lies in their ability to provide insights into upcoming developments. Understanding these trends enables businesses to adapt strategies proactively, enhancing competitiveness. It offers opportunities for innovation and optimization of processes. By being mindful of emerging trends, organizations can leverage predictive analytics effectively.
AI Advancements
Artificial Intelligence (AI) advancements play a pivotal role in shaping the future of predictive analytics. Within the realm of AI advancements, Deep Learning Innovations stand out prominently. Deep Learning focuses on training algorithms to learn by example, mimicking the way human brains operate. This approach allows for more intricate analyses and pattern recognition, leading to enhanced predictive models. While Deep Learning requires significant computational resources, its effectiveness in handling complex data patterns is unparalleled. Despite its computational intensity, the ability of Deep Learning to uncover hidden insights makes it indispensable for predictive analytics strategies. AI-Driven Decision-Making is another aspect revolutionizing predictive analytics. Integrating AI into decision-making processes enhances efficiency and accuracy. AI enables rapid analysis of vast datasets, empowering organizations to make informed decisions promptly. The key characteristic of AI-Driven Decision-Making is its capacity to handle large volumes of data swiftly, providing real-time insights for strategic actions. However, the reliance on AI may lead to potential biases in decision-making if not cautiously monitored. Balancing the automation provided by AI with human judgment is vital in ensuring optimal outcomes.
Industry Adoption
The integration of predictive analytics in various sectors marks a significant trend in the industry. With the proliferation of data-driven decision-making, businesses across diverse domains are capitalizing on the benefits of predictive analytics. Integration in Various Sectors ensures that insights derived from data analytics are integrated seamlessly into existing workflows. This integration streamlines operations, enhances productivity, and fosters innovation within organizations. The key characteristic of this trend is the customization of predictive analytics solutions to suit specific sector requirements. Tailoring predictive analytics tools to industry-specific needs maximizes their effectiveness and utility. However, challenges may arise in implementing predictive analytics across different sectors due to variations in data sources and operational processes. Predictive Analytics as a Competitive Advantage is a game-changer for businesses aiming to outperform competitors. Leveraging predictive analytics not only improves decision-making but also positions organizations strategically in the market. The key characteristic of this trend is the use of predictive analytics to gain insights that offer a competitive edge. By utilizing predictive analytics effectively, businesses can forecast market trends, anticipate customer behavior, and optimize resource allocation. Despite its advantages, maintaining a competitive advantage through predictive analytics requires continuous refinement and adaptation to changing market dynamics.
Technological Developments
Automation and Scalability are pivotal advancements driving the efficacy of predictive analytics. Automation simplifies repetitive tasks involved in data analysis, allowing analysts to focus on strategic initiatives. The key characteristic of Automation and Scalability is their ability to streamline processes and enhance efficiency in handling large datasets. The scalability of predictive analytics solutions ensures they can accommodate growing data volumes and evolving business needs. While automation boosts productivity, it is essential to balance it with human oversight to prevent errors in decision-making. Cloud-Based Predictive Solutions offer flexibility and accessibility, revolutionizing the way predictive analytics is deployed. With the shift towards cloud computing, predictive analytics tools are increasingly being offered as cloud-based solutions. The key characteristic of cloud-based predictive solutions is their scalability and cost-effectiveness. Utilizing cloud infrastructure minimizes the need for extensive IT resources, making predictive analytics more accessible to organizations of all sizes. However, concerns surrounding data privacy and security in the cloud remain pertinent, urging stringent measures to safeguard sensitive information.