Manager Master Data Management: Strategies for Success
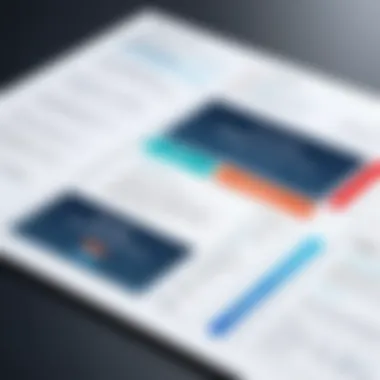
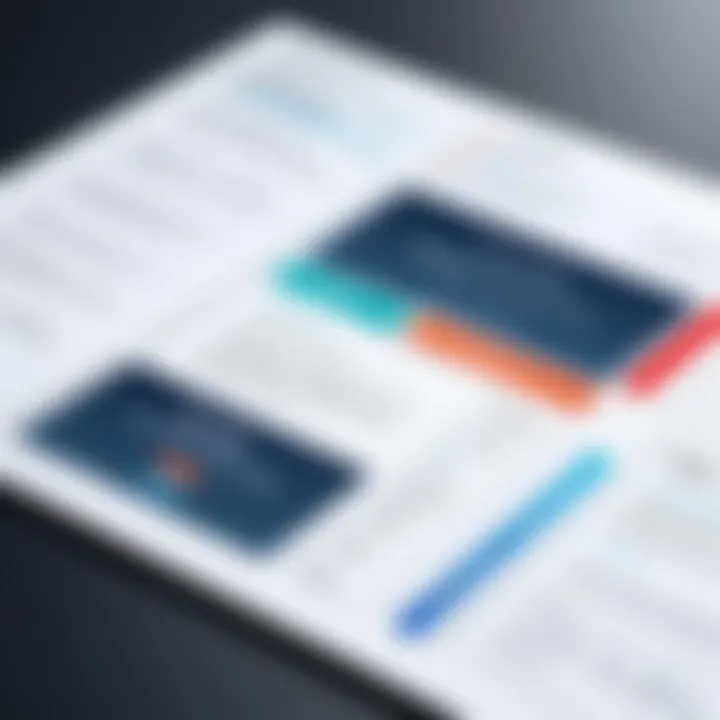
Intro
Master Data Management (MDM) is a vital aspect of modern organizational strategy. Managers play a significant role in ensuring that an organization's master data is accurate, consistent, and accessible. This governance is crucial as the quality of data directly impacts decision-making and operational efficiency. Effective MDM requires a structured approach, integrating processes, technologies, and people across the organization.
Understanding Storage, Security, or Networking Concepts
Master Data Management is not only about managing data but also ensuring its security and effective storage. Understanding basic concepts related to storage and security is essential for any manager involved in data governance.
Intro to the basics of storage, security, or networking
Data storage refers to how information is encoded, maintained, and retrieved. It can be divided into several categories: cloud storage, on-premises storage, and hybrid solutions. Security, on the other hand, involves protecting data from unauthorized access or alterations. Networking is the backbone that enables data transfer across systems. Knowing these disciplines is important in MDM.
Key terminology and definitions in the field
- Master Data: Core data essential for operations within an organization.
- Data Governance: Framework establishing policies and procedures for data management.
- Data Quality: The accuracy and reliability of data over its lifecycle.
- Data Stewardship: The responsibility for managing data assets and ensuring quality.
Overview of important concepts and technologies
Understanding concepts such as data lineage and data lifecycle management can guide managers in making informed MDM decisions. Technologies involved in MDM include data integration tools, data quality software, and data orchestration platforms. These ensure that master data is consistent and trustworthy across various systems.
Best Practices and Tips for Storage, Security, or Networking
To ensure effective MDM, managers can adopt various best practices.
- Invest in Data Quality Tools: Utilize software designed to detect and correct data inconsistencies.
- Implement Strong Security Measures: Enforce policies that protect data assets from threats.
- Regular Audits: Perform regular checks to assess the quality and security of master data.
Implementing these strategies will guide managers in enhancing data governance, ultimately leading to improved business outcomes.
Industry Trends and Updates
Staying updated on industry trends is essential for managers aiming to stay ahead in MDM.
- Latest trends in storage technologies: The migration to cloud storage is becoming more prevalent.
- Cybersecurity threats and solutions: The rise of ransomware attacks demands stronger protection measures.
- Networking innovations: Software-Defined Networking (SDN) is changing how organizations manage their networks.
By aligning with these trends, managers can ensure that their MDM practices are contemporary and effective.
Case Studies and Success Stories
Real-life examples can provide insight into effective MDM practices.
"Organizations that invested in comprehensive data governance saw an improvement in overall data quality and operational efficiency, according to industry reports."
Examples of successful data governance implementations include major retailers, which improved their sales projections through enhanced master data accuracy. Learning from such cases can inform future strategies.
Reviews and Comparison of Tools and Products
When selecting tools for MDM, a thorough review and comparison are crucial. Tools like Informatica and SAP Master Data Governance are popular choices in the industry. Evaluating these against organizational needs will help in making informed decisions.
By focusing on the points discussed, managers can formulate a robust plan for Master Data Management. This is not just about maintaining data but about creating an environment where data can truly drive emerging business strategies.
Understanding Master Data Management
Master Data Management (MDM) serves as a critical framework for organizations striving to maintain consistent, accurate, and reliable data across their operations. It ensures that the core data entities, such as customers, products, and suppliers, are defined and managed uniformly. The significance of MDM cannot be overstated in contemporary business contexts, where data-driven decision-making is paramount. An understanding of MDM allows managers to harness the power of data effectively, contributing to better operational efficiency and strategic insights.
In recognizing the importance of understanding MDM, several key elements come to the forefront:
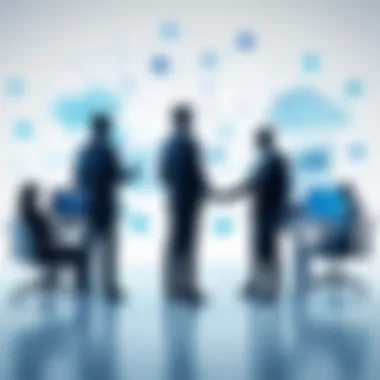
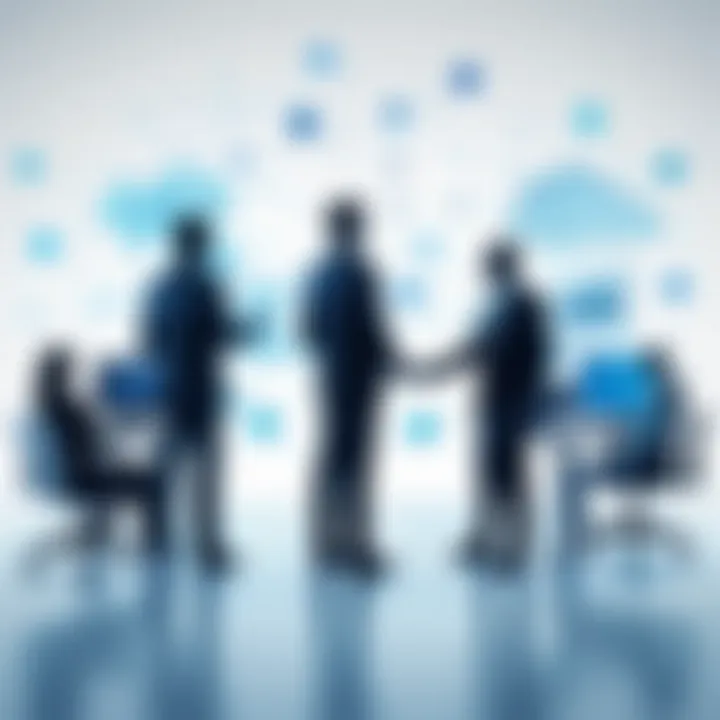
- Enhanced Data Quality: By focusing on mastering data accuracy, organizations can improve the integrity of information, leading to better business outcomes.
- Informed Decision-Making: Reliable master data enables informative analysis and insights, which drives smart strategies and operational improvements.
- Regulatory Compliance: Companies must adhere to various standards and regulations that require accuracy and correctness in their data management practices.
"Effective Master Data Management is not merely a task, but a strategic enabler for organizations, ultimately influencing profitability and growth."
Understanding MDM helps managers not only to mitigate risks associated with poor data quality but also to recognize opportunities for leveraging data as a strategic asset. The following subsections detail essential aspects of MDM in a structured manner.
The Role of Managers in
Effective Master Data Management (MDM) requires strong leadership from managers to ensure the integrity and usability of data. This section delves into the importance of managerial roles in MDM, emphasizing strategic leadership, collaboration, and resource allocation. The responsibilities of managers extend beyond mere oversight; they are crucial in developing frameworks that promote accountability and data stewardship, fostering an environment where quality data can drive business decisions.
Managers must comprehend that data governance is not merely a technical endeavor; it is a strategic imperative that aligns with overall business goals.
Strategic Leadership in Data Management
Strategic leadership is vital in navigating the complexities of data management. Managers need to articulate a clear vision for data governance which aligns with organizational objectives. This vision should encompass not only the aspirations for data quality but also the methodologies for achieving these aims. Effective leaders will cultivate a culture where data is valued and treated as an asset, supporting initiatives that enhance data governance practices.
Moreover, leaders must clearly communicate the significance of MDM across the organization. By promoting an understanding of why accurate and cohesive data matters, they lay the groundwork for organizational buy-in. Leadership in data management includes:
- Setting clear data governance policies.
- Encouraging accountability across teams.
- Supporting ongoing training for staff involved in data handling.
Collaborative Approach to Data Governance
Collaboration is essential for successful MDM. Managers must foster teamwork across different departments to break down data silos, which are significant barriers to effective data governance. When departments operate in isolation, they create inconsistencies that undermine the value of the data generated.
Implementing a collaborative framework involves creating cross-functional teams responsible for data quality. Regular meetings and feedback loops ensure that everyone is aligned on the objectives and understands their roles in maintaining high data standards. This approach benefits organizations by:
- Enhancing communication regarding data-related issues.
- Streamlining data collection and processing methods.
- Encouraging shared accountability among all stakeholders.
Resource Allocation for Initiatives
For MDM initiatives to succeed, adequate resource allocation is crucial. Managers must strategically allocate both financial and human resources to initiatives aimed at improving data quality and governance. They should evaluate the existing resources to determine gaps in capabilities and identify areas that require additional investment.
It is important for managers to:
- Prioritize funding for technologies that facilitate data integration and quality.
- Ensure that teams have access to the right tools for data governance.
- Support talent acquisition or training that enhances the organization's data management capabilities.
In summation, the role of managers in MDM combines strategic vision with operational capabilities. By leading effectively, fostering collaborative environments, and wisely allocating resources, managers can facilitate better data governance and unlock the full potential of master data.
Developing a Master Data Strategy
In any organization, developing a master data strategy is pivotal for maintaining data integrity and enhancing decision-making processes. A master data strategy outlines the approach a company will adopt to manage its critical data consistently. This strategy integrates various components that work together to optimize data usage and governance. By implementing a sound strategy, organizations can ensure data accuracy, reduce redundancy, and promote a unified view of their data assets. The benefits of having a structured master data strategy include improved efficiency, increased accountability, and greater compliance with regulations.
Assessing Current Data Management Practices
A thorough assessment of current data management practices is the first step in establishing a master data strategy. This involves evaluating existing processes, systems, and data quality. Managers should identify gaps that lead to poor data quality or inefficient data handling. Additionally, understanding the tools currently in use helps in determining their effectiveness. Organizations may employ data profiling techniques to analyze data sets for accuracy, completeness, and consistency. This assessment phase not only uncovers issues but also highlights strengths to build upon in the new strategy.
Establishing Data Quality Metrics
Establishing clear data quality metrics is essential for tracking the effectiveness of the master data strategy. Metrics such as accuracy, completeness, consistency, and timeliness of data must be defined and agreed upon by all stakeholders involved. For instance, an accuracy metric could measure how many of the records in the system are free from errors. Moreover, organizations should consider setting benchmarks for these metrics, allowing them to evaluate progress over time. Regular reporting on these metrics keeps everyone informed and accountable, ensuring ongoing commitment to data quality.
Building a Data Governance Framework
A well-structured data governance framework acts as the backbone of a master data strategy. It establishes the policies, processes, and standards for managing data within the organization. This framework should define the roles and responsibilities of all personnel involved in data management, ensuring that everyone understands their part in maintaining data integrity. Collaboration between IT and business units is crucial for creating a governance framework that meets diverse needs. Inclusion of relevant stakeholders in the development process can lead to broader acceptance and compliance. Hence, with a cohesive data governance framework, organizations can systematically address data-related challenges, promote best practices, and drive continual improvement.
"Data governance is not merely a set of rules. It encompasses a cultural shift towards valuing and managing data as an important asset."
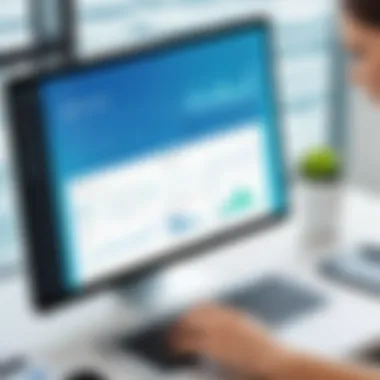
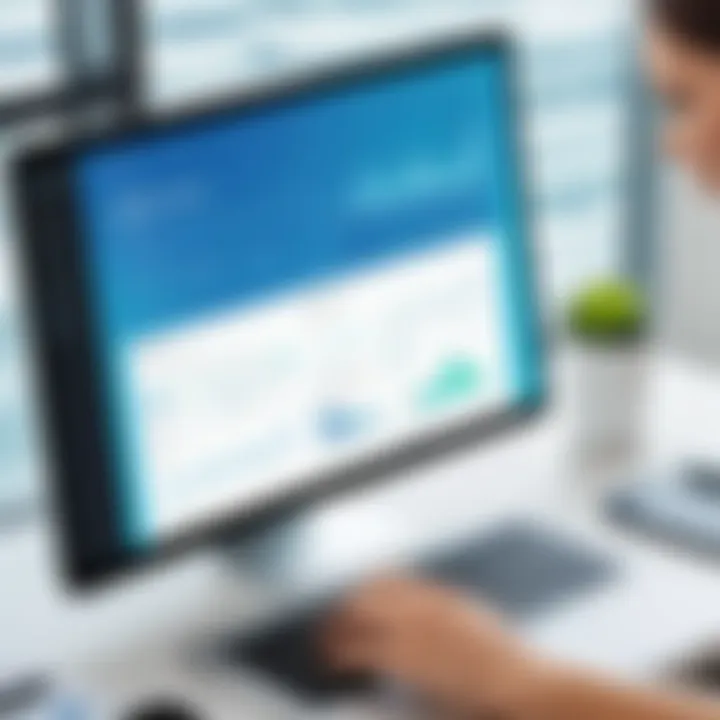
Overall, developing a master data strategy sets the foundation for effective data governance. By assessing current practices, establishing metrics, and building a governance framework, organizations position themselves to leverage their data effectively, driving better outcomes and supporting their overall business strategy.
Challenges in Master Data Management
Master Data Management (MDM) faces several challenges that can hinder the effectiveness of data governance. These challenges are critical to understand for managers seeking to enhance organizational data accuracy and integrity. Each challenge presents unique considerations that require strategic responses. Addressing these challenges can lead to more cohesive data practices, ultimately benefiting the entire organization.
Data Silos Across Departments
Data silos refer to isolated data repositories that prevent seamless flow of information across departments. In many organizations, different departments such as sales, marketing, finance, and operations maintain their own separate data collection systems. This leads to duplicate data entries, inconsistencies in data precision, and significant gaps in meaningful insights.
To mitigate the impact of data silos, organizations must encourage a culture of collaboration and communication. This involves creating a centralized data platform where all departments can access and share master data efficiently. Furthermore, implementing clear protocols for data entry and maintenance helps ensure consistency in the data across various departments. This collaborative approach not only enhances data quality but also fosters a more holistic view of business operations, facilitating better decision-making.
Resistance to Change within Organizations
Resistance to change is a prevalent issue that impacts the implementation of MDM initiatives. Employees often feel apprehensive towards adopting new technologies or processes due to a fear of the unknown or concerns about job security. This resistance can lead to sluggish implementation and, at times, complete failure of MDM strategies.
To counteract this resistance, managers should prioritize change management strategies. This includes engaging employees in the MDM process early on and providing adequate training about new processes and tools. By demonstrating the benefits of these changes, such as improved efficiency and data accuracy, managers can help foster a more accepting environment. Moreover, recognizing and rewarding employees who actively participate in transitions can significantly alleviate resistance and promote a culture of innovation.
Technological Constraints and Limitations
The integration of technology is pivotal for effective MDM. However, many organizations operate with outdated systems or lack the necessary tools to manage master data effectively. These technological constraints inhibit the ability to maintain data consistency and quality.
Organizations should regularly assess their technological frameworks to identify gaps impacting data management. Investing in advanced MDM solutions, which often include automation and analytics capabilities, can yield significant long-term benefits. Furthermore, ensuring that existing systems can integrate with new tools will allow for a more adaptive and flexible data management strategy. Up-to-date technology not only supports improved data governance but also facilitates better responsiveness to changing market demands.
"Effective data governance in MDM is not only about having the right tools but also ensuring that the culture supports data management initiatives."
Implementing Solutions
Implementing Master Data Management (MDM) solutions is a pivotal step for organizations aiming to enhance their data governance practices. This process not only helps in establishing a unified view of data but also ensures that data is consistent, accurate, and reliable across various departments. Effective MDM solutions can significantly improve operational efficiency and decision-making capabilities.
Selecting the Right Tools
Choosing the appropriate MDM tools is crucial. These tools vary in functionality and capabilities. Organizations should select tools based on several criteria:
- Usability: Tools should be user-friendly to facilitate adoption among staff.
- Scalability: They must accommodate future growth in data volume and complexity.
- Integration capabilities: The tools need to integrate seamlessly with existing systems.
- Cost: Organizations should evaluate the total cost of ownership versus benefits gained.
It is also important to ensure that selected tools can support specific business needs. For instance, if an organization deals with multiple data sources, it should look for MDM tools that excel in data consolidation and synchronization.
Integration with Existing Systems
Integrating MDM solutions with current systems is more than just a technical challenge; it requires a strategic approach. Integration ensures that all systems communicate effectively. This is vital for maintaining data integrity and consistency.
Key considerations for integration include:
- Data Mapping: Understanding how data flows between systems is essential for smooth integration.
- APIs and Connectors: Tools should support standard APIs for easy integration with third-party applications.
- Testing and Validation: Before full-scale deployment, thorough testing must be conducted to ensure that data syncs correctly and functions as intended.
An efficient integration process minimizes disruptions and maximizes the benefits of MDM solutions.
Security Considerations in
Security is another key aspect when implementing MDM solutions. As organizations consolidate their data, they open themselves up to potential risks and vulnerabilities. Therefore, data security measures must be put in place. Important considerations include:
- Access Controls: Only authorized personnel should access sensitive data.
- Encryption: Data should be encrypted both in transit and at rest to protect it from unauthorized access.
- Regular Audits: Performing regular security audits helps identify vulnerabilities and ensures compliance with relevant regulations.
Security must never be an afterthought in MDM implementation. A proactive approach to data protection is essential to safeguard organizational assets.
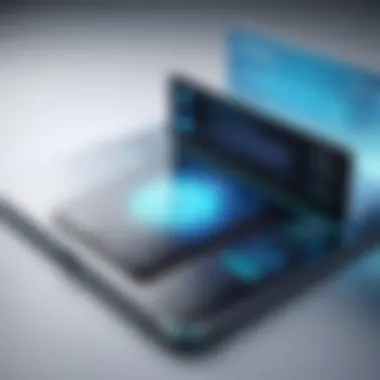
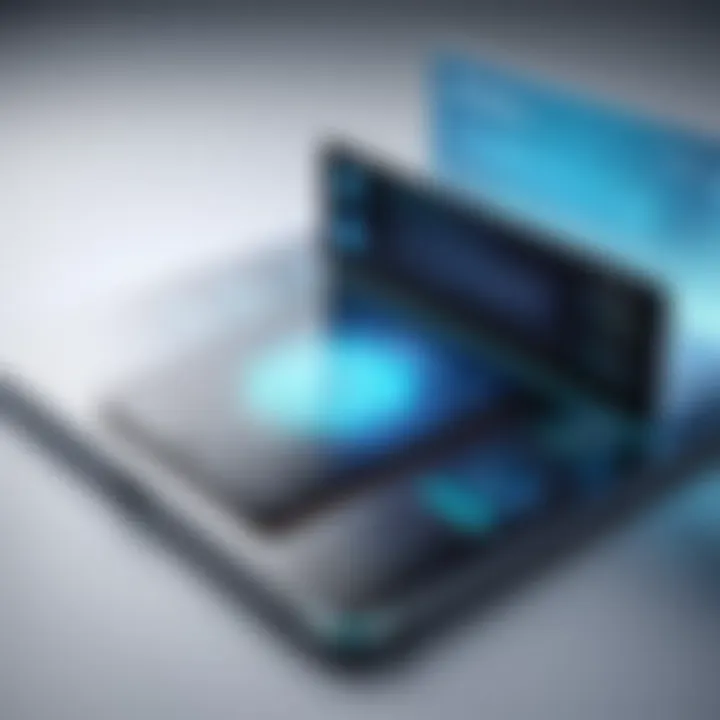
Evaluating Success
Evaluating the success of Master Data Management (MDM) is crucial for ongoing improvements and ensuring that data governance delivers intended outcomes. MDM success evaluation allows organizations to measure the effectiveness of their strategies and practices. Successful MDM results in enhanced data accuracy, consistency, and integrity across the organization. This is not just an operational need; it significantly influences decision-making processes. Moreover, monitoring MDM success can help in identifying areas that require adjustments or enhancements.
The evaluation process helps managers to understand if the MDM strategies align with business goals and if they contribute to overall operational efficiency. In essence, evaluating MDM success ensures money, time, and resources spent on data management yield tangible benefits for the organization.
Key Performance Indicators for
Defining Key Performance Indicators (KPIs) is vital for measuring MDM success. These indicators provide quantifiable metrics to assess how effectively MDM is achieving its objectives. Some critical KPIs include:
- Data Quality Scores: Measures the accuracy and completeness of the master data.
- Data Duplication Rates: Tracks the number of duplicate records existing within databases.
- User Adoption Rates: Monitors how many users actively utilize the MDM system.
- Data Access and Retrieval Speed: Assesses how quickly users can access necessary data.
Establishing these KPIs supports performance scrutiny and facilitates targeted improvements in the MDM framework.
Feedback Mechanisms for Continuous Improvement
Feedback mechanisms are essential for fostering an environment of continuous improvement within MDM practices. They allow for real-time data collection from users interacting with the system. Regular input from data stewards and end-users can lead to identifying bottlenecks, challenges, or areas of concern. This feedback can come in various forms:
- Surveys and Questionnaires to gather user experiences and satisfaction.
- Regular Meetings for discussing MDM strategy successes and challenges.
- Performance Review Sessions that focus on analyzing data management effectiveness.
Utilizing these mechanisms helps in modifying procedures and making data governance more responsive to users' needs.
Adjusting Strategies Based on Outcomes
Once KPIs are established and feedback is collected, it's crucial to adjust strategies based on outcomes. If the evaluation shows underperformance in certain areas, managers must adapt their approach. This might include:
- Refining Data Governance Policies to close gaps in data quality.
- Restructuring Training Programs to improve user adoption and data handling practices.
- Investing in New Technology or tools that enhance data management capabilities.
By being willing to change and innovate based on evaluated outcomes, managers can ensure that their MDM efforts remain aligned with the organizationโs evolving data governance needs.
"The ability to evaluate and adapt MDM strategies is key to maintaining the integrity and reliability of organizational data."
The Future of Master Data Management
In the rapidly evolving landscape of data management, the future of Master Data Management (MDM) holds significant importance. As organizations strive for better data governance, understanding the upcoming trends and technological advancements becomes essential. Effective MDM not only ensures data accuracy and consistency but also facilitates informed decision-making across business units. In this section, we will examine emerging trends, the influence of artificial intelligence, and the role of cloud computing in shaping the future of MDM.
Emerging Trends in
The field of Master Data Management is witnessing various trends that promise to revolutionize how organizations handle their data. Some of the key trends include:
- Data Fabric Architecture: This concept enhances data integration across platforms, allowing for a unified approach to data management and governance. Data fabric offers flexibility and promotes easier access to high-quality data.
- Automated Data Governance: With increasing volumes of data, manual governance becomes impractical. Automation tools are now being utilized to monitor data quality, enabling faster identification of issues and ensuring compliance.
- Self-Service Data Access: Growing demand for real-time insights is pushing organizations toward enabling self-service platforms. Business users can access data without IT intervention, speeding up decision-making processes.
These trends not only improve efficiency but also make organizations more agile, responding better to changing market demands.
Impact of Artificial Intelligence on
Artificial Intelligence is increasingly integral to Master Data Management strategies. AI enhances MDM in several ways:
- Data Cleaning and Enrichment: AI algorithms can automatically detect and rectify inconsistencies within datasets, improving overall data quality by reducing human errors.
- Predictive Analytics: MDM systems powered by AI can analyze trends and forecast future data needs, allowing organizations to make data-driven decisions proactively.
- Natural Language Processing: This facilitates better interaction with data. AI can use natural language queries that make data retrieval easier for non-technical users.
As a result, organizations leveraging AI in MDM can expect improved accuracy, reduced operational costs, and increased speed in data processing.
The Role of Cloud Computing in
Cloud computing is redefining how Master Data Management is implemented. Its role can be summarized in several aspects:
- Scalability: Cloud solutions offer organizations the flexibility to scale their data management services as needed. This is crucial as data volumes continue to grow.
- Cost Efficiency: By utilizing cloud platforms, companies can often reduce costs associated with on-premise infrastructure and maintenance. This allows for reallocating resources to more critical areas.
- Collaboration: Cloud environments support better collaboration across departments and geographies, enabling seamless data sharing and governance between teams.
The adoption of cloud computing facilitates modern MDM initiatives, aligning with organizational goals of efficiency and strategic data management.
"The integration of cloud services, AI, and emerging trends defines the future of Master Data Management, enabling organizations to enhance data governance and maintain competitiveness."