Unleashing the Potential of Deep Learning in Vision Systems for Enhanced Visual Perception
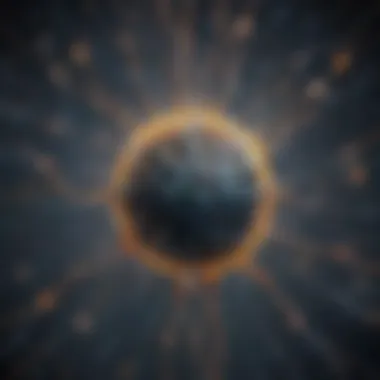
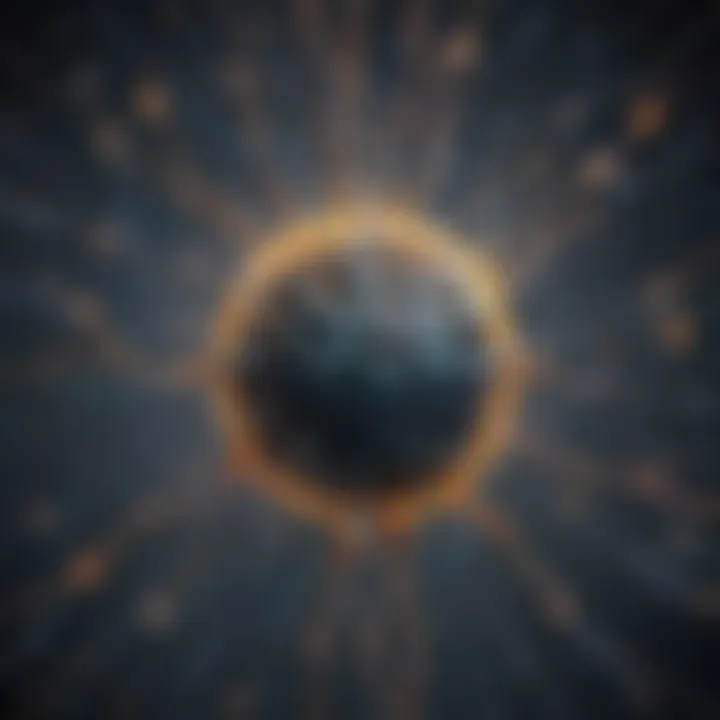
Understanding the Foundation of Deep Learning for Vision Systems
In delving into the discussion of deep learning within vision systems, one cannot dismiss the monumental impact this sophisticated technology has had on transforming how machines decipher and comprehend visual data. The realm of deep learning encompasses intricate algorithms and neural networks that enable machines to not only recognize images but also interpret them in a manner comparable to human understanding, elevating the capabilities of machines in various domains. It is crucial to grasp the fundamental principles of deep learning within vision systems to appreciate the significance it holds in advancing artificial intelligence.
Unveiling the Inner Workings of Deep Learning for Vision Systems
To comprehend the essence of deep learning in vision systems, it is imperative to break down the underlying mechanisms driving this cutting-edge technology. At its core, deep learning involves the utilization of neural networks with numerous layers to process complex data inputs and generate meaningful outputs. These deep neural networks are structured to mimic the functioning of the human brain, enabling machines to learn from vast amounts of labeled data and make intelligent decisions based on patterns they identify.
Navigating the Landscape of Image Recognition and Computer Vision
An essential facet of harnessing deep learning for vision systems lies in its profound impact on image recognition and computer vision capabilities. Through deep learning algorithms such as convolutional neural networks (CNNs) and recurrent neural networks (RNNs), machines can achieve unprecedented levels of accuracy in identifying objects, recognizing patterns, and even understanding the context of visual information. This enhanced image recognition prowess is revolutionizing industries ranging from healthcare and autonomous vehicles to security and retail, opening up new horizons for innovation and efficiency.
Cutting-Edge Applications and Future Prospects
Introduction
In delving into the intricate landscape of deep learning for vision systems, it becomes apparent that the fusion of these two domains holds immense potential for shaping the future of artificial intelligence. Vision systems, fueled by deep learning algorithms, possess the capability to revolutionize the interpretation and understanding of visual data by machines. This article serves as a guiding beacon through the complexities of this transformative amalgamation, shedding light on how deep learning is poised to redefine the boundaries of technological advancement in image processing and computer vision.
Understanding Deep Learning
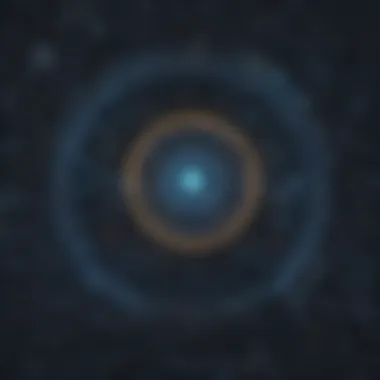
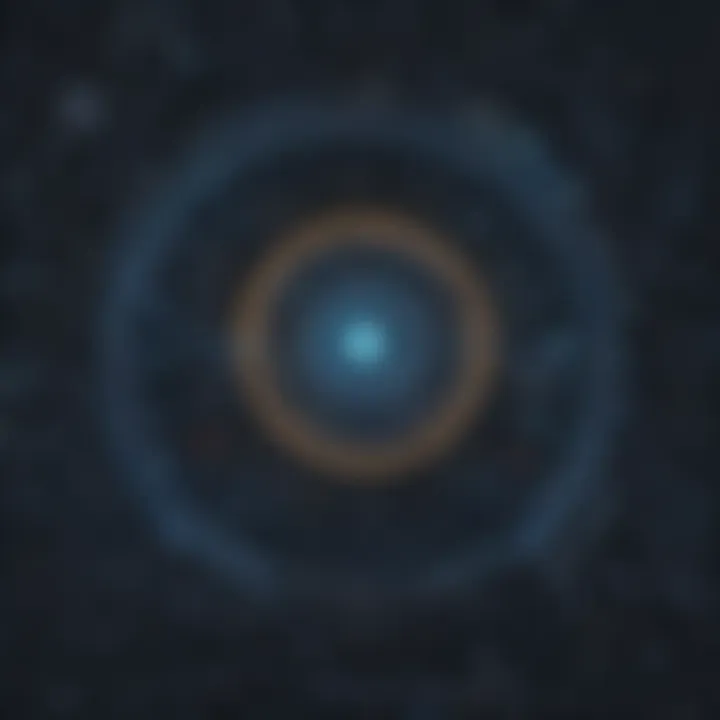
The Basics of Neural Networks
Embarking on an exploration of the fundamentals of deep learning necessitates an examination of the bedrock upon which this technology stands β neural networks. The foundational framework of neural networks epitomizes the essence of deep learning, enabling systems to mimic the intricate workings of the human brain with layers of interconnected nodes. The pivotal characteristic of neural networks lies in their ability to learn from data, adapt to patterns, and make intelligent decisions autonomously. This adaptability renders neural networks a favored choice within the realm of deep learning for their unparalleled capacity to engage in complex data analysis and pattern recognition. However, it is essential to acknowledge that the intricate architecture of neural networks can exhibit both advantages, such as robust learning capabilities, and disadvantages, including computational complexity, in the context of optimization within deep learning models.
The Role of Artificial Intelligence in Deep Learning
Diving deeper into the realm of deep learning unveils the indispensable role played by artificial intelligence in steering the course of technological evolution. Artificial intelligence serves as the driving force behind the development and advancement of deep learning algorithms, providing the cognitive prowess needed to imbue machines with the ability to perceive, learn, and adapt within visual contexts. The crux of artificial intelligence in deep learning lies in its capability to process vast amounts of data, recognize intricate patterns, and derive meaningful insights autonomously. This pivotal role underscores why artificial intelligence is an indispensable component within the deep learning spectrum, empowering vision systems to unravel the complexities of visual data with unprecedented accuracy. However, it is crucial to acknowledge that the integration of artificial intelligence in deep learning frameworks may pose challenges such as ethical considerations and interpretability issues, necessitating a balance between innovation and ethical principles.
Evolution of Vision Systems
From Traditional Image Processing to Deep Learning
Navigating through the evolutionary trajectory of vision systems unveils a significant paradigm shift from traditional image processing approaches towards the transformative landscape of deep learning methodologies. The transition from conventional techniques to deep learning signifies a monumental leap in the realm of visual data analysis, empowering systems to go beyond mere pixel-level manipulations to comprehend the semantic richness embedded within visual cues. This transition underscores the superiority of deep learning in discerning intricate patterns, extracting meaningful features, and enhancing the accuracy of image recognition tasks within vision systems. However, it is pivotal to acknowledge that while the advent of deep learning revolutionizes image processing paradigms, challenges such as model interpretability and computational complexities persist as areas warranting further exploration and refinement.
Impact of Deep Learning on Computer Vision
Delving into the impact unleashed by deep learning on computer vision illuminates the transformative potential inherent in fusing these domains. The integration of deep learning principles into computer vision frameworks has catalyzed a paradigm shift in the efficiency, accuracy, and scalability of visual recognition tasks. Deep learning empowers computer vision systems to navigate through vast troves of visual data, extract semantic information, and make informed decisions with unprecedented precision. The profound impact of deep learning on computer vision lies in its ability to transcend traditional boundaries, enabling real-time object detection, spatial localization, and scene understanding with unparalleled accuracy. Nevertheless, it is imperative to acknowledge that while the impact of deep learning on computer vision is profound, challenges such as model generalization and data bias necessitate continuous scrutiny and enhancement to harness the full potential of this symbiotic relationship.
Fundamentals of Deep Learning for Vision
In the expansive realm of deep learning for vision systems, understanding the fundamentals is paramount to grasping the intricacies of this advanced technology. The core concepts of deep learning set the foundation for revolutionizing how machines perceive and analyze visual data. By delving into Convolutional Neural Networks (CNNs) and Recurrent Neural Networks (RNNs) β two pillars of deep learning in vision systems β a deeper comprehension of image recognition, object tracking, and motion analysis is attained. The significance of comprehending these fundamentals lies in unlocking the true potential of deep learning algorithms to enhance the accuracy and efficiency of vision systems.
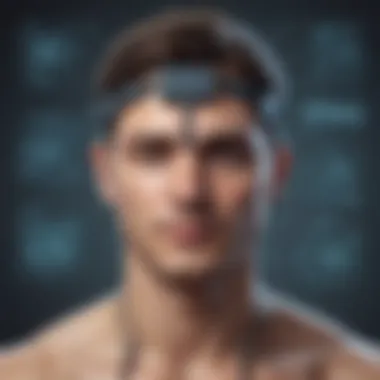
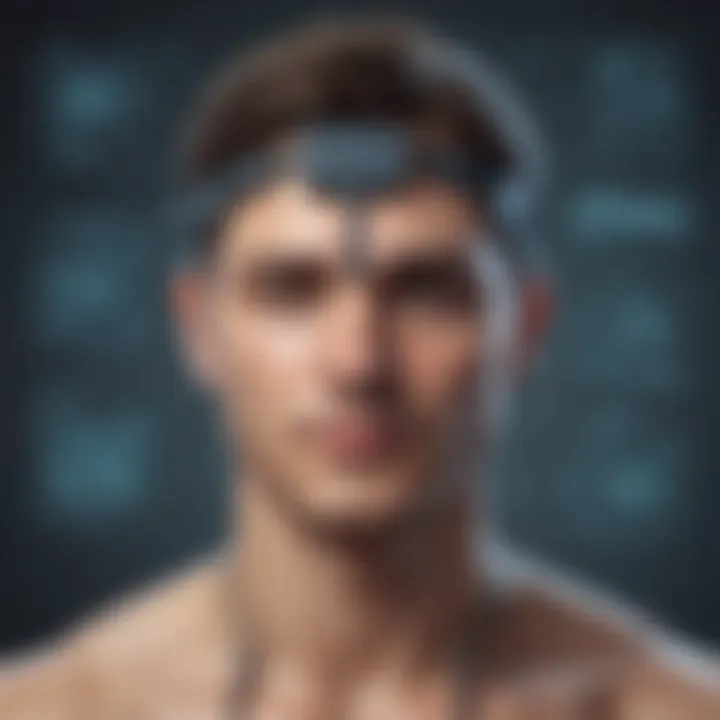
Convolutional Neural Networks (CNNs)
Architecture and Working Principles
The architecture and working principles of Convolutional Neural Networks (CNNs) stand as a cornerstone in the application of deep learning for image processing. CNNs are specifically designed to mimic the visual cortex of the human brain, enabling them to extract intricate features from images through convolutional layers and pooling. This structural design allows CNNs to discern patterns and shapes within visual data, making them highly adept at tasks such as image classification and recognition. The convolutional nature of CNNs facilitates hierarchical learning, where lower layers recognize simple features like edges, while higher layers integrate these features to detect complex structures within images. This hierarchical feature extraction is key to the success of CNNs in tasks requiring sophisticated visual analysis.
Applications in Image Recognition
The applications of Convolutional Neural Networks (CNNs) in image recognition are vast and transformative. In the realm of computer vision, CNNs have revolutionized the accuracy and speed of image classification tasks. Due to their ability to automatically learn hierarchical representations of data, CNNs excel in tasks like object detection, facial recognition, and scene understanding. Their adaptability to various visual data types and robustness against variations in scale, orientation, and lighting conditions make CNNs a popular choice in developing cutting-edge vision systems. However, despite their effectiveness, CNNs may suffer from computational complexity and the need for substantial training data, highlighting areas for further research and optimization.
Recurrent Neural Networks (RNNs)
Understanding Sequential Data in Vision
Recurrent Neural Networks (RNNs) play a pivotal role in processing sequential data within vision systems, offering a unique perspective on temporal relationships and context within visual information. Unlike traditional feedforward neural networks, RNNs possess feedback loops that allow them to retain memory of past inputs. This memory retention enables RNNs to analyze sequences of data, making them indispensable for tasks involving video analysis, speech recognition, and natural language processing. By understanding sequential data in vision, RNNs enhance the temporal understanding of visual content, leading to more accurate predictions and insights in dynamic visual contexts.
Role in Object Tracking and Motion Analysis
In the domain of object tracking and motion analysis, Recurrent Neural Networks (RNNs) showcase their prowess in predicting object trajectories, recognizing motion patterns, and forecasting dynamic changes within visual sequences. The ability of RNNs to capture temporal dependencies in visual data empowers systems to track objects across frames, anticipate motion behaviors, and extrapolate future positions with precision. By leveraging the recurrent connections inherent in RNNs, vision systems can achieve real-time object tracking and motion analysis, essential for applications like surveillance, autonomous vehicles, and augmented reality. However, the challenge of vanishing gradients in training RNNs and the need for attention mechanisms to handle long-term dependencies pose ongoing research opportunities for refining RNN architectures in vision tasks.
Applications of Deep Learning in Vision Systems
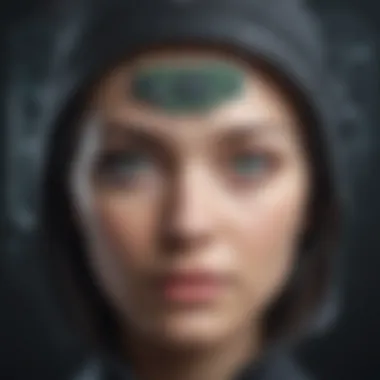
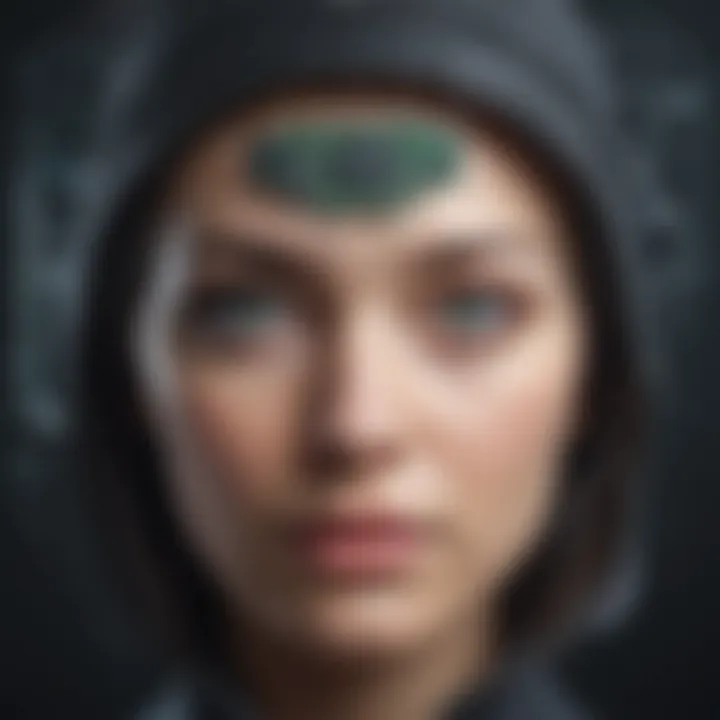
Deep learning has emerged as a groundbreaking technology in the realm of vision systems, reshaping how machines interpret and analyze visual data. The applications of deep learning in vision systems play a pivotal role in advancing image recognition and computer vision tasks. By leveraging deep learning algorithms, machines can process, understand, and draw insights from complex visual information with remarkable accuracy and efficiency.
Image Classification
Enhancing accuracy with deep learning
Enhancing accuracy with deep learning is a critical aspect of image classification that significantly contributes to the overall goal of precise object identification. The key characteristic of enhancing accuracy with deep learning lies in its ability to continuously learn and adapt to diverse visual patterns, thereby improving the identification accuracy of objects in images. This approach is particularly advantageous in this article as it leads to more reliable classification results and facilitates better decision-making processes. The unique feature of enhancing accuracy with deep learning is its capability to handle large datasets and varied image complexities, resulting in enhanced precision and reliability in image classification tasks. While this method offers substantial advantages in enhancing accuracy, it may pose challenges in terms of computational resources and training data.
Challenges and advancements
Delving into the challenges and advancements within image classification unveils essential insights crucial to the evolution of vision systems. Addressing challenges related to dataset diversity, environmental variations, and class imbalances is key to advancing the accuracy and robustness of image classification models. The advancements in techniques such as transfer learning, data augmentation, and model optimization contribute significantly to overcoming these hurdles and enhancing the performance of image classification systems. The distinctive characteristic of these advancements lies in their ability to adapt pre-trained models to new tasks effectively, thereby improving efficiency and reducing the need for extensive labeled data sets. While these advancements propel image classification systems towards greater efficacy, they may face limitations in scenarios with minimal training data or insufficient computational resources.
Object Detection and Localization
Improving precision in identifying objects
The quest for precision in identifying objects is a fundamental aspect of object detection and localization, influencing the overall efficiency of vision systems. Improving precision with deep learning involves enhancing the ability to accurately detect and locate objects within images with exceptional granularity. The key characteristic of this process is its capacity to discern intricate details and subtle differences, leading to precise object identification and localization. This strategic focus on precision is pivotal in this article as it elevates the reliability and accuracy of object detection tasks, ultimately improving the overall performance of vision systems. The unique feature of improving precision lies in its potential to minimize false positives and negatives, thereby refining the object detection process. However, while enhancing precision is advantageous, it may pose challenges in terms of computational complexity and model optimization.
Real-time applications
Real-time applications represent a significant facet of object detection and localization, enabling swift and accurate identification of objects within dynamic environments. The key characteristic of real-time applications is their ability to provide instantaneous feedback and analysis, crucial for applications requiring rapid decision-making based on real-time visual data. This feature is particularly beneficial in this article as it showcases the practical utility of deep learning in time-sensitive scenarios, such as autonomous driving and surveillance systems. The unique feature of real-time applications is their seamless integration with hardware acceleration technologies, optimizing processing speed and enabling prompt object detection and localization. While real-time applications offer expedited object identification, they may encounter challenges related to latency and computational load in resource-constrained environments.
Challenges and Future Trends
This section delves deep into the critical aspects of challenges and future trends within the realm of deep learning for vision systems. Addressing these elements is paramount as they pave the way for advancements and improvements in the field. One key element highlighted here is the importance of overcoming data limitations. With the exponential growth of visual data, techniques such as data augmentation play a pivotal role. These techniques involve manipulating existing data to create variations, thereby enhancing the model's robustness and generalization. Data augmentation is crucial for mitigating issues like overfitting and ensuring the model can perform effectively on diverse datasets.
Another essential aspect discussed is transfer learning for diverse datasets. Transfer learning involves leveraging pre-trained models on vast datasets to extract features that can be applied to new, often smaller datasets. This process helps in accelerating model training and achieving higher performance with limited data. By transferring knowledge from one domain to another, it facilitates enhanced learning capabilities and efficiencies, making it a popular choice for addressing data limitations in vision systems.