Mastering Data Governance for Organizational Success: Insights from McKinsey
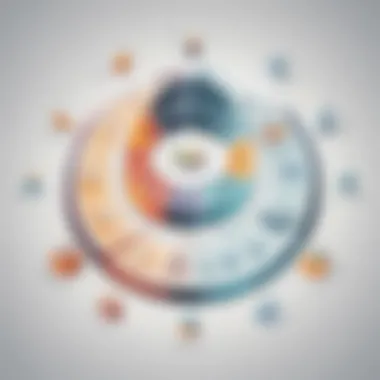
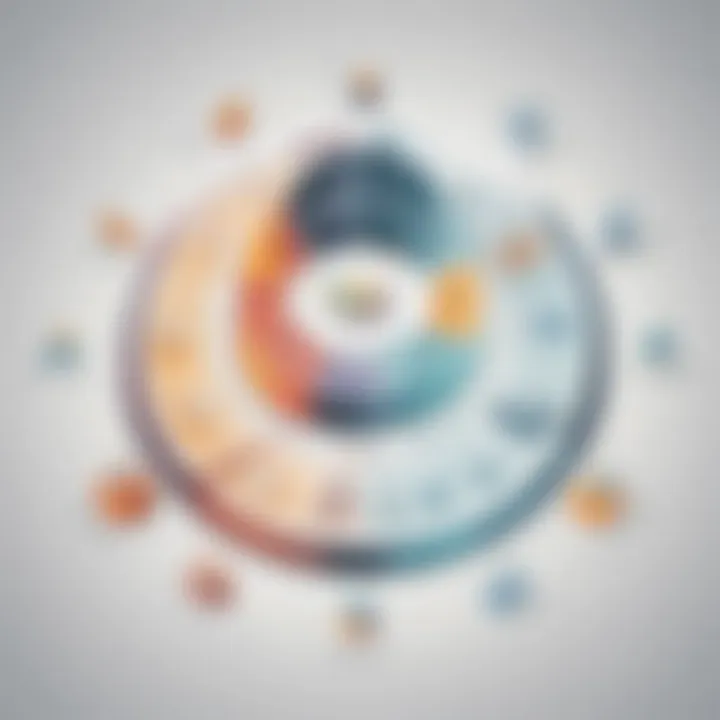
Understanding Data Governance Principles and Strategies
As your journey into the intricate realm of data governance unfolds, it is crucial to grasp the fundamental principles and strategies that underpin effective data management. Data governance, often likened to the custodian of organizational data assets, plays a pivotal role in driving success through structured and disciplined management practices. Exploring key terms such as data stewardship, data quality, and data ownership sets the stage for a comprehensive understanding of data governance. Moreover, an overview of essential concepts and technologies, including data classification, metadata management, and data access controls, lays a solid foundation for optimizing data governance frameworks.
Best Practices and Recommendations for Data Governance
Diving deeper into the intricacies of data governance unveils a tapestry of best practices and recommendations aimed at enhancing the efficiency and efficacy of data management processes. From establishing clear data policies and governance frameworks to implementing robust data monitoring and enforcement mechanisms, optimizing data governance entails a holistic approach that aligns with organizational objectives and regulatory requirements. Embracing data governance best practices not only fosters data integrity and accountability but also cultivates a culture of data-driven decision-making within the organization. Stay tuned as we unravel insightful tips for mastering the art of data governance and harnessing the full potential of your data assets.
Key Trends and Developments in Data Governance
In the dynamic landscape of data governance, staying abreast of the latest trends and developments is paramount to driving innovation and sustaining competitive advantage. Keep pace with emerging technologies such as AI-driven data governance tools, blockchain-enabled data security solutions, and advanced analytics platforms that revolutionize data management practices. Furthermore, vigilance against evolving cyber threats and regulatory changes is crucial in mitigating risks and ensuring compliance in an increasingly digitized world. Explore the cutting-edge trends in data governance that are reshaping the data landscape and revolutionizing the way organizations approach data governance.
Real-world Case Studies and Success Stories
Delve into real-world case studies and success stories that exemplify the transformative impact of effective data governance strategies in diverse organizational settings. From data-driven success stories showcasing substantial ROI and enhanced operational efficiencies to cautionary tales of data breaches and compliance failures, these case studies provide valuable insights into the practical application of data governance principles. Uncover the lessons learned from prominent cybersecurity incidents and data management challenges, offering a holistic view of the risks and rewards associated with data governance initiatives.
Evaluation and Comparison of Data Governance Tools
Navigate the nuanced landscape of data governance tools and solutions with comprehensive evaluations and comparisons that illuminate the strengths and limitations of various offerings. From data quality management platforms to data governance frameworks and compliance tools, evaluating the right suite of solutions is paramount to achieving data governance excellence. Uncover the key features, functionalities, and performance metrics of leading data governance tools, arming yourself with the knowledge needed to make informed decisions that drive organizational success in the ever-evolving data ecosystem.
Introduction to Data Governance
Data governance is a crucial aspect of modern business operations, ensuring that data is managed effectively to drive strategic decision-making and maintain data integrity. In the realm of data management, the ability to govern data assets efficiently can be a game-changer for companies seeking to leverage the power of their information resources. The key elements of data governance encompass establishing policies, ensuring data quality, and implementing robust security measures to safeguard sensitive information. By delving into the intricacies of data governance, organizations can optimize their data management processes and unlock the full potential of their data-driven initiatives.
Understanding Data Governance
Definition and Scope
The definition and scope of data governance revolve around the principles and practices involved in managing, using, and protecting data assets within an organization. It encompasses establishing processes for data management, defining roles and responsibilities, and ensuring compliance with regulatory requirements. The critical characteristic of defining clear guidelines for data handling and utilization sets the foundation for effective data governance. While providing a structured approach to data management, the definition and scope of data governance offer organizations a roadmap to streamline operations and enhance decision-making processes.
Importance in Modern Business
The importance of data governance in modern business cannot be overstated, as organizations increasingly rely on data-driven insights to gain a competitive edge. Implementing robust data governance practices is vital for ensuring data accuracy, consistency, and reliability across the organization. By instilling a culture of data governance, companies can mitigate risks associated with data misuse, enhance operational efficiency, and foster a culture of data-driven decision-making. Embracing data governance as a strategic priority aligns businesses with industry best practices, enabling them to adapt to the evolving landscape of data management effectively.
Evolution of Data Governance
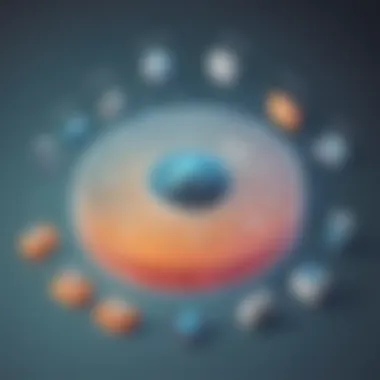
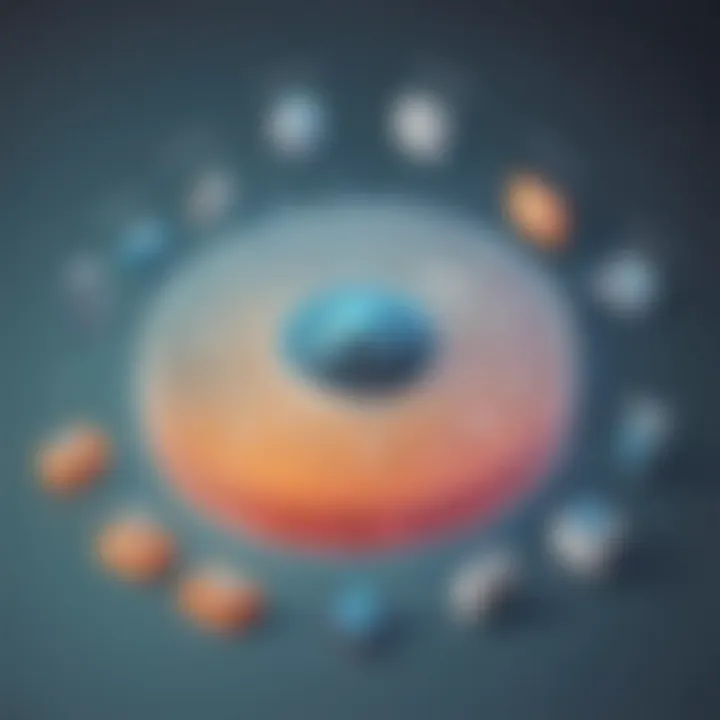
Historical Context
The historical context of data governance traces back to the early days of data management when organizations began recognizing the need to formalize processes for data handling. The evolution of data governance reflects the progression from rudimentary data storage methods to sophisticated data governance frameworks aimed at optimizing data utilization. By understanding the historical evolution of data governance, organizations can appreciate the transformative impact of data management practices and leverage historical insights to inform their current data governance strategy.
Current Trends
Stay current by exploring the latest trends in data governance, including the adoption of advanced technologies such as AI and machine learning to enhance data analytics capabilities. The current trends in data governance emphasize real-time data processing, data democratization, and agile data governance approaches to meet the dynamic needs of modern businesses. By staying abreast of current trends, organizations can future-proof their data governance initiatives and align them with emerging technologies and industry best practices to drive sustainable growth.
Key Components of Data Governance
Policy Development
Policy development plays a fundamental role in shaping data governance initiatives by establishing guidelines and protocols for data handling. The key characteristic of policy development lies in its ability to set the tone for data governance practices across the organization, ensuring consistency and compliance with data management standards. By developing comprehensive policies that outline data access, usage, and retention protocols, organizations can lay a strong foundation for effective data governance and promote data stewardship among employees.
Data Quality Management
Data quality management is essential for ensuring that data assets are accurate, reliable, and fit for purpose. The primary focus of data quality management is on improving data accuracy, completeness, and consistency to enable informed decision-making and enhance operational efficiency. By implementing robust data quality management processes, organizations can address data discrepancies, minimize data errors, and improve data reliability throughout the data lifecycle.
Data Security Measures
Data security measures are paramount in safeguarding sensitive information assets from unauthorized access, data breaches, and cyber threats. The key characteristic of data security measures lies in their ability to protect data integrity and confidentiality through encryption, access controls, and data monitoring practices. By implementing stringent data security measures, organizations can fortify their data infrastructure, comply with data privacy regulations, and instill trust among stakeholders regarding data protection.
McKinsey's Perspective on Data Governance
In this segment of the article, we delve into McKinsey's unique viewpoint on data governance. McKinsey offers a distinct approach that sets it apart in the realm of data management and strategic decision-making. Understanding McKinsey's stance is crucial for organizations looking to optimize their data governance practices and leverage data assets effectively. McKinsey's Perspective on Data Governance sheds light on innovative strategies and methodologies that can propel businesses towards success.
McKinsey's Approach to Data Governance
Framework Overview
McKinsey's framework for data governance is a meticulously crafted structure that encompasses key principles and guidelines for reliable data management. This framework offers a systematic approach to organizing, securing, and utilizing data assets within an organization. One notable characteristic of McKinsey's framework is its emphasis on scalability and adaptability, ensuring that it can cater to the evolving needs of businesses in the digital age. The unique feature of this framework lies in its integration of data governance with overall business strategies, enhancing alignment and coherence in decision-making processes.
Case Studies
McKinsey's case studies present real-world applications of its data governance framework, illustrating its effectiveness in diverse scenarios. These case studies highlight the practical benefits of implementing McKinsey's approach, showcasing tangible outcomes and success stories from organizations that have embraced this model. By examining these case studies, businesses can gain valuable insights into best practices and potential challenges when implementing McKinsey's data governance framework. These real-life examples serve as evidence of the framework's advantages and its ability to drive organizational excellence.
Benefits of Implementing McKinsey's Data Governance Model
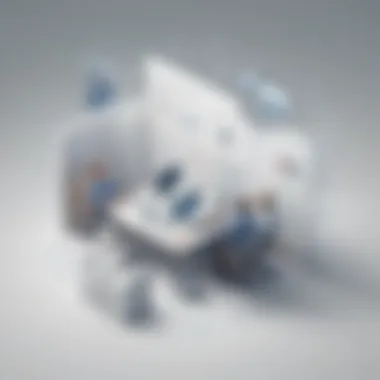
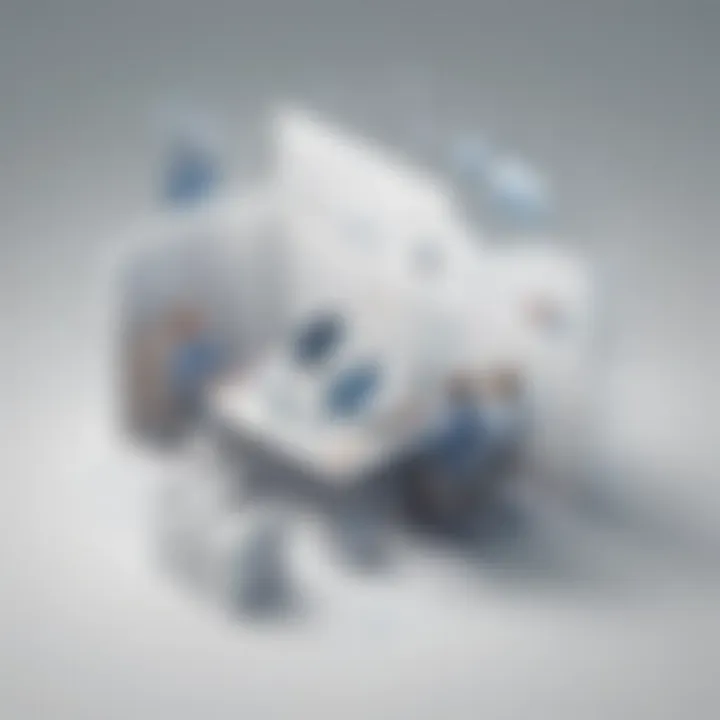
Enhanced Decision-Making
The implementation of McKinsey's data governance model enhances decision-making processes by providing decision-makers with accurate and timely data insights. This critical aspect contributes to informed and strategic decision-making, leading to better business outcomes. The unique feature of this benefit is its ability to enable data-driven decision-making at all levels of the organization, fostering a culture of data literacy and evidence-based strategies. However, one should be cautious about potential challenges related to data interpretation and bias in decision-making processes.
Efficiency Gains
McKinsey's data governance model drives efficiency gains by streamlining data processes and eliminating redundancies. This results in optimized workflows, reduced operational costs, and enhanced productivity across the organization. The key characteristic of this benefit is its role in enhancing operational agility and responsiveness to market dynamics. While the model offers substantial efficiency improvements, organizations must remain vigilant against potential bottlenecks or resistance to change that could hinder its full realization.
Risk Mitigation
Implementing McKinsey's data governance model aids in mitigating risks associated with data breaches, compliance violations, and operational disruptions. By establishing robust security measures and regulatory compliance protocols, organizations can safeguard their data assets and reputational integrity. The unique feature of risk mitigation through this model lies in its proactive approach to identifying and addressing potential vulnerabilities before they escalate. However, organizations should continually reassess and adapt their risk mitigation strategies to combat evolving cyber threats and regulatory changes effectively.
Best Practices in Data Governance
In this section, we delve into the critical topic of Best Practices in Data Governance within the overarching context of McKinsey's insights. Data governance, being the foundation of effective data management, plays a pivotal role in organizational success. By implementing best practices in data governance, companies can streamline processes, enhance decision-making, and ensure regulatory compliance.
When establishing data governance structures, several key elements come into play. The first aspect to consider is Organizational Alignment. This entails ensuring that data governance initiatives align with the overall organizational objectives. Organizational Alignment serves as a guiding principle, ensuring that every aspect of data governance contributes to the strategic goals of the company. While this approach can lead to improved efficiency and clarity in decision-making processes, challenges may arise in terms of changing organizational dynamics and resistance to alignment efforts.
Another crucial component of data governance structures is Roles and Responsibilities. Defining clear roles and responsibilities within the data governance framework is essential for accountability and operational efficiency. By assigning specific roles to individuals or teams, organizations can ensure that data governance processes are effectively executed and monitored. However, delineating roles and responsibilities may sometimes lead to conflicts of interest or gaps in accountability if not clearly defined.
Moving on to Data Governance Metrics and Measurement, one must consider key performance indicators (KPIs) for data governance. KPIs provide organizations with quantifiable metrics to track the effectiveness of their data governance initiatives. By setting measurable goals and monitoring KPIs, companies can assess the impact of data governance on their business outcomes. While KPIs offer valuable insights, selecting the most relevant metrics and aligning them with organizational objectives can be a complex process.
Additionally, Performance Evaluation is crucial in evaluating the success of data governance efforts. By conducting regular performance evaluations, organizations can identify areas for improvement and optimize their data governance strategies. Performance evaluation allows companies to measure the efficiency of their data governance processes and make informed decisions based on tangible results. However, challenges may arise in effectively measuring performance due to diverse data sources and varied organizational requirements.
In the realm of Ensuring Regulatory Compliance, two key aspects take center stage: GDPR and Data Privacy Laws. GDPR, or General Data Protection Regulation, is a crucial regulatory framework that governs data protection and privacy for individuals within the European Union. Compliance with GDPR is essential for organizations handling EU citizen data, as non-compliance can result in severe penalties. On the other hand, Data Privacy Laws vary across regions and industries, requiring companies to navigate a complex landscape of regulations to ensure data security and compliance. Comprehending the nuances of GDPR and Data Privacy Laws is imperative for implementing robust data governance practices and safeguarding sensitive information.
Challenges in Data Governance
In the intricate landscape of data governance, challenges play a pivotal role. These challenges are not mere obstacles but serve as opportunities for growth and enhancement. Addressing these challenges is a critical aspect of ensuring that data governance strategies are robust and effective. By acknowledging and overcoming these challenges, organizations can optimize their processes, drive innovation, and strengthen data utilization.
Overcoming Data Silos
Data silos, a common challenge in data governance, hinder seamless information flow and integration across different departments within an organization. Interdepartmental collaboration emerges as a crucial solution to break down these silos. This collaborative approach involves teams and units working together towards shared objectives, pooling resources, and sharing insights and data across boundaries. The key essence of interdepartmental collaboration lies in fostering transparency, communication, and synergy among diverse teams, leading to improved decision-making and operational efficiency.
Interdepartmental Collaboration
Interdepartmental collaboration thrives on the synergy created by multidisciplinary teams working together towards common goals. Its unique feature lies in its ability to bridge the gap between different functional areas, promoting a holistic view of organizational processes and data utilization. This collaboration paves the way for a comprehensive understanding of data governance challenges and facilitates the alignment of strategies and resources across departments, driving collective success.
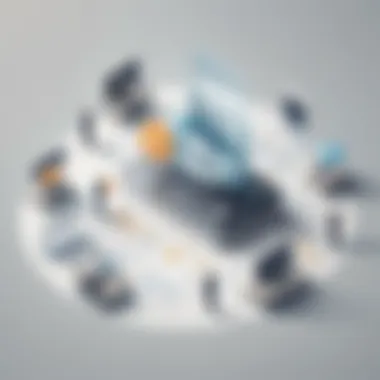
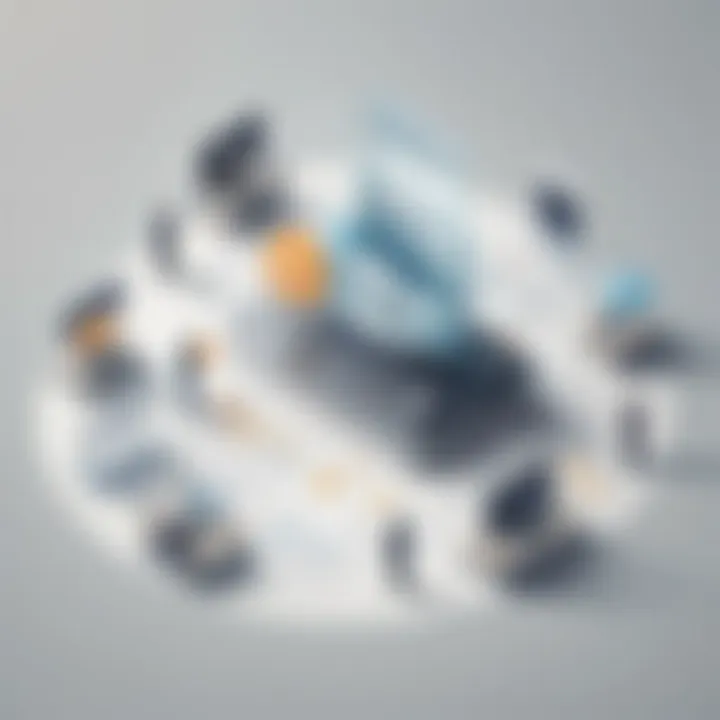
Integration Strategies
Integration strategies form the foundation for cohesive data management within an organization. These strategies focus on harmonizing disparate data sources, systems, and processes to create a unified approach towards data governance. The key characteristic of integration strategies is their emphasis on streamlining data flows, ensuring data consistency, and enabling data accessibility across the organization. While integration strategies offer benefits like improved data accuracy and efficiency, they also pose challenges such as system complexity and the need for robust data integration frameworks.
Data Governance in the Era of Big Data
The era of big data presents unique challenges and opportunities for data governance practices. Scalability challenges arise due to the vast volume, velocity, and variety of data generated in today's digital landscape. Overcoming these challenges requires scalable data governance frameworks that can adapt to the dynamic nature of big data environments. Additionally, data accessibility emerges as a critical aspect, ensuring that relevant stakeholders can access and leverage data effectively to drive informed decision-making and strategic initiatives.
Scalability Challenges
Scalability challenges in data governance revolve around managing the exponential growth of data volumes and the complexity of data sources and formats. The uniqueness of scalability challenges lies in their dynamic nature, requiring data governance strategies to be flexible and scalable to accommodate changing business needs and data requirements. Finding the delicate balance between scalability and data governance integrity is essential for organizations operating in big data ecosystems.
Data Accessibility
Data accessibility plays a decisive role in democratizing data within organizations. The key characteristic of data accessibility is its focus on ensuring that data is available to authorized users whenever needed, without bottlenecks or restrictions. By promoting data accessibility, organizations empower stakeholders at all levels to make data-informed decisions, driving innovation and competitiveness. However, challenges such as data security and privacy concerns must be carefully addressed to maximize the advantages of enhanced data accessibility.
Addressing Data Security Risks
In the digital age, data security risks loom large over organizations, necessitating robust strategies for protection and prevention. Cybersecurity threats pose significant challenges to data governance efforts, requiring proactive measures to safeguard sensitive information and mitigate potential risks. Simultaneously, data breach prevention strategies play a critical role in fortifying data governance frameworks, ensuring that confidential data remains secure and protected from unauthorized access.
Cybersecurity Threats
Cybersecurity threats encompass a wide range of malicious activities aimed at exploiting vulnerabilities in organizational systems and networks. The key characteristic of cybersecurity threats is their pervasive nature, evolving constantly to bypass security measures and compromise data integrity. Mitigating cybersecurity threats demands a multi-layered approach involving advanced security protocols, employee training, and proactive threat detection mechanisms to fortify data governance practices.
Data Breach Prevention
Data breach prevention focuses on preemptive measures to avert unauthorized access to sensitive data and prevent potential data breaches. Its key characteristic lies in its proactive stance towards data security, emphasizing the importance of implementing encryption, access controls, and data masking techniques to protect confidential information. While data breach prevention strategies offer a shield against cyber threats, they require continuous monitoring and enhancement to adapt to evolving security landscapes and emerging threats.
Conclusion
The conclusion segment encapsulates the essence of optimizing data governance, a critical aspect uncovered within the realms of this article. Here, we distill the overarching importance of data governance, emphasizing its pivotal role in modern-day business landscapes. As we navigate through the intricacies of data governance, it becomes evident that establishing robust frameworks and protocols is imperative to harnessing the power of organizational data assets effectively. This section serves as a beacon, illuminating the path towards operational efficiency, informed decision-making, and risk mitigation strategies.
Key Takeaways
Strategic Imperative of Data Governance
The strategic imperative of data governance embodies a cornerstone principle, driving the very essence of data management within organizations. It stands as a bulwark against inefficiencies and data chaos, offering a structured approach towards data utilization. The key characteristic lies in its proactive nature; by preemptively addressing data governance protocols, organizations position themselves for strategic advantages. The unique feature of strategic imperative is its ability to align data initiatives with broader business goals, ensuring a symbiotic relationship between data processes and organizational objectives. While presenting notable advantages in enhancing operational coherence and data integrity, one must remain cognizant of potential complexities that may arise in implementation.
Continuous Improvement
The concept of continuous improvement underscores the iterative nature of data governance, advocating for perpetual enhancements and refinements. Its contribution to the broader data governance landscape lies in fostering a culture of adaptability and agility within organizations. The key characteristic of continuous improvement manifests in its ability to drive incremental advancements, ensuring that data governance practices evolve in tandem with organizational needs. This iterative approach proves to be a valuable choice for this article, as it accentuates the dynamic nature of data governance frameworks. By embracing continuous improvement, organizations pave the way for sustained growth and resilience in the face of evolving data challenges. While it presents clear advantages in fostering innovation and operational excellence, considerations must be given to resource allocation and adaptability to change within the organization.
This meticulous analysis delves into the essence of data governance, underscoring not only its significance but also the actionable insights gleaned from the strategic imperative and continuous improvement facets. By intertwining theoretical frameworks with practical applications, organizations can embark on a journey towards mastery in data governance, unlocking unparalleled opportunities for success and innovation within the digital landscape.