Exploring Image Identification Software and Its Impact
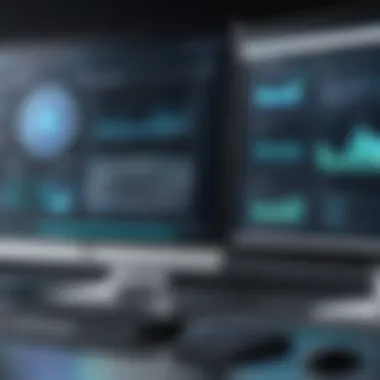
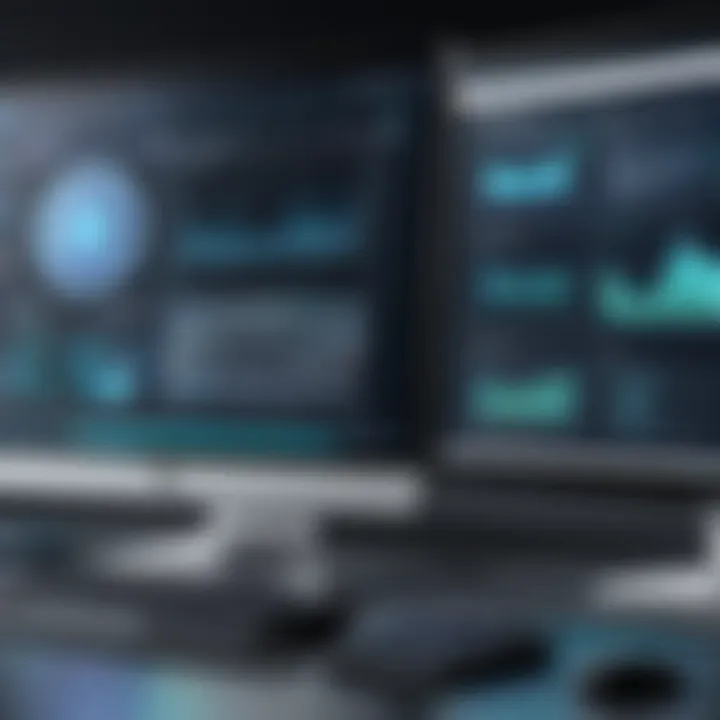
Intro
In today’s fast-paced digital age, the ability to recognize and identify images automatically has transformed various fields from security to retail. The deployment of image identification software is at an all-time high, revealing a fantastic convergence of technology and necessity. This piece aims to break down the complex systems at play, offering a framework for understanding how they function, where they’re applied, and the implications of their rapid evolution.
Understanding Image Identification Systems
A Glimpse into Functionality
Image identification systems leverage advanced algorithms to process and analyze visual data. They function on the principle of machine learning, enabling technology to learn from vast amounts of graphics. It’s a form of artificial intelligence that trains on datasets comprising millions of labelled images, allowing it to recognize patterns and features that define specific objects or entities.
Applications Across Industries
These software systems are not one-size-fits-all; they find applications in numerous sectors. Here’s a short list that illustrates their diverse utility:
- Security: Surveillance systems employ image recognition to detect unwanted intruders or identify persons of interest.
- Healthcare: Medical imaging utilizes these technologies to assist in diagnosing conditions from scans.
- Retail: Stores apply these systems to analyze foot traffic and improve customer experience by understanding behavior patterns.
- Social Media: Platforms automate tagging photos based on user interactions, enhancing user experience in a big way.
Ethical Considerations
While the applications are broad, they do not come without concerns. The ethical implications of image identification software demand scrutiny. Issues such as privacy invasion and data misuse have emerged amid the advancements. As the technology matures, finding a balance between innovation and ethical conduct will surely become a pressing challenge.
Industry Trends and Future Outlook
Current Developments and Trends
Several trends are shaping the future of image identification software. Companies are increasingly integrating these systems with other technologies like augmented reality and predictive analytics. This integration not only enhances functionality but also broadens the scope of applications. For instance, imagine a shopping app that allows you to take a picture of a dress and receive instant outfit recommendations!
Prospective Innovations
Looking forward, it’s plausible that enhanced algorithms will lead to even faster and more accurate identification processes. The integration of neural networks will likely push boundaries, making the software smarter and more user-friendly. As voice and facial recognition technology also continues to evolve, the potential for multi-modal applications is burgeoning—dizzying to think about!
Finale
Image identification software stands as a pillar of modern technology, with implications stretching far beyond mere recognition. Its ability to integrate seamlessly into various industries underscores its importance. Balancing technological advancements with ethical considerations will be key as we venture further into this fascinating realm. This dynamic field continues to evolve, requiring a keen awareness of trends, changes, and impacts on society at large.
Preface to Image Identification Software
Navigating the digital landscape of today, it's hard to overlook the significance of image identification software. This segment serves as the launchpad for understanding how modern technologies intertwine with visual recognition. From the outset, we need to grasp the fundamentals that underpin this software, which is becoming increasingly prevalent across various sectors like security, retail, and healthcare. The relevance of image identification is not merely confined to technical prowess; it's deeply rooted in its practical applications and the way it affects our daily lives.
The essence of image identification software lies in its capability to analyze and interpret visual data. Imagine snapping a picture and, within seconds, receiving information about the objects, people, or environments captured. The technology is not just about identifying simple elements; it incorporates complex algorithms to differentiate nuances in images. With the accelerating pace of technological advancements, comprehending these elements is essential for professionals who wish to stay informed and relevant.
Understanding Image Identification
Image identification involves a mix of technology and science, resulting in the ability to automate the recognition of visual information. This function is grounded in its practical application. For example, a common illustration would be Facebook's facial recognition software—tapping into vast databases to suggest tags as one uploads photos. At its core, image identification utilizes algorithms that can learn and adapt over time, enhancing their performance through training.
The mechanism of this process often combines several techniques, including machine learning, deep learning, and image feature extraction. These terms may sound complex, but they boil down to the ability of systems to learn from data, recognize patterns, and apply that knowledge to new images. This ongoing evolution ensures that image identification remains at the forefront of technological development.
The Importance of Visual Recognition
Visual recognition holds substantial weight in a digitized world that inundates users with images daily. The importance of this capability cannot be overstated. At the heart of visual recognition is the ability to make sense of a chaotic environment. Consider how critical it is for e-commerce platforms to accurately identify products. When users search for items based on images, the underlying software not only speeds up the shopping process but also enhances user experience significantly.
Furthermore, in security applications, visual recognition systems are pivotal. They can monitor spaces with far greater efficiency than human observers, detecting unusual behavior or identifying individuals with precision. Whether it's a retail store employing surveillance technology or a public area focused on crowd safety, the implications of visual recognition are vast.
In a nutshell, the ability to recognize and interpret visual data shapes numerous industries, establishing a need for ongoing research and innovation. As we delve deeper into this topic, keep in mind the multifaceted nature of image identification software and its profound impact on our society.
"Understanding image identification software is not just for tech enthusiasts; it's a trait anyone engaged in modern industries should have."
By unpacking the technical aspects in the sections to come, we will better appreciate how this technology operates, its applications, and the challenges it faces.
Historical Context of Image Recognition Technologies
Understanding the historical context of image recognition technologies is essential to grasp the landscape of modern visual identification systems. The evolution of image identification software reveals not only technological advancements but also the changing mindset towards visual data. Knowing the roots allows us to appreciate the current technological marvels and anticipate future developments. Furthermore, it aids in understanding the challenges and ethical concerns that have emerged alongside these innovations.
Early Developments in Image Processing
The journey of image processing began long before the advent of powerful computers. In the 1960s, researchers began to explore digital images. One noteworthy breakthrough was the development of algorithms that could analyze the brightness of pixel values. This marked the first encounter with the digitization of images. Simple techniques like edge detection emerged, paving the way for more sophisticated methods.
During these early years, the capabilities were limited, with significant challenges in computing power and storage. But the researchers of that time pushed boundaries. They utilized methods like the Fourier transform to convert images into frequency domains, allowing for analysis that was previously unimaginable.
As technology advanced, the 1980s introduced more comprehensive image analysis tools. Concepts like pattern recognition began to take hold, especially in academic circles. Researchers applied machine learning and statistical techniques to images, fostering a new appreciation for computers’ role in image understanding. With the advent of more robust databases in the 90s—think of large image libraries—progress became exponential. However, the capabilities were still far from what we see today.
Milestones in Image Identification
Taking a step back and looking at the key milestones in image identification helps contextualize current technologies. The introduction of neural networks in the late 1980s was a game-changer. Although it took time for them to gain traction, the realization of their potential eventually led to significant innovations.
A turning point came with the development of ConvNets, or convolutional neural networks, in the 1990s by Yann LeCun. This architecture enabled the software to automatically learn features of images, drastically improving accuracy over traditional methods. As computational power increased in the 2000s, deep learning became the new buzzword in the tech space. Companies invested heavily in research to create more efficient models.
These developments did not merely enhance accuracy; they also increased the versatility of image recognition. Applications began to pop up in diverse areas such as security, healthcare, and retail. For instance, technology used in facial recognition became prevalent, changing not just security measures but raising debates around privacy.
"Understanding the evolution of image identification software not only reveals how far we've come but also how much more we have yet to learn."
Despite all these advancements, pitfalls remained. Misclassification issues, bias in algorithms, and ethical concerns surrounding privacy highlighted the need for responsible development. As we stand on the shoulders of past giants, we must also be mindful of the lessons learned, ensuring that future technologies are developed with integrity.
In summary, a thorough grasp of the historical context of image identification technologies serves as a foundation for understanding their impact in today’s rapidly changing tech landscape. The path paved by early developments and major milestones signifies the blend of human ingenuity and technological advancements that continues to shape our visual recognition capabilities.
Technical Aspects of Image Identification Software
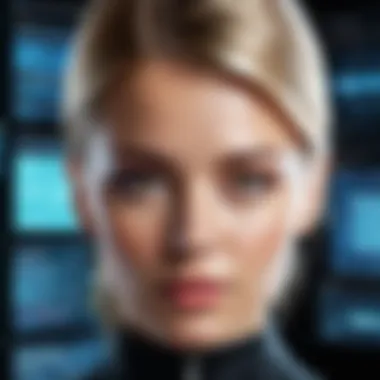
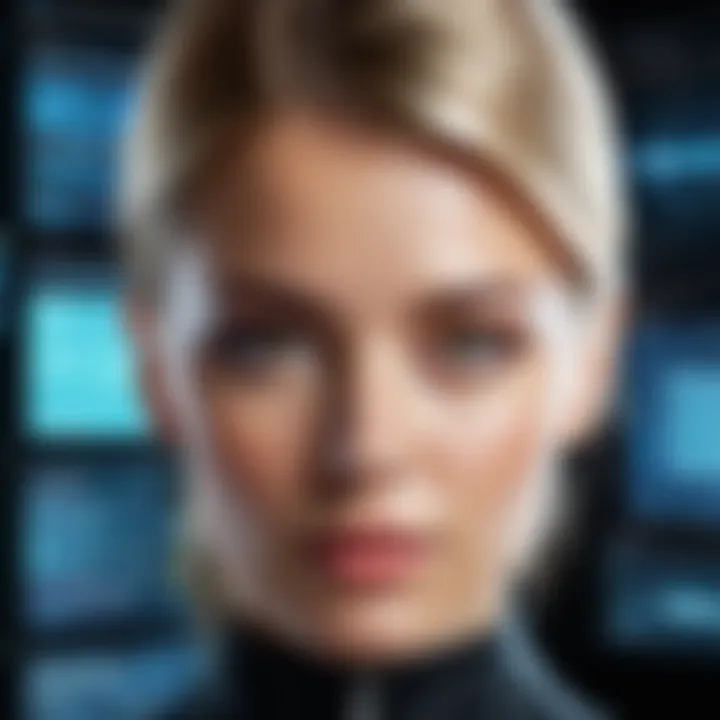
Understanding the technical aspects of image identification software is like peeling back the layers of an onion. Each layer reveals critical components that enhance functionality and improve performance. These aspects are crucial for ensuring that the software can effectively identify, categorize, and interact with visual data. From algorithms that power recognition to the training methods that ensure accuracy, these elements are the backbone of how such software operates in the real world.
Algorithms Behind Image Recognition
Machine Learning Techniques
Machine Learning Techniques form the foundation of many image recognition systems. These techniques enable software to learn from data, evolve, and make accurate predictions without being explicitly programmed for each scenario. A key characteristic of these techniques is their ability to adapt based on new information. As more images are processed, the system improves its accuracy through experience, much like how humans learn.
A unique feature of Machine Learning is its flexibility. For instance, a system trained with thousands of images can distinguish between thousands of categories just by adjusting its parameters. This adaptability makes it a popular choice for image identification, yet it does come with some challenges.
On the downside, the requirement for large datasets can be a hurdle. If the images used for training are biased or lack diversity, the results can be skewed, leading to inaccuracies.
Deep Learning and Neural Networks
Moving up the ladder, Deep Learning and Neural Networks take the game to another level. These algorithms exploit vast amounts of data to find intricate patterns and features in images. A key characteristic of Neural Networks is their multi-layered architecture, where each layer processes information in increasingly abstract ways.
What sets Deep Learning apart is its ability to perform feature extraction automatically, eliminating the need for manual feature selection. In this way, it stands as a powerful tool for complex datasets that other traditional methods might struggle with. However, one must not overlook the computational intensity required. Deep Learning can be resource-heavy, necessitating high-performance GPUs and long training times, which may be a barrier for some.
Image Feature Extraction
Feature Extraction is another noteworthy aspect of image identification software. It involves identifying and quantifying specific attributes of images, like edges or textures, to aid the recognition process. A key characteristic of image feature extraction is its focus on reducing dimensionality while retaining essential information. This means that the system can process images much faster and more efficiently.
The unique feature here is that with effective feature extraction, the algorithm doesn't need to sift through entire images to make identifications, streamlining the process. While this can improve speed and efficiency, it also introduces a risk: if critical features are overlooked during extraction, there might be significant gaps in recognition accuracy.
Data Training and Model Accuracy
Data training is at the heart of any image identification software. The effectiveness of algorithms is significantly impacted by how accurately they can learn from data inputs. Quality training data is paramount; without a solid dataset, even the most sophisticated algorithms can falter.
In this context, model accuracy refers to how well the software can make correct predictions based on its training. Poorly structured training data can lead to high error rates and unreliable outputs. Moreover, regular validation and testing are essential practices to maintain model accuracy over time. This ensures that the software adapts to new trends and changes within the data it processes.
In summary, the technical aspects of image identification software are interlinked in complex ways. Each element plays a crucial role in enhancing the overall effectiveness and reliability of the system. Understanding these components can provide deeper insights into how image identification is revolutionizing various industries.
Applications of Image Identification Software
The application of image identification software cuts across numerous sectors, making it a pivotal aspect of modern technology. Its ability to analyze and interpret visual data has revolutionized various industries, enhancing efficiency and broadening possibilities. In this segment, we’ll dive deep into different realms where image identification software is making waves and what it means for the future.
Use in Security and Surveillance
Security and surveillance stand out as significant fields utilizing image identification software. With the surge in urbanization and security concerns, this technology provides solutions for monitoring environments in real-time. Methods such as facial recognition and behavior analysis help authorities manage public safety. For instance, in metropolitan areas, video feeds can be scanned for known offenders, and alerts can be triggered immediately. This proactive approach not only improves response time but also enhances safety.
Such systems can automatically identify potential threats, aiding security personnel in making timely decisions. However, the use of this technology raises questions about privacy and potential misuse. Striking a balance between ensuring security and respecting the rights of individuals is paramount.
Impact on Retail and E-commerce
The retail industry has not been left behind, as image identification software transforms how consumers engage with products. Shoppers today expect a seamless experience, and visual recognition tools satisfy this demand by bridging the gap between the physical and digital spaces.
Visual Search Capabilities
Visual search capabilities allow consumers to take an image or use a photograph to find similar products online. This functionality streamlines the shopping process, making it more intuitive for customers. For example, platforms like Pinterest and Google Lens let users upload images to discover similar styles or products available for purchase.
One key characteristic of visual search is its accessibility. It makes browsing easier for individuals who may find textual searches cumbersome. The technology's unique attribute is its ability to recognize and filter products based on perceived attributes, enabling a more personalized shopping experience.
However, challenges persist, including ensuring accuracy and consistency in findings. Consumers can sometimes receive unrelated results, leading to frustration. Nevertheless, as algorithms evolve, these visual search tools will likely become an invaluable asset in e-commerce and retail.
Inventory Management
Inventory management is another area where image identification software shines. It simplifies the process of tracking stock levels and enhances operational efficiency, which is crucial for retailers. For example, using image recognition, companies can automate the reordering of stock by scanning shelves and identifying missing items. This tech leads to reduced human error and significant time savings.
The critical feature here is real-time data access. Retailers can monitor inventory levels instantly, preventing stockouts and overstock situations. With precise tracking, decisions regarding purchasing and promotions can be made more accurately based on actual data.
However, integrating such systems into existing workflows can pose obstacles. Initial setup and training are often resource-intensive. But the long-term benefits, like fine-tuning operations and boosting profits, usually outweigh these initial costs.
Role in Healthcare
In healthcare, the impact of image identification software cannot be overlooked. Here, it aids in diagnostics, equipment management, and patient monitoring. For instance, algorithms can analyze medical images to detect abnormalities such as tumors or fractures more accurately than the naked eye. These tools provide physicians with enhanced capabilities to diagnose and make timely decisions about patient care.
Additionally, tracking patient health records through imagery ensures more personalized care, as practitioners can monitor progress over time without manually sorting through paperwork. The integration of image identification in healthcare leads to improved patient outcomes, enhancing the overall efficacy of medical practices.
In summary, the applications of image identification software are vast and varied. From bolstering security to reshaping retail strategies and advancing healthcare, its role in society is ever-increasing. The ongoing challenges will certainly shape its evolution, allowing for more robust, innovative applications in the future.
"The capability to identify and analyze images intelligently is not just about technology; it’s about reshaping human experiences across industries."
Whether navigating complex surveillance environments, delighting online shoppers, or empowering medical professionals, image identification software has embedded itself deeply into the fabric of modern operational frameworks, highlighting its importance and necessity in today's digital age.
Challenges Faced by Image Identification Software
In the rapidly advancing field of image identification technology, several challenges can be quite significant for both developers and users. Understanding these issues sheds light on the real-world implications of using such software, revealing its limitations as well as its potential. The challenges of accuracy, reliability, and ethical considerations are crucial to the ongoing development and deployment of these systems. Addressing these challenges not only aids in creating better technology but also addresses societal concerns surrounding its usage.
Issues of Accuracy and Reliability
Accuracy and reliability stand as cornerstones for any image identification software. The functions of these programs hinge significantly on their capacity to correctly identify and classify images, and any hiccup in performance can have serious ramifications. Often, the models built on deep learning and machine learning rely on vast datasets, which can sometimes be flawed or insufficiently diverse. This lack can lead to misidentification or failure to recognize certain images altogether.
For instance, when a security system misidentifies a person due to a low-quality image or inadequate training data, it may lead to wrongful detainment. Additionally, reliability concerns arise when users expect consistent performance across various conditions. Factors such as lighting, angle, and occlusion can drastically affect the effectiveness of an application. Ensuring a system's accuracy across diverse environments is a task that requires continuous effort and sophistication in model training.
Data Privacy and Ethical Considerations
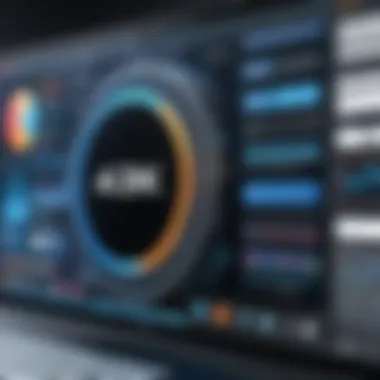
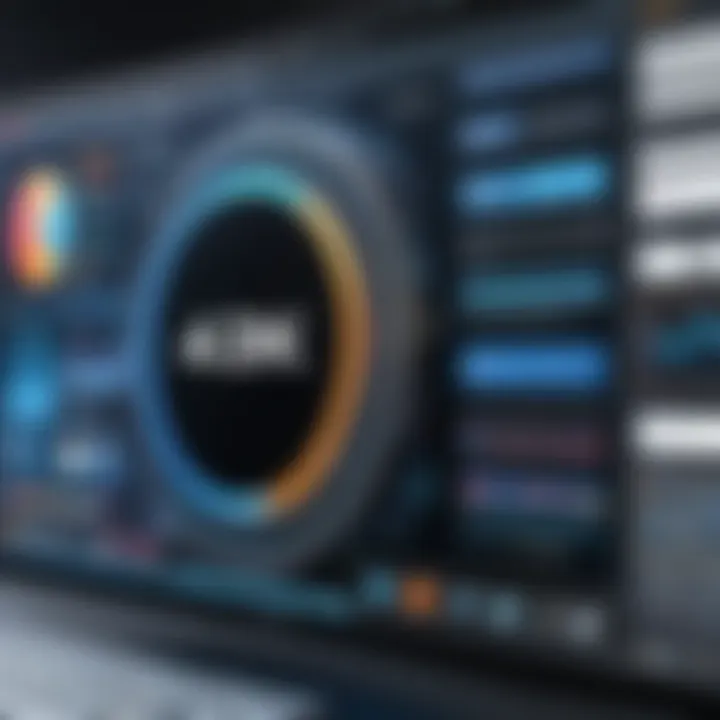
Surveillance Concerns
Surveillance concerns articulate a prominent aspect of the conversation surrounding image identification software. In an era where monitoring capabilities have surged, the implications for privacy have become a hotbed for debate. The act of identifying individuals in public spaces raises important questions about the balance between security and individual rights. This technology can lead to mass surveillance, where citizens feel they are constantly watched, prompting a movement towards advocating for stricter regulation and oversight.
The key characteristic of surveillance concerns is the potential for misuse. While the technology might aim to enhance safety, its application can easily cross into invasive territory. For instance, programs used by governments to track citizens can snowball into a situation where the lines between protection and privacy become blurred. Hence, understanding the unique feature of this concern, which is its dual-use potential, becomes essential in discerning how to responsibly deploy image recognition software.
Bias in Algorithms
Bias in algorithms presents another dimension of challenges that cannot be ignored. Algorithms are not created in a vacuum; they are susceptible to the inherent biases present in their training data. When a dataset historically underrepresents a specific demographic or circumstance, the algorithm may fail to recognize or inaccurately categorize individuals belonging to that group. This can lead to serious implications, especially in critical areas such as law enforcement and recruitment.
The key characteristic of bias in algorithms is its pervasive nature. Often, developers may unintentionally introduce these biases due to a lack of awareness or oversight. The unique feature of this challenge lies in its implications for fairness and equity. Failing to address bias can perpetuate existing stereotypes and inequalities, potentially resulting in harmful outcomes for affected individuals. The call for transparency and accountability in how algorithms are developed and trained is becoming more apparent as society demands ethical considerations in technological advancements.
"As we weave these systems into the fabric of daily life, it is imperative we think critically about their implications."
In sum, drawing attention to the challenges faced by image identification software allows for a more nuanced understanding of its practical implications. The issues of accuracy and reliability, along with profound ethical considerations surrounding surveillance and bias, are fundamental to advancing the technology responsibly. Addressing these challenges is not just a technical hurdle; it’s paramount in fostering a more secure and equitable future.
The Future of Image Identification Software
As we step into a world that's increasingly relying on visuals, the future of image identification software appears more promising than ever. This technology, which allows machines to recognize and process images, holds significant implications for various sectors ranging from healthcare to security. Understanding the future of this software is vital as it shapes our interaction with technology and the data we generate.
Trends in Technology and Innovation
The landscape of image identification software is rapidly changing, driven by advancing technologies. Some of the most notable trends include:
- Enhanced Machine Learning Algorithms: Continuous improvement of machine learning techniques is pushing the bounds of what's possible in image recognition. Algorithms are becoming more adept at distinguishing subtle differences in visuals, leading to more accurate outcomes.
- Integration of Augmented Reality (AR): AR applications are increasingly utilizing image identification to overlay digital information on real-world images. This gives users real-time data that can enhance their understanding of surroundings.
- Real-time Processing: The demand for speed is ever-increasing. Future systems are expected to process images in real-time, enhancing the user experience. Think of how this could revolutionize everything from traffic management systems to public safety applications.
- Improved Accessibility: With the rise of smartphones and affordable technology, image identification software is becoming more accessible. This points to a future where these systems could be utilized by the masses, empowering more people to use visual recognition tools in daily tasks.
- Personalization: The software will not just recognize images but also learn user preferences over time, tailoring experiences based on individual needs and behaviors. Imagine a platform that identifies not just objects but also creates a customized visual feed based on your interests.
Potential Societal Impacts
The influence of image identification software transcends technology; it has profound societal implications:
- Security Enhancements: With improved accuracy in image recognition, security systems will become more efficient. This could enhance public safety in urban areas, with law enforcement agencies making use of real-time image analysis to monitor situations and respond faster.
- Healthcare Revolution: In the medical field, better diagnostic tools through image recognition can lead to early detection of diseases. E.g., software that identifies anomalies in medical imaging could save lives by facilitating timely medical interventions.
- Impact on Employment: As the technology advances, there's a possibility of job displacement in sectors relying heavily on manual image identification. However, it can also create opportunities for new roles that focus on managing, interpreting, and developing these technologies.
- Privacy Concerns: With the capability of tracking and identifying individuals from images, there arise significant privacy concerns. Society must engage in discussions about consent, data ownership, and ethical usage. The prospect of surveillance raises eyebrows, necessitating a balanced approach toward these advancements.
"The unfolding potential of image identification technology offers benefits that are tantalizing, yet it also demands careful scrutiny of ethical practices."
In summary, the future of image identification software is a double-edged sword, poised for remarkable advances while simultaneously raising essential ethical considerations. By staying attuned to these developments, stakeholders can harness the benefits while mitigating risks.
Comparative Analysis of Different Image Identification Platforms
The realm of image identification software is populated with a plethora of platforms, each carving its niche in the crowded marketplace. This comparative analysis aims to unveil the inherent strengths and weaknesses that underscore the diverse functionalities of these platforms. Understanding these aspects is crucial for both developers and users. It allows one to make informed decisions on which system aligns with their needs, whether it be for personal projects or large-scale enterprise solutions. The spotlight on such comparisons not only highlights technological capabilities but also sheds light on cost-efficiency, scalability, and user experience, all of which are vital in today's fast-paced tech environment.
Overview of Leading Image Recognition Systems
Among the frontrunners in the image recognition landscape are systems like Google Vision, Amazon Rekognition, and Microsoft Azure's Computer Vision. Each offers distinct features that cater to different needs:
- Google Vision:
- Amazon Rekognition:
- Microsoft Azure's Computer Vision:
- Utilizes advanced algorithms for detecting objects, landmarks, and logos.
- Provides robust integration with other Google Cloud services.
- Particularly recognized for its ability to analyze multiple languages in images.
- Strong emphasis on real-time facial analysis.
- Offers features like image and video analysis, which make it suitable for diverse applications, from security to marketing.
- Can recognize celebrities, brands, and even detect inappropriate content.
- Excels in extracting textual information from images through Optical Character Recognition (OCR).
- Integrates seamlessly into applications via APIs, appealing to developers.
- Known for providing detailed descriptions of scene contents, which support accessibility.
Understanding these platforms requires more than just looking at their features; it's essential to qualify these tools with their user experience, integration capabilities, and performance metrics.
Strengths and Weaknesses of Major Solutions
Every system has its fair share of advantages and challenges. It's vital to dig deeper and analyze:
- Google Vision:
- Amazon Rekognition:
- Microsoft Azure:
- Strengths:
- Weaknesses:
- Wide-ranging support for different types of media.
- Continuous updates due to Google's vast resources.
- Cost can escalate with extensive use of API calls.
- Strengths:
- Weaknesses:
- High accuracy in person recognition.
- Versatile for applications ranging from safety to entertainment.
- Concerns around data privacy practices, particularly in surveillance contexts.
- Strengths:
- Weaknesses:
- Strong OCR capabilities make it ideal for business documentation.
- Good customer support with resourceful documentation for developers.
- Can be complex for users unfamiliar with Azure's ecosystem.
"Selecting the right image identification platform isn't just about the technology itself; it's about how well it integrates into your operational flow and meets specific use cases."
In summary, while comparing platforms, consider not only their capabilities but how they align with your unique requirements. Whether for security, social media analysis, or business intelligence, the right selection can significantly enhance operational efficiency.
Integration with Other Technologies
The role of integration with other technologies in image identification software cannot be overstated. Today, visual recognition systems are not standalone; they exist within a broader ecosystem that leverages advancements across multiple technological fields. As this integration continues to evolve, it opens up new avenues of application and enhances the capabilities of image identification systems, creating more robust solutions that cater to a diverse array of needs.
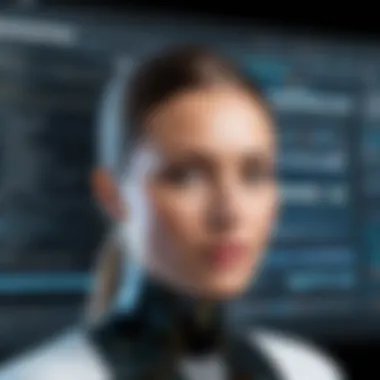
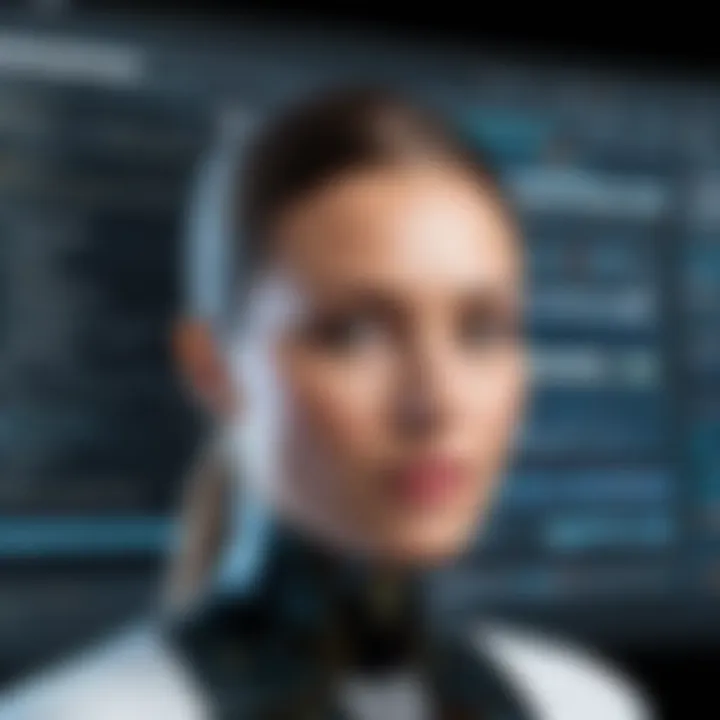
Synergy with Artificial Intelligence
Artificial Intelligence (AI) is a key partner in the realm of image identification. The combined forces of AI and visual recognition technologies have been a game changer in how images are processed, categorized, and utilized. Here are a few important aspects to consider:
- Enhanced Accuracy: AI algorithms, particularly those leveraging machine learning and deep learning techniques, have shown significant improvements in accurately recognizing and classifying images. By training on extensive datasets, these algorithms are capable of learning complex patterns, leading to better identification results.
- Automation of Tasks: Integrating image identification with AI enables automation of many previously manual tasks. For instance, in a retail setting, products can be quickly scanned and sorted without human intervention, reducing the labor required for stock management.
- Real-time Processing: AI-enhanced systems are capable of processing images in real-time. This speed is crucial for applications in security and surveillance, where immediate reactions to threats may depend on timely image analysis.
In summary, the fusion of AI with image recognition enhances performance and widens the scope of applications, influencing sectors ranging from healthcare to retail.
Image Recognition in IoT Applications
The Internet of Things (IoT) signifies a new horizon for image identification software. As increasing numbers of devices become interconnected, the potential for image recognition to further augment IoT functionalities is profound. Points to ponder include:
- Merging Data Sources: IoT applications often deal with a variety of data inputs, including sensor data and images. Combining these streams allows for comprehensive analyses, where visual context complements raw data. For example, a smart home security camera can not only record activities but also classify and react to them based on learned patterns, enhancing overall security measures.
- Improved User Interactivity: In a consumer context, the synergy between IoT and image recognition leads to smart appliances that understand user needs through visual inputs. Imagine a refrigerator that identifies food items and suggests recipes, simplifying meal planning and reducing waste.
- Remote Monitoring and Management: For industries like agriculture, IoT cameras equipped with image recognition systems can monitor crop health from afar. This integration empowers farmers to make data-driven decisions to optimize yield and manage resources more effectively.
Integrating image identification with other technologies is not just a technical enhancement; it's a pivotal shift that reshapes industries and daily life.
User Experience and Interface Design
User experience (UX) and interface design play a pivotal role in how effective image identification software becomes in real-world applications. With the rapid advancement in underlying technologies, the aligning of user needs with technical capabilities can often mean the difference between the success or failure of an application. While the algorithms and systems might be complex, the interface itself should be as straightforward as a pie. This isn’t just a matter of aesthetics but of function, giving the end-user a sense of ease and confidence while interfacing with the software.
Importance of Intuitive Design
Intuitive design is the glue that holds the user experience together. When users engage with image identification software, they ought to feel as though they are navigating through a familiar environment rather than wrestling with a labyrinth of complex controls. An intuitive design incorporates several elements:
- Simplicity: The interface should present options clearly without overloading the user with choices. Too many buttons or sliders can leave users feeling like a deer caught in headlights.
- Clarity of Purpose: Every function must have an easily understood purpose. If a user can't discern what a button does, it could lead to frustration and abandonment of the tool altogether.
- Feedback Mechanisms: Providing immediate feedback for user actions is critical. For example, when a user uploads an image, a progress bar or confirmation notification can reassure them the software is responding.
- Accessibility: An intuitive design should account for users of varying skill levels. This includes adaptable features for those requiring assistive technologies. Ensuring everyone can use the software without barriers is essential for a positive experience.
Ultimately, intuitive design is all about creating an interface that caters to the user’s comprehension rather than a showcase of technological prowess.
Case Studies of Effective Interfaces
Real-world examples can often illustrate the principles of effective user experience in action. Here are a couple of noteworthy instances of image identification software that nailed their interfaces:
- Google Lens: This platform is a standout in intuitive design, allowing users to employ their smartphone cameras to identify objects, landmarks, and even translate text. The layout is clean, with a simple interface that guides you through the process. Users can instantly see their camera view alongside options for searching related information. "Point and click, and you get results; it's as easy as it gets."
- Pinterest Lens: Pinterest transformed the way users discover ideas through images. With its Lens feature, users can take photos and find visually similar items listed on the site. Importantly, the interface offers related suggestions as the search unfolds, keeping the user engaged without feeling lost in the process. The thoughtful division of search results into clear categories aids users in narrowing down their interests swiftly.
In both these cases, giving thought to user experience and design didn’t just enhance functionality; it directly contributed to the widespread uptake of the technologies. When designing image identification systems, creating an interface that matches user expectation is crucial for both satisfaction and retention.
"Design is not just what it looks like and feels like. Design is how it works." - Steve Jobs
By prioritizing intuitive design and learning from real-world successes, developers can create tools that not only meet the needs of users but also elevate their overall experience. In an era where technology is central to everyday life, the interface design should arm users with confidence, enabling them to harness the full power of image identification software.
Regulatory Landscape Surrounding Image Identification
In the realm of image identification software, the regulatory landscape plays a crucial role, shaping how these technologies operate and evolve. With the rising utilization of image recognition in various sectors—from security to retail—it's pivotal to ensure that the frameworks governing their use are robust, comprehensive, and adaptable to rapid technological advancements. Following a structured regulatory approach not only protects individual rights but also builds public trust in these systems.
As image identification technology continues to permeate daily life, the boundaries of privacy, security, and ethical considerations often blur. Consequently, a thoughtful regulatory framework can guide developers and businesses in adopting best practices. Furthermore, regulations can also serve to enforce accountability among companies and promote responsible innovation.
Current Regulations and Standards
Currently, various regulations govern the use of image identification technologies across different jurisdictions. A prominent example includes the General Data Protection Regulation (GDPR) introduced in the European Union. This regulation emphasizes the importance of user consent and mandates transparency in how data is handled. Under GDPR, companies must inform users about what data is collected, how it is used, and with whom it is shared. This regulation has set a high bar for compliance, compelling organizations using image recognition to adopt stringent measures to protect personal data.
In the United States, although there isn’t a unified federal law specifically tailored to image recognition, a patchwork of state-level regulations is emerging. For instance, California's Consumer Privacy Act (CCPA) grants consumers rights regarding their personal information, establishing transparency and giving individuals more control over data sharing practices. Additionally, several cities have taken it a step further, imposing outright bans on the use of facial recognition technology by law enforcement agencies, echoing concerns about civil liberties.
Key Standards Include:
- ISO/IEC 27001: This standard focuses on managing information security, providing a framework for organizations to protect sensitive data, including images.
- NIST Special Publication 800-53: This publication provides guidelines for selecting and specifying security controls for information systems to ensure security and privacy.
- IEEE 7000: It addresses the ethical considerations in system design, advocating for the inclusion of ethics in the developmental processes of technologies like image recognition.
Proposed Changes and Impacts
As technology progresses, the regulatory landscape must also shift to address emerging challenges. Proposed changes often revolve around stricter enforcement of data privacy laws and the introduction of new standards that specifically cater to AI and image identification technologies. One significant proposed amendment is to enhance consent frameworks, making them clearer and easier for users to understand. This could mean that organizations must not only acquire consent but also demonstrate their ongoing commitment to safeguarding personal data.
Another potential regulatory shift involves establishing more comprehensive guidelines on algorithmic bias. As image identification technologies rely heavily on data sets, there's a risk of perpetuating existing biases, leading to unequal outcomes. New standards could mandate companies to conduct regular audits and assessments of their algorithms, ensuring fairness and non-discrimination.
"The regulatory landscape for image identification is not just about compliance; it's about earning public trust and ensuring ethical development in the tech industry."
The impacts of these proposed changes can be significant. Improved regulations can promote innovation by encouraging developers to create systems that respect user privacy while also enhancing user experience. In turn, this can lead to broader acceptance of image identification technologies, fostering a sustainable ecosystem where technology serves the public good.
In summary, as image identification software continues to grow, navigating the regulatory landscape remains a complex but essential task. Adapting existing frameworks and proposing new regulations will be vital to safeguard personal data, mitigate biases, and enable ethical usage of these advanced technologies. Through careful consideration and stakeholder collaboration, we can create a regulatory environment that not only protects individuals but also encourages innovation.
End: The Impact of Image Identification Software on Society
In the age of rapid technological advancement, image identification software stands at the forefront of innovation, impacting various aspects of daily life. These tools are no longer just fancy gadgets used for fun; they have evolved into essential components of industries such as security, healthcare, and retail. Understanding their societal implications is crucial as we navigate a world increasingly reliant on visual recognition technologies.
The contribution of image identification software to enhancing security measures is profound. From facial recognition in public spaces to identifying potential threats in real-time, these systems work tirelessly to keep communities safer. However, with this capability comes a heavy responsibility. Policymakers and the public must grapple with the ethical dilemmas surrounding privacy and data security.
"As the use of image identification software expands, so does the conversation about consent and surveillance. It’s a double-edged sword that requires careful consideration."
Recap of Key Insights
Reflecting on the various discussions throughout this article, several key insights emerge regarding image identification software. These include:
- Widespread Applications: Industries such as retail and healthcare utilize this technology for improved customer experience and patient diagnosis.
- Algorithmic Challenges: While these tools can achieve impressive accuracy, issues relating to bias remain prevalent, complicating their reliability further.
- Regulatory Needs: As the capabilities of these technologies grow, so do the calls for stricter regulations and ethical guidelines to govern their use.
These factors illustrate the need for a balanced approach, recognizing the benefits while addressing potential pitfalls.
Looking Ahead
The future of image identification software appears bright yet fraught with complexities. Ongoing developments in algorithms, particularly in the realm of deep learning, promise to enhance the accuracy and versatility of these tools. Furthermore, as the Internet of Things (IoT) becomes more integrated into daily life, the potential for image recognition to transform how we interact with technology is enormous.
However, careful stewardship is essential.
- Continued Research: Investment in research to improve algorithm transparency and reduce bias is crucial. Experts must work to build systems that truly reflect the diversity of society.
- Public Dialogue: Open discussions about the trade-offs between security and privacy must be prioritized. Engaging with communities can foster trust and understanding.
- Regulatory Evolution: Expect changes in regulations as society adapts to these technologies. Governance will need to evolve in tandem with innovation to address emerging ethical concerns.
By fostering an environment where technology serves humanity while honoring ethical boundaries, we can unlock the full potential of image identification software, ensuring it benefits all sectors of society.