Unveiling the Significance of Data Weighting in Information Security
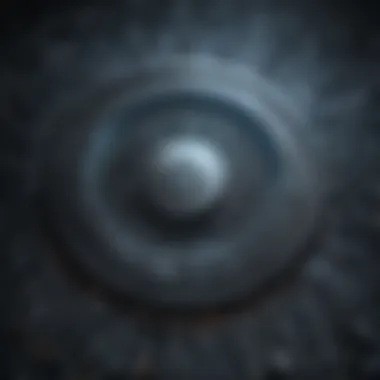
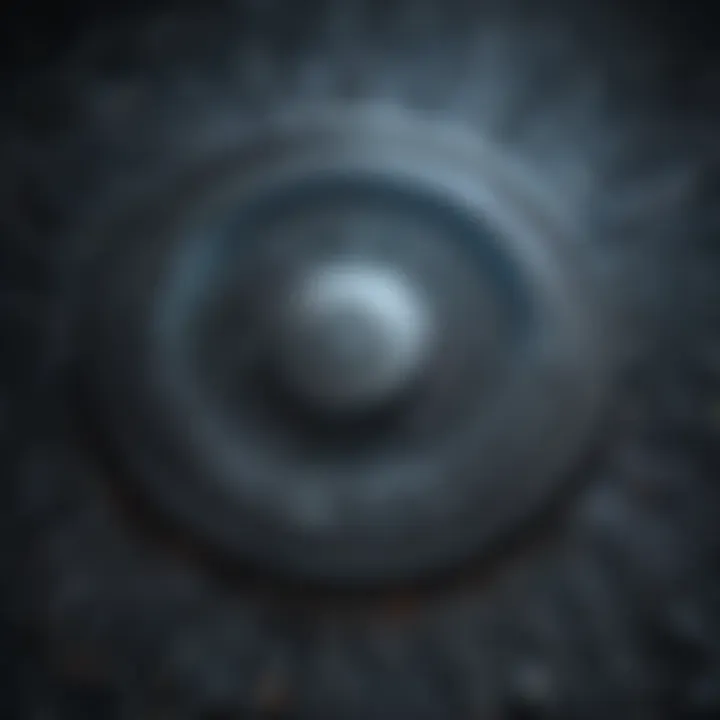
Understanding Storage, Security, or Networking Concepts
The foundation of information security rests on the critical element of data weighting. Data weighting involves assigning different levels of importance to various data sets, influencing decision-making processes and enhancing cybersecurity measures within organizations. By prioritizing data sets based on their significance, businesses can bolster their defenses against cyber threats and optimize their overall data management strategies.
Within the realm of information security, data weighting plays a pivotal role in fortifying cybersecurity defenses and streamlining organizational processes. By understanding the nuances of data prioritization, companies can proactively mitigate risks and enhance their overall security posture.
Introduction to Data Weighting
Data weighting plays a crucial role in information security by assigning varying degrees of importance to different data sets. This process influences decision-making processes and enhances cybersecurity measures. By understanding the nuances of data weighting, organizations can fortify their defenses against cyber threats and optimize their data management strategies, ensuring a robust security framework.
Defining Data Weighting
The concept of assigning values to different data sets
Assigning values to different data sets involves prioritizing information based on its significance and relevance to organizational operations. This method allows for the identification of critical data that requires heightened protection and handling. It facilitates the efficient allocation of resources towards securing valuable information while streamlining processes for less critical data sets. By categorizing data based on importance, organizations can tailor their security protocols to address specific vulnerabilities and threats effectively.
Impact on data analysis and decision-making
The practice of data weighting significantly impacts data analysis and decision-making processes within an organization. By assigning distinct weights to various data sets, businesses can prioritize their review and analysis efforts. This prioritization ensures that critical information receives prompt attention, enabling informed decision-making based on reliable data insights. Moreover, data weighting enhances the accuracy and reliability of analyses, reducing the likelihood of errors or oversights in decision-making processes.
Historical Context
Evolution of data weighting in information security
The evolution of data weighting in information security traces back to the growing complexity of cyber threats and the increasing volume of data generated and stored by organizations. As technology advanced, the need to differentiate between data sets based on their importance became imperative. This evolution led to the development of sophisticated data weighting techniques that empower organizations to adapt their security strategies to dynamic cyber landscapes.
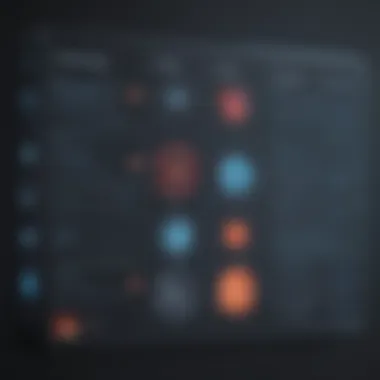
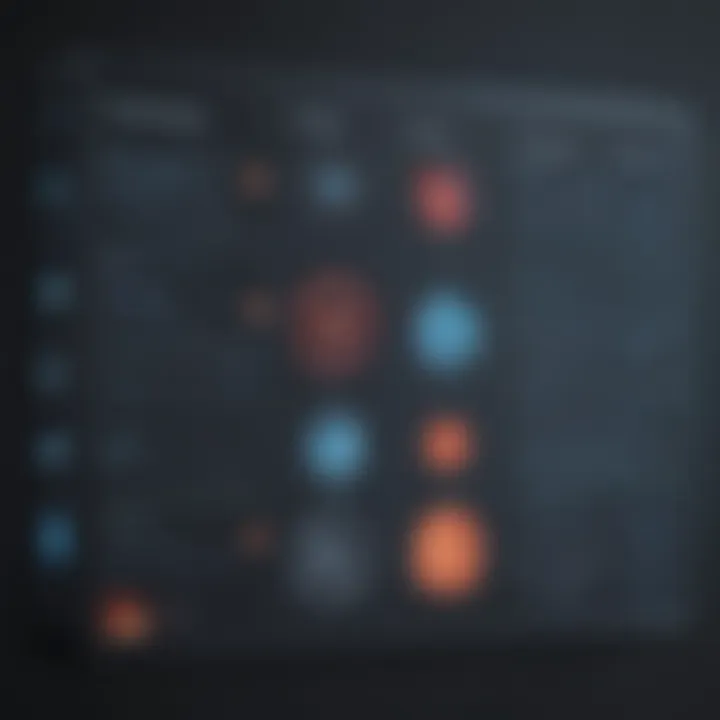
Key milestones and developments
Significant milestones in the development of data weighting include the introduction of advanced algorithms for data prioritization, the integration of machine learning for automated weight assignment, and the alignment of data weighting practices with industry regulations. These milestones have transformed how organizations safeguard their sensitive information and shape their cybersecurity policies to combat evolving threats effectively.
Importance of Data Weighting
Enhancing data prioritization
Data weighting enhances data prioritization by enabling organizations to identify and classify critical data segments that require stringent security measures. This process ensures that resources are allocated efficiently to safeguard valuable information resources, reducing the risk of data breaches and unauthorized access. By enhancing data prioritization, organizations can focus on protecting high-value assets proactively, thereby bolstering their overall security posture.
Customizing security measures
Customizing security measures based on data weighting allows organizations to tailor their defenses to address specific security needs effectively. By understanding the importance of different data sets, organizations can implement targeted security controls and access restrictions to protect critical information. This customization ensures that security measures align with the value and sensitivity of organizational data, optimizing security investments and mitigating potential risks effectively.
Implications for Cybersecurity
In the exploration of the Importance of Data Weighting in Information Security, the section regarding Implications for Cybersecurity holds paramount significance. This portion delves into crucial elements that underpin the integration of data weighting in security measures. By focusing on the specific elements within Implications for Cybersecurity, such as risk mitigation strategies, threat detection, response protocols, and compliance alignment, the article elucidates how data weighting can bolster cybersecurity defenses. The benefits of incorporating data weighting include an enhanced ability to prioritize data sets based on importance, leading to more effective security measures. Moreover, considerations about the adaptability of security protocols based on the importance of data shed light on the responsive nature of modern data security techniques.
Risk Mitigation Strategies
Role of data weighting in mitigating cyber risks
Exploring the role of data weighting in mitigating cyber risks unveils a strategic approach to identifying and addressing potential vulnerabilities within an organization's data infrastructure. By assigning varying degrees of importance to different data sets, organizations can focus their resources on safeguarding critical information. This targeted approach enhances the overall risk mitigation strategy, fortifying defenses against cyber threats. The key characteristic of leveraging data weighting for cyber risk mitigation lies in its ability to prioritize proactive security measures based on the significance of data sets. This approach proves to be a popular choice in the realm of information security due to its efficacy in preemptively addressing potential threats. The unique feature of data weighting for risk mitigation is its scalability and adaptability to evolving cybersecurity landscapes, offering organizations a dynamic tool to combat emerging threats effectively.
Adapting security protocols based on data importance
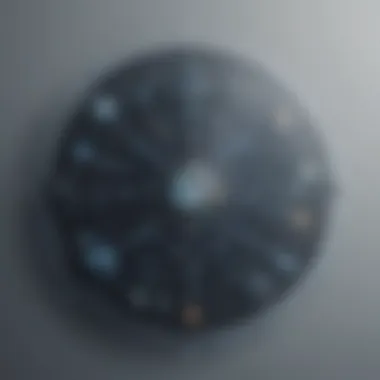
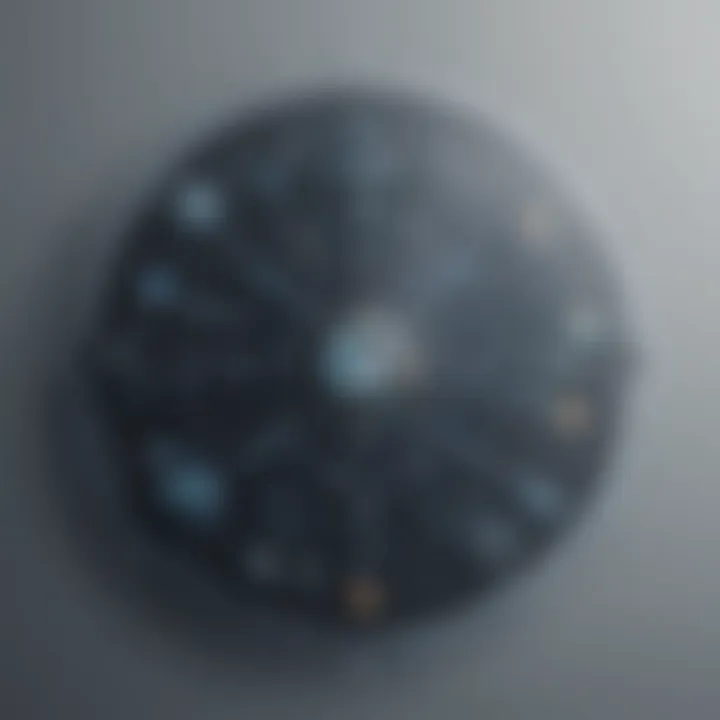
The adaptation of security protocols based on data importance represents a proactive stance towards cybersecurity. By tailoring security measures to align with the significance of different data sets, organizations can optimize their defenses against specific threats. This tailored approach ensures that critical data receives heightened protection, while less crucial information is adequately secured. The key characteristic of adjusting security protocols to data importance lies in the precision of resource allocation and response strategies. This customization proves to be a beneficial choice for organizations seeking bespoke security solutions tailored to their unique data landscape. The unique feature of adapting security protocols based on data importance is the nuanced approach to incident response, enabling organizations to allocate resources efficiently and respond promptly to security breaches.
Threat Detection and Response
Utilizing weighted data for early threat detection
The utilization of weighted data for early threat detection revolutionizes the approach to identifying potential risks within an organization's data ecosystem. By assigning different weights to data sets, organizations can implement early warning systems that pinpoint anomalies and potential threats promptly. This proactive approach enhances the overall defense mechanism, enabling organizations to detect threats before they escalate. The key characteristic of leveraging weighted data for threat detection lies in the predictive capabilities that prioritize critical data for monitoring. This approach proves to be a popular choice due to its ability to preemptively address security threats and vulnerabilities. The unique feature of utilizing weighted data for early threat detection is the integration of automated analytics that streamline threat identification processes, enhancing overall response efficiency.
Streamlining incident response processes
The streamlining of incident response processes through the utilization of weighted data signifies a strategic shift towards a more efficient and effective cybersecurity framework. By assigning importance to data sets, organizations can delineate response protocols that align with the significance of the information at hand. This tailored response strategy ensures that critical incidents receive immediate attention, while lower-priority events are managed appropriately. The key characteristic of streamlining incident response processes based on data weighting is the optimization of resource utilization and response timeframes. This approach proves to be a beneficial choice for organizations looking to enhance their incident response mechanisms by prioritizing critical data assets. The unique feature of this approach is the development of response playbooks tailored to specific data sets, facilitating a coordinated and swift response to security incidents.
Compliance and Regulatory Alignment
Impact of data weighting on regulatory compliance
The impact of data weighting on regulatory compliance signifies a strategic alignment between security measures and legal requirements. By weighting data sets based on regulatory mandates, organizations can ensure that their data management practices adhere to industry-specific standards. This alignment not only fosters compliance with regulations but also enhances overall data protection efforts. The key characteristic of integrating data weighting for regulatory compliance lies in the systematic approach to prioritizing data based on legal requirements. This strategy proves to be a popular choice for organizations seeking to maintain regulatory alignment while optimizing security measures. The unique feature of ensuring compliance through data weighting is the development of tailored data management policies that streamline compliance efforts and safeguard organizational data assets.
Ensuring data protection standards
Ensuring data protection standards through the utilization of data weighting epitomizes a proactive approach to safeguarding sensitive information. By prioritizing data sets based on protection requirements, organizations can fortify their security measures to mitigate privacy risks. This targeted approach ensures that data protection efforts are aligned with the importance of information assets, bolstering overall data security. The key characteristic of maintaining data protection standards via data weighting is the precision in implementing security controls tailored to different data categories. This approach proves to be a beneficial choice for organizations seeking comprehensive data protection solutions that align with evolving privacy regulations. The unique feature of this approach is the strategic integration of encryption and access controls based on data importance, enhancing data confidentiality and integrity measures.
Data Management Optimization
Data Management Optimization plays a crucial role in understanding the importance of data weighting within the realm of information security. Varying degrees of importance assigned to data sets enable organizations to fortify their defenses against cyber threats and optimize their data management strategies effectively. By prioritizing data sets based on their significance, organizations can improve decision-making processes and enhance cybersecurity measures. Data Management Optimization encompasses various elements such as efficient data storage, streamlined resource allocation, and effective data lifecycle management, contributing significantly to bolstering overall data security and operational efficiency.
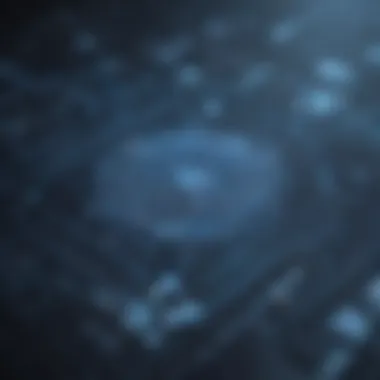
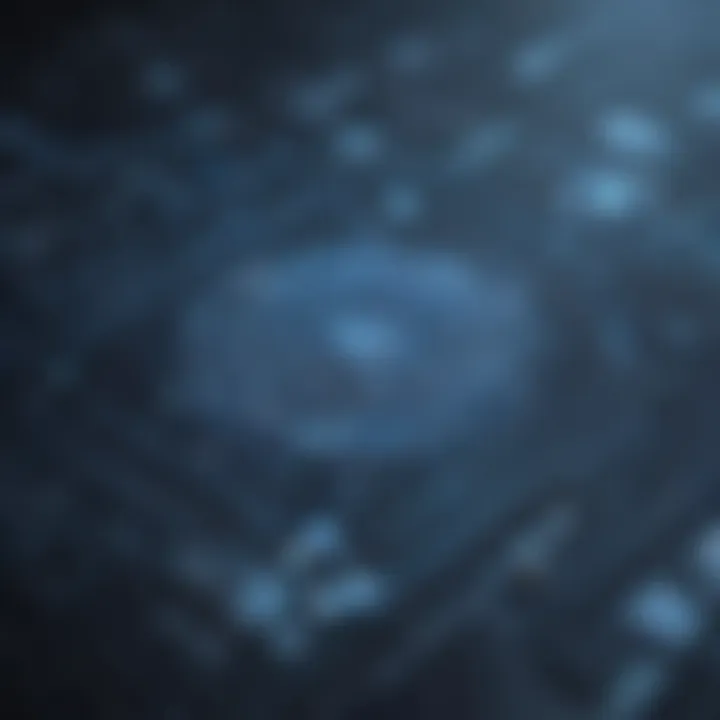
Enhancing Data Efficiency
In Data Management Optimization, enhancing data efficiency is paramount for ensuring streamlined operations and effective utilization of resources. Streamlining data storage based on importance involves categorizing data sets according to their relevance and criticality to the organization's operations. This process allows for easy access to crucial data and quick decision-making based on the significance of each dataset. Streamlining data storage based on importance is essential for enhancing operational efficiency, reducing data clutter, and optimizing data retrieval processes. While this approach enhances data access speed and organizational responsiveness, it may require careful planning and data categorization to ensure accurate prioritization and storage optimization.
Improving data retrieval and access is another critical aspect of enhancing data efficiency within Data Management Optimization. By implementing robust data retrieval processes and optimizing data access routes, organizations can expedite decision-making processes and improve operational agility. Improving data retrieval and access involves establishing efficient data retrieval mechanisms, reducing data latency, and enhancing system responsiveness when accessing critical information. This approach not only accelerates data access but also boosts overall operational efficiency and contributes to a more responsive and proactive organizational culture. However, challenges may arise in terms of data security and access control when optimizing data retrieval and access processes, necessitating stringent data protection measures and authentication protocols to safeguard sensitive information.
Resource Allocation Strategies
In the context of Data Management Optimization, resource allocation strategies are critical for optimizing operational performance while maintaining robust data security measures. Optimizing resource allocation through data weighting involves assigning resources based on the importance and criticality of data sets within an organization. By prioritizing resource allocation according to data weighting criteria, organizations can ensure that vital data receives adequate resources and attention, leading to enhanced data security and operational efficiency. This approach allows organizations to balance performance and security effectively, aligning resource allocation decisions with data significance and operational requirements.
Balancing performance and security is a key component of resource allocation strategies within Data Management Optimization. Organizations must strike a delicate balance between maximizing operational performance and maintaining robust data security measures. By optimizing resource allocation to accommodate both performance and security considerations, organizations can enhance operational efficiency without compromising data protection protocols. Balancing performance and security involves implementing data weighting mechanisms that allocate resources proportionately to data importance, ensuring that operational performance aligns with data security requirements. While this approach optimizes resource utilization and improves operational effectiveness, organizations must continuously monitor and adjust resource allocation strategies to adapt to evolving security threats and operational challenges.
Data Lifecycle Management
Effective data lifecycle management is essential for organizations to optimize data utilization, storage, and deletion processes based on data weighting criteria. Implementing effective data retention policies enables organizations to retain data strategically, ensuring that vital information is preserved for future reference and operational needs while minimizing storage costs and data redundancy. Effective data retention policies involve defining clear retention timelines, categorizing data based on importance, and establishing secure data storage mechanisms to prevent data loss or unauthorized access.
Archiving and deleting data based on weighting is another critical component of data lifecycle management within Data Management Optimization. By archiving and deleting data based on weighting criteria, organizations can free up storage space, enhance data retrieval efficiency, and mitigate data security risks associated with unnecessary data retention. Archiving essential data for long-term storage and securely deleting obsolete or low-priority data help organizations streamline data storage processes, improve data access speed, and reduce storage costs. While archiving and deleting data based on weighting enhances data management efficiency and security, organizations must implement robust data backup mechanisms and retrieval protocols to safeguard archived data and prevent data loss.
Future Trends and Innovations
In this section, we delve into the significance of Future Trends and Innovations within the realm of information security. The constant evolution of technology necessitates an exploration of upcoming trends to stay ahead in safeguarding valuable data assets. Understanding these innovative trends is crucial for organizations to adapt and enhance their cybersecurity strategies effectively. By analyzing cutting-edge developments, companies can proactively prepare for emerging threats and challenges, ultimately fortifying their defenses against cyber intrusions. Moreover, embracing innovations ensures that organizations remain at the forefront of cybersecurity advancements, promoting a resilient and adaptive security environment.
Machine Learning Applications
- Automated data weighting algorithms: Within the context of information security, Automated data weighting algorithms play a pivotal role in streamlining data prioritization processes. Their automation capabilities enable swift and accurate assignment of importance levels to various data sets, optimizing decision-making procedures. The key characteristic of Automated data weighting algorithms lies in their ability to efficiently allocate weights based on predefined criteria, enhancing the overall data management efficiency. This automated approach reduces manual intervention, saving time and resources while ensuring consistent and reliable results.
- Predictive analytics for data prioritization: In the landscape of information security, Predictive analytics for data prioritization stands out as a proactive mechanism to anticipate security needs. By analyzing historical data patterns and trends, predictive analytics can forecast potential security risks and prioritize data accordingly. The distinctive feature of Predictive analytics lies in its predictive power, enabling organizations to pre-empt threats and vulnerabilities before they materialize. This proactive approach enhances response times, minimizes security incidents, and optimizes resource allocation for improved cybersecurity effectiveness.
Blockchain and Data Security
- Distributed ledger technologies for weighted data: The incorporation of Distributed ledger technologies in managing weighted data adds a layer of transparency and integrity to information security practices. These technologies offer a decentralized and immutable platform for storing and managing weighted data, ensuring secure and tamper-proof data records. The key characteristic of Distributed ledger technologies lies in their ability to provide a trustless environment for data transactions, enhancing data security and confidentiality. While the benefits of enhanced security and data integrity are prominent, challenges such as scalability and integration complexities may arise when implementing these technologies in traditional security frameworks.
- Enhancing data integrity and confidentiality: Strengthening data integrity and confidentiality are paramount in ensuring information security. Innovations focused on enhancing data integrity and confidentiality aim to safeguard sensitive information from unauthorized access or tampering. The unique feature of these innovations lies in their intent to implement robust encryption methods and access controls, ensuring data confidentiality alongside maintaining data integrity. The advantages of improved data protection and confidentiality outweigh potential disadvantages related to implementation complexities and operational overhead faced by organizations adopting these stringent security measures.
Quantum Computing Implications
- Potential impact of quantum computing on data weighting: The potential integration of quantum computing in data weighting poses a paradigm shift in information security protocols. Quantum computing's unparalleled processing power has the potential to influence data weighting methodologies by introducing complex algorithms and cryptography techniques. The key characteristic of quantum computing lies in its ability to process massive datasets at speeds unattainable by traditional computers, revolutionizing data weighting strategies. While the advantages of quantum computing in data processing are vast, challenges such as algorithmic security and hardware vulnerabilities pose potential risks that organizations must address. Navigating these complexities requires a comprehensive understanding of quantum computing's implications on data security measures.
- Advancements in data encryption and security: The advancements made in data encryption and security technologies play a crucial role in fortifying information security defenses. Innovations in encryption algorithms and security protocols enhance data protection measures, mitigating threats posed by cyber adversaries. The key characteristic of these advancements lies in their ability to provide a multi-layered approach to data security, incorporating encryption standards and authentication methods to safeguard sensitive information. While the benefits of enhanced encryption and security measures are evident, organizations must address challenges such as compatibility issues and regulatory compliance when implementing these advanced security solutions.
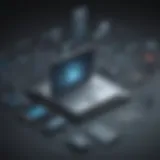
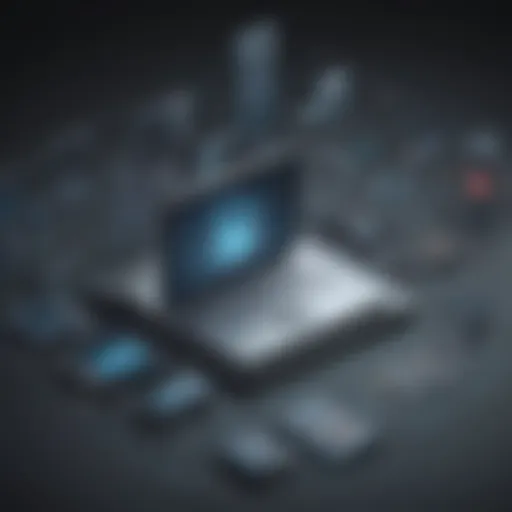