In-Depth Analysis of Leading Big Data Platforms: A Comparative Study
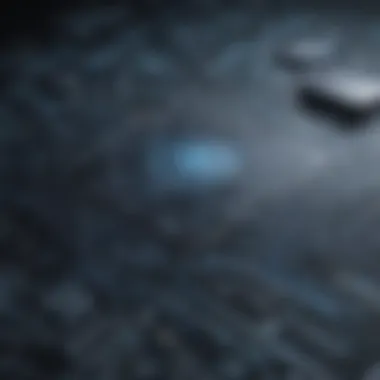
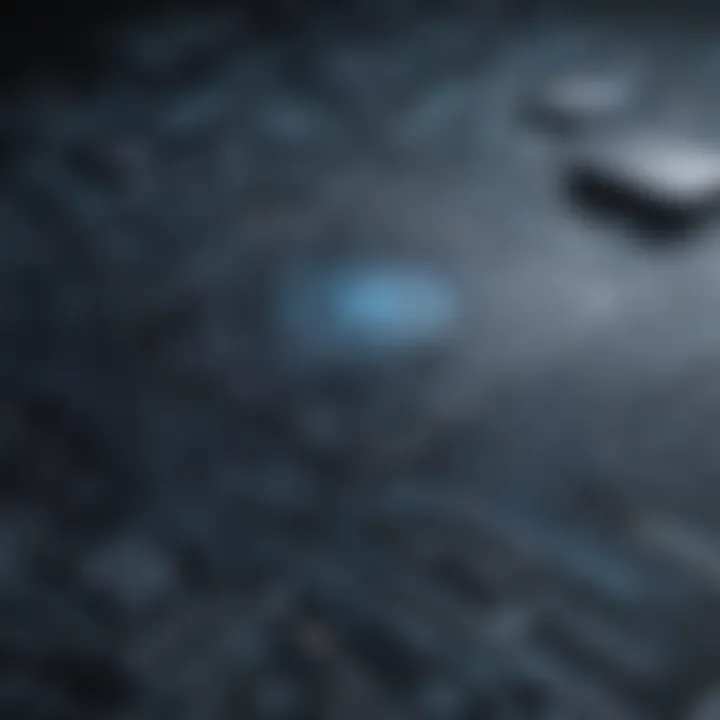
Understanding Storage, Security, or Networking Concepts
As we embark on a journey through the intricate realm of big data platforms, it is imperative to lay a solid foundation by comprehending the fundamental concepts of storage, security, and networking. Storage pertains to the mechanisms used for storing and managing vast volumes of data efficiently. Security encompasses the array of measures employed to safeguard data integrity and privacy, while networking focuses on optimizing data transfer and communication processes within a system.
Best Practices and Tips for Storage, Security, or Networking
In the realm of big data, optimizing storage solutions is paramount to ensure seamless data management and accessibility. Implementing security best practices is crucial to mitigate the risks posed by cyber threats and breaches. Networking strategies play a pivotal role in enhancing performance and enabling robust connectivity for efficient data processing.
Industry Trends and Updates
Keeping abreast of the latest trends in storage technologies is indispensable for staying ahead in the ever-evolving landscape of big data. Cybersecurity threats continue to evolve, necessitating proactive solutions and tools to combat emerging risks. Innovations in networking technologies drive advancements in data transfer speeds and reliability, shaping the future of data-driven enterprises.
Case Studies and Success Stories
Delving into real-life case studies offers valuable insights into successful storage implementations, shedding light on the strategies that contribute to operational efficiency and data security. Examining cybersecurity incidents and the ensuing lessons learned equips organizations with the knowledge to fortify their defenses against potential threats. Networking case studies provide exemplary instances of effective strategies that optimize network performance and facilitate seamless data flow.
Reviews and Comparison of Tools and Products
Thorough reviews of storage software and hardware elucidate the functionalities and performance metrics of different solutions, aiding businesses in making informed decisions. Comparing cybersecurity tools and solutions enables organizations to evaluate the efficacy of various security measures and choose the most suitable options. Evaluating networking equipment and services helps in identifying solutions that align with the scalability and performance requirements of modern data environments.
Introduction
In the realm of big data platforms, making informed choices is crucial for businesses aiming to harness the power of data analytics effectively. Understanding the nuances and capabilities of various platforms such as Apache Hadoop, Apache Spark, Amazon EMR, and Google BigQuery is essential to optimize performance and scalability. This article embarks on a journey to dissect and compare these significant players, offering a panoramic view of their functionalities and advantages tailored towards diverse business needs and data management strategies. Through a meticulous analysis, readers will gain profound insights into the intricate world of big data platforms, enabling them to make strategic decisions aligning with their organizational goals and requirements.
Overview of Big Data Platforms
Defining Big Data Platforms
Big data platforms serve as the backbone for handling and processing vast volumes of data efficiently. These platforms are characterized by their ability to store, manage, and analyze structured and unstructured data to extract valuable insights. One key aspect of defining big data platforms is their capability to scale horizontally, allowing seamless expansion as data volumes grow. This scalability feature is vital for organizations dealing with massive data sets, ensuring that performance remains optimal even as the data load increases. The unique selling point of defining big data platforms lies in their distributed computing framework, which enhances data processing speed and enables parallel computation for quicker analytical results. However, this distributed nature can also pose challenges in terms of data consistency and synchronization, necessitating robust data management strategies to mitigate such risks effectively.
Importance in Data Management
The importance of big data platforms in data management cannot be overstated, given their role in facilitating data-driven decision-making and driving business success. These platforms offer a centralized infrastructure for integrating data from various sources, enabling seamless data processing and analysis. A key characteristic of big data platforms is their ability to handle real-time data streams, allowing organizations to derive actionable insights promptly. This real-time capability is particularly beneficial in scenarios requiring rapid decision-making and agile responses to changing market dynamics. Moreover, big data platforms support advanced analytics techniques like machine learning and predictive modeling, empowering businesses to uncover valuable patterns and trends within their data sets. Despite these advantages, the complexity of implementing and managing big data platforms can be a deterrent for some organizations lacking specialized expertise or resources.
Significance of Choosing the Right Platform
Impact on Performance
The choice of big data platform significantly impacts the overall performance of data processing and analytics tasks. Platforms with efficient data processing algorithms and optimized resource utilization can significantly enhance processing speed and reduce latency. The key characteristic of platforms that positively impact performance is their ability to leverage parallel processing and distributed computing, enabling faster execution of complex queries and computations. By harnessing in-memory processing capabilities, platforms can store and retrieve data rapidly, eliminating the need for frequent disk accesses and enhancing overall performance. However, the extensive use of memory for in-memory processing can lead to higher costs and resource constraints, necessitating careful optimization to achieve the desired balance between performance and cost-efficiency.
Scalability Considerations
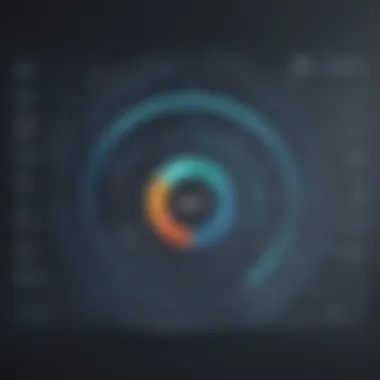
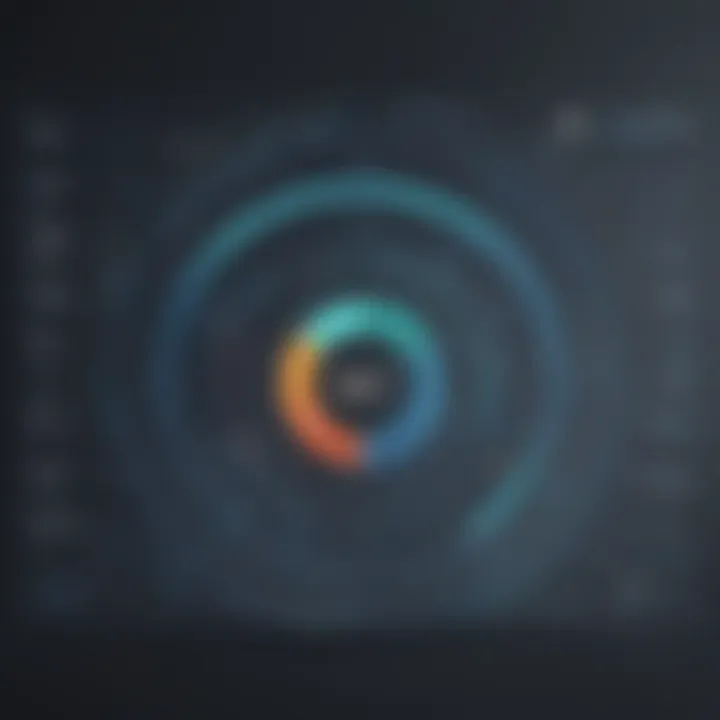
Scalability considerations play a pivotal role in determining the suitability of a big data platform for varying workloads and data volumes. Platforms that offer seamless scalability by adding or removing nodes dynamically can adapt effectively to fluctuating data demands. The key characteristic of scalable platforms is their elastic infrastructure that can expand or contract based on workload requirements, ensuring optimal resource utilization at all times. This flexibility is especially beneficial for organizations experiencing seasonal spikes in data processing needs or sudden surges in user activity. Moreover, scalable platforms enable horizontal scaling, distributing data processing tasks across multiple nodes to enhance performance and accommodate growing data sets. However, achieving optimal scalability often requires fine-tuning of cluster configurations and workload distribution, posing challenges for organizations without sufficient expertise in cluster management and optimization.
Key Players in Big Data Technologies
Key players in big data technologies play a crucial role in this article by encompassing Apache Hadoop, Apache Spark, Amazon EMR, and Google BigQuery. These technologies act as pillars in the realm of big data management and analytics, offering a wide array of features and capabilities essential for diverse business requirements. Understanding the nuances and intricacies of each key player is paramount for IT professionals, cybersecurity experts, and students delving into the realm of big data. From their architecture, ecosystem components, managed frameworks to their integration with various services, these key players present a rich tapestry of possibilities, challenges, and opportunities in the big data landscape.
Apache Hadoop
Architecture Overview
Apache Hadoop's architecture overview serves as the backbone of its distributed processing capabilities, enabling the handling of large-scale data processing tasks. The key characteristic of Hadoop's architecture lies in its ability to distribute data and computation across clusters of computers, offering high availability and fault tolerance. This architectural design proves beneficial for this article as it aligns with the scalability considerations crucial in big data applications. However, the intricacies of setting up and maintaining a Hadoop cluster may present challenges in certain contexts.
Ecosystem Components
The ecosystem components of Apache Hadoop form a robust set of tools and frameworks that complement its core functionalities. Hadoop's ecosystem features various tools such as HDFS, MapReduce, YARN, and Hive, each serving specific data processing or management tasks. This diversity within the ecosystem highlights Hadoop's versatility and adaptability to different data processing needs. While this ecosystem offers a wide range of options, the complexity of choosing the right tools for specific use cases can sometimes be overwhelming.
Apache Spark
In-Memory Processing
Apache Spark's in-memory processing capability revolutionizes big data analytics by caching data in memory for faster iterative computations. This feature significantly enhances processing speed and performance, making it a favored choice for handling real-time analytics and iterative algorithms. The essence of in-memory processing aligns perfectly with the speed and efficiency considerations vital for demanding big data applications. Nevertheless, the resource-intensive nature of in-memory processing may require careful resource management to optimize performance.
Unified Analytics Engine
The unified analytics engine of Apache Spark integrates streaming, batch processing, SQL queries, machine learning, and graph processing within a single framework. This holistic approach simplifies the development and deployment of complex data pipelines, offering a seamless experience for data engineers and analysts. The versatility of Spark as a unified analytics engine makes it an attractive choice for organizations looking to streamline their data processing workflows. However, mastering the diverse functionalities of Spark may involve a steep learning curve for users new to the platform.
Amazon EMR
Managed Hadoop Framework
Amazon EMR provides a managed Hadoop framework that simplifies the deployment and scaling of Hadoop clusters on the AWS cloud infrastructure. The key characteristic of EMR's managed framework lies in its ability to automate cluster provisioning, configuration, and tuning, reducing operational overhead significantly. This managed approach ensures faster time-to-insight for organizations leveraging big data technologies. Yet, the trade-off between convenience and customization poses a consideration for users requiring specific configurations or optimizations tailored to their unique use cases.
Integration with AWS Services
Amazon EMR seamlessly integrates with a wide range of AWS services, facilitating data transfer, storage, and analytics across the AWS ecosystem. The key characteristic of this integration lies in the seamless interoperability between EMR and other AWS services, enabling users to leverage a holistic cloud ecosystem for their big data workflows. This integration enhances workflow efficiency and cost-effectiveness, aligning well with the scalability considerations essential for dynamic big data environments. However, the dependency on AWS services for integration may limit flexibility for organizations operating in multi-cloud environments.
Google BigQuery
Serverless Data Warehouse
Google BigQuery offers a serverless data warehousing solution that eliminates the need for infrastructure management, allowing users to focus solely on analyzing data. The key characteristic of BigQuery's serverless architecture lies in its on-demand pricing model and automatic scaling, making it a cost-effective and scalable choice for handling big data analytics workloads. This serverless approach aligns with the ease of use and scalability requirements critical for modern data-driven organizations. Nevertheless, users must carefully monitor costs and query efficiency to maximize the benefits of BigQuery's serverless model.
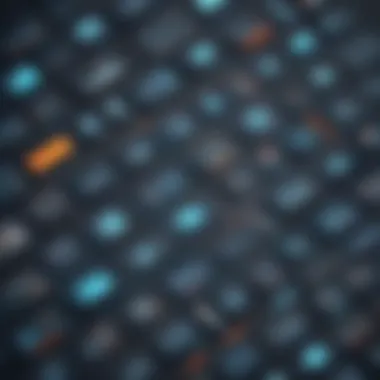
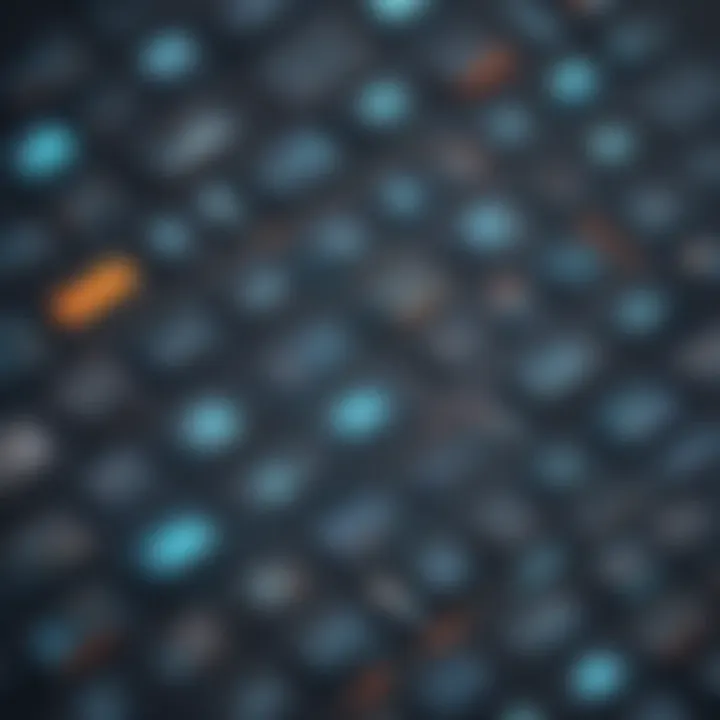
Scalable and Cost-Effective
Google BigQuery's scalable and cost-effective nature stems from its separation of storage and compute, enabling independent scalability for both components. This approach ensures that organizations can scale their analytical outputs without impacting storage costs or performance. The key characteristic of this scalable and cost-effective model makes BigQuery a desirable choice for organizations with fluctuating data processing needs. However, optimizing cost-efficiency while maintaining performance levels requires strategic allocation of resources and continuous monitoring of usage patterns.
Comparative Analysis
In the realm of big data platforms, Comparative Analysis plays a pivotal role in assisting organizations in making informed decisions regarding the selection of the most suitable platform for their specific requirements. By delving deep into the Performance Metrics, Scalability and Flexibility, and Ease of Use and Integration of various big data platforms, Comparative Analysis provides a comprehensive understanding of their strengths and limitations. It enables IT professionals, cybersecurity experts, and students to gauge the effectiveness of each platform in handling large volumes of data, managing diverse workloads, and integrating seamlessly with external tools.
Performance Metrics
Throughput
Throughput, a critical element in evaluating the efficiency of big data platforms, refers to the amount of data processed within a given time frame. Higher throughput signifies enhanced processing speed and performance, making it a preferred choice for organizations dealing with massive datasets. The unique feature of Throughput lies in its ability to accelerate data processing tasks, improving overall operational productivity. However, high throughput may also lead to increased resource consumption, requiring meticulous resource management strategies.
Latency
Latency, another essential performance metric, indicates the delay between submitting a query and receiving a response. Low latency is highly desirable in real-time data processing environments as it ensures quick insights and decision-making. The distinctive characteristic of Latency is its impact on the speed and responsiveness of data analytics processes. While low latency enhances user experience and operational efficiency, excessive emphasis on minimizing latency can result in complex and costly infrastructure configurations.
Resource Utilization
Resource Utilization measures the efficiency of hardware and software resources utilized by a big data platform to execute tasks. Optimized resource utilization leads to better cost-effectiveness and scalability, as it ensures maximum output with minimal resource wastage. The key feature of Resource Utilization lies in its ability to optimize resource allocation dynamically based on workload requirements, enhancing performance and operational flexibility. However, inefficient resource utilization can lead to bottlenecks, slowdowns, and increased operational costs.
Scalability and Flexibility
Handling Large Volumes
The ability of a big data platform to handle large volumes of data efficiently is crucial for organizations dealing with exponential data growth. Platforms with robust handling capabilities can process petabytes of data seamlessly, enabling organizations to derive valuable insights from vast datasets. The defining characteristic of Handling Large Volumes is its scalability to accommodate increasing data volumes without compromising performance or data integrity. Nevertheless, scaling up infrastructure to handle large data volumes necessitates careful capacity planning and resource provisioning to prevent performance degradation.
Adaptability to Diverse Workloads
Adaptability to Diverse Workloads signifies the platform's capability to support various types of workloads, ranging from batch processing to real-time analytics. Platforms that can adapt flexibly to changing workload requirements offer operational versatility and agility, catering to the dynamic needs of modern businesses. The unique feature of Adaptability to Diverse Workloads lies in its capacity to optimize performance based on workload characteristics, ensuring efficient resource allocation and task prioritization. However, managing diverse workloads effectively requires thoughtful workload scheduling, resource allocation mechanisms, and monitoring strategies.
Ease of Use and Integration
User-Friendly Interfaces
User-Friendly Interfaces play a vital role in simplifying platform interactions for users across diverse skill levels. Intuitive interfaces enhance user productivity, reduce training overhead, and promote self-service data analytics tasks. The key characteristic of User-Friendly Interfaces is their focus on usability, accessibility, and user experience, ensuring seamless navigation and task execution. Nevertheless, designing user-friendly interfaces requires a fine balance between simplicity and functionality to cater to the varying needs of users effectively.
Compatibility with External Tools
Compatibility with External Tools enhances the interoperability of big data platforms with a wide array of third-party tools and applications, facilitating seamless data integration and analysis workflows. Platforms that offer extensive compatibility options enable organizations to leverage existing tools and technologies, streamlining data processes and enhancing productivity. The unique feature of Compatibility with External Tools lies in its ability to support custom integrations, data pipelines, and analytics workflows, fostering a collaborative and integrated data environment. However, ensuring compatibility with external tools requires robust API support, data format compatibility, and version control mechanisms to prevent integration conflicts.
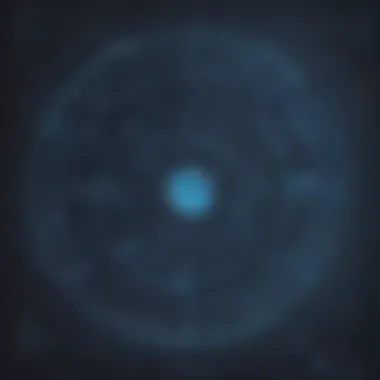
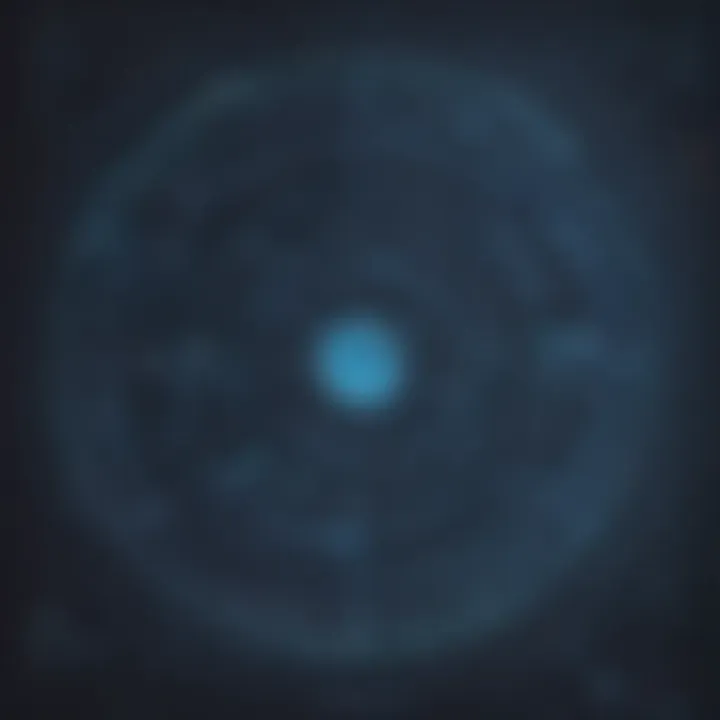
Use Case Scenarios
In this article, it is quintessential to delve into the Use Case Scenarios of big data platforms, particularly focusing on their practical applications and relevance in the realm of data management and analytics. Understanding the Use Case Scenarios provides valuable insights into how these platforms can address different business needs and challenges efficiently.
Enterprise Data Processing
Batch Processing
Batch Processing plays a pivotal role in the overall data processing landscape by facilitating the processing of large volumes of data in scheduled batches. Its key characteristic lies in the ability to process high volumes of data without the need for real-time response, making it a preferred choice for scenarios where data can be processed in non-real time. The unique feature of Batch Processing is its capability to handle complex data transformations and calculations efficiently, although it may introduce latency in data processing timelines.
Real-Time Analytics
Real-Time Analytics is instrumental in enabling businesses to derive immediate insights from incoming data streams, fostering quick decision-making processes. Its key characteristic revolves around processing data instantaneously as it arrives, allowing for up-to-the-moment insights. Real-Time Analytics is a beneficial choice when businesses require immediate data analysis for timely interventions. Its unique feature lies in the ability to provide instant feedback on evolving data trends, yet it may pose challenges in handling extremely high data volumes swiftly.
IoT Data Management
Sensor Data Handling
Sensor Data Handling plays a crucial role in managing the vast streams of data generated by IoT devices and sensors, ensuring efficient collection, storage, and processing of sensor data. Its key characteristic lies in the ability to capture real-time data from sensors and devices, providing valuable insights into operational activities. Sensor Data Handling is a popular choice for IoT scenarios due to its capacity to extract actionable intelligence from sensor data swiftly. The unique feature of Sensor Data Handling is its capability to support real-time data analysis, yet it may face challenges with data accuracy and reliability.
Predictive Maintenance
Predictive Maintenance empowers businesses to forecast potential equipment failures based on historical data patterns, enabling proactive maintenance strategies. Its key characteristic revolves around using advanced analytics and machine learning techniques to predict equipment failures before they occur, thus reducing downtime and operational costs. Predictive Maintenance is a popular choice for industries reliant on machinery and equipment for operational success. The unique feature of Predictive Maintenance is its predictive modeling capabilities, though it may require extensive historical data for accurate predictions.
E-Commerce Analytics
Customer Segmentation
Customer Segmentation aims to categorize customers into distinct groups based on shared characteristics, leading to personalized marketing and sales strategies. Its key characteristic lies in dividing customers into segments for targeted marketing efforts and product recommendations, enhancing customer engagement and retention. Customer Segmentation is a beneficial choice for e-commerce businesses seeking to tailor their offerings to specific customer groups. The unique feature of Customer Segmentation is its ability to uncover hidden patterns in customer behavior, yet it may lead to oversimplification of customer preferences.
Recommendation Systems
Recommendation Systems are essential in predicting customer preferences and recommending relevant products or services based on past behaviors and interactions. Its key characteristic revolves around utilizing algorithms to provide personalized recommendations, enhancing customer satisfaction and loyalty. Recommendation Systems are a popular choice in e-commerce for increasing sales and customer engagement. The unique feature of Recommendation Systems is their ability to improve user experience through personalized suggestions, although they may encounter challenges in handling diverse product catalogs and evolving customer preferences.
Conclusion
In the realm of big data platforms, the Conclusion serves as the pivotal segment where all the threads woven throughout this comprehensive comparison converge. Understanding the nuances of various platforms like Hadoop and Spark, and how they align with diverse business needs, is imperative for making informed decisions. The Conclusion encapsulates the key takeaways, emphasizing the significance of meticulous platform selection for optimizing data management and analytics processes. In this conclusive section, we dissect the essential elements, benefits, and considerations surrounding big data platforms, shedding light on their impact on modern enterprises.
Final Thoughts on Big Data Platforms
Considerations for Selection
Delving into the Considerations for Selection within the big data landscape unveils a critical undertaking in this article. This aspect dissects the intricacies involved in choosing the ideal platform tailored to specific organizational requirements. Emphasizing scalability, performance, and cost-efficiency, this segment offers a detailed exploration of the key characteristics defining platform selection. Expounding on the elaborate nature of this decision-making process highlights the nuanced approach needed for aligning platforms with business objectives seamlessly. Investigating the unique feature sets of various platforms underscores the importance of balancing features with operational practicality, ensuring a deliberate and strategic selection process.
Future Trends
The exploration of Future Trends within the big data domain catapults readers into the evolving landscape of data technologies. This segment forecasts the trajectory of big data platforms, predicting advancements that shape the future of data analytics. Highlighting innovation, interoperability, and automation, this section elucidates the key drivers propelling the industry towards greater efficiency and agility. Scrutinizing the unique features underpinning future trends illuminates the potential benefits and pitfalls that organizations may encounter in adopting cutting-edge technologies. Observing the transformative potential of emerging trends underscores the imperative for continual adaptation and optimization within the dynamic sphere of big data platforms.