Navigating the Diverse World of Data Careers
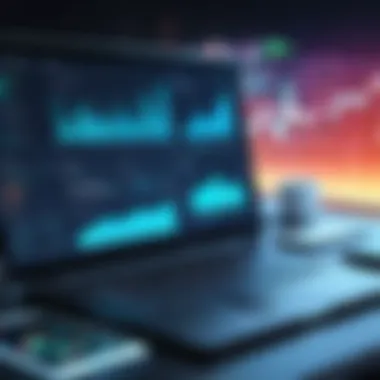
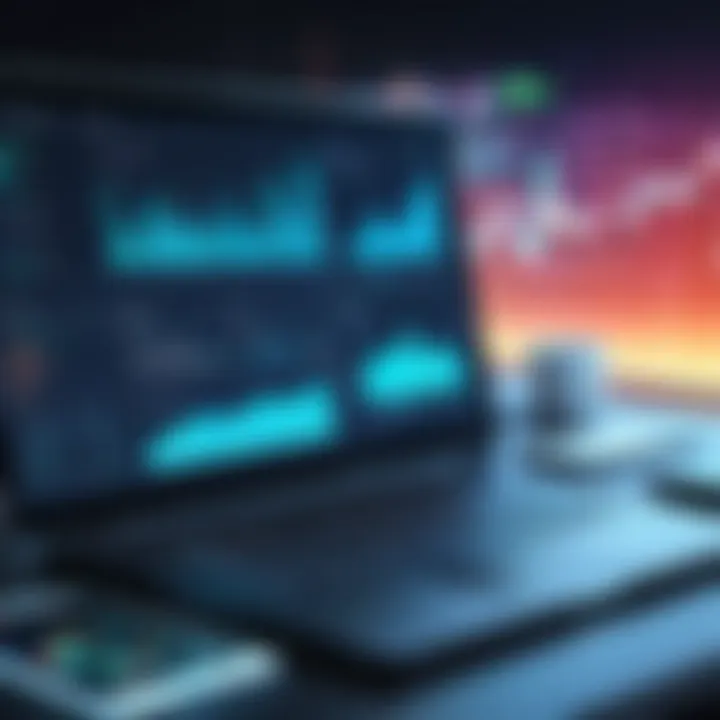
Intro
As the digital world expands like a balloon filled with air, the field of data jobs appears to explode with possibilities. Organizations are now leaning heavily on data-driven strategies, which means the demand for skilled professionals is at an all-time high. With roles ranging from data analysts to data engineers, thereās no shortage of career paths for those looking to dip their toes in this vibrant pool.
Data professionals not only handle numbers and statistics but also unravel insights that drive decision-making. With businesses sweating over competition, tapping into data has become essential. Letās break down the roles, required skills, and what it all means in this dynamic landscape of data employment.
Understanding Data Roles
Data jobs can be broadly categorized into roles such as data analysis, data science, and data engineering. Each of these roles comes with its own unique responsibilities and a specific set of skills necessary for success.
Data Analyst
Data analysts are like detectives for businesses. They collect, process, and perform statistical analyses on large datasets. Their findings help organizations understand trends and support decisions.
Key Skills:
- Proficiency in analytical tools like Excel, SQL, and Tableau.
- Strong statistical skills and the ability to interpret complex data.
- Communication skills to convey findings to non-technical stakeholders.
Data Engineer
Data engineers build the foundations that data scientists and analysts use. Their focus lies in creating robust systems that collect and store data efficiently. They ensure that data flows smoothly from source to storage and is accessible for analysis.
Key Skills:
- Knowledge of programming languages like Python or Java.
- Experience with database technologies such as MySQL, Hadoop, or NoSQL systems.
- Familiarity with system architecture to design effective data pipelines.
Data Scientist
Data scientists are often seen as wizards in the data realm, combining statistical analysis with machine learning to predict outcomes and guide strategy. They dive deep into data to derive insights that frequently lead to innovation.
Key Skills:
- Mastery of programming languages like R or Python.
- Expertise in machine learning algorithms and data mining.
- Strong statistical knowledge along with data visualization skills.
"In the age of data, understanding how to leverage information is often what sets successful businesses apart from the rest."
Best Practices and Tips for Pursuing a Career in Data
Navigating through the data job landscape requires not just understanding the roles, but also mastering the best practices for securing a place in this field.
- Networking is Key: Joining professional forums like Reddit communities or LinkedIn groups dedicated to data careers can open doors.
- Continuous Learning: Data technologies change fast, so taking online courses or attending workshops is essential.
- Build a Portfolio: Practical experience can set a candidate apart. Showcasing projects on platforms such as GitHub can highlight skills effectively.
Industry Trends and Developments
Keeping an eye on industry trends is crucial in a fast-evolving field.
Increasing Emphasis on Data Privacy
With more data being collected, companies are faced with growing concerns over privacy and security. Understanding regulations such as GDPR is now vital for any data professional.
The Rise of Data Automation
Automation tools are streamlining the data collection and analysis processes. Familiarity with automation platforms can give applicants an edge in the job market.
Epilogue
The landscape of data jobs is as intricate as a spider's web, with various roles wrapped around skillsets and competencies. For anyone serious about exploring this terrain, it's imperative to invest time in learning and adapting to the relevant skills. As organizations continue to prioritize data-driven decisions, the value of these roles will only grow, making it a promising field for aspiring professionals.
Preamble to Data Careers
In todayās fast-paced digital world, the phrase "data is the new oil" has taken root and thrived. This highlights just how crucial data has become for organizations aiming to steer their successes and make informed decisions. With the integration of technology in everyday business operations, the demand for professionals equipped with data skills has soared.
For anyone stepping into the realm of data careers, itās vital to recognize the landscape and the opportunities that lie within. The options are vast, ranging from data analysis to engineering and science, creating a rich tapestry of roles tailored to different skill sets. Understanding this spectrum is not just beneficial; itās essential for students, IT professionals, and cybersecurity experts looking to leverage their expertise in meaningful ways.
The Importance of Data in Modern Organizations
In modern organizations, the significance of data cannot be overstated. As businesses collect mountains of information daily, the capability to translate that data into actionable insights is invaluable. This is where data professionals come into play. They sift through numbers and trends, unraveling patterns that can lead to strategic improvements or innovations.
Furthermore, companies that harness data effectively can gain a competitive advantage. For instance, a retail firm analyzing consumer behavior through data can optimize its inventory and marketing strategies, ultimately enhancing customer satisfaction. In a nutshell, data is the backbone that supports decision-making, risk management, and operational efficiency.
Overview of Data Job Opportunities
The world of data jobs is as expansive as it is varied. From entry-level positions to expert roles, thereās something that caters to almost every skill set and interest.
- Data Analyst: Focused on interpreting complex datasets to help organizations make strategic choices.
- Data Scientist: They take the proverbial wheel, employing advanced statistical and analytical techniques to predict future trends.
- Data Engineer: Tasked with building and maintaining infrastructure that allows for proper data flow and access.
- Machine Learning Engineer: Merges the art of statistical analysis with cutting-edge technology, forging algorithms that learn from and make predictions based on data.
Additionally, new roles are emerging in tandem with advancements in technology, such as data privacy analysts and cloud data specialists. The possibilities are endless, with each role offering distinct paths for growth and specialization.
Understanding the diversity in data jobs helps aspiring professionals tailor their skills and career paths effectively.
Data Analyst Roles
Data analysts are often termed the unsung heroes of the data landscape. Their role is paramount because they bridge the gap between raw data and actionable insights. Whether it is through transforming messy datasets into clear narratives or presenting findings in ways that drive strategic decisions, the footprint of data analysts extends far and wide. In this rapidly evolving world where data is omnipresent, organizations need committed individuals who can sift through the noise and provide clarity. The benefits of having skilled data analysts on board include better-informed decision-making and enhanced operational efficiency. Without their expertise, data would remain just numbersāunseen and uninfluential.
Key Responsibilities of Data Analysts
The responsibilities of a data analyst may vary across different organizations but some common duties include:
- Data Collection: They gather data from various sourcesābe it databases, spreadsheets, or even unstructured data from social media.
- Data Cleaning: Analysts must ensure that data is accurate and uniform. This step is crucial since dirty data can lead to misguided conclusions.
- Statistical Analysis: They apply various statistical methods to dissect data, looking for trends or patterns that can inform corporate strategies.
- Data Visualization: Converting complex results into visual formats like graphs or charts is essential for easier interpretation by stakeholders.
- Reporting: Regularly presenting findings to management ensures that decisions are grounded in solid evidence and well-rounded insights.
Data analysts are often the first to see the potential in numbers. They take pride in their meticulous approach and problem-solving proficiency.
Essential Skills for Data Analysts
For someone looking to step into the shoes of a data analyst, certain skills are critical:
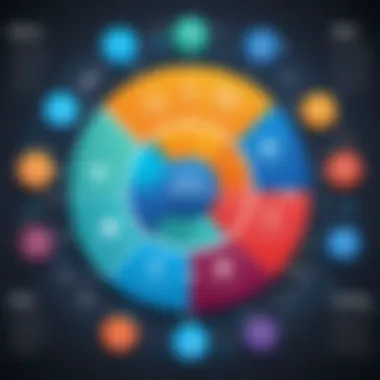
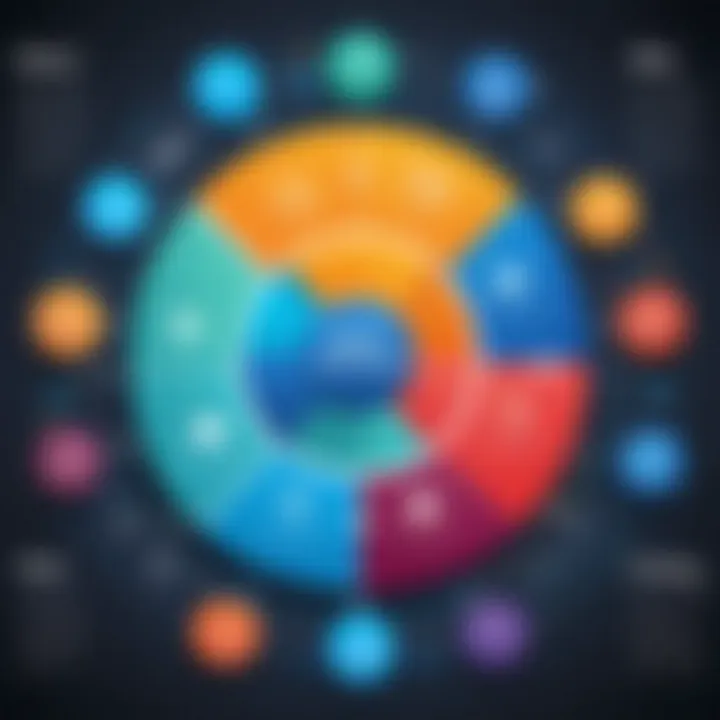
- Analytical Thinking: The ability to piece together different parts of data and create meaningful conclusions is vital.
- Technical Proficiency: Familiarity with tools such as SQL, Python, and Excel is essential for data manipulation and analysis.
- Attention to Detail: Small errors can lead to big issues in data; thus, a keen eye is indispensable.
- Communication Skills: Articulating insights clearly to non-technical stakeholders is equally important as diving into data.
- Business Acumen: Understanding the industry context helps analysts align findings with strategic goals.
"To a data analyst, every dataset tells a story waiting to be told, often leading to opportunities that can reshape business outcomes."
Career Progression and Opportunities
The journey for a data analyst is filled with opportunities. Starting off as a junior analyst, one can climb the professional ladder through continuous learning and skill enhancement:
- Junior Data Analyst: Entry-level position focusing on assisting in data collection and preparing reports.
- Senior Data Analyst: At this level, analysts have more responsibilities regarding project management and may oversee junior analysts.
- Data Analytics Manager: Leading a team of analysts and shaping the data strategy of an organization.
- Data Scientist: Many analysts transition into data science roles, where they apply advanced analytical techniques, including machine learning.
- Business Intelligence Analyst: Some may opt to specialize further into business intelligence, utilizing tools like Tableau to visualize data on a higher level.
Data analysts not only dissect data but also pave the way for a broader range of career paths, thereby ensuring that their skill set remains relevant and adaptable in this dynamic field.
Data Scientist Positions
Data science has emerged as a crucial pillar in the landscape of data jobs. Data scientists wield the power to turn vast amounts of raw data into actionable insights. This significance stems from several specific elements that make the role highly sought after in todayās job market.
One major benefit of having data scientists on a team is their ability to analyze complex data sets and derive patterns that can significantly influence business strategies. Companies find themselves relying more and more on data-driven decision-making to stay ahead in competitive markets.
However, the role of a data scientist is not just about crunching numbers. It demands a blend of analytical prowess, technical skills, and a good understanding of the business landscape. This makes the position both challenging and rewarding.
Defining the Role of a Data Scientist
Typically, a data scientist harnesses statistical methods and machine learning to interpret data. Their primary responsibility is to extract insights from data that can guide company strategies. Rather than seeing data as mere figures, they explore relationships and trends that affect business outcomes.
To put it simply, data scientists analyze user behavior, forecast trends, and provide evidence-based recommendations to stakeholders. They use programming languages such as Python or R to build models that predict future trends based on current data.
In essence, they become the bridge between data and decision-making, translating complex data findings into understandable formats for non-technical stakeholders.
Tools and Technologies Familiar in Data Science
To effectively perform their duties, data scientists utilize a plethora of tools and technologies designed to manage, visualize, and analyze data. Hereās a list of some commonly used tools:
- Python: A versatile programming language, favored for its libraries like Pandas and NumPy that simplify data manipulation.
- R: Another statistical language used extensively for data analysis and visualization.
- SQL: A standard language for managing and querying relational databases.
- Tableau and Power BI: Both are robust tools for data visualization, helping turn raw numbers into interactive dashboards.
- Hadoop and Spark: These big data frameworks are essential for processing large sets of data efficiently.
By mastering these technologies, data scientists can manipulate big data, create visualizations, and ultimately derive actionable insights from complex datasets.
Challenges Faced by Data Scientists
Despite the glamour associated with being a data scientist, the position comes with its own set of challenges. One significant hurdle is the need to constantly keep up with evolving technology and methodologies. The field is growing so fast that professionals need to continuously reskill to stay relevant.
Additionally, data cleaning and preparation often consume a major portion of a data scientistās time. Ensuring data is accurate and usable is no small feat and requires attention to detail.
To make matters a bit more complex, data scientists must often work with stakeholders who might not understand the nuances of data. Bridging the communication gap between technical data findings and business objectives can be an additional challenge.
"Data is the new oil, but just like oil, it requires refinement to be valuable."
These challenges can be daunting, yet they also provide opportunities for growth and advancement in the field. As organizations continue to recognize the value of data science, those who navigate these challenges effectively will remain valuable assets in their organizations.
Data Engineer Functions
In today's data-driven age, data engineering emerges as a linchpin in the broader landscape of data jobs. The role of a data engineer is crucial, as they design, construct, and maintain the systems that allow companies to collect, store, process, and retrieve data effectively. Without these professionals, even the most sophisticated analytics and data science efforts can fall flat, as they rely on robust data architectures and pipelines to function optimally.
Understanding Data Engineering
Data engineering is about making data accessible. Itās like building the infrastructure of a city, where data flows from its origin through pipelines into lakes and warehouses before being used for analysis. A data engineerās primary focus is on the architecture of data systems. They leverage various methodologies to ensure that data is reliable, timely, and high-quality. This work often includes:
- Designing data models based on organizational needs.
- Implementing ETL (Extract, Transform, Load) processes to shift data between platforms.
- Working on data warehousing solutions that enable easy access and retrieval of data.
This foundation allows business analysts, data analysts, and data scientists to derive insights without getting bogged down in data management issues.
Key Technologies Used in Data Engineering
A proficient data engineer must be well-versed in a variety of technologies tailored for different aspects of their role. Here are some critical technologies commonly utilized:
- Apache Hadoop: A framework that allows for the distributed processing of large data sets across clusters of computers.
- Apache Spark: This is used for big data processing and is known for its speed and ease of use when applying machine learning algorithms.
- Data Warehousing Solutions: Technologies like Snowflake and Google BigQuery help in storing large volumes of structured data efficiently.
- Database Management Systems: Knowledge of systems like SQL Server, MongoDB, or PostgreSQL is essential for managing and querying data effectively.
These technologies enable data engineers to build scalable data solutions that can handle growing volumes of data.
Collaboration with Other Teams
A successful data engineer doesnāt work in isolation. Collaboration is vital, and these professionals often find themselves working closely with several teams, such as:
- Data Scientists: They depend on data engineers to provide clean, organized datasets that can be used for analysis, modeling, and predictive analytics.
- Data Analysts: By tailoring data flows and storage solutions to meet the analystsā needs, data engineers ensure accurate and quick insights.
- IT and Operations Teams: Proper integration with IT systems is needed, as operationalizing data solutions depends on the architecture they create.
"The collaboration between data engineers, scientists, and analysts is a dance of sorts, creating a streamlined flow of actionable insights from raw data."
Machine Learning Engineer Roles
Machine learning (ML) engineers are at the forefront of a technological revolution that is reshaping industries across the globe. Their role is increasingly crucial as organizations leverage machine learning models to extract insights from vast datasets. In this section, we will explore what machine learning engineers do, the skills required for success in this domain, and how they integrate machine learning solutions into business operations. Understanding these aspects is essential for anyone interested in pursuing a career in this influential field.
What Machine Learning Engineers Do
Machine learning engineers are the bridge between data science and software engineering. They focus on creating systems that can automatically learn and improve from experience without being explicitly programmed.
Their primary responsibilities include:
- Developing ML Models: Crafting algorithms that can process data and make predictions or classifications accurately.
- Data Preparation: Cleaning and organizing data, ensuring it is ready for training machine learning models.
- Testing and Optimization: Continuously refining models to improve accuracy, efficiency, and generalization.
- Collaboration with Cross-Functional Teams: Working alongside data scientists, software developers, and product managers to deliver functional ML solutions.
To sum it up, they wear many hats. Whether it's data wrangling or fine-tuning complex algorithms, their work is a juggling act, ensuring that the entire process from data ingestion to delivery of insights is seamless and effective.
Skills Required for Machine Learning Engineering
Excelling as a machine learning engineer requires a multi-faceted skill set. The landscape of machine learning is always evolving, and thus practitioners need to keep their skills sharp. Some essential skills include:
- Proficiency in Programming: Languages like Python, R, and JavaScript are vital for building efficient models.
- Understanding of ML Algorithms: Familiarity with supervised and unsupervised learning techniques, as well as reinforcement learning, is a must.
- Mathematics and Statistics: Mastery in statistics, linear algebra, and calculus helps in understanding and modeling data correctly.
- Data Handling Skills: Experience with databases and tools for data manipulation, such as SQL and Pandas, is crucial.
- Familiarity with Cloud Services: Knowledge of tools like Google Cloud, AWS, or Azure is beneficial, as many organizations deploy ML solutions in the cloud.
While technical skills are important, soft skills such as problem-solving, analytical thinking, and effective communication can make a significant difference in project outcomes.
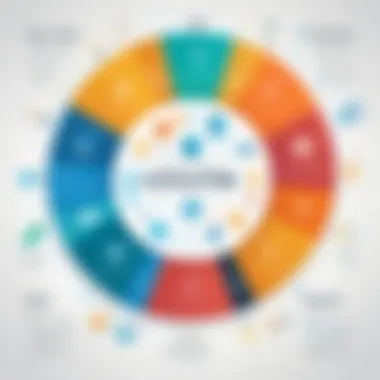
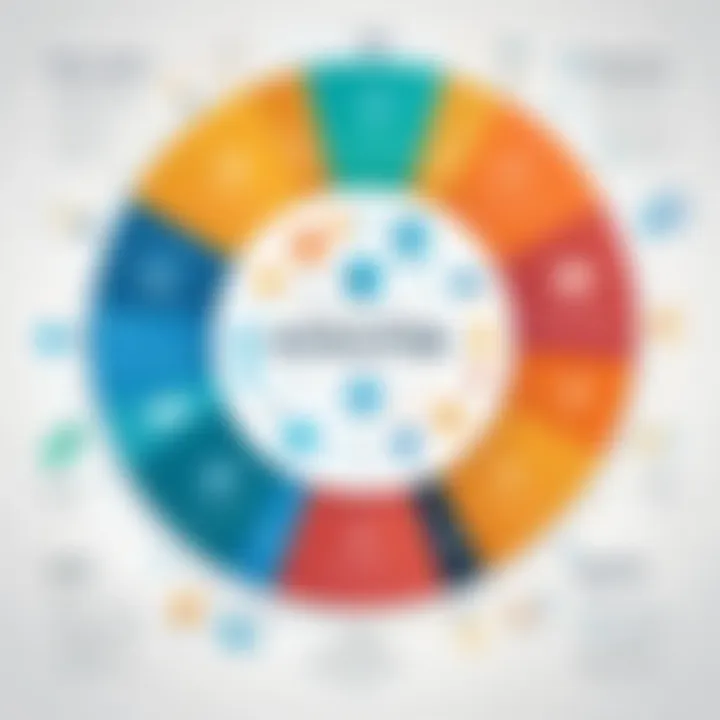
Integrating into Business Solutions
Integrating machine learning into business solutions is where the magic happens. Machine learning engineers work closely with stakeholders to align algorithms with business objectives. Hereās how they generally proceed:
- Identifying Business Needs: They begin by assessing specific challenges that machine learning could address, whether that's optimizing supply chain logistics or enhancing customer experiences.
- Building MVPs: Developing minimum viable products that provide quick insights and functionality is key. This allows businesses to test the waters before fully committing to a comprehensive solution.
- Implementation and Testing: After development, they work towards deploying the model, ensuring itās thoroughly tested in real-world scenarios to avoid any discrepancies or errors.
- Monitoring and Maintenance: Post-deployment, continuous monitoring is crucial. They need to adjust models based on new data or changing business requirements.
"The successful integration of ML into business isnāt just about technology; itās about understanding the business context behind the data."
In this way, machine learning engineers add significant value, enabling businesses to make data-backed decisions. The importance of their role cannot be understated, as they empower organizations to harness the potential of intelligent data processing.
Data Architect Responsibilities
Data architecture is increasingly becoming the backbone of efficient data management in organizations. The responsibilities of a data architect are not only pivotal in shaping the data landscape but also essential in ensuring that data systems can evolve alongside business needs. This section will delve into the various aspects of the role, emphasizing how they drive data initiatives and contribute to overall organizational success.
Role Overview of Data Architects
At its core, a data architect is responsible for designing and managing the structure of data frameworks. They are akin to architects in construction, laying the blueprint for data flow.
- Responsibilities include:
- Defining how data will be stored, consumed, and integrated across various environments.
- Adhering to best practices to ensure data integrity and accessibility.
- Collaborating with business stakeholders to understand requirements and translating them into data models that enhance efficiency.
Data architects ensure that the architecture aligns with the organization's goals, providing a clear direction and framework for data strategies. Particularly, they play a role in identifying the essential data sources that provide substantial value and deciding how best to harness these resources.
Designing Data Solutions
Designing data solutions involves creating a comprehensive framework that simplifies data management. Effective solutions often incorporate scalability, reliability, and high performance. Some vital aspects of this process are:
- Data Modeling: Creating conceptual, logical, and physical models that represent data flows effectively.
- Selecting Technologies: Evaluating and choosing appropriate technologies and tools that cater to data storage and processing needs.
- Performance Optimization: Ensuring that the data infrastructure is optimized for performance by implementing strategies for data archiving and retrieval.
A thoughtful approach to designing data solutions can save organizations substantial time and resources, enabling swift adaptation to changing conditions.
The Intersection with IT Infrastructure
Data architects must work closely with IT infrastructure teams to harmonize data architecture with existing systems. This intersection is crucial for several reasons:
- Integration: Ensuring that data architecture seamlessly integrates with other technological components, such as databases, storage systems, and cloud services.
- Compliance and Security: Collaborating to establish protocols that ensure compliance with data regulations and safeguarding against potential vulnerabilities.
- Monitoring Performance: Working together to monitor system performance, identify bottlenecks, and implement effective solutions rapidly.
In many respects, data architects act as liaisons across departments, bridging the gap between data management and IT, ensuring that the data architecture is not just functional but also strategically sound.
"A good data architect thinks several steps ahead, anticipating both immediate needs and long-term growth."
In summary, understanding the responsibilities of data architects is invaluable for grasping the broader implications of data strategy and management. Their role is crucial in navigating the complexities of data in modern organizations, ensuring that data resources are effectively leveraged to meet evolving demands.
Business Intelligence Specialists
Business Intelligence (BI) specialists play a pivotal role in any data-centric organization. Their primary aim includes transforming raw data into actionable insights that help steer companies in the right direction. With a flood of information flowing in daily, the capacity of these professionals to sift through vast amounts of data means they can uncover patterns and trends that the naked eye often misses. In essence, they act as a bridge between data analysis and executive decision-making, ensuring that businesses aren't just collecting data, but are actually using it effectively.
Defining Business Intelligence Roles
In the realm of BI, the responsibilities can vary widely depending on the organization, yet key elements remain consistent. BI specialists are tasked with gathering data from different sources, analyzing it, and then presenting their findings through visualizations like dashboards or reports. Their role isn't just about crunching numbers; it's about telling a story through data that resonates with stakeholders at various levels.
Moreover, a BI specialist often collaborates with IT departments to ensure the integrity and accessibility of data streams. They need to understand business needs and supply crucial insights, allowing companies to adapt strategies based on real-time data.
"Business Intelligence is not just about the numbers. It's about understanding the narratives behind those numbers and how they can influence decisions." - Anonymous
Tools Commonly Used in BI
The toolkit for a Business Intelligence specialist can be quite extensive, filled with numerous applications and software that streamline the gathering, processing, and presenting of data. Hereās a look at some common tools:
- Tableau: Renowned for its data visualization capabilities, helping users create interactive and shareable dashboards.
- Microsoft Power BI: A versatile option that integrates well with existing Microsoft products, making it a popular choice for many organizations.
- QlikView: A tool that uses in-memory data processing, enabling swift data analysis.
- Looker: Integrated with Google Cloud, it allows companies to visualize data in unique ways while facilitating shared insights across teams.
These tools enable BI specialists to turn complex datasets into clear and compelling narratives.
Impact of BI on Decision Making
The influence of Business Intelligence on organizational decision-making cannot be overstated. By providing structured insights, BI specialists empower leaders to make informed choices that can affect the entire scope of the business. The immediacy and accuracy of data analytics mean that businesses can quickly pivot strategies, optimize operations, and enhance customer experiences based on solid evidence rather than guesswork.
Consider how a retail chain might use BI insights to adjust stock levels in real time based on buying patterns. Similarly, a healthcare organization might analyze data on patient outcomes to streamline services and improve care.
Furthermore, the impact of BI extends beyond short-term gains. By integrating sophisticated data analyses into their strategic planning, organizations position themselves for long-term success and viability in an increasingly competitive landscape.
Data Governance and Quality Assurance
Data governance and quality assurance are two peas in a pod when it comes to ensuring the integrity and reliability of data in any organization. In a world where decision-making is increasingly data-driven, the concepts of governance and quality have taken center stage, significantly impacting how organizations handle their data assets.
Data governance refers to the overall management of data availability, usability, integrity, and security. It establishes the policies, standards, and processes required to manage data throughout its lifecycle. On the other hand, data quality assurance focuses on maintaining the precision, reliability, and relevance of that data. Together, they form a robust framework ensuring that data remains trustworthy and valuable.
Importance of Data Quality Management
The significance of data quality management cannot be overstated. Poor-quality data can lead to decisions based on faulty analyses, which may affect everything from operational efficiency to customer interactions. Some key benefits of robust data quality management include:
- Enhanced Decision-Making: High-quality data provides a solid foundation for making informed decisions. Accurate data leads to insights that are relevant and actionable, which helps businesses stay agile in a crowded marketplace.
- Improved Customer Trust: When customers see that organizations are consistently delivering accurate information, their trust deepens. This can translate into customer loyalty and repeat business.
- Operational Efficiency: Clean data prevents time wastage on fixing errors and rectifying mistakes. Management can focus instead on strategic initiatives rather than spending time putting out data-related fires.
Investing in data quality management is not just a best practice; it's a necessity for organizations seeking to lead in their industries.
Roles in Data Governance
In the realm of data governance, various roles play pivotal parts in ensuring that data is managed correctly. A few key positions include:
- Data Steward: This individual is the guardian of data integrity. They are responsible for ensuring that data policies are adhered to across the organization.
- Chief Data Officer (CDO): Positioned at the executive level, the CDO oversees data management strategies and governance practices, ensuring alignment with business objectives.
- Data Governance Analyst: This role often involves monitoring the effectiveness of governance policies and providing support in audits and compliance efforts.
Each role embodies unique responsibilities but works toward a common goal: ensuring that data is a strategic asset for the organization.
Frameworks for Data Quality Initiatives
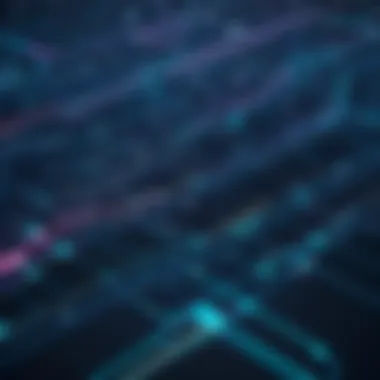
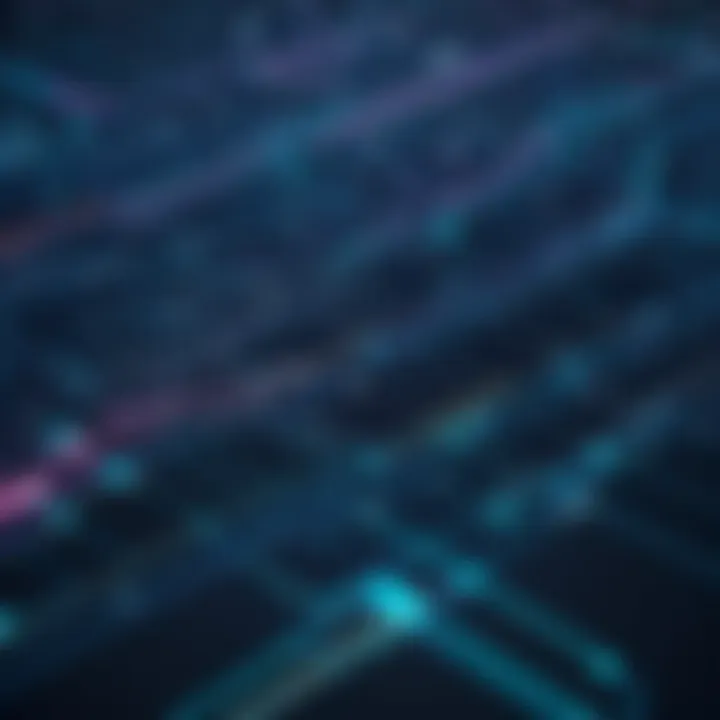
When it comes to implementing data quality initiatives, several frameworks provide a solid foundation. These frameworks guide organizations in developing a data-driven culture, especially in terms of quality assurance.
- DAMA-DMBOK: This framework is published by the Data Management Association. It outlines best practices for data governance, ensuring that thereās a structured approach to managing organizational data.
- ISO 8000: This international standard addresses data quality management and covers principles that should be applied throughout the data lifecycle.
- 3-Rule Framework: Originating from data quality industry veterans, this straightforward approach emphasizes three vital rules for data quality: data accuracy, completeness, and consistency.
Adopting any of these frameworks helps organizations avoid the rampant pitfalls of poor data quality and reinforces a culture committed to high standards.
"Data governance is not just about compliance or risk management; it's about ensuring that you can trust the data you rely on every day." - Data Governance Expert
Emerging Data Roles
As the digital landscape continues to evolve, new data roles have emerged to meet the demands of an increasingly complex world. These positions address not only the conventional aspects of data handling but also the responsibilities associated with privacy, cloud technologies, and large-scale data processing. Each of these emerging roles contributes to an organization's ability to leverage data effectively while maintaining compliance with regulations and ensuring security.
Data Privacy and Protection Analysts
In a time when data breaches are more common than ever, Data Privacy and Protection Analysts play a pivotal role. Their primary responsibility is to safeguard sensitive information from unauthorized access and ensure compliance with data protection regulations like GDPR and CCPA. This role requires a mix of legal knowledge, cybersecurity awareness, and technological proficiency.
Key responsibilities include:
- Assessing data handling processes to identify potential vulnerabilities.
- Implementing security measures to protect sensitive data.
- Conducting regular audits and privacy assessments.
- Keeping up-to-date with evolving data protection laws and regulations.
These analysts often collaborate with legal, IT, and management teams to ensure that data practices align with organizational policies and legal requirements. Their work not only reduces the risk of data breaches but also fosters consumer trust, which is invaluable in today's data-driven environment.
Cloud Data Specialists
With more businesses shifting to cloud-based solutions, Cloud Data Specialists have found themselves in high demand. These professionals manage data stored in cloud environments, optimizing its accessibility and security. They also ensure that data architecture is aligned with the organization's cloud strategy.
Responsibilities of Cloud Data Specialists typically involve:
- Designing and managing cloud data systems, often using platforms like AWS, Google Cloud, or Microsoft Azure.
- Ensuring data security and compliance within the cloud framework.
- Optimizing data storage and retrieval processes to improve performance and reduce costs.
- Monitoring data consistency and integrity, crucial for successful cloud operations.
Their expertise not only enhances operational efficiency but also allows businesses to harness cloud technology to innovate and scale more effectively.
Big Data Technologies and Roles
As businesses encounter ever-increasing volumes of data, the demand for experts skilled in Big Data technologies grows. These roles are essential for organizations seeking to extract actionable insights from vast datasets. At the core of this field lies the ability to process, analyze, and visualize data on a grand scale.
Some of the prominent roles in Big Data include:
- Big Data Engineer: Focuses on building scalable architecture and data pipelines.
- Data Scientist: Engages in complex data analysis, employing algorithms to uncover patterns and predictions.
- Data Visualization Specialist: Translates data findings into visual formats to facilitate decision-making.
Technologies like Hadoop, Apache Spark, and NoSQL databases are commonly utilized in this space. The integration of Big Data into business strategies can lead to significant improvements in performance, customer engagement, and overall strategic direction.
The landscape of data jobs is continuously shifting, adapting to technological advancements and market needs. Professionals in emerging data roles must stay vigilant and flexible to thrive in this dynamic environment.
Navigating Data Career Paths
In todayās tech-driven world, navigating a career path within the data realm is not just important; itās paramount. The rapid expansion of data roles presents an array of choices, each offering distinct challenges and rewards. When stepping into data employment, knowing the way around these career options could very well be the difference between settling for a mundane position and snagging that dream job that truly fulfills your potential.
A well-charted career path in data can lead to impressive advancements, making it crucial for aspirants to align their skills, education, and networking efforts with the right opportunities. The ability to pivot among various rolesābe it data analyst, data scientist, or data engineerāsignificantly broadens oneās scope, opening doors that might seem otherwise locked.
Essential Skills for Aspiring Professionals
Not all data roles are created equal, yet there are core skills that nearly every data job values. These skills arenāt necessarily about being a whiz with numbers alone; they encompass a multifaceted skillset that balances technical know-how with strategic thinking:
- Analytical Thinking: The ability to interpret data and extract actionable insights. One might be comparing apples to oranges, but in data jobs, itās more about understanding the real significance of those apples.
- Technical Proficiency: Familiarity with tools like Python, R, SQL, and data visualization platforms like Tableau. Think of these as your Swiss Army knives for tackling data problems.
- Communication Skills: Data professionals must often translate complex findings into digestible insights for stakeholders. A good way to frame it is: you can have all the data in the world, but if you can't convey the message, whatās the point?
- Problem Solving: Approaching issues with an analytical lens to spark creative solutions. The data landscape is often a puzzle, implying that the ability to piece together disparate data points is invaluable.
Educational Paths and Certifications
In the data field, educational backgrounds vary widely. You donāt need to have a degree in data science specifically; relevant degrees in statistics, computer science, or even business can serve just as well. What matters more is how you leverage your education into a career. Here are some educational paths and certifications to consider:
- Degrees: Beyond a bachelorās, pursuing a masterās or an MBA with a focus on data analytics can give you an edge.
- Online Courses: Platforms like Coursera and edX offer specialized courses, many backed by recognized institutions, that can supplement your knowledge.
- Certifications: Earning certifications such as Microsoft Certified: Azure Data Scientist Associate or Google Data Analytics Professional Certificate can bolster your resume, proving your commitment to the profession.
In this tech-savvy world, self-learning is key; pursue projects and build portfolios that showcase your capabilities.
Networking and Industry Involvement
In the tapestry of data careers, networking plays a subtle yet significant role. The connections you make can lead to new opportunities or collaborations that would never surface without a warm introduction. Engaging in professional communities, whether online or offline, can provide insights into industry trends and job openings:
- Attend Meetups and Conferences: Get out there and meet folks in the industry. Whether it's a small local gathering or a larger conference, these events are fertile ground for building professional relationships.
- Join Online Communities: Leverage platforms like LinkedIn, Reddit, or specialized groups on Facebook where data professionals exchange ideas and job leads. Being part of these communities can lead to valuable advice from individuals already navigating the waters you wish to explore.
- Engagement: Don't just be a silent member; participate actively. Share your knowledge, ask questions, and connect with others who share your passion for data.
"Your network is your net worth."
Culmination and Future of Data Jobs
As we wrap up our exploration of the diverse and ever-evolving field of data jobs, itās clear that these careers play an increasingly vital role in shaping the modern workplace. From businesses needing insights to drive strategic decisions to governments utilizing data for better policy-making, the implications of expertise in data are profound. Understanding the conclusion of this landscape helps clarify the trajectory of data roles and highlights the importance of skilled professionals to meet the growing demand.
One crucial element to consider is that data-related positions are not solely for those with advanced technical degrees. They often require a combination of soft skills, critical thinking, and domain knowledge. As such, aspiring professionals should look beyond just technical prowess; knowledge of the industry, strong communication abilities, and an understanding of ethical data usage can significantly enhance one's career prospects.
The benefits of entering the data job market right now are manifold. Job security is arguably among the most significant, given the ongoing digitization trends in various industries. Positions in data science, engineering, and analysis are projected to remain in high demand. Furthermore, professionals in the data domain often enjoy competitive salaries and the opportunity for continual learning and growth, as they adapt to new tools and methodologies.
However, with the advantages come certain considerations. The rapid pace of change in technology means that continuous learning is not just beneficial but essential. Professionals need to invest in their education and remain up-to-date with emerging trends, such as the advancement of artificial intelligence and machine learning. Networking and active participation in communities can also help anyone in data roles stay relevant and informed.
Ultimately, the future of data jobs is bright but competitive. Itās about being proactive and ready to seize opportunities as they arise while being aware of potential challenges.
"Data is the new oil" - a phrase that has gained traction in recent years; it emphasizes the necessity of skilled professionals who can transform raw data into actionable insights.
Predicting Trends in Data Employment
Looking ahead, several trends are likely to shape the landscape of data employment. Firstly, the growth of automation and AI will continue to influence the nature of data jobs. The routine and repetitive aspects of data processing may be taken over by intelligent systems, making room for professionals who can harness these technologies and leverage them strategically.
Secondly, the popularity of remote work has pushed organizations to adopt more flexible structures for data teams. This trend is leading to a broader talent pool, allowing companies to hire skilled individuals from diverse locations.
Here are a few specific trends to keep an eye on:
- Increased focus on data ethics and compliance: As data privacy regulations tighten, roles that specialize in ethical practices will emerge.
- Cross-disciplinary roles: Professionals who can bridge the gap between data analytics and specific industries, such as finance or healthcare, will be more valuable.
- Continued growth of visualization tools: As businesses demand better ways to present data, specialists in data visualization will find increasing relevance in their skills.
Final Thoughts on the Data Job Landscape
In summary, the landscape of data jobs is multifaceted and constantly in flux. The increasing reliance on data for decision-making has not only opened numerous career paths but has also underscored the need for continual learning and adaptability.
As organizations increasingly prioritize data-driven strategies, the roles available will likely become more specialized, necessitating professionals to develop niche skills and expertise.
Guiding the upcoming professionals through these changes is essential, ensuring they possess both technical and soft skills. Finally, the commitment to ethical standards in data handling will become a defining factor not just for individual careers, but for the industry as a whole. Embracing this trajectory, with all its complexities and distinctions, can pave the way for a more insightful and responsible future in data-related careers.