Unraveling the Crucial Role of a Data Quality Manager in Organizations
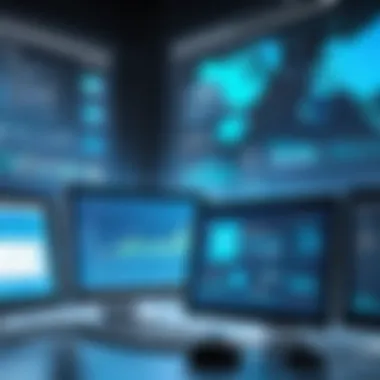
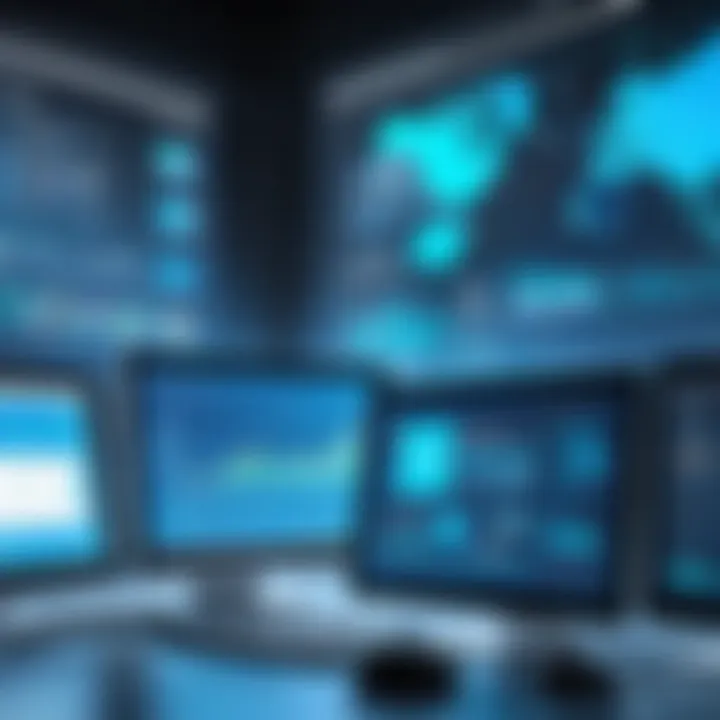
This article delves into the intricate responsibilities and significance associated with the role of a data quality manager. It explores the core functions, skills, and challenges that data quality managers face in ensuring the accuracy, integrity, and reliability of organizational data. Discover the pivotal role of a Data Quality Manager π§ Dive deep into their vital tasks, skills, and challenges in maintaining precise, trustworthy organizational data!
Introduction to Data Quality Management
Data quality management is a critical function within organizations, ensuring the accuracy and reliability of data assets. The role of a data quality manager encompasses a range of responsibilities that are essential for the smooth operation and decision-making processes of a company. From defining data quality standards to implementing data cleansing processes, these professionals play a crucial role in maintaining high-quality data.
Key Responsibilities and Tasks
One of the primary responsibilities of a data quality manager is to establish and enforce data quality standards across various data sources. This involves understanding the different types of data anomalies and inconsistencies that can impact data quality and working towards resolving them. Data profiling, data cleansing, and data governance are integral aspects of a data quality manager's daily tasks.
Best Practices for Data Quality Management
To excel in the role of a data quality manager, it is essential to adopt best practices that ensure data integrity and accuracy. Implementing automated data quality checks, conducting regular data audits, and fostering a data-driven culture within the organization are some of the key best practices that can elevate the effectiveness of data quality management processes.
Skills and Qualifications
Data quality managers need a combination of technical skills, such as proficiency in data analysis tools and database management systems, and soft skills, including communication and problem-solving abilities. A keen eye for detail, a strategic mindset, and the ability to collaborate cross-functionally are also crucial for success in this role.
Challenges in Data Quality Management
Despite advancements in data quality tools and technologies, data quality managers often face challenges such as data silos, inconsistent data definitions, and resistance to change within organizations. Overcoming these challenges requires a proactive approach, strong leadership skills, and a deep understanding of both data management principles and business objectives.
Conclusion
Introduction to Data Quality Management
Data quality management plays a pivotal role in organizations, ensuring that the accuracy, reliability, and integrity of data are maintained at the highest standards. In the realm of information-driven decision-making, the significance of effective data quality management cannot be overstated. It forms the bedrock of organizational operations, guiding strategic initiatives and enhancing overall performance. By focusing on data accuracy, credibility, and consistency, businesses can derive valuable insights to drive informed decisions and stay ahead in a competitive landscape. Understanding the intricacies of data quality management is imperative for IT professionals, cybersecurity experts, and students to navigate the complexities of modern data ecosystems effortlessly.
Defining Data Quality Manager
The Role in Organizations
The role of a data quality manager within organizations is multifaceted and crucial. These professionals are responsible for overseeing the entire data quality process, from data collection to analysis, ensuring that information remains accurate, relevant, and actionable. They act as a bridge between technical teams and upper management, translating complex data concepts into understandable insights that drive strategic decision-making. The data quality manager's key characteristic lies in their ability to harmonize data processes across departments, fostering a culture of data-driven decision-making within the organization. Their unique feature lies in their adeptness at identifying data patterns, resolving inconsistencies, and establishing robust data quality frameworks that elevate operational efficiency.
Importance in Data-Driven Decision-Making
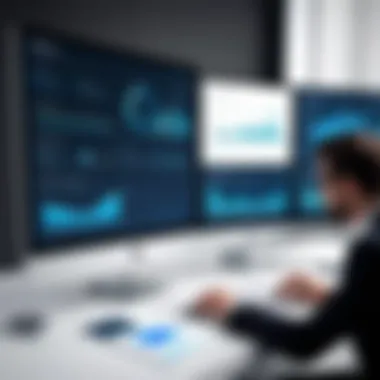
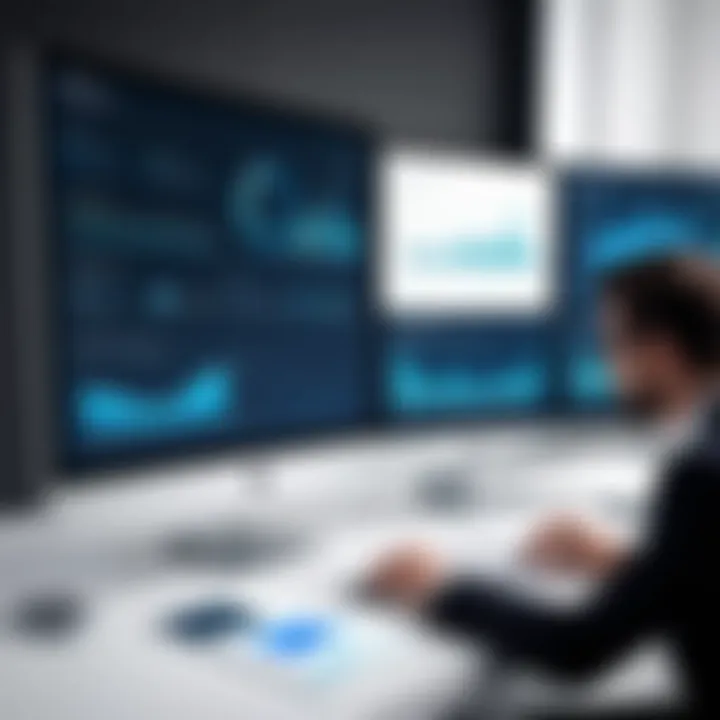
In the landscape of data-driven decision-making, the data quality manager emerges as a linchpin. Their role in ensuring data accuracy and reliability directly influences the quality of decisions made at all organizational levels. By maintaining high data quality standards, these professionals facilitate the extraction of meaningful insights from vast datasets, empowering stakeholders to make informed choices that align with business objectives. The data quality manager's unique feature in this arena is their capacity to enhance the overall data governance structure, enabling organizations to leverage data as a strategic asset effectively. While their contributions are invaluable, challenges such as resource constraints and evolving data compliance regulations continue to pose obstacles in the path of data quality excellence.
Key Responsibilities of a Data Quality Manager
In the realm of data quality management, the role of a Data Quality Manager is paramount. These professionals bear the critical responsibility of ensuring that organizational data maintains high standards of accuracy, integrity, and reliability. Tasked with overseeing various facets of data quality, Data Quality Managers play a crucial role in facilitating informed decision-making processes within organizations. By analyzing, assessing, and monitoring data quality metrics, these individuals contribute significantly to the enhancement of data-driven strategies. The proactive nature of their work involves not only identifying data issues but also implementing solutions to rectify and prevent such discrepancies. By establishing robust data governance frameworks and aligning data quality initiatives with organizational goals, Data Quality Managers ensure that data remains a valuable asset driving business success.
Data Quality Assessment and Profiling
Identifying data issues
One of the fundamental tasks within data quality management is the identification of data issues. This process involves scrutinizing data sets to pinpoint inconsistencies, inaccuracies, or anomalies that may impact the overall quality of information. By recognizing and addressing these issues promptly, Data Quality Managers contribute to the maintenance of data integrity and reliability. The ability to discern patterns in data discrepancies enables these professionals to enact targeted solutions, thus preempting potential setbacks in data analysis processes. However, the intricacies of identifying data issues go beyond mere error detection; it encompasses a proactive approach towards mitigating risks and fostering a culture of data diligence.
Analyzing data quality metrics
Analyzing data quality metrics is a fundamental aspect of data quality assessment and profiling. This process involves evaluating predefined key performance indicators (KPIs) that measure the accuracy, completeness, and consistency of data sets. By delving into the intricacies of data quality metrics, Data Quality Managers gain valuable insights into the overall health of organizational data. This analytical endeavor aids in identifying trends, anomalies, and areas for improvement within data sets, ultimately leading to more informed decision-making processes. However, the analysis of data quality metrics necessitates a keen eye for detail and a comprehensive understanding of data management principles to extract meaningful interpretations from diverse data sources.
Data Quality Monitoring and Reporting
Establishing data quality KPIs
To maintain a high standard of data quality, Data Quality Managers must establish robust data quality KPIs. These key performance indicators serve as benchmarks for evaluating and monitoring the accuracy, timeliness, and completeness of organizational data. By setting clear and measurable KPIs, Data Quality Managers enable proactive tracking of data quality trends and deviations. This foresight allows organizations to address data issues promptly and implement corrective measures efficiently. However, the establishment of data quality KPIs requires a strategic approach that aligns with organizational objectives and emphasizes continuous improvement in data quality practices.
Generating data quality reports
The generation of data quality reports is a cornerstone in the arsenal of Data Quality Managers. These reports provide comprehensive insights into the status of data quality within an organization, highlighting areas of strength and opportunities for enhancement. By compiling and presenting data quality findings in a structured manner, Data Quality Managers facilitate informed decision-making processes for key stakeholders. The creation of data quality reports requires a meticulous attention to detail and a proficiency in data visualization techniques to convey complex information effectively. Additionally, the ability to communicate data quality trends and recommendations in a clear and concise manner is essential for ensuring that data quality insights are actionable and impactful.
Data Governance and Strategy
Implementing data governance frameworks
Implementing robust data governance frameworks is a cornerstone of effective data quality management. Data Quality Managers are tasked with defining and implementing policies, procedures, and controls that govern data usage, security, and integrity within an organization. By establishing data governance frameworks, these professionals enhance transparency, accountability, and compliance regarding data practices. The implementation of data governance frameworks requires a multidisciplinary approach that integrates legal, technical, and operational perspectives to create a cohesive framework that safeguards data assets and fosters data-driven decision-making.
Aligning data quality initiatives with organizational goals
Aligning data quality initiatives with organizational goals is a strategic imperative for Data Quality Managers. By ensuring that data quality endeavors complement and contribute to the achievement of broader organizational objectives, these professionals solidify the value of data as a strategic asset. The alignment of data quality initiatives with organizational goals requires a holistic understanding of business objectives, stakeholder requirements, and industry regulations. By forging strong connections between data quality activities and strategic outcomes, Data Quality Managers empower organizations to leverage data effectively for competitive advantage and operational excellence.
Skills and Qualifications Required
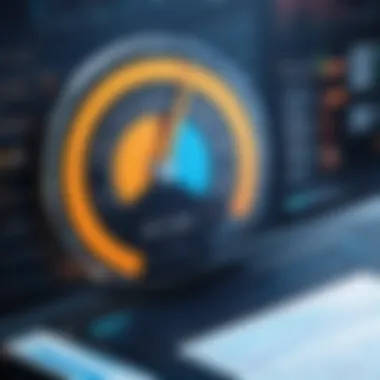
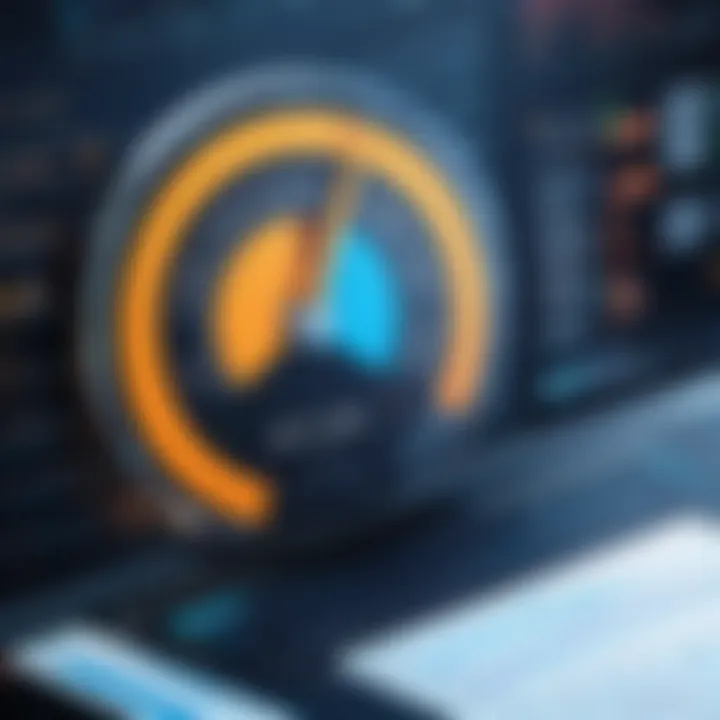
In delving into the realm of a Data Quality Manager, possessing the necessary Skills and Qualifications is paramount. These attributes serve as the foundation upon which a professional in this field can thrive and excel. Technical Proficiency stands tall as a primary requirement, encompassing an in-depth understanding of data management tools and data modeling. Analytical Skills entail the capability to decipher intricate data patterns and apply critical thinking in problem-solving. Furthermore, Communication and Collaboration skills play a pivotal role in facilitating effective stakeholder engagement and ensuring clear articulation of data quality concepts.
Technical Proficiency
Knowledge of data management tools
Within the domain of data quality management, the comprehension of data management tools holds immense significance. It serves as a catalyst in streamlining processes, optimizing data integrity, and enhancing organizational efficiency. The prowess in utilizing data management tools allows Data Quality Managers to orchestrate data flows, implement robust data governance frameworks, and execute data quality assessments with precision. Embracing knowledge of data management tools empowers professionals in this role to drive informed decision-making and steer data-centric initiatives with finesse.
Understanding of data modeling
Equipped with a profound understanding of data modeling, Data Quality Managers can navigate the intricacies of data structures, relationships, and dependencies. This expertise is instrumental in designing data models that encapsulate business requirements, ensure data accuracy, and support organizational objectives. By grasping the nuances of data modeling, professionals in this position can harmonize disparate data sources, construct data architectures that resonate with operational needs, and foster a data-driven culture within the organization.
Analytical Skills
Ability to interpret data patterns
The ability to interpret data patterns empowers Data Quality Managers to unravel insights buried within vast datasets. By discerning data patterns, professionals can uncover anomalies, trends, and correlations that influence decision-making processes. This skill aids in identifying data discrepancies, enhancing data quality metrics, and refining data quality enhancement strategies. With a keen eye for data patterns, Data Quality Managers can proactively address data inconsistencies, fortify data validation processes, and fortify the organizational data ecosystem.
Critical thinking in problem-solving
Critical thinking plays a pivotal role in the problem-solving repertoire of a Data Quality Manager. By critically analyzing data challenges, professionals in this role can devise innovative solutions, overcome data hurdles, and drive continuous improvement in data quality standards. The application of critical thinking fosters a proactive approach to mitigating data quality issues, augments data remediation strategies, and propels data quality frameworks towards operational excellence.
Communication and Collaboration
Effective stakeholder engagement
The ability to engage stakeholders effectively is a cornerstone skill for Data Quality Managers. By nurturing stakeholder relationships, professionals can garner support for data quality initiatives, elicit valuable feedback, and align data quality objectives with organizational imperatives. Effective stakeholder engagement fosters synergy between diverse teams, fosters a collaborative data governance ecosystem, and cultivates a shared understanding of data quality objectives and outcomes.
Clear articulation of data quality concepts
Clear articulation of data quality concepts is essential for conveying complex ideas in a succinct and comprehensible manner. Data Quality Managers must adeptly communicate data quality principles, processes, and outcomes to diverse stakeholders, ensuring clarity and transparency in data-related discussions. By articulating data quality concepts clearly, professionals can eliminate ambiguity, foster organizational buy-in for data quality initiatives, and drive a culture of data-centric decision-making throughout the organization.
Challenges Faced by Data Quality Managers
In this section, we delve deep into the pivotal challenges encountered by data quality managers, shedding light on the crucial aspects that demand their attention. A data quality manager's role is intricate and multifaceted, with challenges that range from data fragmentation to ensuring seamless data integration. By understanding and addressing these challenges, data quality managers play a crucial role in maintaining the accuracy, integrity, and reliability of organizational data.
Data Silos and Integration
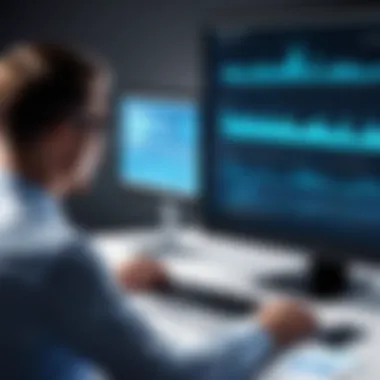
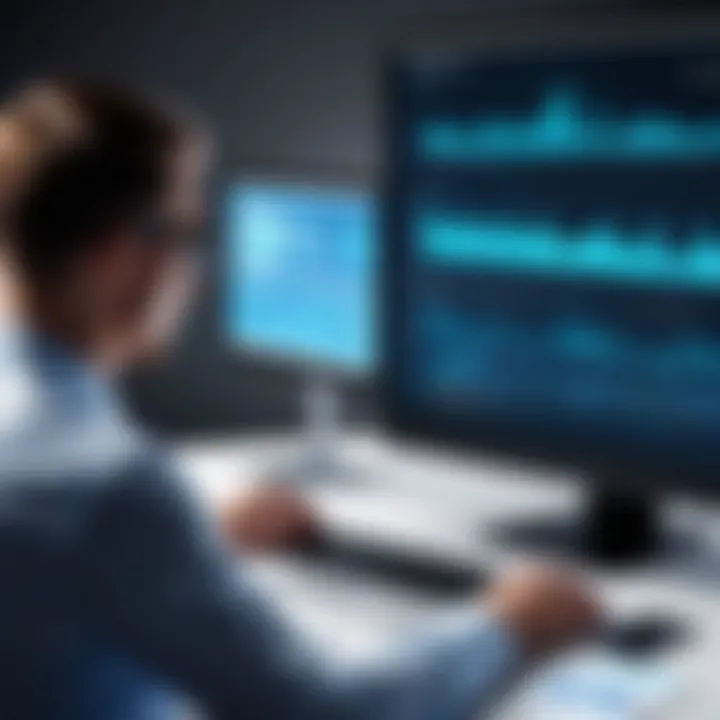
Overcoming data fragmentation
When it comes to overcoming data fragmentation, the key lies in consolidating disparate data sources into a unified ecosystem. This consolidation allows for a comprehensive view of organizational data, minimizing errors and redundancies. By integrating data silos, data quality managers can enhance data quality metrics and streamline data processes. The unique feature of overcoming data fragmentation is its ability to holistically improve data quality, paving the way for more informed decision-making within the organization.
Ensuring seamless data integration
Seamless data integration is essential for facilitating smooth data flows and interconnections across different systems and platforms. By ensuring seamless data integration, data quality managers promote data consistency and accuracy throughout the organization. The key characteristic of seamless data integration is its capacity to harmonize diverse data sets, enabling efficient data exchange and collaboration. While the process of seamless data integration may pose technical challenges, its advantages in enhancing data quality and operational efficiency make it a popular choice for organizations striving for data optimization.
Managing Data Privacy and Compliance
Adhering to regulatory standards
Adhering to regulatory standards is a critical aspect of data quality management, ensuring that data processing activities comply with legal requirements and industry guidelines. By aligning data practices with regulations such as GDPR and HIPAA, data quality managers uphold data integrity and protect sensitive information. The key characteristic of adhering to regulatory standards is its emphasis on data governance frameworks and privacy measures, safeguarding organizational data from breaches and non-compliance issues. While adhering to regulatory standards may require investment in compliance tools and training, the advantages of maintaining data legality and trustworthiness justify its significance in data quality management.
Protecting sensitive data
Protecting sensitive data involves implementing robust security measures to prevent unauthorized access and data breaches. By encrypting sensitive information and restricting data access based on security protocols, data quality managers safeguard confidential data from internal and external threats. The key characteristic of protecting sensitive data is its proactive approach to data security, reducing the risk of data leaks and confidentiality breaches. While the process of protecting sensitive data requires ongoing monitoring and updates to security protocols, its advantages in preserving data confidentiality and organizational reputation make it an essential priority in data quality management.
Future Trends in Data Quality Management
In the realm of data quality management, staying abreast of future trends is paramount to ensuring relevance and efficacy in organizational data practices. The evolution of AI and Machine Learning has revolutionized data quality processes, offering automation that enhances efficiency and accuracy, ultimately reshaping predictive analytics for informed decision-making. The incorporation of these advanced technologies introduces a paradigm shift in how data quality is approached, paving the way for more streamlined operations and proactive insights.
AI and Machine Learning in Data Quality
Automating data quality processes
The automation of data quality processes signifies a pivotal advancement in managing large datasets effectively. By leveraging AI algorithms, routine data cleansing tasks are executed with precision and speed, reducing manual intervention and potential errors. The key benefit of automating these processes lies in its ability to scale operations effortlessly, freeing up resources for more strategic initiatives. However, the challenge lies in ensuring the algorithms are trained correctly to avoid biases and inaccuracies, underscoring the importance of human oversight in algorithmic decision-making.
Enhancing predictive analytics
Enhancing predictive analytics through AI and Machine Learning empowers organizations to forecast trends and behaviors with enhanced accuracy. By dissecting vast datasets and identifying patterns, predictive analytics aids in proactive decision-making, mitigating risks, and capitalizing on opportunities. The key characteristic of this capability is its ability to process complex data landscapes swiftly, uncovering invaluable insights that drive performance and competitive advantage. Nevertheless, the reliance on algorithms presents the risk of reinforcing existing biases or overlooking nuanced factors, necessitating continuous refinement and validation processes.
Emphasis on Data Ethics and Transparency
As data becomes increasingly ubiquitous, the emphasis on ethical data practices and transparency is indispensable in fostering trust and accountability. Promoting ethical data practices involves establishing frameworks for responsible data usage and ensuring compliance with regulatory standards. The key advantage of prioritizing ethics in data management is the cultivation of a responsible data culture that upholds integrity and builds credibility. However, the challenge lies in balancing ethical considerations with operational demands, aligning organizational objectives with ethical imperatives to maintain stakeholder trust.
Promoting ethical data practices
Promoting ethical data practices involves setting clear guidelines for data collection, storage, and usage to safeguard privacy and mitigate risks. By adhering to ethical principles, organizations can elevate their reputation, attract customers, and avert potential legal repercussions. The unique feature of ethical data practices is its ability to engender consumer trust and enhance data stewardship, fostering long-term relationships and sustainability. Yet, the adherence to stringent ethical standards may entail additional resources and constraints, necessitating a delicate balance between compliance and innovation.
Ensuring data transparency
Ensuring data transparency signifies making data processes and practices open, accessible, and understandable to stakeholders. By promoting transparency, organizations demonstrate accountability and integrity in handling data, fostering increased trust and engagement. The key characteristic of data transparency is its potential to enhance customer loyalty, regulatory compliance, and operational efficiency, driving organizational growth and resilience. However, achieving comprehensive data transparency requires meticulous documentation, effective communication, and proactive engagement with stakeholders, presenting a resource-intensive yet rewarding endeavor.