The Role of SPSS in Business Analytics
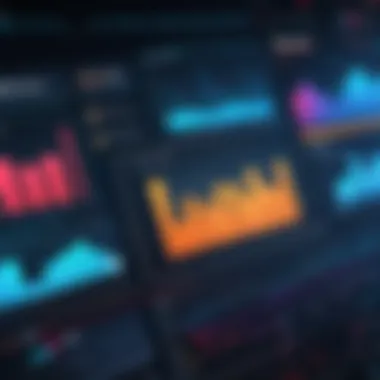
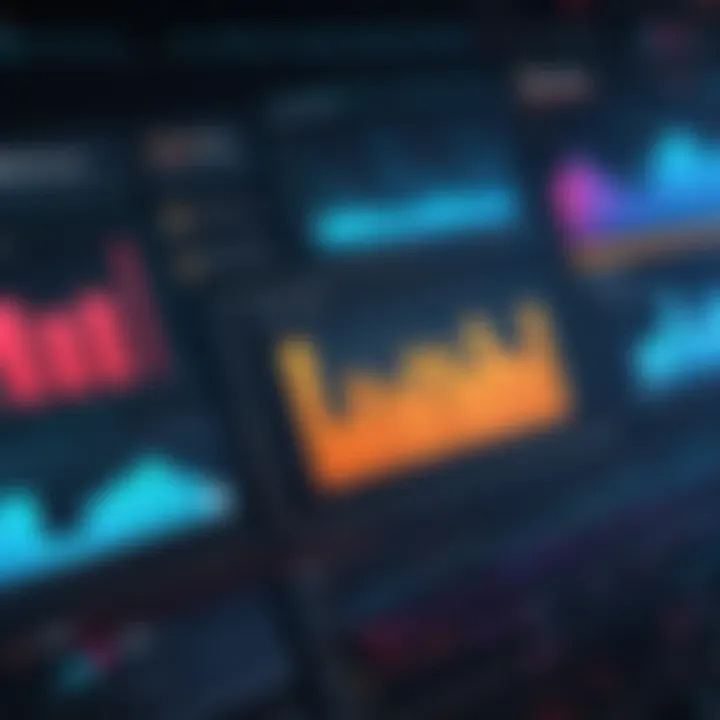
Intro
In today's fast-paced business environment, making informed decisions hinges on the ability to analyze data efficiently. The use of software tools, like SPSS, plays a significant role in helping organizations make sense of the complexities surrounding their data. By breaking down vast quantities of information, SPSS facilitates well-informed decisions that can drive a company's success, ultimately laying the groundwork for a competitive edge.
SPSS stands for Statistical Package for the Social Sciences. Initially designed for social science applications, it has since evolved into a powerful tool for various industries, aligning with trends in data analytics and business intelligence. At its core, SPSS allows users—both seasoned analysts and those just starting out—to perform robust statistical analysis without needing an extensive background in statistics. From descriptive statistics to advanced predictive modeling, SPSS has a broad array of features that cater to different analytical needs.
Understanding SPSS is crucial for IT professionals, business analysts, and students alike, as it serves not just as a data analysis tool but also as a foundation for making data-driven decisions. In this article, we will explore the multifaceted role of SPSS in business analytics, highlighting its features, relevant applications, and how organizations integrate it with other technologies. With a keen grasp of these elements, you'll find SPSS less intimidating and much more approachable for your analytical needs.
What to Expect
The following sections of this article will delve into distinct aspects of SPSS as it relates to business analytics:
- The basic functionalities of SPSS and its applications in various business scenarios.
- Best practices for leveraging SPSS to its fullest potential in analytics.
- Recent trends driving the evolution of SPSS and analytics tools.
- Real-world examples showcasing how organizations have successfully used SPSS.
- Conclusion summarizing key takeaways from the discussion.
Each section will unpack the breadth and depth of SPSS, ensuring clarity and insights for our discerning readership.
Prologue to SPSS
SPSS, originally crafted as a statistical software tool, has become an indispensable asset in the business analytics arsenal. Its capability to dissect vast amounts of data and present actionable insights is what makes it a crucial component for modern organizations. Understanding SPSS sets the stage for mastering data analysis techniques that can lead to informed decision-making processes. The relevance of SPSS in business analytics can't be overstated; it offers a structured way to analyze diverse datasets, revealing trends and patterns that might otherwise go unnoticed.
History of SPSS
The journey of SPSS began in 1968, thanks to the vision of Norman H. Nie, C. Hadlai Hull, and William G. Graham. Initially, it was designed to help researchers manage and analyze survey data efficiently. Over the decades, SPSS evolved significantly, adapting to the technological landscape and user needs. By the 1980s, it expanded its reach beyond academia, finding its niche in business, healthcare, and government sectors. Significant milestones have included the integration of advanced statistical techniques and the shift towards a more user-friendly interface, making it accessible to those who may not have a deep statistical background.
SPSS's rich history reflects a constant evolution, aiming to serve a wide range of users, from statisticians to business analysts. Its foundational emphasis on data integrity and user-centric design has made it a cornerstone in the field of data analysis.
Core Functionality of SPSS
SPSS offers a breadth of functionalities that empower users to generate comprehensive analyses of their data. At its core, the software includes:
- Descriptive Statistics: Basic statistics like mean, median, and mode that provide an overview of the data.
- Inferential Statistics: Techniques such as t-tests, ANOVA, and regression analysis help draw conclusions about a population based on sample data.
- Data Management: Users can manipulate, merge, and transform datasets easily, ensuring accuracy and efficiency.
- Customizable Output: Results can be displayed in various formats, accommodating different presentation needs.
These capabilities not only streamline the analytical process but also enable organizations to derive meaningful insights that can directly influence strategy and operations. In essence, SPSS transforms complex data into understandable information, which is essential for effective decision-making in today’s competitive landscape.
"The use of statistical tools like SPSS empowers businesses to make decisions based on facts, not just intuition."
The Importance of Data Analysis
In today's fast-paced business environment, the importance of data analysis cannot be overstated. It serves as the backbone for informed decision-making and strategic planning within organizations. Riveting insights gleaned from thorough data evaluation turn raw numbers into actionable intelligence that empower businesses to thrive.
Defining Business Analytics
Business analytics revolves around the systematic exploration of an organization’s data. At its core, it's about converting data into knowledge. This can encompass a wide range of practices—ranging from simple reporting to complex statistical analysis and predictive modeling. Essentially, it includes the methods and tools used to collect, analyze, and interpret vast amounts of data for better business outcomes. In essence, it's about telling a story that the data wants to share. Think of it as piecing together various fragments of information: each piece crucial to understanding the overall picture.
- Descriptive Analytics: This gives organizations insight into the past by summarizing historical data, helping them recognize patterns and trends.
- Predictive Analytics: Looking ahead, this form predicts future possibilities based on historical patterns, helping businesses anticipate shifts in market behavior.
- Prescriptive Analytics: On the front lines of decision-making, this method advises on possible outcomes and the strategies to take.
By embracing these analytics methods, companies can make fact-based decisions, rather than relying on gut feelings, thus minimizing risks associated with ambiguity.
Role of Data in Business Decisions
The role of data in business decisions is multi-faceted and highly significant. Data acts as a compass guiding organizations toward strategic initiatives and optimized processes. It allows executives to dig deeper into customer preferences, market trends, and internal efficiencies to make informed choices.
Consider this: no business has ever thrived on intuition alone. Understanding consumer sentiment, performance metrics, and existing market conditions requires hard data analysis.
"Data is the new oil." - this phrase underscores the idea that data, much like oil, is a valuable resource that requires refinement to be truly useful.
Some benefits of leveraging data analysis include:
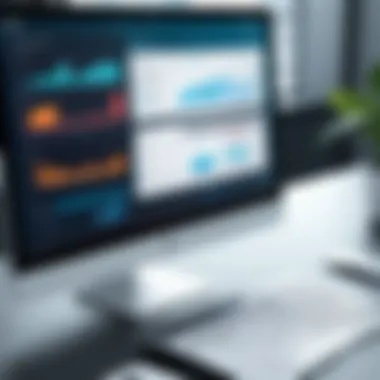
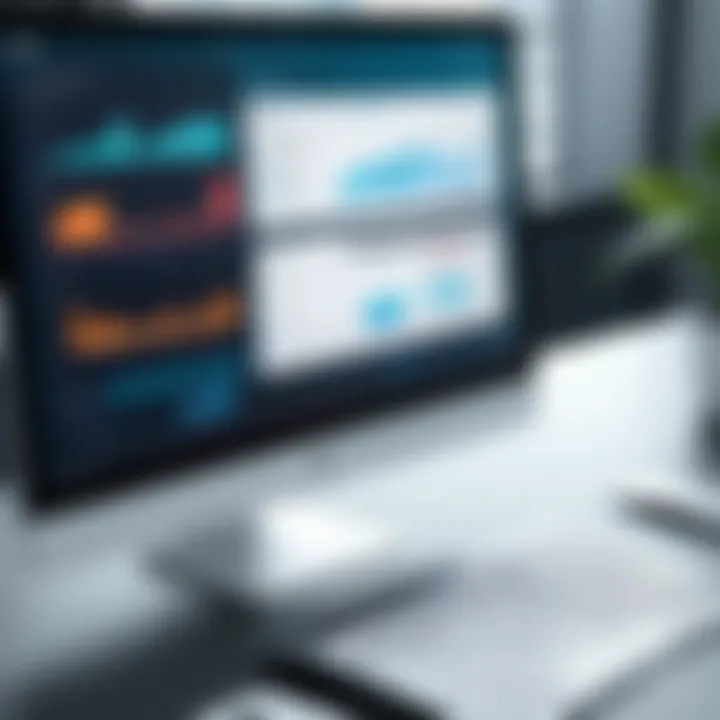
- Enhanced Customer Insights: Data enables organizations to understand what customers truly want, tailoring products and services accordingly.
- Better Risk Management: By analyzing patterns and trends, organizations can identify potential risks and develop strategies to mitigate them.
- Operational Efficiency: Through data-driven insights, companies can streamline operations, reduce costs, and improve overall productivity.
How SPSS Enhances Business Analytics
In the realm of business analytics, SPSS plays a pivotal role. It equips organizations with the tools necessary to decode vast amounts of data effectively. By producing comprehensive analyses, it enables better decision-making and strategic planning. SPSS's capabilities extend across various areas, making it a key player in the analytics toolkit. Understanding how SPSS enhances business analytics is not just a matter of recognizing its functions, but also appreciating how these functions translate into real-world benefits.
Statistical Analysis Capabilities
One of SPSS's hallmark features is its statistical analysis capabilities. It allows users to perform a myriad of statistical tests, from basic descriptive statistics to more complex inferential statistics. For instance, businesses can identify trends in their sales data over time, assess customer satisfaction through surveys, or even conduct longitudinal studies to forecast future market conditions.
Using SPSS, analysts can run regression analyses to predict outcomes based on historical data. This means that organizations can, for example, anticipate customer purchase patterns, which can lead to more targeted marketing strategies. The software also provides options for hypothesis testing, allowing companies to confirm or refute assumptions based on real data.
Another significant advantage is SPSS's user-friendly interface, which enables users with varying levels of statistical knowledge to glean insights from their data without needing to become statistical experts themselves.
Data Management Features
Beyond analysis, SPSS offers robust data management features. Effective data handling is crucial, as poor data quality can lead to misleading results. SPSS assists users in cleaning and organizing their data sets, ensuring that the analyses are based on high-quality inputs.
Data can be imported from various sources, such as Excel spreadsheets or SQL databases, making SPSS highly adaptable. Users can create new variables, merge data sets, and manipulate existing data to suit their specific needs, thereby ensuring they are working with the most relevant information.
Moreover, SPSS provides functionalities for data transformation. Users can easily recode data, conduct filtering, and aggregate data in convenient ways. This feature is essential for businesses that need to streamline their datasets for clearer analysis without losing key insights.
Visualization Tools
Visual representation of data is often what turns complex numbers into understandable narratives. SPSS shines in this area as well, offering powerful visualization tools. Analysts can create an assortment of charts—bar graphs, histograms, and scatterplots, to name just a few.
These visualization tools help communicate findings effectively. For instance, suppose a company is trying to illustrate customer demographics to guide product development. Using SPSS, they could create a detailed pie chart displaying various age groups of their customers. These visuals make it easier for stakeholders to grasp complex data quickly and encourage data-driven discussions in strategic meetings.
Furthermore, by integrating visualizations within reports, businesses can present a compelling narrative about their data findings, facilitating better push for decision-based actions.
In essence, SPSS transforms raw data into actionable insights, streamlining both the analysis and presentation processes, essential for any data-driven organization.
Key Applications of SPSS
Understanding the various applications of SPSS is crucial for grasping its significance in the field of business analytics. SPSS, which stands for Statistical Package for the Social Sciences, is not just a data analysis tool but a versatile software that holds great potential in helping organizations make informed decisions. By diving into specific applications, we can better appreciate how this software empowers companies to harness data effectively, thus improving operational efficiency and strategic planning.
Market Research
Market research is perhaps one of the most prevalent applications of SPSS. Businesses rely on market research to gather insights into consumer preferences, market trends, and competitive dynamics. SPSS provides robust statistical capabilities that assist in survey data analysis, segmentation, and predictive modeling.
For instance, consider a company launching a new product. Using SPSS, they can analyze survey responses to determine which demographics show the most interest in their product. Techniques like factor analysis and cluster analysis enable researchers to identify patterns and group similar responses together. Consequently, this allows the marketing team to tailor their campaigns more effectively, optimizing both reach and engagement.
Moreover, SPSS can also facilitate regression analysis to forecast sales based on varying market conditions. When a data analyst enters historical sales data into SPSS, the software can project future trends, giving teams a clearer picture of potential outcomes. Thus, SPSS turns raw data into actionable insights that can significantly influence business strategy in a competitive marketplace.
Quality Control
In today’s fast-paced environment, maintaining high standards in product quality is indispensable. SPSS offers powerful tools that are invaluable in a company’s quality control processes. By analyzing data related to production and quality performance, organizations can pinpoint weaknesses in their processes and implement corrective measures before they escalate.
Take, for example, a manufacturing firm that faces high defect rates in its production lines. Using SPSS, quality assurance teams can employ techniques such as control charts and hypothesis testing to analyze defect data. This helps them identify trends and variations in production quality over time.
Additionally, through analysis of variance (ANOVA), teams can evaluate the effectiveness of changes in production methods. If one factory consistently produces fewer defects than another, SPSS can help determine whether this is due to operational differences, thereby guiding best practices across the organization.
Customer Analytics
Understanding customer behavior is critical for driving sales and fostering loyalty. SPSS aids businesses in delving deeply into customer analytics by processing vast amounts of customer data efficiently. Organizations can leverage SPSS to build customer profiles, track purchasing behavior, and even predict future purchases.
For instance, retailers find customer-centric insights particularly beneficial. By analyzing shopping patterns and website interactions, SPSS can assist in identifying segments that are most likely to respond to personalized marketing campaigns. Predictive analytics powered by SPSS allows businesses to anticipate customer needs, thus honing their marketing initiatives.
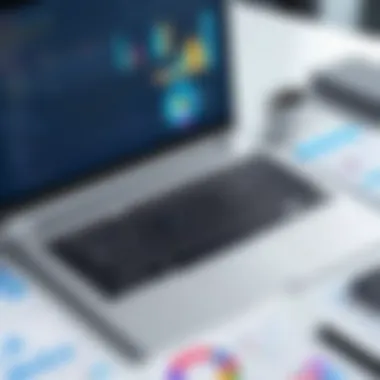
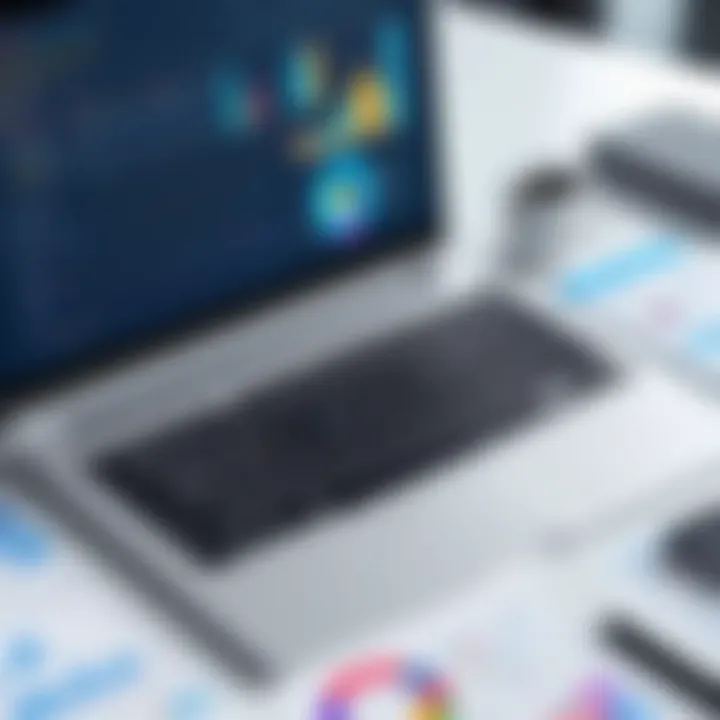
Furthermore, SPSS's ability to perform sentiment analysis on customer feedback can provide insight into customer satisfaction and preferences. Companies can refine their offerings based on direct feedback, thus fostering a stronger relationship with their clientele.
In sum, the applications of SPSS in market research, quality control, and customer analytics demonstrate its far-reaching impact on informed decision-making and strategic planning.
Integrating SPSS with Other Technologies
In today's increasingly interconnected digital world, the ability to leverage various technologies in tandem with SPSS can significantly enhance business analytics capabilities. SPSS's power does not merely lie in its standalone features; rather, its true potential reveals itself when it's woven into the fabric of broader technological ecosystems. By integrating SPSS with other tools, growing organizations unlock enhanced data analysis functionalities and boost their decision-making agility.
SPSS and SQL Databases
SPSS naturally complements SQL databases, as both platforms serve distinct but essential roles in data management and analysis. SPSS excels in statistical analysis, while SQL databases are adept at storing large volumes of structured data efficiently. When these technologies converge, businesses can draw upon the best of both worlds.
Using SPSS in conjunction with SQL databases enables seamless data retrieval and manipulation. For instance, a marketing team may maintain customer data within a SQL database. By querying specific segments of this data directly into SPSS, analysts can swiftly generate insights, without getting bogged down in the complexities of data migration.
Additionally, SPSS offers various methods to connect to SQL databases, including ODBC (Open Database Connectivity) and JDBC (Java Database Connectivity). This flexibility allows organizations to adapt their integration strategy based on unique requirements. Ultimately, this collaboration streamlines the analytical process and facilitates more informed, data-driven decision making.
Working with R and Python
The growing popularity of programming languages like R and Python among data scientists has encouraged SPSS to embrace integration with these powerful tools. Each language has its own strengths, enabling users to perform diverse analytical tasks effectively; therefore, the ability to leverage them alongside SPSS is a major advantage.
R, known for its robust statistical capabilities, draws a vast community of users who constantly contribute packages and libraries. When SPSS is integrated with R, analysts can apply advanced statistical methods, enriching the analytical output with cutting-edge techniques.
On the other hand, Python’s versatility shines when it comes to data manipulation and machine learning. With packages like Pandas for data analysis and Scikit-learn for predictive modeling, Python offers an effective toolkit for data preparation and modeling processes. By incorporating Python scripts in SPSS, businesses can enhance their analytics workflows, automating repetitive tasks and implementing intricate data transformations.
When SPSS integrates seamlessly with R and Python, the synergy between these platforms can vastly elevate the analytics landscape within any organization.
Challenges of Using SPSS
While SPSS stands as a powerful tool in the realm of business analytics, it's not without its hurdles. Recognizing these challenges is essential for businesses aiming to maximize their investment in this software. By addressing these difficulties, organizations can ensure smoother implementation and utilization, facilitating better data-driven decision-making.
Cost Considerations
One of the most immediate challenges associated with SPSS is the cost. Acquiring a license can be a hefty investment, particularly for small to medium-sized enterprises. The price can vary depending on the usage—whether it's for a single user or an enterprise license.
- License Types: SPSS offers various licensing options, but organizations need to clearly define their requirements to choose the most cost-effective package. Enterprises may find that paying for a premium version provides advanced analytics features that can offset the initial cost in terms of better insights and improved results.
- Additional Costs: Beyond the license, there are costs related to training employees and maintaining the latest software updates. It's crucial for companies to factor these expenses into their overall budget when considering SPSS.
Despite the substantial initial outlay, many organizations find that the insights gained from using SPSS generate a strong return on investment, but that’s often a long-term perspective that requires patience and strategic planning to realize.
Learning Curve
Another significant hurdle is the learning curve that comes with SPSS. For new users, especially those without a strong statistical background, navigating the software can feel akin to learning a foreign language. It demands not only familiarity with the interface but also a solid grasp of statistical concepts.
- Training Needs: Investing in comprehensive training for staff is imperative. Without proper training, employees may struggle to leverage the full potential of SPSS, hindering data analysis efforts. For example, a business that deploys SPSS without adequate training may miss out on key features, such as predictive analytics or advanced statistical modeling.
- User Support: It’s important to have a supportive environment where users can share tips, troubleshoot, and collaborate on projects. This can often mitigate the struggle associated with onboarding new users.
As daunting as this learning curve may seem, it can be overcome with the right resources and a dedicated approach.
Data Privacy Concerns
In the age of data-driven decisions, concerns around data privacy are paramount. SPSS processes sensitive information, and businesses must be diligent about securing that data.
- Regulatory Compliance: Organizations must ensure that their use of SPSS complies with all relevant regulations, such as GDPR or HIPAA. Non-compliance can lead to hefty fines, reputational damage, and loss of customer trust.
- Data Handling Practices: Proper data handling practices are essential. This involves not just securing data during analysis but also ensuring that it's anonymized where necessary. That means setting up protocols for data storage and accessibility, so only authorized personnel can access sensitive information.
"A robust data privacy strategy is not just an obligation; it's a critical component of business reputation and customer trust."
Addressing these challenges related to SPSS does take effort, but it’s an investment that can pave the way for enhanced data-driven decision-making and ultimately success in business analytics.
Future of SPSS in Business Analytics
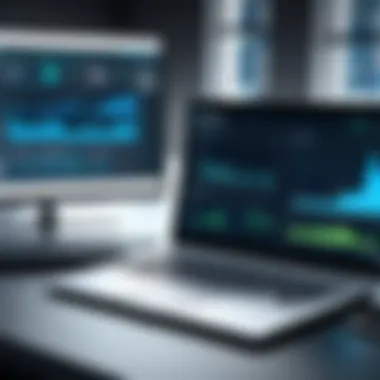
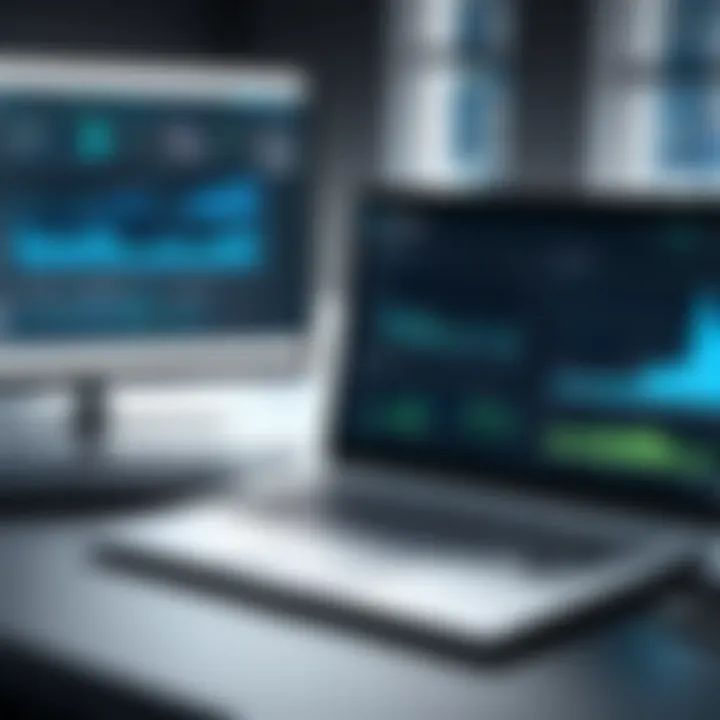
As businesses strive to keep up with the rapid changes in technology and consumer expectations, the future of SPSS in business analytics holds significant importance. Understanding how SPSS adapts to evolving landscapes and integrates new technologies will help organizations leverage its full potential. The relevance of this discussion lies in not only the features SPSS currently offers but also the anticipated advancements that could shape future analytics processes.
Emerging Trends
Technology constantly ebbs and flows, and SPSS must keep pace with those shifts. Several emerging trends are evident that will likely influence the trajectory of SPSS in business analytics:
- Cloud Computing: Many companies are migrating towards cloud-based solutions. SPSS will need to enhance its capabilities to function seamlessly in cloud environments, allowing for better data sharing and collaboration among teams.
- Real-time Data Processing: Consumers now expect instant insights. Future iterations of SPSS may need to focus on quick data processing abilities, enabling businesses to make timely decisions based on up-to-date information.
- User-Friendly Interfaces: Trust me when I say that a complex interface can turn users away. Emerging trends indicate a demand for more intuitive designs and user experiences that ease data handling, making it more accessible to non-technical users.
- Integration with Other Tools: Businesses are using a variety of software. SPSS’s capacity to integrate well with other data analytics tools and platforms will be essential, where seamless connectivity can offer more comprehensive analytics approaches.
Consideration of these trends will better position SPSS in the analytics landscape, ultimately benefiting organizations that rely on it for critical business decisions.
Impact of Artificial Intelligence
Artificial intelligence is a game changer in the analytics space. SPSS is on the brink of embracing AI, which could reshape how data analysis is done:
- Predictive Analytics: One of the most significant impacts AI can make is in predictive analytics. Integrating AI models can enhance SPSS’s predictive capabilities, allowing businesses to anticipate future trends and behaviors with greater accuracy.
- Machine Learning Enhancements: Future developments may include more robust machine learning algorithms within SPSS that automate aspects of the analytical process. This can ease the data mining workload for users, freeing them to focus on interpretation and strategy.
- Natural Language Processing: AI can improve user interactions with SPSS through natural language processing. Imagine typing a query in plain English and getting the analysis without needing to delve into complex statistical jargon! This feature could make data analytics accessible to a wider audience.
- Automated Insights: With AI, SPSS might offer automated insights based on the data it analyzes. By indicating trends and anomalies, it enables users to act more swiftly and decisively without delving into the nitty-gritty of the data form.
"The fusion of SPSS and AI promises not only to drive efficiencies but also infuse strategic foresight into business operations through data-driven insights."
In essence, the future of SPSS in business analytics isn't a static landscape; it is a rapidly evolving tapestry woven together by technological trends and consumer needs. This evolution not only enhances the software but also empowers organizations to make informed decisions that can ultimately shape their trajectory.
Case Studies: SPSS in Action
In the realm of business analytics, case studies serve as vital benchmarks, showcasing real-world applications of theories and tools. This section focuses on the significance of employing SPSS through practical examples. It demonstrates how organizations leverage this powerful software to translate data into actionable insights. Through collected narratives, we will examine how actual businesses navigate challenges and capitalize on opportunities using SPSS.
Successful Implementations
When discussing successful implementations of SPSS in businesses, it is essential to look at specific scenarios where companies obtained promising results. For instance, consider a retail giant that wanted to optimize its inventory management system. By utilizing SPSS, the company was able to analyze historical sales data, examine seasonal trends, and predict future demand. This statistical analysis not only streamlined inventory levels but also boosted profit margins, as items were neither overstocked nor understocked.
Such application of SPSS can be observed across various sectors. In the healthcare industry, hospitals often employ SPSS for patient data analysis. By evaluating admission rates, recovery times, and resource allocation, medical facilities can enhance patient care significantly. One specific hospital adopted SPSS to study readmission rates and identify patterns associated with higher risks. The analysis led to tailored care plans that reduced readmission rates by over fifteen percent in one year.
"By embracing statistical tools like SPSS, organizations can transform raw data into strategies that work."
In another scenario, consider a marketing agency that needed to assess customer satisfaction levels. They gathered survey responses and used SPSS's robust analytics capabilities to uncover trends. Through segmentation and factor analysis, they could pinpoint critical factors affecting customer satisfaction and develop targeted campaigns to address those concerns.
Lessons Learned
As with any initiative, implementing SPSS comes with its own share of learning opportunities. Through the examined cases, some common lessons can be gleaned.
- Importance of Clean Data
The foundation of any successful SPSS implementation is quality data. Organizations should focus on cleaning and preparing their datasets before diving into analysis. A clear strategy for data management can save time and enhance the reliability of results. - Cross-Department Collaboration
Cases show that successful use of SPSS often involves collaboration across departments. For example, merging insights from marketing and sales teams can lead to a more comprehensive understanding of consumer behavior, facilitating more effective campaigns. - Continuous Learning
Many companies learned the hard way that analytics is not a one-off task. Continuous training for employees on SPSS can lead to ongoing insights. Investing in professional development can ensure that the organization stays ahead in the competitive analytics landscape. - Keeping an Eye on Ethical Considerations
As businesses employ SPSS for analyzing sensitive data, the lesson learned is to remain vigilant about data privacy. Ethical data handling, coupled with compliance with regulatory frameworks, becomes paramount.
In summary, examining successful implementations and the subsequent lessons learned highlights that the role of SPSS in business analytics is not just about numbers and statistics. It embodies a strategic approach to decision-making that continually evolves, adapts, and grows. This serves as a reminder of how vital data analysis tools are in today’s business environment.
Closure
The conclusion serves as a critical focal point in any rich discussion about SPSS in business analytics. It is not merely a summation, but rather an opportunity to reflect on the significance of the insights presented throughout the article. Understanding the multifaceted role SPSS plays allows organizations to harness the power of data effectively, paving the way for informed decision-making.
Recap of Key Insights
In summarizing key insights from our exploration of SPSS, a few elements stand out:
- Data-Driven Decision Making: SPSS empowers businesses to leverage their data, transforming raw numbers into actionable insights. This process is essential for shaping strategies that can resonate with target demographics and respond accurately to market needs.
- Integration with Technology: The ability to seamlessly integrate SPSS with other technologies like SQL and programming languages, for instance, R and Python, broadens its utility. It enables users to manipulate and analyze extensive datasets with greater flexibility and precision.
- Visualization and Reporting: Effective communication of data insights is enhanced by SPSS’s visualization tools, which help present complex information in an understandable format, making it easier for stakeholders to grasp important trends and metrics.
Each of these points reinforces the idea that the right tools, such as SPSS, are vital for organizations to thrive in a competitive landscape.
Final Thoughts on SPSS's Role in Business Analytics
As we conclude this discussion, it is essential to recognize that the role of SPSS in business analytics stretches far beyond basic data analysis.
"Data is the new oil, and SPSS is the refinery that turns it into a product of valuable insights."
SPSS not only helps in the meticulous examination of data but also enhances predictive frameworks and facilitates strategic planning. In an age where data-driven insights are paramount, SPSS emerges as a powerful ally in the quest for efficiency and effectiveness in business processes. Its adaptability to emerging technologies and the ability to keep pace with industry trends further solidify its place in the toolkit of IT professionals and analysts alike.
Ultimately, investing time and resources into mastering SPSS can bear significant fruit, fostering a culture of informed decision-making and continuous improvement within organizations. The landscape of business analytics will only continue to evolve, and those who leverage SPSS effectively will indeed find themselves several steps ahead.