Key Trends Transforming the Data Analytics Landscape
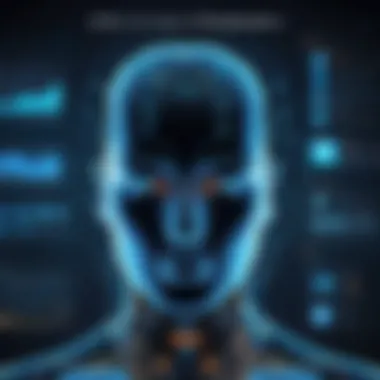
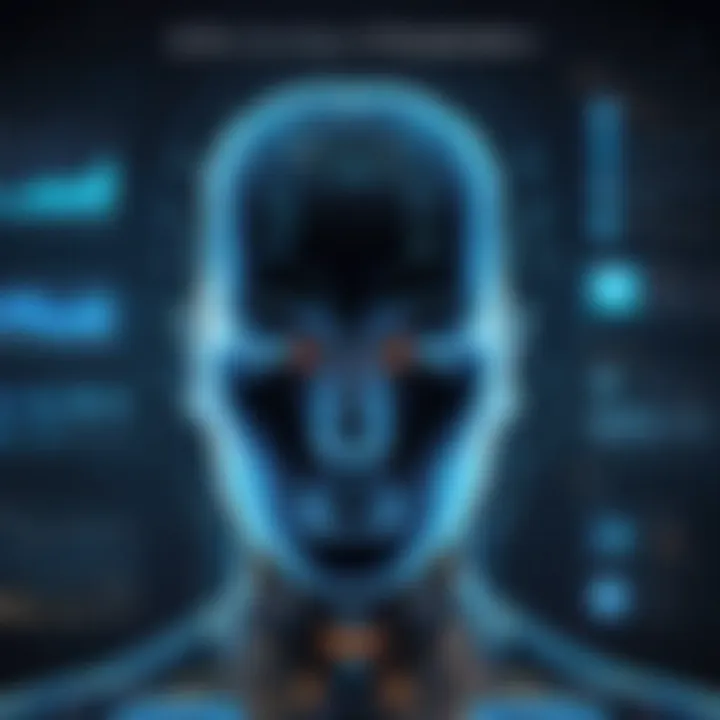
Intro
The data analytics landscape is shifting faster than a New York minute, and keeping up with the trends is not just a good idea; it’s essential. As organizations increasingly harness data for decision-making, understanding what’s on the horizon in this field is paramount. This narrative dives deep into the nuances defining the data analytics industry today, revealing layers of emergent trends that are well worth exploring.
Understanding Key Trends Affecting Data Analytics
Integration of Artificial Intelligence
Artificial Intelligence has firmly planted its flag in the realm of data analytics. It’s not merely a tool anymore; it’s becoming the backbone of data-driven insights. With machine learning algorithms learning from data patterns, businesses can predict outcomes with a distinguished accuracy. This allows organizations to make informed choices, shifting from reactive to proactive strategies. What’s more, tools like Tableau and Microsoft Power BI are embedding AI capabilities to improve data visualization, making the analysis more intuitive than ever before.
Data Privacy Concerns
As the saying goes, too many cooks spoil the broth, and the same can be said about data. With increasing volumes of data comes an amplified responsibility to protect it. The implementation of regulations like GDPR and CCPA illustrates the escalating importance of data privacy. Customers now demand transparency, pushing organizations to rethink their data handling practices. It’s essential for businesses not just to comply but to create a strong culture of data ethics, ensuring that trust remains intact between them and their clients.
Rise of Self-Service Analytics
Gone are the days when data was locked away in high walls, accessible only to a select few. Self-service analytics is democratizing data access. Employees across various departments can interact with data and draw insights without the need for constant IT support. Companies like Google have made strides with tools such as Looker, allowing users to create their own reports and dashboards. This trend fosters a data-driven culture, empowering all team members to make decisions backed by analytics.
Advanced Visualization Tools
A picture is worth a thousand words, and in data analytics, that holds true. Advanced visualization tools are changing the game. By presenting complex datasets in a clear and appealing manner, organizations can communicate insights more effectively. Tools like D3.js or Microsoft’s Power BI provide rich visual representations of data trends, making it easier to grasp critical insights at a glance. As more decision-makers recognize the importance of these tools, investing in their use has become a priority.
The Impact of Big Data Technologies
Big data technologies pave the way for uncovering insights that were previously obscured. Technologies such as Hadoop and Spark can process extensive amounts of data in real time, giving organizations the capability to react to trends dynamically. As consumers become more aware, businesses must adapt quickly to their evolving needs. This capability not only drives efficiency but can also enhance customer satisfaction by delivering a tailored experience.
The End
As we traverse through the trends reshaping the data analytics industry, one thread remains constant: organizations must adapt swiftly and intelligently. While AI integration, data privacy, self-service tools, and advanced visualization are only a handful of the many factors at play, embracing them is crucial for success. Stakeholders who understand these emerging trends can position themselves ahead of the curve in this fast-paced industry. The future of data-driven decision-making looks bright, and staying informed will offer competitive advantages to those who dare to leverage these insights.
Prelude to Data Analytics Industry Trends
In a world that’s increasingly driven by information, the data analytics industry stands at the forefront of transforming raw data into actionable insights. This section aims to peel back the layers and uncover the emerging trends reshaping this dynamic field. Knowing these trends isn’t just for the data aficionados; they hold significant relevance for everyone in the tech space, from IT professionals to budding analysts.
Understanding Data Analytics
Data analytics refers to the systematic computational analysis of data, which is essential in deciphering patterns and extracting meaningful information. Some would argue that data analytics is the new oil in today’s digital landscape. Think about it: every click, every purchase, and every interaction online creates data. Understanding how to analyze this data effectively can lead to better decision-making, optimized business processes, and improved customer experiences.
Today, the landscape has expanded to include various branches like predictive analytics, descriptive analytics, and prescriptive analytics, each offering unique perspectives and capabilities. For instance, while descriptive analytics provides insights into historical data trends, predictive analytics helps forecast future trends based on that historical data. This evolution is crucial as organizations look for ways to maintain competitiveness in an ever-changing market.
Why Trends Matter
Keeping an eye on the prevailing trends in data analytics is similar to having a compass in a dense forest; it helps guide organizations through the labyrinth of information. As stakeholders increasingly rely on data-driven decisions, understanding the underlying trends becomes imperative.
- Fosters Innovation: The data landscape is marked by rapid advancements, especially in technologies like artificial intelligence and big data. Companies that stay updated on these trends can leverage them to innovate their products and services effectively.
- Enhances Competitive Advantage: Organizations that systematically adopt notable trends can outpace their competitors. For instance, embracing real-time analytics can lead to quicker response times in customer interactions, fostering loyalty and satisfaction.
- Navigates Regulatory Challenges: As data privacy and security concerns become more pressing, keeping up with trends helps organizations not only comply with regulations but do so efficiently.
"The best way to predict the future is to create it." — Peter Drucker.
Being aware of trends allows businesses to take proactive steps rather than reactive ones, positioning them favorably within their respective industries.
Overall, the intersection of technology and analytics is a burgeoning field that demands our attention. Understanding emerging trends will empower professionals to harness the potential of data, ultimately shaping a data-centric future.
Artificial Intelligence and Machine Learning
The integration of Artificial Intelligence (AI) and Machine Learning (ML) significantly redefines the landscape of the data analytics industry. These technologies are not merely enhancements; they are becoming the backbone of how organizations analyze data, extract valuable insights, and make informed decisions. The benefits are numerous, including increased efficiency, improved accuracy, and the ability to handle vast quantities of data in real time. As we see more organizations turning to data-driven strategies, the deployment of AI and ML is crucial for staying competitive.
Integration of AI in Analytics
Integrating AI into analytics transforms how data is processed and understood. Traditional analytics often relies on manual processes, which can be time-consuming and prone to errors. In contrast, AI-powered analytics automates these tasks, speeding up the process and allowing data scientists to focus on interpretation rather than data preparation.
AI technologies can sift through large datasets quickly, revealing patterns that may not be immediately visible to human analysts. For instance, consider retail companies that utilize AI to analyze customer purchase history. By identifying buying trends, these businesses can better tailor their marketing strategies. Additionally, AI systems continuously learn and evolve from new data, improving their predictive capabilities.
Some of the critical considerations when integrating AI in analytics include:
- Data Quality: AI needs high-quality, relevant data to function effectively. Poor data can lead to skewed insights.
- Infrastructure: Organizations need to have the necessary infrastructure. Cloud-based solutions often provide the flexibility required for AI.
- Skill Requirements: It’s essential for teams to have the necessary skills in AI and analytics tools. Upskilling or hiring specialists can bridge this gap.
Predictive Analytics Through Machine Learning
Machine Learning takes predictive analytics to a whole new level. By using algorithms to analyze historical data, ML can forecast future outcomes with impressive accuracy. This capability is particularly valuable in sectors like finance, healthcare, and marketing, where data-driven decisions can lead to significant competitive advantages.
In finance, for example, ML algorithms can assess credit risk by analyzing past credit data along with social media patterns, enabling financial institutions to make more informed lending decisions. Similarly, in healthcare, predictive models can identify which patients are at risk of developing certain conditions, allowing for early intervention and better patient outcomes.
To effectively implement predictive analytics using ML, companies should:
- Define clear use cases: Understanding where predictive analytics can add value is crucial.
- Choose the right models: Not every model fits all problems; selecting the right one involves careful consideration of the data and desired outcomes.
- Monitor and refine: Machine learning is not a "set it and forget it" solution. Continuous monitoring ensures that models maintain their accuracy and relevance over time.
"The most significant trends in data analytics come not just from tools, but from the informed application of these advanced technologies in real-world scenarios."
As organizations adopt AI and ML into their analytics processes, they not only gain insights but also enhance their capacity to solve complex problems. In the evolving realm of data analytics, these technologies stand as pivotal elements setting the standard for industry practices.
Data Privacy and Security
Data privacy and security have come to the forefront of discussions in the analytics realm. With a mine of personal data being analyzed for insights, safeguarding this information is crucial. Organizations that overlook data privacy could face significant repercussions, ranging from hefty fines to eroded trust among consumers. Cultivating a culture that prioritizes data security not only protects sensitive information but also fuels innovation by giving stakeholders a sense of safety and reliability.
In the fast-evolving landscape of the data analytics industry, maintaining robust data privacy and security measures can prevent potential breaches, ensuring that businesses can conduct their operations with confidence. Choosing to navigate the complexities of data privacy shows a company's commitment to responsible data use, aligning it with global standards and ethical practices.
Regulatory Landscape Impacting Data Analytics
Regulations surrounding data usage are intensifying and changing rapidly. Laws like the General Data Protection Regulation (GDPR) in Europe and the California Consumer Privacy Act (CCPA) in the USA dictate how personal data should be handled. Organizations need to remain vigilant and compliant to these regulations, as non-compliance can lead to serious legal issues.
- Compliance protocols: Companies must have well-defined protocols in place to comply with regulations, including data mapping and consent management.
- Data subject rights: Laws like GDPR empower users to request access and deletion of their data, necessitating firms to construct systems to address these requests efficiently.
- International considerations: For businesses operating in multiple regions, understanding local regulations is key, as laws may differ significantly.
"Failure to comply isn’t optional; it’s a recipe for disaster."
Best Practices for Data Protection
Implementing best practices in data protection not only mitigates risks but also establishes a framework that allows organizations to operate effectively within the regulatory landscape. Here are some essential strategies:

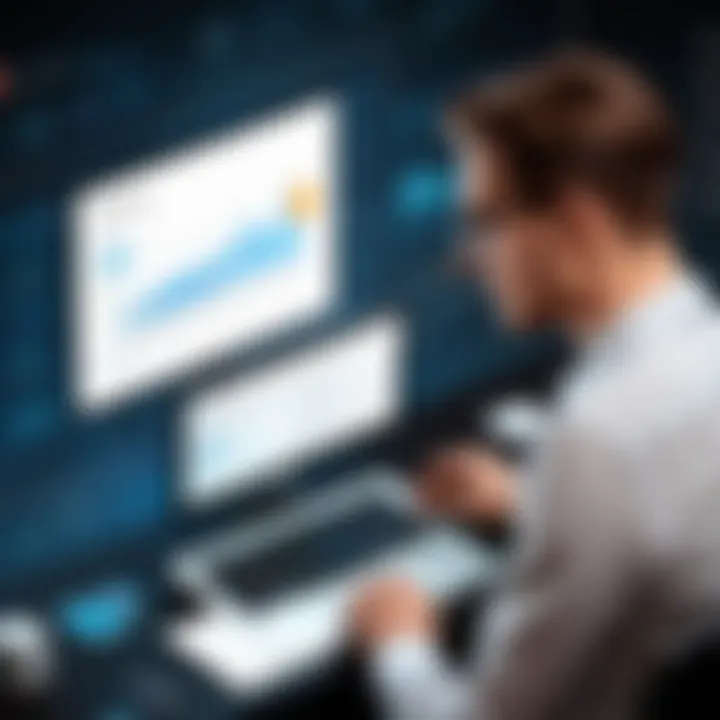
- Data encryption: Encrypt data at rest and in transit to protect it from unauthorized access.
- Access controls: Limit data access only to authorized personnel. Employ role-based access control to ensure that employees access only the data necessary for their roles.
- Training and awareness: Conduct regular training sessions for staff to foster a culture of security awareness; employees often represent the weakest link in data security.
- Regular audits: Carry out frequent security audits and assessments to identify vulnerabilities in your data handling processes.
- Incident response plan: Develop a clear data breach response plan that outlines immediate actions and communication strategies.
Adopting these practices not only secures data effectively but also promotes a proactive approach to the ever-changing challenges associated with data privacy. Organizations that take data privacy seriously position themselves as trustworthy entities in the eyes of customers, which is crucial in today’s data-driven business environment.
Self-Service Analytics
In today's data-driven landscape, self-service analytics plays an essential role in enabling organizations to make informed decisions without heavy reliance on technical teams. This paradigm shift empowers employees across various roles to dig into data on their own terms. The growing demand for quick insights, paired with the desire for data democratization, has made self-service analytics a hot topic among data professionals and organizational leaders alike.
Empowering Non-Technical Users
Self-service analytics creates a bridge between complex data sets and non-technical users, leveling the playing field in data accessibility. The democratization of analytics means that someone like a marketing specialist or HR professional can access and interpret data without needing a degree in statistics or computer science.
Consider a marketing team that needs to assess the performance of a recent campaign. Instead of funneling requests through the data science team, they can utilize self-service tools to generate reports, visualize trends, and understand customer behavior. This hands-on interaction enables faster decision-making and fosters a culture of data-driven thinking throughout the organization.
Moreover, the convenience of these platforms means that employees can experiment with data at their own pace. They can ask questions, test hypotheses, or even learn new skills without waiting for assistance, significantly boosting productivity. As the adage goes, "Give a man a fish, and you feed him for a day; teach a man to fish, and you feed him for a lifetime."
Tools and Resources for Self-Service Analytics
To facilitate self-service analytics, numerous tools have emerged, each designed to make data exploration intuitive and accessible. These platforms allow users to create dashboards, run analyses, and generate visualizations effortlessly. Some notable tools in this space include:
- Tableau: Renowned for its powerful visualization capabilities, Tableau helps users create shareable dashboards effortlessly, allowing non-technical professionals to gain insights from data.
- Power BI: From Microsoft, this tool integrates seamlessly within the Microsoft ecosystem and is favored for its user-friendly interface, empowering users to transform raw data into compelling visuals.
- Looker: Part of the Google Cloud Platform, Looker allows users to explore and understand their data using a customizable modeling language.
- Domo: This platform consolidates data from various sources into one dashboard, offering real-time insights that foster collaborative decision-making.
Furthermore, many organizations are opting for training programs aimed at enhancing the analytical skills of their employees. Online platforms like Coursera or Udemy provide courses focused on data analytics that can bridge knowledge gaps.
Adopting self-service analytics does, however, come with its own set of challenges that need addressing. Companies must emphasize data governance, ensuring that the right data is accessible and users are trained properly to avoid misinterpretations.
"The key to successful self-service analytics is not just in providing the tools but also in fostering a culture that recognizes data as a critical asset for decision-making."
In summary, the rise of self-service analytics signifies a substantial shift in how organizations approach data. By empowering non-technical users and providing the right tools, companies can enhance their analytical capabilities, making them more agile, innovative, and prepared for the complexities of today’s marketplace.
Data Visualization Techniques
In today's complex data landscape, the way we visualize data has become increasingly paramount. Effective data visualization techniques have transformed raw data into meaningful insights that can steer strategic decisions. Creating a clear graphical representation of information can make patterns, trends, and correlations much easier to spot than sifting through spreadsheets.
Visualization can cross boundaries where simple numbers fall flat, making it an essential component in presenting data analytics findings. This helps not only to convey information quickly but also to engage stakeholders who may not have the time or capability to digest large amounts of raw data.
Importance of Effective Visualization
Understanding the importance of effective visualization is crucial, particularly in a world where data volumes grow exponentially. Here are key points to consider:
- Clarity and Efficiency: A well-designed chart or graph can convey substantial information at a glance. For instance, while two pages of text might tell a story, a single pie chart could illustrate the same idea in seconds. This efficiency is vital for professionals who often have limited time to make informed decisions.
- Engagement: Visuals capture attention. An infographic or a vivid dashboard can spark interest and provoke discussions. Engaging presentations can transform a mundane boardroom report into a lively dialogue.
- Storytelling: Visualization isn’t just about aesthetics; it’s about telling a story. Data becomes compelling when it’s laid out in a way that highlights key insights. Think of how infographics distill complex information into digestible narratives that can be shared across various platforms.
- Accessibility: Not all stakeholders have deep analytical backgrounds. Effective visuals bridge knowledge gaps, making it easier for non-technical users to understand insights and contribute to the discussion.
"The greatest value of a picture is when it forces us to notice what we never expected to see." — John W. Tukey
Emerging Visualization Tools
The landscape of data visualization tools is evolving rapidly, providing new capabilities that enable better data storytelling. Here are some noteworthy tools making waves in the industry:
- Tableau: Known for its robust dashboard capabilities, Tableau allows users to create interactive visualizations that help cut through complexity.
- Power BI: This Microsoft tool integrates seamlessly with other Microsoft products, making it a popular choice in enterprise environments for quick insights and extensive reporting options.
- D3.js: For the hands-on user, D3.js offers dynamic, flexible data-driven documents that allow for fully customized visualizations. This is especially useful for those with coding experience, as it can create stunning, bespoke visuals.
- Google Data Studio: This tool enhances collaboration by allowing teams to create shareable reports that connect to various data sources, making analytics accessible for everyone.
- QlikView: With its associative data model, QlikView enables users to explore data more freely, making it easier to discover insights within massive datasets.
The importance of these tools cannot be understated. They empower organizations to leverage data in innovative ways, enhancing the decision-making process by making complex statistics more understandable. As data analytics continues to evolve, staying updated with emerging visualization tools will be essential for IT professionals and analysts alike.
Big Data Transformation
In the ever-evolving landscape of data analytics, the metamorphosis brought about by big data is no small potatoes. The sheer volume, variety, and velocity of data generated today surpasses anything we’ve seen before, creating an immense opportunity for businesses. This transformation isn't just a fad; it’s reshaping the way decisions are made across industries and sectors.
The Role of Big Data in Analytics
Big data serves as the backbone of modern analytics. Organizations are investing heavily in technologies that allow them to leverage this abundant data to glean valuable insights.
- Enhanced Decision-Making: Data-driven decisions are taking the front seat, steering companies towards greater operational efficiency. By harnessing big data, firms can shift from gut-feel strategies to decisions backed by concrete data, leading to smarter outcomes.
- Predictive Capabilities: The predictive power derived from analyzing large datasets allows organizations to foresee trends and behaviors, significantly increasing the precision of forecasting. This capability is vital in sectors such as retail and healthcare where consumer behavior can be erratic.
- Customization and Personalization: Companies can tailor their services to fit individual customer preferences. By understanding customer interactions with various channels, businesses can innovate their approach to meet specific needs. This personalized touch can foster brand loyalty and trust among consumers.
However, integrating big data into an organization isn't without its challenges. It demands significant investment in data infrastructure, skilled professionals, and sophisticated analytic tools. Businesses that embrace this challenge are often the ones who stay ahead of the curve.
Handling Volume, Variety, and Velocity
As businesses strive to tap into big data, understanding the three V's—volume, variety, and velocity—becomes crucial.
- Volume: The amount of data produced is staggering. Every minute, countless transactions occur, creating a torrent of information. Businesses must find ways to manage, store, and process this data effectively. Solutions such as cloud databases are a popular choice to manage significant volumes without overwhelming internal systems.
- Variety: Data comes in different formats, from structured data like databases to unstructured data such as text, images, and video. This variety adds complexity to analytics. Modern analytics tools can now handle these diverse datasets, allowing businesses to extract insights from mixed information sources.
- Velocity: The speed at which new data is generated and processed is staggering. Real-time data processing is no longer the exception, but rather an expectation. Companies use techniques like stream processing to analyze data as it arrives, keeping their insights timely and relevant.
"In a world where data is dynamically changing, those who can keep up with the pace of information are the ones who will lead the pack."
Understanding these elements enables organizations to cultivate a more resilient data analytics strategy, transforming vast amounts of data into actionable insights. Companies that can skillfully navigate these challenges will position themselves favorably in the competitive landscape, paving the way to innovation and growth.
Real-Time Analytics and Insights
In today’s fast-paced world, the ability to analyze data in real-time is becoming a necessity rather than a luxury. With businesses facing a mountain of data from various sources, understanding the importance of real-time analytics can’t be overstated. These insights not only offer a timely glimpse into organizational performance but also fuel strategic decisions that can make or break a company in competitive markets.
The benefits of real-time analytics are manifold. Firstly, organizations can respond swiftly to changing market conditions or customer needs. When something important occurs, whether it’s a significant drop in sales or an uptick in customer queries, businesses leveraging real-time insights can take immediate action. This agility is what sets successful organizations apart in a landscape where timelines are tighter than ever.
Moreover, real-time analytics enables enhanced decision-making. Traditional analysis often relies on historical data, which can lag and give a distorted view of current scenarios. In contrast, real-time data provides insights as events unfold. This capability allows decision-makers to pivot their strategies based on the most current information, ensuring that plans are grounded in reality rather than assumptions.
A good example to consider is in the realm of e-commerce. Companies like Amazon use real-time analytics to track customer behavior on their platform. Whether it’s identifying a drop-off during the checkout process or monitoring which products receive the most clicks, this data informs everything from marketing to logistics, resulting in a significantly improved user experience and increased conversion rates.
"In the data-driven era, speed isn't just a bonus; it's a fundamental requirement for survival."
Need for Speed in Data Processing
The velocity at which data is processed is crucial in the realm of real-time analytics. Traditional methods often involve batch processing, where data collected over a period is analyzed as a bulk. However, with the increasing amount of information flowing in, batch processing can no longer keep up. Customers don’t wait for reports to be generated every month—they want answers at their fingertips right now.
Speed in data processing allows organizations to act on insights as they come in. Whether it’s responding to customer inquiries, adjusting pricing strategies based on demand fluctuations, or even detecting security threats in cybersecurity, having a rapid response can prevent long-term impacts.
The technological advancements have made it possible for even smaller organizations to adopt real-time analytics capabilities. Tools such as Apache Kafka or Google BigQuery allow firms to process data streams as they flow in, making it possible to glean insights almost instantaneously.
Best Practices for Achieving Speed in Data Processing
- Invest in the Right Technology: Choose tools and platforms designed for real-time processing rather than simply relying on traditional data warehousing solutions.
- Prioritize Data Quality: Slow speed can often be a result of dealing with poor-quality data. Ensure high standards of data collection and management to make real-time analytics smoother.
- Train Your Team: Engaging non-technical users and training existing staff on the importance and use of real-time analytics can enhance the organization’s overall efficiency.
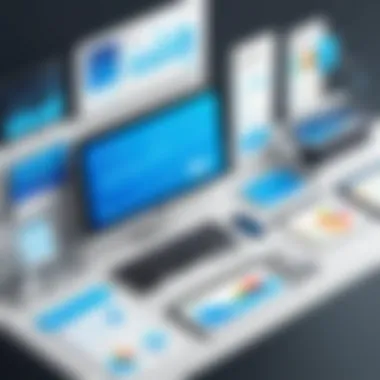
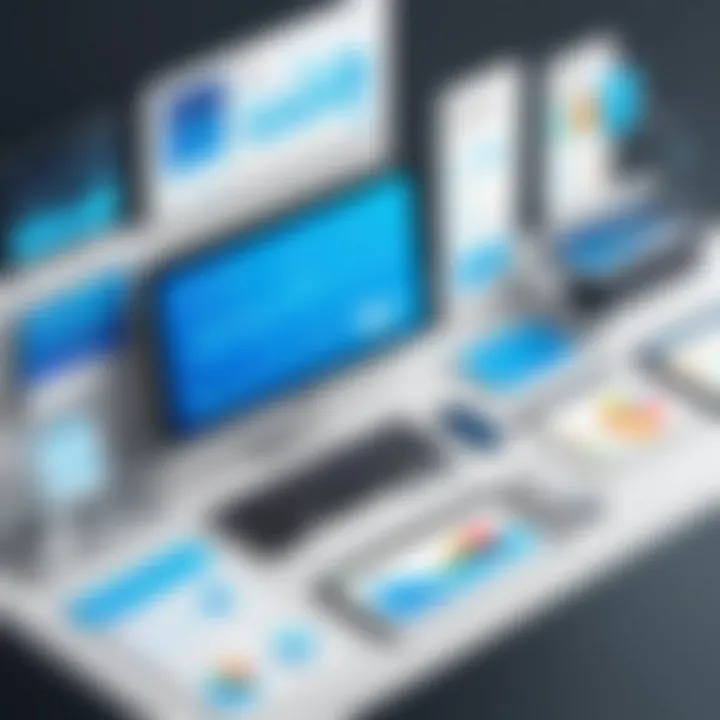
Applications of Real-Time Analytics
Real-time analytics can be applied in numerous fields, each with unique requirements. The utilization of immediate data insights can ultimately transform how sectors operate, adding efficiency, improving customer service, and enabling smart decision-making.
- Healthcare: Hospitals and clinics now increasingly rely on real-time analytics to monitor patient vitals, track equipment availability, and manage patient flow. The implications are lifesaving; immediate alerts can be generated if a patient’s condition worsens, leading to quicker interventions.
- Finance: In banking, real-time fraud detection systems employ machine learning algorithms that analyze transaction patterns instantly, flagging any irregular behavior that could indicate fraud.
- Telecommunications: Network providers analyze real-time usage statistics to optimize bandwidth distribution and offer seamless service to users. Immediate feedback allows organizations to scale their resources dynamically.
- Manufacturing: Real-time data from production lines can help optimize processes and identify bottlenecks. By having immediate visibility into operations, managers can troubleshoot production issues as they arise, reducing downtime and improving efficiency.
- Retail: Retailers know that inventory management is critical. Real-time analytics can help track stock levels and predict restocking needs based on current sales trends, thus avoiding stockouts and overstock situations.
In wrapping up, the significance of real-time analytics can't be ignored in this dynamic data landscape. Organizations must adapt to keep pace, or they risk falling behind. The capacity to act on insights in an uninterrupted flow will dictate the winners and losers in the analytics game.
Industry-Specific Analytics Adoption
In an age where data reigns supreme, focusing on industry-specific analytics adoption is crucial. Each sector has its own unique challenges and opportunities, making a one-size-fits-all approach to analytics less effective. By tailoring analytics solutions to the idiosyncrasies of specific industries, organizations can derive far greater value from their data.
Trends in Healthcare Analytics
Healthcare analytics has emerged as a game changer in improving patient care and operational efficiency. Hospitals and health systems are increasingly using data insights to streamline processes, predict patient admissions, and even identify potential outbreaks through pattern recognition. For instance, with the advent of electronic health records, healthcare providers can dig deeper into patient data.
Analysts can now leverage these vast data troves to:
- Enhance patient outcomes by identifying risk factors before they escalate.
- Reduce costs by optimizing resource allocation and operational workflows.
- Improve regulatory compliance, ensuring that all health service providers meet legal and ethical standards with precision.
Moreover, predictive analytics plays a significant role in healthcare. Hospitals can anticipate the demand for specific services, enabling them to stock necessary supplies and schedule staff accurately. In a nutshell, this trend allows healthcare providers to be proactive rather than reactive, ultimately enhancing overall patient care.
Analytics in Finance and Banking
The finance and banking sector also reaps benefits from data analytics by boosting security, understanding customer preferences, and streamlining operations. As financial institutions look to stay ahead, the integration of analytics is becoming indispensable. By harnessing vast amounts of transactional data, banks can analyze customer behaviors and preferences, leading to more personalized financial products.
Key areas where analytics shines in finance include:
- Fraud detection: Advanced analytics can scrutinize transactions in real-time, flagging unusual patterns that may indicate fraudulent activity.
- Risk management: Institutions can better assess credit risks and analyze market fluctuations to make informed investment decisions.
- Regulatory reporting: Compliance remains a top priority, and analytics simplifies the process of gathering necessary data for reporting to regulatory authorities.
In summary, the importance of adopting analytics tailored for specific industries cannot be overstated. As different sectors face distinct challenges, responding with bespoke analytics approaches can drive efficiency and optimize outcomes across the board.
Skills and Education in Data Analytics
In today’s fast-paced digital world, the significance of skills and education in data analytics cannot be overstated. Organizations are increasingly dependent on data to drive their decision-making processes. This dependency instills a need for highly competent analysts who can sift through vast amounts of data, extract meaningful insights, and contribute to strategic growth. Given this context, understanding the required skill set and the role of continuous education is critical for anyone looking to thrive in the analytics landscape.
Essential Skills for Analysts
When it comes to the skill set of data analysts, a mix of technical and soft skills is paramount. Here are some core competencies that stand out:
- Data Manipulation and Analysis: Analysts should be proficient in tools like SQL, R, or Python. These tools allow for effective data extraction, transformation, and application of statistical analyses.
- Statistical Knowledge: A strong grasp of statistics is essential. Analysts must understand concepts like probability distributions, hypothesis testing, and regression analysis to interpret data accurately.
- Data Visualization: Skills in using visualization tools such as Tableau, Power BI, or even programming libraries like Matplotlib in Python can transform raw data into understandable visual stories. This clarity is crucial for stakeholders.
- Domain Knowledge: Industry-specific knowledge enriches an analyst’s ability to contextualize data findings. For example, an analytics professional in finance should have a strong understanding of economic indicators and financial models.
- Critical Thinking: The ability to analyze situations, identify problems, and come up with logical solutions is critical. This skill allows analysts to make informed recommendations rather than simply reporting results.
An analyst's arsenal must also include technical aptitude for working with databases, awareness of tools like Excel for day-to-day tasks, and an understanding of data governance standards. These core skills enable the application of data insights effectively across various business scenarios.
Growing Importance of Continuous Learning
The world of data analytics is anything but static; it changes as quickly as technology evolves. Because of this, the commitment to continuous learning is not merely beneficial—it’s essential. Here’s why:
- Keeping Up with Technological Advancements: New tools and methodologies are frequently introduced, such as advancements in machine learning algorithms or new data management systems like Snowflake or BigQuery. Analysts must stay abreast of these changes to utilize the best solutions available.
- Adapting to Regulatory Changes: With increasing emphasis on data privacy, legislation like GDPR and CCPA requires analysts to familiarize themselves with compliance and ethical data handling practices. Continuous education provides the framework for this.
- Promoting Versatility: As analytical techniques and requirements grow in complexity, acquiring new skills means greater versatility in approaching data problems. Being multi-skilled can help professionals pivot their strategies in various business situations.
- Enhancing Career Opportunities: Professionals who embrace lifelong learning often discover broader career paths. Understanding new fields like artificial intelligence in analytics not only sharpens one's abilities but opens doors for advancement beyond traditional analytics roles.
"The best way to predict the future is to create it." – Peter Drucker
Collaboration Between Analytics and Other Fields
In today's data-driven world, the significance of collaboration between analytics and various fields cannot be overstated. Organizations are increasingly recognizing that the integration of data analytics into multiple domains not only enhances decision-making but also drives innovation. By harnessing the strengths of analytics with insights from related disciplines, businesses can create a comprehensive understanding of their operations and the marketplace.
Data Science and Its Interplay with Analytics
Data science, often seen as the backbone of modern analytics, has a profound impact on how businesses leverage their data. At the core of this interplay lies a foundation of statistics, programming, and domain knowledge. Data scientists deploy their skills to derive actionable insights from vast amounts of data, blending techniques from traditional statistics with techniques from machine learning.
Benefits of Integrating Data Science with Analytics:
- Enhanced Predictive Power: Data scientists use models that can adapt and learn from new data, improving the accuracy of predictions over time.
- Deeper Insight Generation: Interdisciplinary collaboration often uncovers hidden patterns within data that might be overlooked in siloed environments.
- Optimization of Processes: By analyzing data from various perspectives, businesses can streamline operations, leading to increased efficiency and reduced costs.
However, it’s important to recognize some of the considerations that come with this collaboration. Integrating data science into existing analytical frameworks can present challenges such as aligning different methodologies, ensuring consistent data quality, and addressing the varying levels of technical expertise among team members. Companies must invest in training programs to empower their workforce while promoting a culture that encourages knowledge sharing and collaboration.
The Dynamic Relationship with Business Intelligence
Business intelligence (BI) and analytics are two sides of the same coin, yet they serve distinct purposes. While BI focuses on descriptive analytics—what happened in the past—analytics take it a step further by predicting future trends and behaviors. This dynamic relationship creates a robust environment for decision-makers to act upon insights rather than mere historical data.
Key Elements of Collaboration Between BI and Analytics:
- Streamlined Reporting: BI tools provide a platform for standardized reporting, making it easier for analytics to build on that foundation with more sophisticated data models.
- Real-Time Access to Data: A close collaboration allows analytics to leverage real-time data from BI tools, ensuring that insights are not just accurate but also timely.
- User-Friendly Dashboards: Integrating advanced analytics into BI systems can result in user-friendly dashboards, which help non-technical stakeholders understand complex data without getting lost in technical jargon.
A successful collaboration hinges on mutual understanding and respect for each field's contributions. Organizations must create pathways for continuous feedback and knowledge exchange between analytics teams and BI teams, fostering an environment where both can thrive.
"In essence, the cooperation between analytics and related disciplines serves as a catalyst for innovation, enabling organizations to not only survive in the marketplace but to lead it."
Through such synergy, businesses not only gain a competitive edge but also become more adaptable to changing market conditions, thus ensuring their long-term success.
Cloud-Based Analytics Solutions
In the rapidly evolving landscape of data analytics, cloud-based solutions have emerged as a cornerstone for innovation and efficiency. Organizations today are faced with massive data volumes. The growing reliance on cloud infrastructures has prompted changes to how businesses store, process, and analyze data. This shift has introduced notable benefits that streamline operations while posing new challenges about security and migration.
Advantages of Cloud Analytics
The benefits of adopting cloud analytics are manifold:
- Scalability: Cloud platforms can easily scale resources up or down based on current demands. This flexibility means organizations can adjust analytics capabilities as their needs change without heavy investments in physical hardware.
- Cost-Effectiveness: Traditional analytics setups often require significant upfront capital for hardware and software. With cloud solutions, the pay-as-you-go model allows companies to manage costs more effectively, particularly for smaller enterprises.
- Accessibility: Analysts can access cloud-based analytics tools from anywhere, provided they have an internet connection. This level of accessibility promotes a more collaborative work environment, enabling teams to share insights in real-time.
- Integration of Advanced Tools: Cloud environments often feature integration with AI and machine learning tools. This integration can enhance data analysis accuracy and help organizations derive insights faster.
- Automatic Updates: With cloud solutions, updates and upgrades are automatically managed by the service provider, ensuring users have access to the latest features and security protections.
"The cloud is all about interoperability, flexibility, and responsiveness. As we move forward, companies must adopt these solutions to not just survive, but thrive in the data economy."
Challenges in Migration to Cloud Platforms
While the advantages are clear, migrating to cloud platforms can be fraught with obstacles that organizations must navigate carefully:
- Data Security Risks: The primary concern for many organizations revolves around data security. Sensitive data in the cloud can attract unauthorized access. Hence, serious consideration is necessary to employ robust encryption and access controls.
- Compliance Issues: Different sectors have their regulations pertaining to data privacy. Moving to the cloud can complicate compliance requirements, which necessitates careful planning to ensure that all regulations are met.
- Downtime During Migration: Transitioning to cloud solutions can lead to downtimes, impacting business operations. Effective planning and execution are vital to minimize these disruptions.
- Complexity of Integration: Organizations often have legacy systems they must integrate with new cloud solutions. This can be a complex task requiring significant technical expertise and careful execution.
- Cost Management: While cloud analytics can be cost-effective, uncontrolled usage can lead to unexpected costs. Organizations need to continuously monitor and manage cloud resources to avoid overspending.
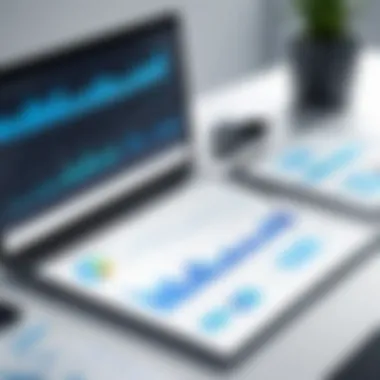
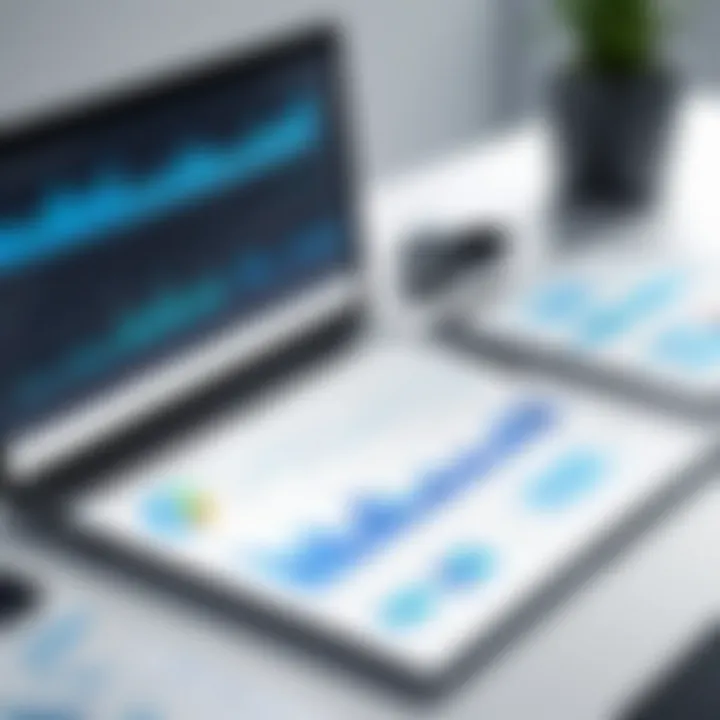
Navigating these challenges requires a comprehensive strategy that balances the need for innovation with the necessary caution that comes with handling vast amounts of data. As companies continue to explore cloud-based solutions, understanding both advantages and challenges will be key to unlocking the full potential of data analytics.
Future Outlook of the Data Analytics Industry
Understanding the future outlook of the data analytics industry is not just an academic exercise; it’s crucial for organizations aiming to stay relevant in today's fast-paced market. As data continues to swell and technologies evolve, the analytics landscape presents an array of possibilities that businesses would do well to anticipate. This understanding goes beyond just recognizing potential changes; it involves crafting strategic responses that align with these predicted developments.
The focus on data-driven decisions only intensifies as organizations seek competitive advantages. Being able to predict future trends can lead to better planning and execution. Thus, grasping the future outlook equips IT professionals and business leaders with the knowledge to harness upcoming technologies that can dramatically alter the analytics paradigm.
Predicted Trends in Data Analytics
The landscape of data analytics is like watching a chess game unfold—each move creates new opportunities and challenges. As we look forward, a few trends are shaping how organizations perceive and utilize data analytics:
- Augmented Analytics: Incorporating AI tools will assist analysts in deriving insights more efficiently, helping to bridge gaps in expertise and speeding up analysis workflows.
- No-Code/Low-Code Platforms: These platforms are becoming a staple in making data analytics accessible to users without deep technical skills. Hence, empowering a broader range of employees to make sense of data.
- Data Democratization: There is a growing emphasis on making data accessible to all employees, regardless of their tech background. This cultural shift requires organizations to rethink their data strategies.
- Edge Analytics: With the growth of IoT (Internet of Things), processing data at the edge (closer to the source) will minimize latency and improve real-time decision-making.
- Data Quality Management: As more data floods in, organizations will find that robust frameworks for data quality will be essential. This is all about ensuring that the data is accurate and reliable before any analytics can happen.
The relevance of these trends cannot be understated. They offer both innovative opportunities and necessary adaptations in an increasingly data-driven world. Companies ignoring these developments may soon find themselves at a disadvantage.
Long-Term Implications for Businesses
Considering these trends, the long-term implications for businesses are profound. Firstly, companies that embrace augmented analytics and low-code platforms may witness a radical shift in how decisions are made. Imagine a financial analyst getting real-time insights into market trends without needing a data science degree. This shift could enhance agility and responsiveness, allowing organizations to pivot quickly in changing circumstances.
Furthermore, as data democratization becomes commonplace, the synergy between departments may foster a culture of collaboration, where insights can be shared freely across teams. This collaborative approach can lead to more innovative solutions as different perspectives come together to analyze data holistically.
However, there are challenges too. As organizations democratize data access, oversight must be maintained to avoid potential misuse. Implementing strong data governance protocols will become essential for mitigating risks while reaping the benefits of open access. Companies that are adept at finding this balance will find themselves thriving in a landscape that is increasingly rich in data yet fraught with complexities.
"The future belongs to those who prepare for it today." - John F. Kennedy
Navigating the future of data analytics means not just adapting to change but anticipating it. Those that do, with clear strategies and an understanding of these emerging trends, will not only survive but also thrive.
Case Studies of Successful Analytics Implementations
In the fast-evolving data analytics industry, understanding how organizations effectively implement analytics solutions is vital. These case studies not only showcase successful applications but also provide insights into challenges faced and solutions found. They serve as blueprints for other companies aiming to harness the power of data.
Examining Real-World Applications
Real-world applications of data analytics reveal how various industries leverage data to improve operations and decision-making. Consider a large retail chain that implemented a predictive analytics system to optimize inventory management. By analyzing historical sales data and market trends, they could anticipate demand for specific products. This reduced overstock situations and minimized lost sales due to stockouts. The success of such implementations demonstrates the importance of data-driven strategies in enhancing efficiency and profitability.
Another noteworthy case involves a healthcare provider utilizing analytics to enhance patient care. The organization employed advanced data visualization tools to track patient outcomes and treatment effectiveness in real-time. This enabled doctors to identify issues quickly and make informed decisions regarding patient treatment plans. Such applications not only improved patient satisfaction but also resulted in significant cost savings for the hospital.
Impact Measurement and Outcomes
Measuring the impact of analytics implementations is crucial for determining their effectiveness. Without clear metrics and outcomes, organizations may struggle to justify their investments in analytics technology. For the retail chain mentioned earlier, key performance indicators (KPIs) such as reduced carrying costs and improved sales volumes were monitored closely. Successful outcomes were not only quantified in financial terms but also in customer satisfaction levels, indicating a well-rounded approach to evaluation.
For the healthcare case study, an increase in treatment success rates and patient recovery times were significant indicators of improved care. By establishing a robust framework for measuring outcomes, the healthcare provider could continually refine its practices, staying ahead of industry trends.
Metrics might include:
- Return on Investment (ROI): Assesses the financial gains achieved from analytics initiatives versus the costs involved.
- Customer Satisfaction Scores: Evaluates how data-driven changes positively affect customer interactions.
- Operational Efficiency Improvements: Analyzes time and resources saved due to analytics-derived decisions.
These case studies illustrate that successful analytics implementations rely on rigorous assessments and a commitment to continuous improvement. Organizations that effectively measure and interpret outcomes can pivot quickly and maintain a competitive edge in the data analytics market.
Ethical Considerations in Data Analytics
In an era where data reigns supreme, the ethical dimensions surrounding its use are gaining traction. As organizations increasingly harness the power of data analytics, the implications of how this data is collected, analyzed, and utilized cannot be overlooked. Ethical considerations in data analytics involve navigating a labyrinth of privacy concerns, regulatory frameworks, and moral responsibilities. These elements are pivotal not only for building trust with consumers but also for ensuring compliance with evolving legislation.
Understanding these considerations highlights the necessity of ethical awareness among professionals in the field. Without a foundation built on responsible practices, the advanced methodologies we're developing risk not just reputational damage but legal repercussions as well. Essentially, ethical data management is not just a fancy add-on but a core requirement in making sound data-driven decisions.
Addressing Ethical Dilemmas
As analytics takes on more complex forms, the ethical dilemmas we're facing become more pronounced. Take, for instance, the issue of consent. Often, individuals may not fully grasp the extent of how their personal data is being utilized. This raises questions about informed consent. Moreover, biases inherent in machine learning algorithms can lead to unfair decision-making processes. Numerous cases have come to light where biased data resulted in discriminatory practices, particularly in sectors like recruitment and law enforcement.
Organizations must therefore cultivate a culture of ethical mindfulness. Here are some practical steps:
- Clarity in Consent: Make it clear to users what data is being collected and how it’s used.
- Regularly Assess Algorithms: Continually review machine learning models for biases and consistently aim to rectify them.
- Engagement with Stakeholders: Invite feedback from consumers, data subjects, and even advocacy groups to understand their concerns.
Addressing these dilemmas is challenging but imperative. It’s about striking a balance between leveraging data for insights and respecting individual rights.
Frameworks for Ethical Data Use
Establishing frameworks for ethical data use forms the backbone of responsible analytics practices. Various guidelines and ethical considerations have emerged, aimed at guiding organizations toward transparency and fairness.
A noteworthy framework is the Fair Information Practices, which includes:
- Transparency: Organizations must disclose how they collect and use data.
- User Control: People should have control over their data and the power to request its deletion.
- Data Security: Measures must be implemented to protect data from breaches and unauthorized access.
In addition, setting up Ethics Review Boards can help monitor and evaluate data practices within organizations. These boards are tasked with:
- Evaluating the ethical implications of new analytic tools and technologies.
- Ensuring that data practices comply with relevant laws and are ethically sound.
Implementing such frameworks and boards fosters an environment where ethical considerations are at the forefront, thus nurturing public confidence in an increasingly data-driven world.
"In the digital age, ethical oversight is not merely an obligation, but an essential tool for sustainable and responsible business operations."
Organizations must strive for not only compliance but also the embodiment of ethical principles within their analytical practices. The layers of complexity surrounding data today require a commitment to ethical stewardship that resonates with their clientele and meets societal expectations.
Closure: Preparing for Change
As we look to the horizon of the data analytics industry, understanding the evolving landscape is crucial. The rapid pace of technological advancement means that businesses and professionals must adapt continuously to stay relevant. The key takeaway here is that change is not merely an option—it’s a necessity. Organizations that are in tune with emerging trends have a significant advantage in leveraging data for informed decision-making.
The Evolving Role of Data Analytics
Data analytics is transitioning from a supportive role to a strategic one. It’s now at the heart of many organizations’ operations, influencing everything from marketing strategies to supply chain efficiency. The increasing complexity of data demands that analytics professionals not only understand data but also its broader implications on business strategies. The lines between data analytics, artificial intelligence, and data science are blurring, resulting in a need for professionals who are skilled in multiple domains.
Today, the analyst is no longer merely a number cruncher. Instead, they are storytellers—conveying insights gleaned from data that can drive business outcomes. It’s about connecting the dots between data points to provide narratives that stakeholders can comprehend and act upon. Moreover, with data visualization tools becoming more accessible, the ability to present data in an easily digestible manner is paramount. This shift emphasizes the need for cross-disciplinary skills, not just technical prowess in handling data.
Strategies for Staying Competitive
Navigating this dynamic field requires a proactive approach. Here are some strategies to maintain a competitive edge:
- Invest in Continuous Learning: The analytics field is ever-evolving with new tools and methodologies. Professionals should pursue ongoing education through workshops, online courses, and certifications to keep their skills sharp.
- Embrace Collaboration: Cross-functional teams can produce richer insights. Analysts should work closely with departments such as marketing, finance, and IT to deliver integrated solutions that reflect the needs across the organization.
- Utilize Advanced Tools: Stay updated on the latest analytics tools. For instance, platforms like Tableau and Power BI offer advanced visualization capabilities, while programming languages like Python and R provide extensive data processing functionalities.
- Focus on User Experience: Whether it’s your dashboard, reports, or data delivery, the user experience should be seamless. Tools should be intuitive and geared toward the end user to enhance adherence and enable data-driven decision making.
"The only constant in the world of data analytics is change."
- Network and Share Knowledge: Engaging with other professionals through forums, conferences, and online communities can offer insights into best practices and emerging technologies.