Understanding Customer Analytics: Insights and Applications
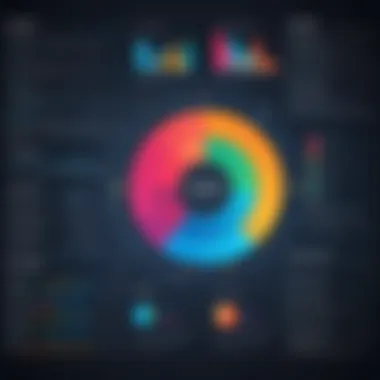
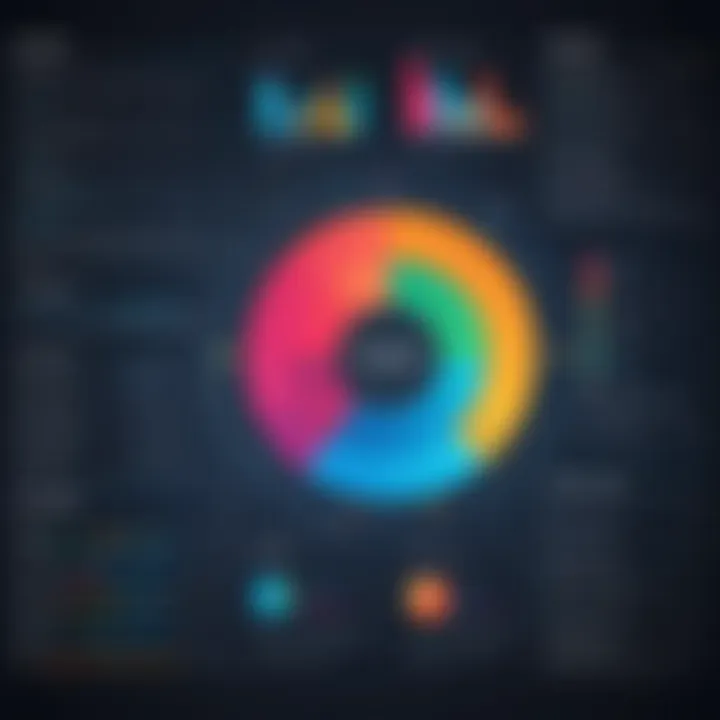
Intro
Customer analytics refers to the systematic study of customer data that businesses utilize to make informed decisions. It has become more critical in recent years due to the explosive growth of data generated by customer interactions across different touchpoints. From online purchases to social media engagement, every interaction provides valuable information if analyzed correctly. This article will unpack the methodologies, significance, and multifaceted nature of customer analytics. We will explore not only how businesses can harness this data but also the broad implications it has on strategies, customer experiences, and results.
Understanding Analytics Applications in Business
Businesses leverage customer analytics to boost operational efficiency and improve strategies. There are several ways these analytics can manifest:
- Segmentation: Understanding distinct customer groups based on behavior, preferences, and demographics.
- Predictive Analytics: Using customer data to forecast future buying behaviors and trends.
- Personalization: Tailoring experiences suited to individual customer needs, enhancing loyalty and satisfaction.
“Analytics allows businesses to make data-driven decisions that align closely with changes in consumer behavior.”
These applications lay the groundwork for strategic developments within companies, as they remain intimately connected with consumer satisfaction and retention.
Key Challenges in Interpreting Customer Data
Despite the benefits, challenges abound in interpreting customer data:
- Data Quality: Inaccurate or incomplete data can lead to misguided conclusions.
- Integration: Businesses often struggle to aggregate data from disparate sources due to varying formats or systems.
- Privacy Concerns: Increasing regulations over data privacy (such as GDPR) have implications on how companies collect and manage customer data.
Future Implications of Customer Analytics
The implications of how customer data analytics will evolve suggest much promise. With advancements in artificial intelligence and machine learning, the future holds the possibility of more nuanced and real-time analytics that can drive decision-making. Organizations investing into these emerging technologies are likely to offer customer experiences that genuinely resonate with today’s fast-paced environment.
Understanding customer analytics ultimately positions a business to gain a slender advantage over competitors by making informed decisions that not only address current needs but anticipate future consumer behavior.
Preface to Customer Analytics
Customer analytics has become an essential tool for businesses seeking a competitive edge. With the explosion of data generated from various customer interactions, the ability to translate this information into actionable insights can make the difference between success and failure. Understanding customer behavior, preferences, and trends is key. This section sets the stage for comprehending the various aspects of customer analytics.
Definition of Customer Analytics
Customer analytics refers to the practice of collecting, analyzing, and interpreting customer data to improve business outcomes. It encompasses various data-driven techniques and methodologies aimed at gaining deeper insights into customer behavior and preferences. The definition further highlights how organizations leverage this information to inform their marketing and customer service strategies.
From demographic data to behavioral patterns, customer analytics involves several components that combine to reveal trends. Its primary goal is to enhance the engagement level with customers and drive more effective decision-making in a variety of sectors.
Historical Evolution
The history of customer analytics can be traced back to the mid-20th century, when businesses first began compiling customer data for market research. In its early forms, customer analytics was a labor-intensive endeavor, primarily relying on surveys and questionnaires. As technology evolved, so did the methodologies employeed for data gathering.
By the late 1990s, advancements in computing led to the rise of analytical tools, allowing for faster processing of customer information. The introduction of Customer Relationship Management (CRM) systems marked a significant milestone in customer analytics. Design of CRM systems enabled real-time data collection, paving the way for more advanced analytical methods. Today, organizations leveraging big data technologies and artificial intelligence in their customer analytics practices demonstrate sophistication unseen in the past.
Customer analytics continues to evolve, keeping pace with new trends in technology and market demands. This ongoing advancement suggests that businesses can expect even more powerful tools that will further help them to understand their customer base in a deep and meaningful way.
Importance of Customer Analytics
Customer analytics plays a pivotal role in today's highly competitive landscape. It refers to the systematic gathering and examination of customer data to derive insights that inform businesses about customer needs, preferences, and behaviors. Its importance can largely be categorized into three significant elements: enhancing customer experience, supporting informed decision-making, and creating competitive advantage.
Enhancing Customer Experience
The use of customer analytics directly elevates customer experience. By analyzing data collected from various touch points, businesses identify what customers appreciate and what discontents they experience. For instance, a retail company that employs customer feedback analysis can precisely understand which products attract interest based on purchase patterns and reviews. Leveraging this insight, companies can tailor offerings or make necessary adjustments to optimize satisfaction.
Key benefits include:
- Personalized interactions based on past behavior.
- Swift response mechanisms to resolve issues.
- Improved product recommendations, tailored to individual needs.
Such enhancements result in fostering loyalty, likely making customers return for future purchases, which minimizes churn.
Informed Decision-Making
Informed decision-making is another critical aspect whose value cannot be overstated. Organizations that tap into customer analytics can rely on empirical data rather than intuition. They understand trends, track performance, and pivot strategies when necessary. This reality contrasts with businesses where decisions are based on guesswork, yielding less favorable outcomes.
For instance, imagine a company that regularly evaluates its marketing campaigns based on customer data. It can discern which channel yields the highest engagement or even segment audiences for more targeted campaigns. Some decisions informed by data analysis may involve:
- Allocating marketing budgets judiciously.
- Identifying opportunities in emerging markets.
- Developing customized products based on unmet needs.
While subjective practice may lead to randomness, big data enables a systematic approach that minimizes risks associated with decision-making.
Competitive Advantage
Customer analytics also equips businesses with tools to achieve a competitive advantage. In an era where customer preferences evolve swiftly, staying ahead in understanding these shifts is vital.
Organizations that consistently refine their approach based on analytical findings can set themselves apart. For example, firms that utilize analytics effectively often outperform competitors in customer engagement metrics, enhancing both brand value and market share.
Key differentiators may include:
- Capacity to forecast customer tendencies, allowing proactive measures.
- Deployment of dynamic pricing strategies that reflect market demand.
- Crafting personalized marketing that resonates more deeply with specific demographics.
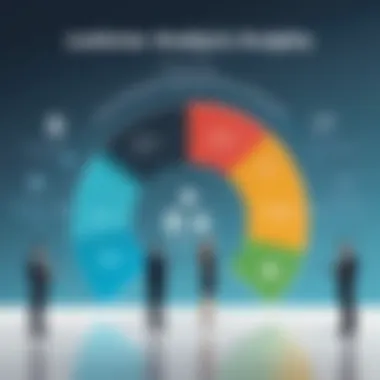
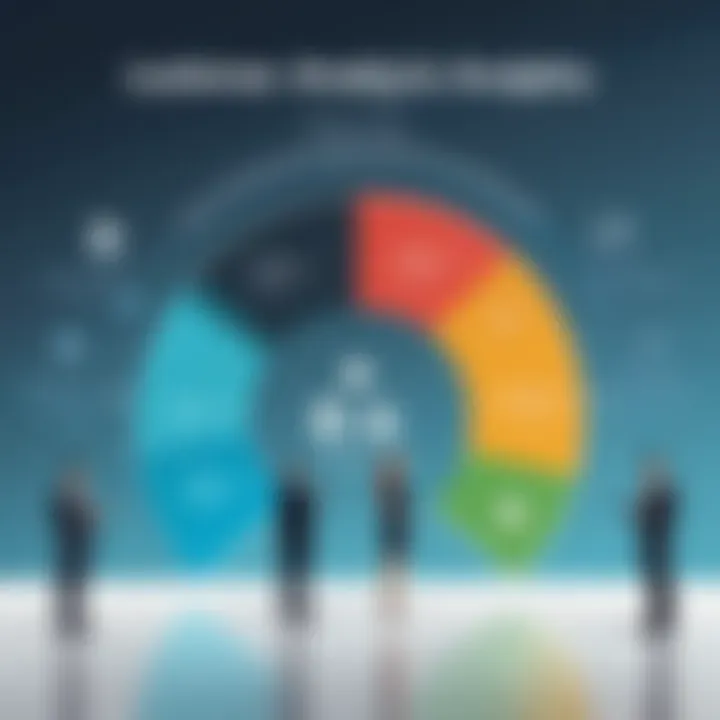
Adopting a data-centric approach can enhance positioning in crowded markets, serving both existing clients and attracting potential clients.
Customer analytics transforms customer interactions into actionable insights, fueling both growth and innovation. It is indispensable for any firm aiming for sustainability and relevance in the modern economy.
Key Components of Customer Analytics
Understanding the key components of customer analytics is crucial in maximizing the benefits of data-driven strategies. Each element within this framework offers unique insights that informed decision-making and strategy formulation. Proper insight into these components helps organizations align their objectives with deeper customer understanding, driving growth.
Data Collection Methods
The first phase in any analytics process is data collection. Organizations harness various data collection methods to gather pertinent information from their customers. Common approaches include:
- Surveys: Distributed through channels such as email, website pop-ups, or social media, surveys can provide direct insights into customer preferences and opinions.
- Web Analytics: This involves tracking user behavior on websites using tools like Google Analytics. It reveals how customers navigate through digital platforms.
- Customer Feedback: Utilizing feedback forms or reviews provides unfiltered customer sentiment.
- CRM Systems: Customer Relationship Management tools capture detailed records of customer interactions and behaviors, helping to identify trends over time.
Careful selection of methods ensures relevant data is obtained for effective analysis. Each method also requires thoughtful implementation to ensure data quality and representativity.
Data Processing Techniques
Once data is collected, it requires processing. This step is essential as raw data can be messy and cluttered, leading to errors if used directly. Key techniques include:
- Data Cleaning: This involves identifying and correcting errors or inconsistencies in datasets, ensuring the reliability of subsequent analyses.
- Data Integration: Combining data from various sources into a single view provides a unified perspective, making it easier to analyze interactions and trends.
- Data Transformation: Adjusting the format or structure of data helps in enhancing compatibility within various analytical models.
Keeping a focus on these techniques allows organizations to better prepare their data for in-depth levels of analysis.
Data Analysis Models
The final critical component of customer analytics is the employment of data analysis models. Different methodologies exist to empower organizations in extracting actionable insights from processed data:
- Regression Analysis: Identifies relationships between variables to predict future customer behavior based on historical data.
- Cluster Analysis: Groups customers with similar characteristics or behaviors, fostering targeted marketing approaches.
- Decision Trees: Offer visual representation for decision-making paths within complex analytics.
- Time Series Analysis: Examines data points collected over time to identify trends and cyclical patterns.
Integrating these models into the analytics process ensures data-driven decisions lead to efficient strategies tailored to distinct customer needs. Their application provides profound understanding to inform strategic directions generated by real data analyses.
Comprehensive analysis helps in gaining a clear insight into customer preferences, ultimately enhancing business growth and customer satisfaction.
Techniques Used in Customer Analytics
Customer analytics employs various techniques to analyze data and extract value from it. Understanding these techniques is critical for businesses as they endeavor to enhance decision-making, develop marketing strategies, and improve customer relations. Each method has its unique purpose yet contributes to a unified goal: improved business performance by understanding consumer behavior.
Descriptive Analytics
Descriptive analytics is centered on understanding historical data to inform business decisions. It focuses on summarizing past events and identifying trends, helping organizations comprehend what has already happened.
Key Benefits of Descriptive Analytics:
- Data Summarization: Provides a summary of past behaviors and events through metrics and KPIs.
- Trend Analysis: Identifies patterns in consumer behavior over designated periods.
- Visual Representation: Employs data visualization tools to facilitate immediate understanding for stakeholders.
By utilizing descriptive analytics, a retail company can study previous sales trends to inform future inventory purchases and promotional campaigns.
Predictive Analytics
Predictive analytics takes a forward-looking approach, using statistical methods and algorithms to forecast future events. It employs historical data along with machine learning techniques to predict outcomes. This enables businesses to stay ahead of consumer demands and make well-informed decisions.
Considerations for Implementing Predictive Analytics:
- Data Integrity: Ensuring accurate and timely data capture is critical for reliable predictions.
- Model Selection: The choice of predictive model influences the outcome; common types include regression analysis and time series forecasting.
- Continuous Improvement: Models require updates based on new data to maintain accuracy over time.
As an example, financial institutions might implement predictive analytics to anticipate default risk among borrowers, helping mitigate financial losses before they happen.
Prescriptive Analytics
Prescriptive analytics builds on predictive data by recommending actions. It often uses optimization techniques and algorithms to find the best course of action under given circumstances. Rather than just forecasting an outcome, it focuses on the potential pathways to achieve desired results.
Implementing Prescriptive Analytics:
- Scenario Analysis: Evaluate multiple potential scenarios and their consequences to guide strategy.
- Resource Allocations: Optimize resource allocations or product offerings based on consumer preferences and buying behaviors.
- Improvement Recommendations: Glean insights from data, suggesting improvements in marketing techniques, operational efficiency, and customer engagement.
In practice, a logistics company might utilize prescriptive analytics to maximize delivery efficiency based on current traffic patterns and past delivery times.
Sentiment Analysis
Sentiment analysis involves examining opinions or emotions from text or voice data. Businesses leverage this technique to understand customer feelings about their services or products. This can largely shape brand perception and has direct implications on customer loyalty and satisfaction.
Importance of Sentiment Analysis in Customer Analytics:
- Enhances Feedback Loops: By capturing customer opinion, organizations can adapt and refine products.
- Brand Health Monitoring: Determines how well a brand resonates with consumer feelings over time.
- Social Media Insights: Monitors sentiment across platforms like Facebook and across forums like Reddit to stay connected to broad public feelings.
Deploying sentiment analysis can enable marketers to understand how a spent ad campaign is perceived. If negative emotions emerge from the analysis, a business can quickly pivot its approach to address potential issues.
Understanding these techniques allows companies to not just react, but anticipate, fostering greater customer loyalty and driving holistic business growth.
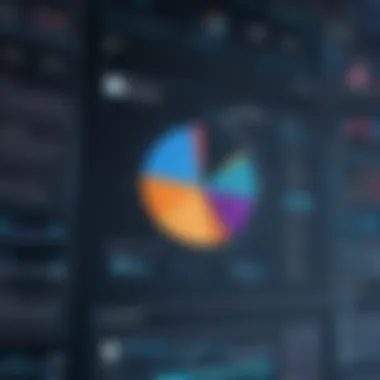
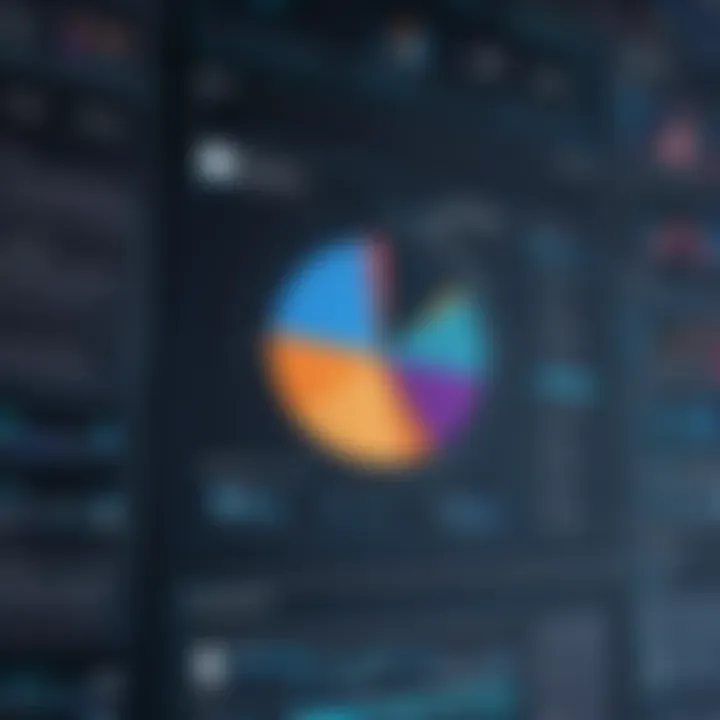
Ultimately, the varied techniques of customer analytics empower businesses to grasp consumer behaviors and market dynamics, tailoring their strategies to enhance success.
Challenges in Customer Analytics
Customer analytics holds tremendous potential, but it comes with its share of difficulties. More understanding of these challenges can lead to effective solutions. Companies must address various obstacles to truly harness the benefits of customer analytics. These challenges include data quality issues, privacy concerns, and the integration of customer analytics within existing systems. By tackling these challenges, organizations can improve their decision-making and enhance customer experiences.
Data Quality Issues
Data quality is fundamental for generating valuable insights. If the information gathered is incomplete or incorrect, it leads to flawed analysis. High-quality data contributes to accurate forecasting and helps in identifying customer behavior trends. Key considerations in data quality are:
- Accuracy: Data must be correct and without mistakes.
- Completeness: Need full data to prevent misinformed decisions.
- Consistency: Harmonizing data from different sources aids integration and avoids discrepancies.
Uninferring oerrors in data collection methods can could enhance overall reliability. Efforts to facilitate clean data management are vital. In doing so, organizations can maintain trust in analytics outcomes.
Privacy Concerns
Today’s customers are increasingly aware of their data privacy rights. Companies face a dual challenge: safeguarding user data while still capturing insights. Privacy practices need to be transparent and compliant with regulations like GDPR. Companies must focus on:
- User Consent: Always ask for user approval before gathering data.
- Data Anonymization: Protect identities by removing personal identifiable information.
- Secure Data Storage: Safeguarding against potential breaches ensures customer trust.
Consumers expect businesses to uphold their privacy while also providing personalized experiences. Neglecting growing privacy concerns can diminish brand equity and lead to loss of customer. So, managing privacy sensitively must become a core part of business strategy.
Integration with Existing Systems
Introducing customer analytics insights requires seamless integration with current frameworks. If the analytics tools clash with existing systems, companies will face operational inefficiencies. Key factors influencing successful integration include:
- Scalability: Ensure tools can grow with the company.
- Compatibility: Check analytics platforms work well with current software.
- Operational Training: Provide staff with appropriate training to leverage analytics results.
Companies need to align their technology infrastructure effectively with analytics tools. When successful, this husbandry settle analytics at the core of business operations, driving enhanced performance.
Data quality, privacy, and systems integration present pivotal challenges in customer analytics. Addressing these areas effectively fosters both confidence in decision-making and customer loyalty.
Applications of Customer Analytics
The field of customer analytics has various applications that allow businesses to refine processes, enhance customer satisfaction, and achieve better profits. In this section, we explore three core applications to highlight their importance: marketing strategies, customer segmentation, and customer retention strategies.
Marketing Strategies
Marketing strategies are critical frameworks that help how a company reaches its customers. Customer analytics aids in shaping these strategies by offering insights into consumer behaviors and preferences. With data at hand, businesses can tailor their campaigns more effectively, leading to increased engagement and conversions.
- Targeted Advertising: Customer analytics allows companies to identify the most responsive audience segments, generating targeted advertising efforts.
- Campaign Optimization: By evaluating what types of ads or what messages work best, firms can continuously refine their approach. This leads to streamlined marketing processes and reduced costs.
- Performance Tracking: Businesses must track the effectiveness of different marketing initiatives. Customer analytics provides the necessary metrics to evaluate performance in real time.
Real-time feedback serves as a vital aspect in today’s fast-paced market, where a firm can adjust campaign direction quickly.
Customer Segmentation
Customer segmentation involves dividing a broad customer base into identifiable groups that exhibit similar characteristics. This is traditionally done using demographic, behavioral, or psychographic factors. Employing customer analytics to optimize segmentation brings numerous benefits:
- Personalization: Applying main insights into customer purchasing behaviors allows businesses to personalize services, leading to enhanced customer experience.
- Targeted Promotions: Groups identified through segmentation geology allow firms to run promotions that resonate effectively, yielding higher conversion rates.
- Resource Allocation: Recognizing where budget and effort should focus can aid efficiency in marketing spend.
In evaluation, analytics assist companies in painting a clearer picture of their target demographics, elevating marketing success.
Customer Retention Strategies
Growing a business is not only about acquiring new customers. Retaining them is a crucial task requiring thoughtful strategies. Analytics can play an informative role in customer retention efforts:
- Predictive Analysis: By evaluating customer behaviors, businesses can determine which customers are at risk of leaving and implement retention strategies proactively.
- Program Effectiveness: Measuring loyalty programs through customer feedback analytics aids fine-tuning designs such that they truly support returning customers.
- Feedback Mechanisms: Customer analytics can streamline how feedback is collected to explore what modifications and offers attract returning business.
The value of retaining a customer can exceed acquiring one significantly, amplifying the case for a well-strategized retention plan backed by data-driven insight.
Technological Advancements Impacting Customer Analytics
Technological advancements have revolutionized the way businesses approach customer analytics. The emergence of new technologies plays a crucial role in enhancing the capabilities related to data processing and customer understanding. By leveraging advanced technological tools, companies are able to extract deeper insights from customer data, refining their strategies and outcomes overall. This section delves into the key advancements shaping the landscape of customer analytics today.
Artificial Intelligence and Machine Learning
Artificial intelligence (AI) and machine learning (ML) are at the forefront of technological advancements impacting customer analytics. These technologies enable organizations to process vast amounts of data efficiently. AI algorithms can analyze behavioral patterns, anticipate future actions, and even automate decision-making processes.
The applications of AI and ML within customer analytics are manifold. Businesses can use these technologies to conduct:
- Behavioral Analysis: Identifying patterns in customer behavior helps to tailor marketing strategies and predict future trends.
- Churn Prediction: Machine learning models can categorize customers based on the likelihood of leaving, allowing companies to implement timely intervention strategies.
- Personalization at Scale: AI can deliver a unique customer experience through tailored recommendations across platforms.
However, implementing AI and ML comes with fundamental considerations. Data security is paramount, requiring organizations to strike the balance between leveraging customer insights and addressing privacy concerns. Misuse of these powerful tools could lead to damaging consequences, affecting customer trust and company reputation.
Big Data Technologies
Big data technologies represent another critical advancement in customer analytics. The term encompasses techniques that handle large data sets efficiently, extracting useful information from diverse sources. The ability to process big data allows organizations to tap into a broad spectrum of customer input, driving decisions based on comprehensive data insights.
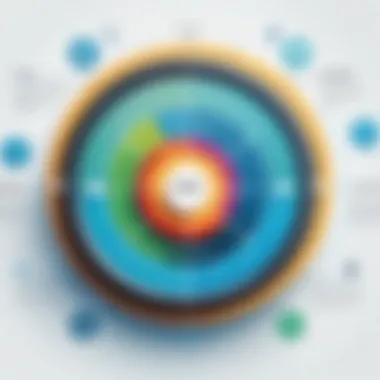
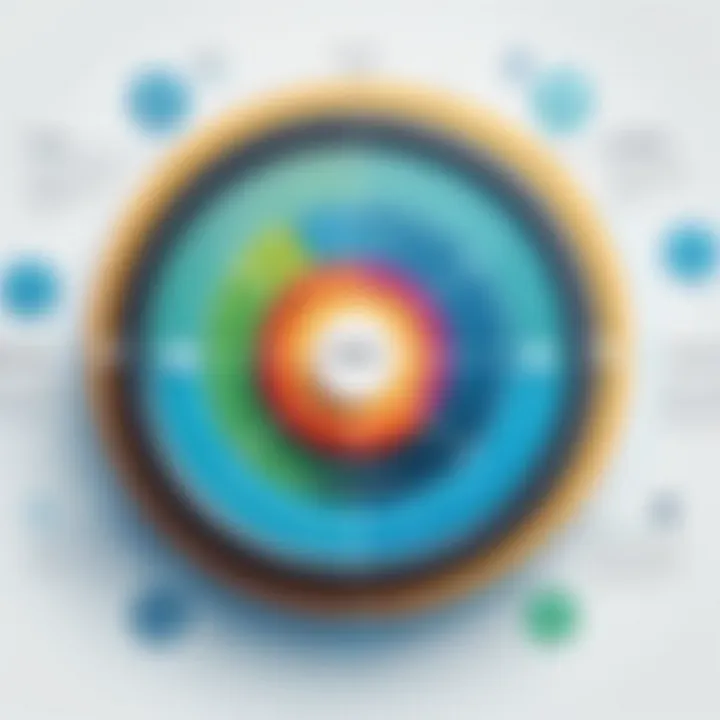
Utilization of big data enhances analytics efforts through:
- Advanced Analytics: Companies can employ sophisticated analytics tools to reveal actionable insights from seemingly disparate data.
- Real-Time Engagement: Immediate data processing leads to more responsive strategies, enabling everything from consumer behavior tracking in real time to campaign optimization based on immediate feedback.
- Holistic View of Customer: Access to an extensive range of customer data offers a complete picture, empowering marketers and strategists to visualize trends effectively.
Success in harnessing technological advancements lies not only in adopting new tools but also in understanding their implications and responsibilities.
Case Studies in Customer Analytics
Case studies provide more than just examples; they act as real-world snapshots that showcase how businesses leverage customer analytics for practical applications. Understanding how various industries implement these analytical techniques can illuminate strategies that achieve tangible results. For IT professionals, cybersecurity experts, and students, these case studies serve as foundational learning tools to extract best practices while highlighting the potential pitfalls encountered. One must pay attention to specific elements, such as the method of platform integration, scope of analytics applied, and the measuring of outcomes after implementation.
Retail Sector
The retail sector vividly illustrates the power of customer analytics in driving sales and improving customer satisfaction. Companies like Walmart have employed analytics to optimize inventory management processes. By harnessing point-of-sale data, Walmart effectively predicts which products will sell and when, guiding purchasing decisions and reducing excess stock. This practice maximizes operational efficiency and enhances customer experience by ensuring popular items are available when needed.
Another notable case is Target’s guest shopping behavior analysis using customer purchase history. Post-analysis, they discovered customers’ preferences from aggregated data, enabling personalized marketing tactics, which resonated well with the audience. This case confirms how a strategic focus on data can result in tailored communications, ultimately fostering brand loyalty.
Financial Services
In the more scrutinized financial services sector, customer analytics extend beyond marketing to fraud detection and risk management. Financial institutions, such as Bank of America, gather large volumes of transaction data to develop customer profiles. This analysis aids in identifying suspicious activities that deviate from established patterns, providing necessary red flags for security teams.
Furthermore, the enhanced understanding of customer behavior fosters the design of new financial products that cater to specific demographics or consumer behaviors. For instance, Citibank uses analytics not only to detect anomalies but also to tailor credit offerings based on customer profiles. Thus, targeting products to solve real customer problems becomes achievable, which boosts satisfaction and retention rates.
E-Commerce
E-commerce has revolutionized the way customer analytics are utilized owing to its inherently data-driven nature. Companies such as Amazon brilliantly integrate analytics to refine product recommendations based on browsing history, purchase patterns, and user feedback. This personalized approach stems from thorough data analysis, often leading to higher conversion rates. The deployment of collaborative filtering algorithms showcases some of the advanced techniques employed to make insightful recommendations—the essence of customer-driven strategy.
Additionally, the use of heatmaps allows businesses like eBay to identify consumer navigation patterns on their website. This analysis leads to improved site structure and enhances usability, directly impacting time spent on the site and the likelihood of conversion, which exemplifies effective use of analytics in anticipating user behavior.
Effective customer analytics not only boosts business outcomes but also enhances end-user satisfacion. Understanding these case studies is crucial for professionals looking to innovate within their fields.
Byexamining how these sector leaders maneuver through customer analytics, various benefits become apparent, such as improved customer insights and optimized business processes model that aligns with strategic goals.
Future Trends in Customer Analytics
The landscape of customer analytics is constantly evolving. Future trends reflect how businesses adapt to changing consumer behaviors, technology, and market demands. Understanding these trends is essential to stay ahead in a competitive environment. This section explores significant trends shaping customer analytics today. Adaptation to these elements provides organizations with a distinct advantage, fostering both customer satisfaction and loyalty.
Personalization Techniques
Personalization has become a vital aspect of customer engagement. Businesses increasingly leverage data analytics for tailored customer experiences. With advanced algorithms, companies can create personalized marketing strategies based on customer preferences and behavior.
- Utilizing historical data, organizations can predict future needs, making offers timely.Demographic data also plays a critical role in customization.
- Engaging customers through channels they prefer enhances communication.
Furthermore, technology such as Artificial Intelligence (AI) will amplify personalization. Companies like Amazon and Netflix successfully utilize algorithms to recommend products that resonate with individual tastes. Ultimately, embracing personalization is not merely about differentiation; it is about forging lasting relationships with customers.
Real-time Analytics
Real-time analytics emerges as a game-changing trend in customer analytics. Increased connectivity and data processing capabilities allow businesses to access information as it emerges. This immediacy enables efficient decision-making processes and enhances responsiveness to market changes.
- Companies can quickly identify trends, get insights into customer interactions, and optimize internal operations based on real-time feedback.
- On platforms such as Facebook, user engagement data is analyzed instantly, allowing brands to adjust their outreach strategies on the fly.
A significant benefit of real-time analytics is the enhancement of customer service. For instance, organizations providing support can address customer concerns as they arise, ultimately fostering a more loyal customer base. As real-time analytics continues to gain traction, it will undeniably redefine how businesses understand and serve their customers effectively.
Greatly understanding news trends within the customer behavior can lead to more ingrained strategies that promote long-term success.
Ending
The significance of the conclusion in this article lies in its ability to synthesize the vast landscape of customer analytics into clear insights. It serves as an essential component, tying together various themes and points discussed while highlighting their interconnections. Understanding customer analytics is crucial for companies seeking to leverage data for strategic advantages.
Customer analytics is a tool that speaks to enhancing overall business function, improving customer relations, and positively affecting the bottom line. Organizations must grasp how customer data influences decisions in various business environments. Furthermore, emphasizing the need for continual innovation and adaptation in customer analytics can be seen as a positive awakening for businesses aiming to stay relevant. Comprehending these points brings forth several benefits:
- Informed Strategic Planning: Knowledge of customer behavior informs product development and marketing strategies.
- Competitive Edge: Utilizing correct analytic methods allows organizations to position themselves favorably against competitors.
- Increased Customer Loyalty: An improved experience based on analytics fosters customer loyalty and retention.
- Operational Efficiency: Analytic insights can significantly elevate operational effectiveness.
As one integrates customer analytics into their business framework, there are increasing steps to acknowledge and navigate:
- Organizations must prioritize data management for precision.
- Businesses need to maintain transparency regarding analysis operations to build consumer trust.
- Continuous education on changing technological landscapes is needed given the rapid pace of shifts in customer data analyses.
Critical engagement with the data collected transforms struggles of interpretation into actionable items that can spark innovation and ultimately contribute to sustained business success.
Thus, a robust understanding of customer analytics culminates in a significant organizational asset. However, firms ought to be vigilant about its practical applications to enhance not only the decision-making process but also the customer-centric ethos every business strives to embrace.
Summary of Key Points
In summary, customer analytics plays a pivotal role across various business disciplines. This conclusion recaps the essentials covered within the article:
- Definition and evolution are vital for contextual understanding;
- Importance addresses performance and strategic improvements;
- Components include essential processes influencing satisfactory outcomes;
- Techniques showcase evolving methodologies to optimize data insights;
- Challenges remind organizations of barriers to success;
- Applications extend across tangible fields to affect overall performance.
These key elements synthesize into a coherent understanding that its wide acceptance reflects its true importance in business viability.
Final Thoughts on Customer Analytics
Final reflections point to customer analytics being more than a semiannual check-up but rather a constant evaluation approach for businesses aiming for customer-centric excellence. Organizations have the power to transcend mere transactional relations and develop sustained interactions through meticulous analysis of information. Furthermore, the future of customer analytics holds immeasurable promise as technologies like artificial intelligence further enhance recommendations and targeted customer interactions.
Adapting each analytical step can culminate in improved business framework efficiency while affecting consumer trust profoundly. For IT professionals, wanting to discover the nuances of advanced analytics requirements continues adding valuable dimensions to their operational pragmatic approaches, keeping organizations on the cutting edge.
Ultimately, to shoot for progressively leaning operational formats doesn’t merely represent knowledge mastery; it signifies readiness to mold the uptake in evolving channels, thus widening the strategic forefront where informed choices rule business success.
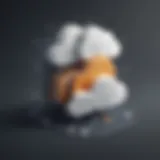
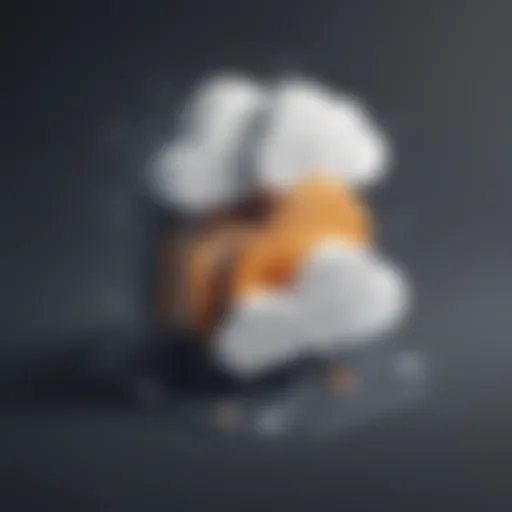