Unlocking the Strategic Importance of Data Governance in Today's Digital World
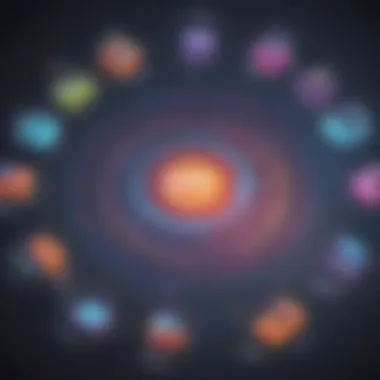
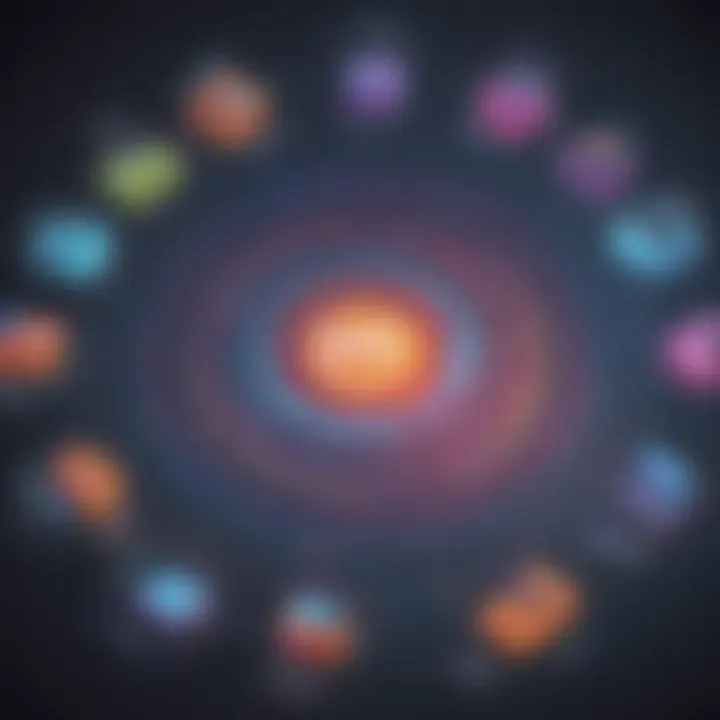
Understanding Data Governance and its Vital Role
In the fast-paced digital landscape, organizations grapple with vast amounts of data daily. Data governance emerges as a crucial aspect to navigate this complexity, ensuring data reliability, security, and compliance. It encompasses the framework, processes, and policies that define how data is managed across an organization. Understanding the fundamental concepts of data governance is paramount for IT professionals and cybersecurity experts striving for effective data management.
Keys to Effective Data Governance
Effective data governance rests on foundational principles that drive decision-making processes and regulatory adherence. Key considerations include data quality, access controls, metadata management, and data lifecycle management. By implementing robust data governance practices, organizations empower themselves to make informed decisions, comply with regulations, and mitigate risks associated with data misuse or breaches.
Embracing Data Governance Best Practices
Optimizing data governance involves adhering to best practices that enhance data reliability and security. Organizations should focus on establishing clear data ownership, implementing data quality assessments, enforcing compliance with data regulations, and fostering a culture of data stewardship. By following these best practices, businesses bolster their data governance framework and pave the way for improved decision-making processes.
Navigating Data Governance in Contemporary Business
In today's dynamic business landscape, data governance faces evolving challenges and opportunities. Stay updated on the latest trends in data governance, cybersecurity threats, and data protection regulations. By embracing innovative solutions and strategies, businesses can proactively address data governance issues, mitigate security risks, and ensure regulatory compliance.
Realizing the Impact of Data Governance: Case Studies
Explore real-life examples of successful data governance implementations and cybersecurity incidents. By delving into these case studies, readers gain practical insights into the value of robust data governance and cybersecurity measures. Learning from past experiences equips organizations with the knowledge to fortify their data governance strategies and safeguard sensitive information.
Evaluation and Comparison of Data Governance Tools
In-depth reviews and comparisons of data governance software and tools provide valuable insights for organizations seeking to bolster their data management practices. By evaluating various solutions, businesses can make informed decisions on selecting the most suitable data governance tools that align with their unique requirements. Stay ahead by leveraging cutting-edge data governance products that enhance operational efficiency and data security.
Introduction to Data Governance
Data governance plays a pivotal role in modern business operations, especially within the digital landscape. It encompasses the strategies, policies, and processes that organizations institute to manage their data efficiently. The significance of data governance lies in its ability to ensure data integrity, facilitate decision-making based on accurate information, and uphold regulatory compliance. This section will delve into various elements of data governance, shedding light on its importance and implications.
Understanding Data Governance
Data Governance Definition
Data governance definition pertains to the set of practices that ensure high data quality and compliance with regulations throughout a data's lifecycle. It involves establishing frameworks for data management, defining ownership, and outlining accountability. The unique aspect of data governance definition is its focus on maintaining data accuracy and reliability. By implementing robust data governance definitions, organizations can enhance operational efficiency and foster trust in their data assets.
Key Components of Data Governance
Key components of data governance include data policies, data processes, and data tools. These components collectively shape how an organization manages and utilizes its data resources. The critical characteristic of key components of data governance is their role in standardizing data practices and ensuring consistency. They serve as the foundation for establishing data governance frameworks tailored to organizational needs.
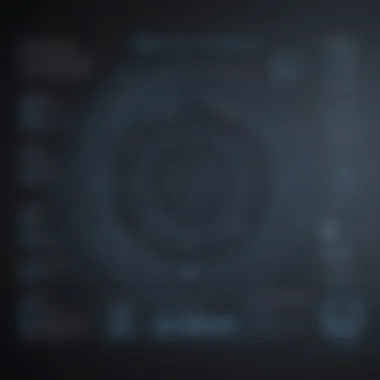
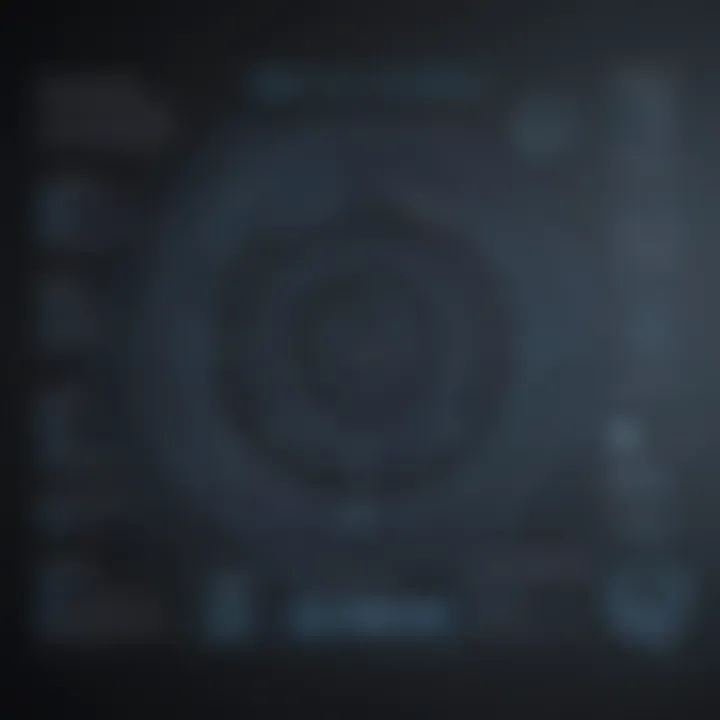
Objectives of Data Governance
The objectives of data governance revolve around improving data quality, enhancing data security, and promoting operational efficiency. By setting clear objectives, organizations can align their data governance strategies with overarching business goals. The key characteristic of data governance objectives is their direct impact on organizational performance and decision-making. Achieving these objectives empowers businesses to leverage data as a strategic asset, driving growth and resilience.
Importance of Data Governance
Ensuring Data Quality and Integrity
Ensuring data quality and integrity is crucial for reliable insights and informed decision-making. This aspect of data governance focuses on maintaining data accuracy, consistency, and completeness. By upholding data integrity, organizations can trust the information they rely on, driving more robust business outcomes. The unique feature of ensuring data quality and integrity is its proactive approach to data management, mitigating risks associated with poor data quality.
Enabling Regulatory Compliance
Data governance plays a key role in enabling regulatory compliance by establishing protocols for data handling and transparency. Regulatory compliance ensures that organizations adhere to legal requirements governing data privacy, security, and usage. By abiding by regulations, companies mitigate compliance risks and build a reputation for trustworthiness. The distinct feature of enabling regulatory compliance is its dual benefit of legal adherence and organizational resilience.
Facilitating Effective Decision-Making
Effective decision-making hinges on access to accurate and timely data, a cornerstone of data governance. By structuring data governance frameworks that support decision-making processes, organizations can enhance their agility and competitiveness. The critical characteristic of facilitating effective decision-making is its impact on strategic alignment and business performance. Data-driven decision-making fueled by robust data governance practices leads to better outcomes and sustainable growth.
Challenges in Data Governance Implementation
Cultural Resistance to Data Governance
Cultural resistance to data governance refers to the reluctance or opposition encountered when introducing data governance practices within an organization's culture. This resistance can stem from a lack of awareness, fear of change, or conflicting priorities. The key characteristic of cultural resistance to data governance is its potential to impede data initiatives and hinder organizational progress. Overcoming this challenge requires effective communication, stakeholder engagement, and change management strategies.
Lack of Standardized Processes
The lack of standardized processes in data governance implementation poses challenges to consistency and efficiency. Without established protocols and procedures, organizations may struggle to govern data effectively and maintain data quality. The unique feature of addressing this challenge is the opportunity to streamline operations, improve decision-making, and enhance data management practices. Implementing standardized processes fosters a culture of data governance excellence and compliance.
Data Security Concerns
Data security concerns encompass the risks and threats associated with safeguarding sensitive data from unauthorized access, breaches, and misuse. This challenge underscores the importance of data protection measures and cybersecurity protocols. The critical characteristic of data security concerns is their potential to disrupt operations, tarnish reputation, and incur regulatory penalties. Addressing data security concerns requires a holistic approach that prioritizes data encryption, access controls, and ongoing monitoring.
Benefits of Robust Data Governance
Improved Data Quality
Improved data quality leads to better decision-making, operational efficiency, and stakeholder trust. Organizations that prioritize data quality enhancements through robust data governance practices experience fewer errors, enhanced analytics accuracy, and improved business outcomes. The key characteristic of improved data quality is its ripple effect on overall organizational performance and competitiveness. By investing in data quality improvements, businesses position themselves for long-term success and sustainability.
Enhanced Data Security
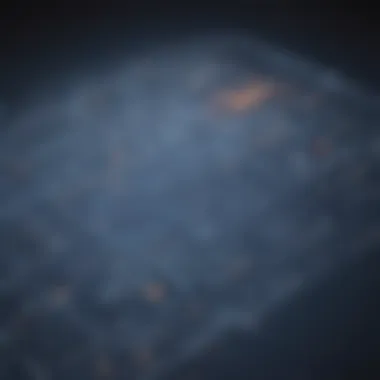
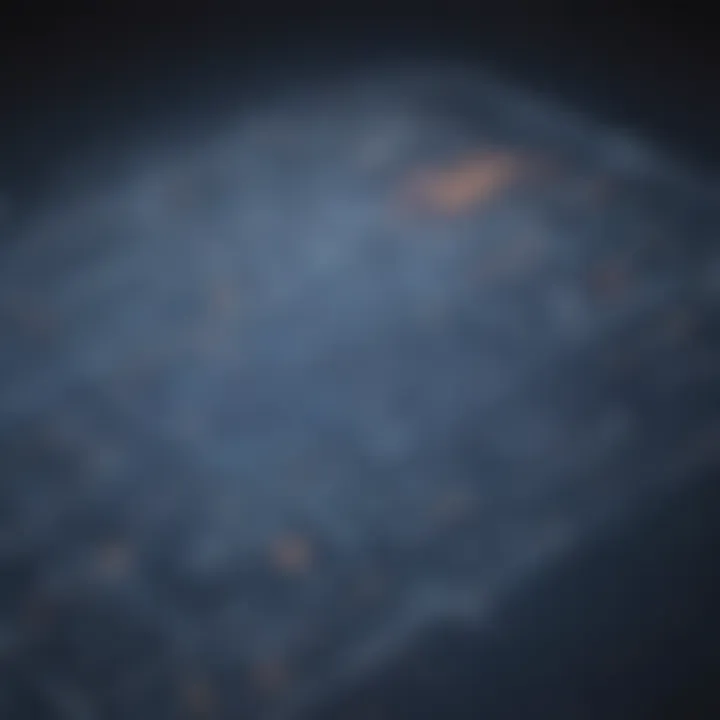
Enhanced data security is paramount in safeguarding sensitive information, maintaining compliance, and mitigating cyber threats. Through stringent data governance measures, organizations can fortify their data infrastructure, detect vulnerabilities, and respond to security incidents effectively. The unique feature of enhanced data security lies in its proactive stance against evolving cyber risks, ensuring data confidentiality and integrity. Prioritizing data security empowers organizations to operate securely in the ever-changing digital landscape.
Greater Operational Efficiency
Greater operational efficiency is a direct outcome of streamlined processes, optimized workflows, and data-driven decision-making facilitated by robust data governance frameworks. By enhancing operational efficiency, organizations can reduce redundancies, improve resource allocation, and accelerate time-to-insight. The critical characteristic of greater operational efficiency is its impact on cost savings, productivity gains, and organizational agility. Embracing data governance principles that prioritize efficiency enables businesses to adapt to market dynamics and drive sustainable growth.
Key Elements of Data Governance Framework
In discussing the Significance of Data Governance in the Digital Landscape, the Key Elements of Data Governance Framework form a crucial focal point. This section delves into the fundamental components that constitute a robust data governance structure, highlighting their importance in modern business operations. The framework consists of key elements such as Data Governance Policies, Data Governance Processes, Data Governance Tools, and Data Governance Best Practices. These elements collectively contribute to enhancing data quality, security, and operational efficiency within organizations, emphasizing the value they bring to data management strategies.
Data Governance Policies
Development and enforcement of data policies
The development and enforcement of data policies are foundational to establishing a structured approach to data governance. By defining clear and concise guidelines for data usage, access, and management, organizations can ensure adherence to regulatory requirements and internal standards. This aspect of data governance empowers enterprises to mitigate risks associated with data misuse or unauthorized access, creating a secure data environment that fosters trust among stakeholders. The proactive implementation of data policies not only safeguards sensitive information but also promotes accountability and transparency throughout the data lifecycle.
Establishing data usage guidelines
Establishing data usage guidelines involves setting parameters for how data is collected, stored, and utilized within an organization. By outlining best practices for data handling and dissemination, companies can optimize data utilization while mitigating misuse or non-compliance risks. Clear data usage guidelines enable employees to make informed decisions regarding data access and sharing, aligning with the overarching objectives of data governance. This aspect of the framework emphasizes the importance of incorporating data ethics and privacy considerations into everyday data practices, ensuring responsible data management across all business functions.
Defining roles and responsibilities
Defining roles and responsibilities within the data governance framework is essential for ensuring accountability and accountability in data-related activities. By assigning specific duties and authority to individuals or teams, organizations can streamline decision-making processes and enhance data governance oversight. Clear role definitions enable effective coordination between different departments and personnel, fostering a collaborative data governance culture. This aspect of the framework underscores the significance of promoting a data-driven mindset across the organization, where every member acknowledges their role in upholding data integrity and security.
Data Governance Processes
Data classification and categorization
Data classification and categorization involve organizing data assets based on predefined criteria, such as sensitivity, criticality, or accessibility. This process enables organizations to prioritize data management efforts, focusing resources on high-value data sets while implementing appropriate security controls for sensitive information. By categorizing data according to its relevance and usage context, companies can streamline data retrieval processes, enhance data quality, and ensure regulatory compliance. Data classification lays the foundation for effective data governance by providing a structured framework for data handling and protection.
Data access control mechanisms
Data access control mechanisms refer to the tools and protocols implemented to regulate data access and authorization within an organization. By enforcing access controls based on user roles, permissions, and authentication mechanisms, enterprises can prevent unauthorized data exposure and misuse. Robust data access controls enhance data security posture, reducing the risk of data breaches and insider threats. This aspect of the data governance framework emphasizes the importance of balancing data accessibility with security requirements, ensuring that data access aligns with business objectives while maintaining data integrity.
Data lifecycle management
Data lifecycle management encompasses the processes and technologies used to manage data from creation to disposal. This includes data capture, storage, processing, analysis, archiving, and deletion activities that occur throughout the data lifecycle. Effective data lifecycle management ensures data availability, consistency, and longevity, supporting informed decision-making and compliance requirements. By implementing structured data lifecycle workflows, organizations can optimize data usage, reduce storage costs, and adhere to data retention policies. This aspect of the framework highlights the significance of treating data as a valuable asset that requires comprehensive management throughout its lifecycle.
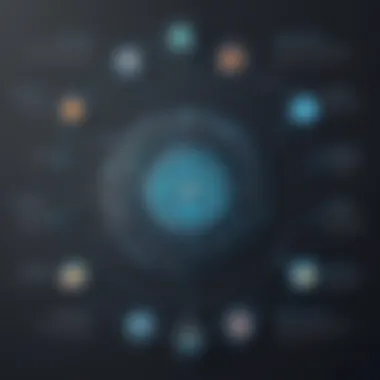
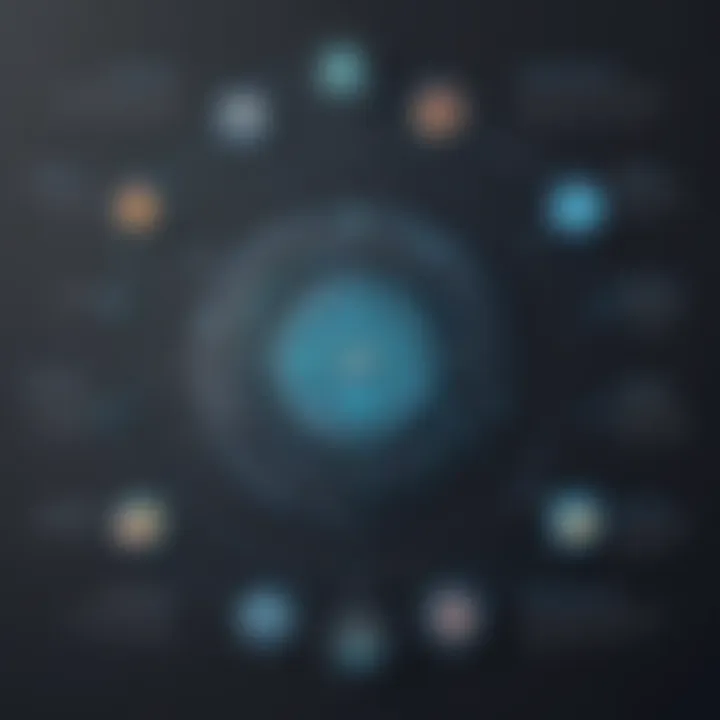
Data Governance Tools
Metadata management systems
Metadata management systems play a vital role in data governance by providing organizations with insights into the structure, usage, and context of their data assets. These systems capture metadata attributes, such as data lineage, definitions, and relationships, enabling stakeholders to understand data provenance and semantics. By centralizing metadata management, companies can improve data discoverability, lineage tracking, and compliance reporting. Metadata management systems enhance data governance by facilitating data transparency, collaboration, and decision-making based on accurate and reliable metadata information.
Data lineage tools
Data lineage tools offer visibility into the end-to-end data flow within an organization, showcasing how data moves from source to destination and undergoes transformations along the way. These tools track data origins, transformations, and dependencies, providing stakeholders with a comprehensive view of data lineage and impact analysis. By visualizing data lineage, organizations can assess data quality, trace data errors, and ensure regulatory compliance throughout the data lifecycle. Data lineage tools empower data governance initiatives by promoting data accountability, accuracy, and trustworthiness across different data processes and systems.
Data quality assessment tools
Data quality assessment tools enable organizations to evaluate the accuracy, completeness, consistency, and timeliness of their data assets. These tools use predefined metrics and algorithms to assess data quality levels, identify anomalies or errors, and recommend corrective actions. By conducting regular data quality assessments, companies can enhance data reliability, usability, and relevance for decision-making purposes. Data quality assessment tools support data governance efforts by facilitating continuous data validation, remediation, and improvement, ensuring that data remains a valuable and trusted business asset.
Data Governance Best Practices
Establishing a data governance council
Establishing a data governance council involves forming a dedicated group of stakeholders responsible for overseeing data governance initiatives and decisions. The council collaborates with key business units to define data governance objectives, policies, and priorities, aligning data management strategies with organizational goals. By establishing a structured governance framework, companies can institutionalize data governance practices, promote cross-functional communication, and drive data-driven insights across the enterprise. This best practice underscores the significance of executive sponsorship, cross-departmental collaboration, and continuous improvement in sustaining effective data governance principles.
Continuous monitoring and audit
Continuous monitoring and audit processes are essential for tracking data governance performance, identifying issues, and ensuring compliance with data policies and regulations. By regularly monitoring data usage, access patterns, and quality metrics, organizations can proactively address data governance challenges and mitigate risks. Continuous auditing of data management practices enables enterprises to validate data processes, detect vulnerabilities, and implement corrective measures to enhance data security and integrity. This best practice emphasizes the importance of real-time data monitoring, proactive risk management, and accountability in maintaining data governance standards.
Regular review and updates
Regular review and updates of data governance policies, processes, and tools are imperative to adapt to changing business needs and regulatory requirements. By periodically reviewing data governance frameworks, organizations can identify emerging trends, technologies, and risks that may impact data management strategies. Conducting regular updates ensures that data governance practices remain effective, relevant, and aligned with business objectives, promoting agility and resilience in data governance implementation. This best practice highlights the importance of agility, adaptability, and continuous learning in optimizing data governance frameworks for long-term success.
Implementing Data Governance Strategies
Every organization navigating the digital landscape inevitably encounters the crucial task of Implementing Data Governance Strategies. This section delves into the intricacies surrounding the setup of robust data governance mechanisms. Implementing Data Governance Strategies is not just a mere routine but a fundamental pillar affecting the core of modern businesses. Without a well-thought-out data governance strategy in place, businesses risk encountering data chaos and compliance issues. A strategic approach to Implementing Data Governance Strategies can bolster data quality, enhance organizational efficiency, and ensure regulatory adherence. Therefore, navigating the confluence of elements, benefits, and considerations associated with Implementing Data Governance Strategies becomes imperative for any organization striving for data-driven success.
Assessing Organizational Readiness
Evaluating Existing Data Management Practices
In the realm of Assessing Organizational Readiness, Evaluating Existing Data Management Practices serves as a pivotal step. This facet involves scrutinizing the current data management protocols and systems within the organization. By meticulously examining how data is currently handled, organizations can identify loopholes, strengths, and areas warranting improvement. When it comes to Evaluating Existing Data Management Practices, the focus rests on shedding light on the efficacy of current data handling mechanisms. This appraisal aids in paving the way for a seamless transition towards more streamlined and data-centric practices, laying a solid foundation for effective data governance implementation.
Identifying Stakeholders and Champions
Another critical facet of Assessing Organizational Readiness is Identifying Stakeholders and Champions. This element entails pinpointing key individuals within the organization who play a pivotal role in driving data governance initiatives forward. By recognizing stakeholders with a vested interest in data governance and champions who advocate for its importance, organizations can ensure a smoother transition towards embracing data governance practices. Identifying Stakeholders and Champions not only fosters internal support but also helps in cultivating a data-driven culture within the organization, crucial for successful data governance implementation.
Conducting a Gap Analysis
Conducting a Gap Analysis emerges as a vital component in the process of Assessing Organizational Readiness for data governance implementation. This phase involves a meticulous examination of existing practices against industry standards and best practices. By conducting a thorough Gap Analysis, organizations can pinpoint areas where their current data governance framework falls short and where improvements are needed. The insights garnered from this analysis serve as a roadmap for organizations to bridge existing gaps, thereby paving the way for a more robust and effective data governance strategy.