Unleashing the Potential of Self-Service Analysis: An Extensive Guide
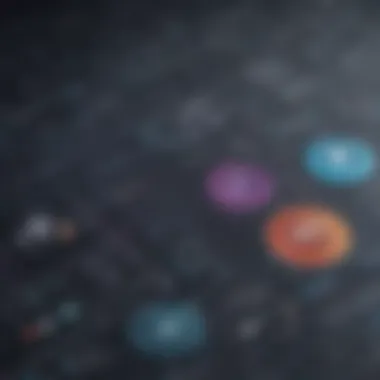
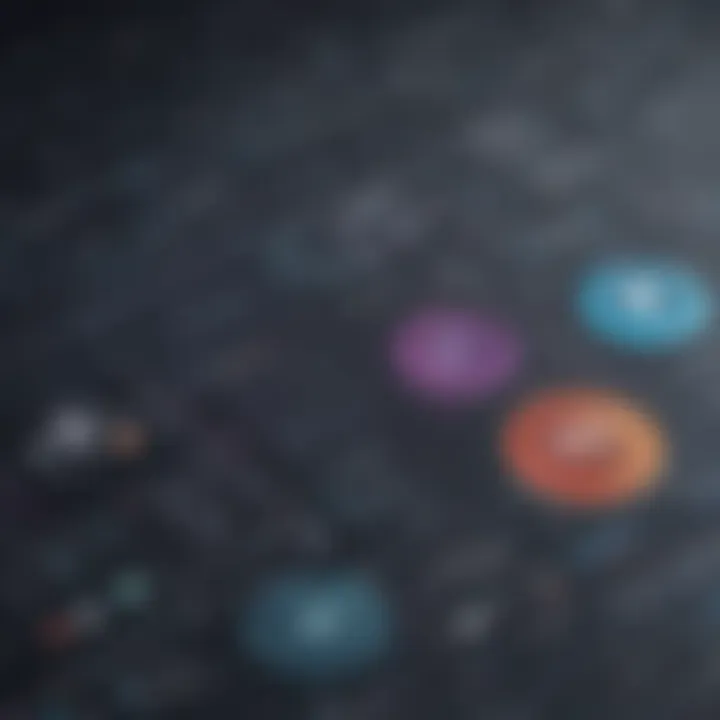
Understanding Storage, Security, or Networking Concepts
Self-service analysis empowers individuals to extract valuable insights from data without requiring extensive technical expertise. In today's rapidly evolving digital landscape, the ability to navigate through vast datasets efficiently has become a critical skill. Understanding the basics of storage, security, and networking lays the foundation for effective self-service analysis. From comprehending storage infrastructure to grasping security protocols and networking structures, a solid grounding is essential.
Key terminology in this realm includes storage types such as cloud storage, databases, and data warehouses; security terms like encryption, authentication, and access control; and networking concepts including routers, switches, and protocols. Familiarity with these terms is fundamental for engaging in self-service analysis confidently.
An overview of important concepts is crucial for successfully unlocking the power of self-service analysis. Storage concepts encompass data retrieval, backup, and archiving; security includes threat detection, incident response, and compliance; networking covers bandwidth, latency, and packet routing. By recognizing these concepts, individuals can navigate the complexities of data analysis more effectively.
Introduction to Self-Service Analysis
In the dynamic landscape of data analytics, embracing self-service analysis has become indispensable. The realm of self-service analysis offers a gateway to empower users from various domains to harness the potential of data-driven insights efficiently. By introducing readers to the fundamental concepts and advanced techniques of self-service analysis, this guide aims to equip individuals with a profound understanding of its significance in the contemporary data ecosystem. Through a comprehensive exploration of self-service analysis, this section will delve into the intricacies that define this transformative practice.
Defining Self-Service Analysis
Self-service analysis stands as a pivotal cornerstone in the realm of modern data analytics, revolutionizing traditional approaches towards data interpretation and decision-making processes. Within this section, we will dissect key elements integral to understanding self-service analysis and its practical implications.
Impact of Self-Service Analysis on Decision-Making
At the core of self-service analysis lies its profound impact on decision-making processes. By enabling users to independently access and analyze data, self-service analysis empowers swift and informed decision-making. This unique characteristic not only streamlines operational efficiency but also fosters a culture of data-driven decision-making within organizations. It revolutionizes the decision-making landscape and propels organizations towards agile and data-centric frameworks.
Evolution of Self-Service Analysis Tools
The evolution of self-service analysis tools signifies a paradigm shift in data accessibility and utilization. These tools have undergone substantial advancements, catering to the evolving needs of users across diverse industries. The integration of user-friendly interfaces, augmented analytics features, and real-time collaboration capabilities has redefined the way users interact with data. However, while these tools offer unparalleled convenience and flexibility, they also pose challenges related to data governance and security, necessitating a balanced approach towards their implementation.
Benefits of Self-Service Analysis in Business Operations
The adoption of self-service analysis yields a myriad of benefits that resonate across various facets of business operations. By promoting data literacy and democratizing data access, organizations can cultivate a data-driven culture that transcends siloed information. Enhanced data exploration and visualization capabilities enable users to derive actionable insights, driving operational efficiencies and strategic decision-making. Despite these advantages, organizations must navigate potential pitfalls such as ensuring data quality and fostering a collaborative data governance framework.
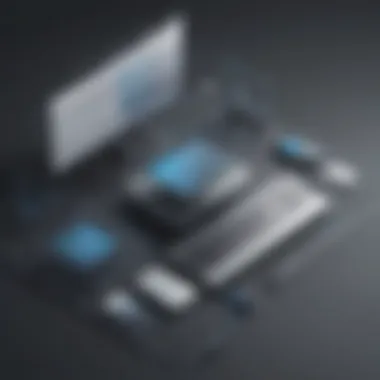
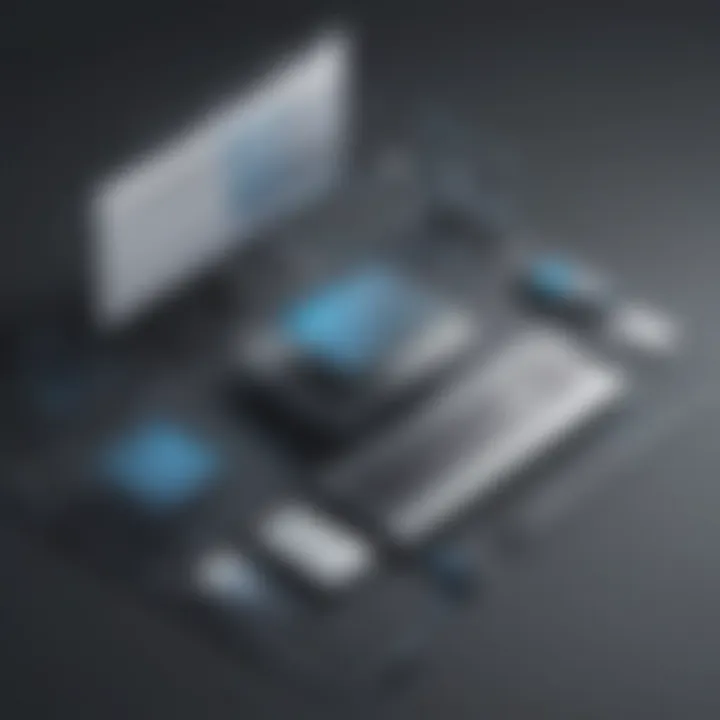
Practical Applications of Self-Service Analysis
Implementing Self-Service Analysis in Enterprises
Challenges and Opportunities:
One of the key aspects to address when implementing self-service analysis in enterprises is navigating through the challenges and opportunities presented. Enterprises face the challenge of data silos, where information is fragmented across departments or systems. However, this challenge also signifies an opportunity to centralize data and streamline analytics processes for enhanced decision-making. By addressing data integration issues and ensuring data quality and consistency, enterprises can unlock the full potential of self-service analysis tools. This section sheds light on the importance of tackling challenges head-on to leverage opportunities for growth and innovation within organizations.
Best Practices for Successful Implementation:
When it comes to successful implementation of self-service analysis in enterprises, certain best practices play a pivotal role. Emphasizing training and upskilling employees in using these tools is essential for adoption and maximizing their benefits. Establishing clear data governance policies and promoting a data-driven culture are foundational aspects of successful implementation. By fostering a user-centric approach and providing adequate support and resources, enterprises can overcome implementation hurdles and drive sustainable growth through self-service analysis.
Case Studies of Self-Service Analysis Integration:
In this section, we examine real-world case studies that demonstrate the integration of self-service analysis within enterprises. By exploring how companies have successfully implemented these tools to drive data-driven decision-making, we can draw valuable insights and learn from their experiences. Case studies offer a practical lens through which readers can understand the impact and outcomes of self-service analysis integration. These examples showcase the diverse applications and benefits of self-service analysis across different industry verticals, providing a comprehensive view of its potential within enterprise settings.
Self-Service Analysis in Data Science
Enhancing Data Discovery and Exploration:
Enhancing data discovery and exploration through self-service analysis tools is instrumental in unlocking valuable insights and patterns within large datasets. By empowering users to interact with and visualize data in intuitive ways, these tools facilitate a deeper understanding of information landscapes. The capability to explore data dynamically and uncover hidden trends enhances the decision-making process and drives data-driven strategies within data science environments. This section delves into the specific features and benefits of enhancing data discovery and exploration through self-service analysis, highlighting its pivotal role in driving actionable insights.
Predictive Analytics Using Self-Service Tools:
Predictive analytics is a key component of data science, enabling organizations to forecast trends and anticipate future outcomes based on historical data. By utilizing self-service tools for predictive analytics, users can leverage machine learning algorithms and AI capabilities to generate accurate forecasts and model complex scenarios. The integration of predictive analytics within self-service analysis empowers users to make informed decisions and strategize effectively in rapidly evolving business landscapes. This subsection explores the unique advantages and considerations of incorporating predictive analytics into self-service analysis frameworks.
Data-Driven Decision-Making Strategies:
Data-driven decision-making strategies are at the core of leveraging self-service analysis tools for business growth and innovation. By formulating strategies that prioritize data validity, relevance, and timeliness, organizations can harness the power of self-service tools to drive informed decision-making. This section emphasizes the importance of aligning decision-making processes with data insights to optimize operational efficiency and drive competitive advantage. By adopting data-driven decision-making strategies, enterprises can stay agile, proactive, and responsive to evolving market dynamics, ensuring sustainable growth and success.
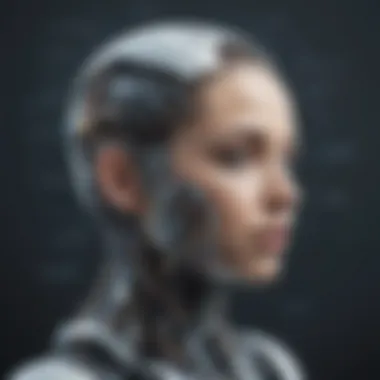
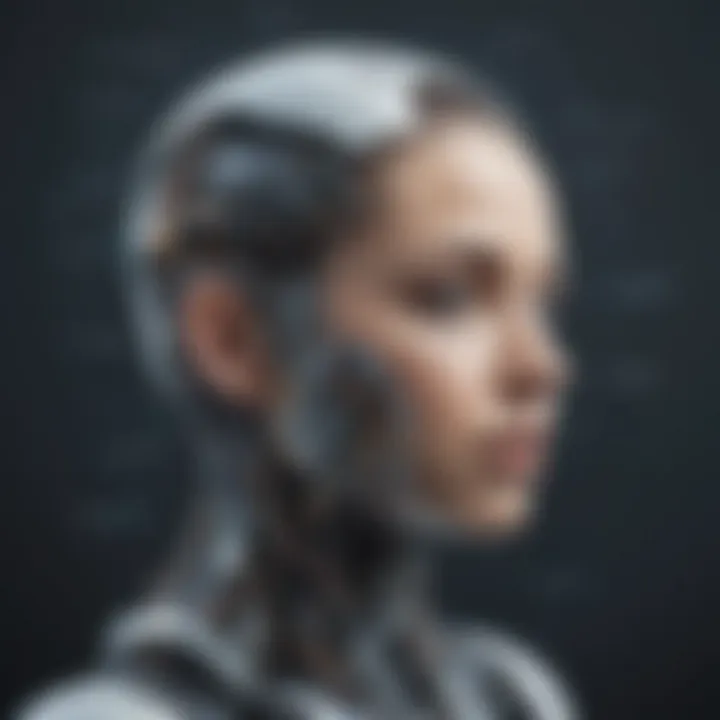
Advanced Techniques in Self-Service Analysis
Advanced Techniques in Self-Service Analysis play a pivotal role in empowering users to extract valuable insights and make informed decisions in today's data-driven ecosystem. These techniques encompass cutting-edge methodologies that elevate the efficiency and accuracy of analysis processes, ultimately enhancing business operations and strategic decision-making. By leveraging advanced techniques, organizations can delve deeper into their data, uncovering hidden patterns and trends that may not be apparent through traditional analysis methods. The integration of advanced techniques in self-service analysis revolutionizes how data is processed and interpreted, enabling users to unlock the full potential of their datasets.
Machine Learning and AI in Self-Service Analysis
Automating Insights Generation
Automating Insights Generation is a crucial aspect of self-service analysis that automates the process of deriving valuable insights from complex datasets. This automation streamlines the analysis workflow, saving time and reducing the margin of error. By implementing machine learning algorithms, automated insights generation enhances the accuracy of analysis results, leading to more reliable decision-making. The key characteristic of Automating Insights Generation lies in its ability to handle large volumes of data swiftly, identifying significant patterns and anomalies efficiently. While the advantages of automation are evident in improving operational efficiency and scalability, potential disadvantages include the need for continuous fine-tuning to ensure the accuracy and relevance of generated insights.
Personalizing User Experiences
Personalizing User Experiences in self-service analysis tailors analytical processes to individual user preferences and requirements. This customization enhances user engagement and comprehension by presenting data in a user-friendly and intuitive manner. The key characteristic of personalization is its ability to adapt analysis interfaces and functionalities based on user interactions, ultimately improving user satisfaction and productivity. Personalization is a popular choice in self-service analysis as it promotes user adoption and facilitates seamless navigation through complex datasets. However, while personalization offers benefits in enhancing user experience, challenges may arise in balancing customization with data integrity and compliance requirements.
Forecasting Trends with AI Integration
Forecasting Trends with AI Integration in self-service analysis involves leveraging artificial intelligence algorithms to predict future patterns and trends based on historical data. This predictive capability enables users to anticipate market changes, customer behaviors, and emerging opportunities, empowering proactive decision-making. The key characteristic of Forecasting Trends with AI Integration is its capacity to analyze vast datasets rapidly and generate accurate forecasts with minimal human intervention. This feature is particularly beneficial for organizations seeking to stay ahead of the competition and capitalize on upcoming trends. While the advantages of AI integration in forecasting are significant, considerations surrounding data privacy, bias, and model interpretability should be addressed to ensure ethical and reliable decision support.
Collaborative Analytics Platforms
Facilitating Team-Based Analysis
Facilitating Team-Based Analysis in self-service analysis fosters collaboration and knowledge-sharing among team members, enhancing collective problem-solving and decision-making processes. This collaboration platform allows multiple users to work together on analyzing data, exchanging insights, and evaluating results in real-time. The key characteristic of team-based analysis lies in its ability to streamline communication, increase transparency, and boost productivity within organizational teams. Team-based analysis is a beneficial choice for this article as it promotes a synergistic approach to data analysis, combining diverse perspectives and expertise for comprehensive insights. While the advantages of collaborative platforms are evident in promoting teamwork and knowledge transfer, challenges related to data security and access control necessitate robust governance and control mechanisms.
Real-Time Collaboration Features
Real-Time Collaboration Features in self-service analysis enable users to collaborate instantaneously on data analysis tasks, regardless of geographical locations or time zones. This real-time interaction fosters agile decision-making processes, enabling stakeholders to respond promptly to changing conditions and evolving insights. The key characteristic of real-time collaboration features is their ability to synchronize data views and workflows, providing a seamless experience for users working concurrently on analysis projects. Real-time collaboration features are a popular choice for this article as they promote efficiency, agility, and cross-functional collaboration in dynamic business environments. While the benefits of real-time collaboration are significant in accelerating decision-making and enhancing team productivity, considerations related to data consistency, version control, and communication protocols are essential for successful implementation.
Enhancing Data Governance and Security
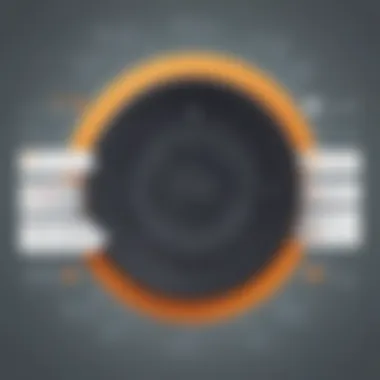
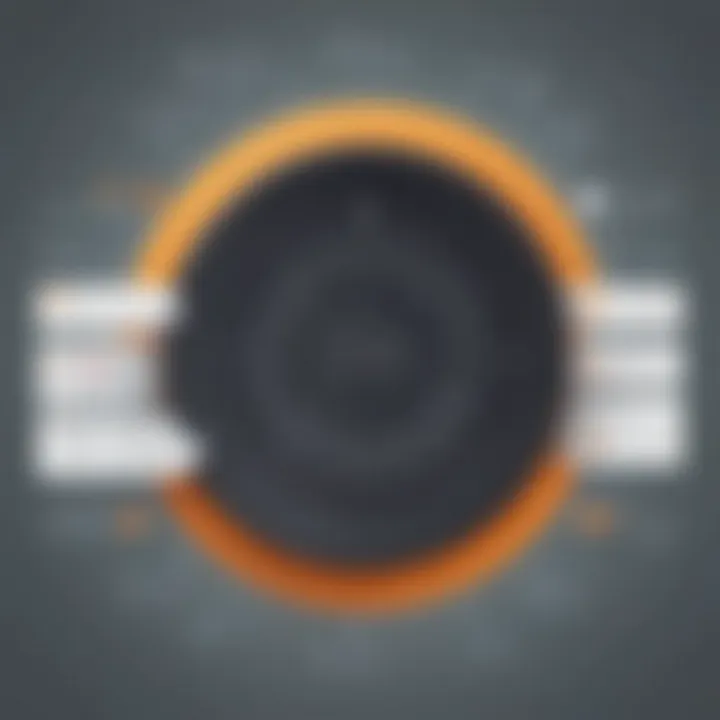
Enhancing Data Governance and Security in self-service analysis involves implementing robust policies, procedures, and technologies to ensure data integrity, confidentiality, and compliance throughout the analysis lifecycle. This governance framework encompasses data access controls, auditing mechanisms, encryption protocols, and risk mitigation strategies to protect sensitive information and maintain regulatory adherence. The key characteristic of data governance and security lies in its capacity to safeguard organizational data assets, build trust with users, and uphold ethical standards in data handling. Enhancing data governance and security is a beneficial choice for this article as it underscores the importance of maintaining data quality, reliability, and confidentiality in self-service analysis environments. While the advantages of data governance and security are paramount in fostering data trust and regulatory compliance, challenges related to data silos, user permissions, and data breaches necessitate continuous monitoring and proactive measures to mitigate risks.
Challenges and Future Trends in Self-Service Analysis
In the ever-evolving landscape of data analytics, discussing the challenges and future trends in self-service analysis is of utmost importance. As organizations strive to harness the power of data, they encounter hurdles related to data silos and integration issues. Addressing these challenges is crucial for unlocking the full potential of self-service analysis tools. Overcoming data silos involves breaking down barriers between different data sources to ensure seamless data flow and accessibility across the organization. Integration issues, on the other hand, revolve around the seamless integration of various tools and technologies to enable cohesive data analysis processes. These challenges highlight the need for robust strategies and innovative approaches to streamline data processes and drive informed decision-making.
Overcoming Data Silos and Integration Issues
Ensuring Data Quality and Consistency
Ensuring data quality and consistency is a critical aspect of overcoming data silos and integration issues in self-service analysis. By establishing data governance frameworks and quality standards, organizations can maintain data integrity and accuracy. Consistent data quality ensures that insights derived from self-service analysis tools are reliable and actionable. Implementing data quality checks, validation processes, and data cleansing techniques are essential to enhance the trustworthiness of analytical outcomes. In this article, we emphasize the significance of data quality and consistency in driving effective decision-making processes and maximizing the value of self-service analysis tools.
Interoperability of Self-Service Tools
Interoperability of self-service tools plays a key role in connecting disparate data sources and enabling seamless data exchange. This interoperability empowers organizations to integrate multiple tools and technologies, allowing data to flow smoothly across various platforms. The seamless interaction between different self-service analysis tools enhances collaboration, accelerates data processing, and improves overall efficiency. Leveraging interoperable tools enables users to access, analyze, and share data effortlessly, leading to a more integrated analytical environment. While interoperability offers numerous benefits in enhancing data accessibility and connectivity, organizations must also address compatibility challenges and ensure smooth integration to optimize the use of self-service analysis solutions.
Strategies for Unified Data Access
Strategies for unified data access focus on breaking down data silos and promoting a centralized approach to data management. By implementing robust data access strategies, organizations can consolidate data repositories, eliminate redundancies, and provide users with a unified view of data assets. Unified data access ensures that stakeholders across the organization can access relevant data sources efficiently, facilitating a cohesive data analysis process. This centralized approach fosters collaboration, enhances data visibility, and streamlines decision-making processes. While strategies for unified data access offer substantial benefits in promoting data democratization and enhancing data utilization, organizations must adopt tailored approaches to address specific data requirements and ensure data security and governance.
Predictive Analytics and Prescriptive Insights
Predictive analytics and prescriptive insights represent advanced capabilities that drive informed decision-making and shape future trends in self-service analysis. By leveraging emerging technologies, organizations can forecast trends, gain actionable insights, and empower users with AI-driven decision support. Emerging technologies such as machine learning and artificial intelligence revolutionize the data analysis landscape, enabling organizations to automate insights generation, personalize user experiences, and forecast trends with high accuracy. These technologies play a pivotal role in enhancing predictive analytics capabilities, enabling organizations to make data-driven decisions based on real-time insights.
Emerging Technologies Shaping the Future
The integration of emerging technologies is reshaping the future of self-service analysis by introducing advanced analytical capabilities and innovative functionalities. Machine learning algorithms facilitate predictive modeling, anomaly detection, and pattern recognition, empowering organizations to derive valuable insights from complex data sets. Additionally, artificial intelligence enhances decision-making processes by providing AI-driven recommendations, automating repetitive tasks, and enabling proactive problem-solving. The seamless integration of emerging technologies not only enhances analytical efficiency and accuracy but also paves the way for predictive modeling, trend analysis, and intelligent decision support systems. By exploring the potential of emerging technologies, organizations can stay ahead of the curve, drive innovation, and unlock new possibilities in self-service analysis.
Ethical Considerations in Data Usage
Ethical considerations in data usage are paramount in the realm of self-service analysis, where data privacy, consent, and transparency are critical aspects. Organizations leveraging self-service analysis tools must prioritize ethical practices to ensure data protection, privacy compliance, and responsible data usage. Upholding ethical standards entails safeguarding sensitive information, obtaining user consent for data processing, and maintaining data security measures throughout the analytical lifecycle. By embedding ethical considerations into data analysis processes, organizations can build trust with stakeholders, mitigate risks associated with data misuse, and uphold integrity in decision-making. Ethical data usage not only aligns with regulatory requirements but also cultivates a culture of responsible data management and transparency in self-service analysis practices.
Empowering Users with AI-Driven Decision Support
Empowering users with AI-driven decision support amplifies the capabilities of self-service analysis tools by providing intelligent insights, automated recommendations, and personalized user experiences. AI-driven decision support systems leverage machine learning algorithms to deliver predictive analytics, optimize decision models, and enhance data visualization. By empowering users with AI-driven capabilities, organizations can streamline decision-making processes, accelerate data-driven insights, and drive organizational performance. The integration of AI-driven decision support not only enriches user experiences and analytical outcomes but also enables organizations to embrace a data-centric culture, foster innovation, and adapt to dynamic market trends. Empowering users with AI-driven decision support represents a transformative approach to self-service analysis, enhancing analytical agility, and driving sustainable growth.