Data Cleansing: Importance and Effective Strategies
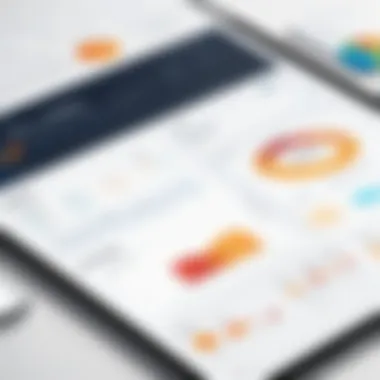
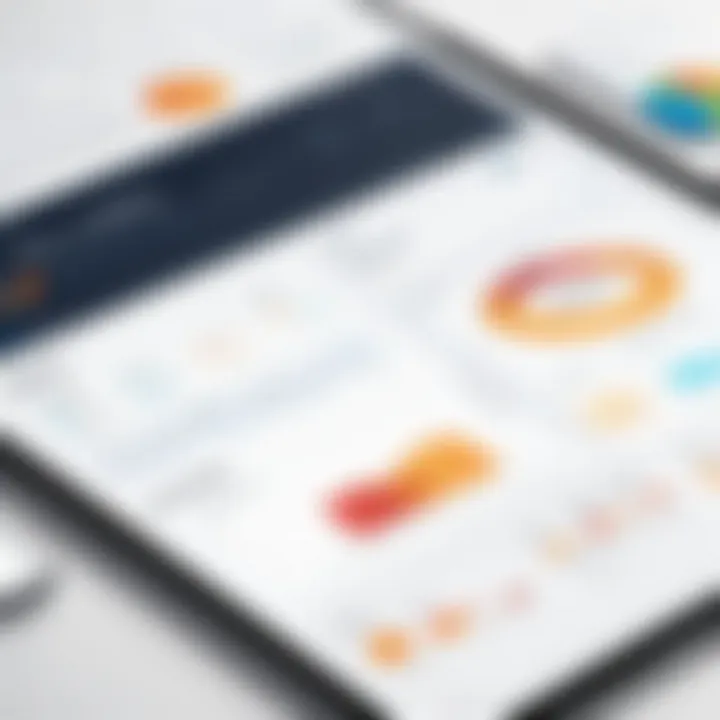
Intro
Data cleansing, often considered the unsung hero in the data management process, holds paramount importance in today’s data-driven world. It’s not merely about throwing out the bad apples in the barrel; it’s about enriching the entire harvest. Inaccurate data can lead organizations down a winding road of poor decision-making, inefficiencies, and lost opportunities. Meanwhile, clean data serves as a solid foundation for business intelligence, enabling organizations to uncover valuable insights and make informed choices.
The significance of data cleansing goes beyond simple tidying-up; it’s about establishing a culture of data integrity that permeates every layer of an organization. When employees trust the data they’re working with, it fosters confidence in decision-making processes and promotes a stronger organizational performance.
In this guide, we’ll dive into an array of strategies for efficient data cleansing. From recognizing common data quality issues to understanding the tools available for the task, this article aims to provide comprehensive knowledge that equips IT professionals, cybersecurity experts, and students alike. As we explore this subject, readers will understand not just the how, but the why, illuminating the path toward intelligent data management.
Understanding Storage, Security, or Networking Concepts
To grasp the full scope of data cleansing, it's essential to understand its relationship with storage, security, and networking concepts. In an era where data breaches and misinformation are rampant, organizations must prioritize the cleanliness and security of their data while also ensuring effective storage solutions.
Key Terminology
Before moving further, let’s clarify some key terminology that will be beneficial:
- Data Quality: The state of the data regarding its accuracy, completeness, reliability, and relevance.
- Duplicate Data: Instances of the same record appearing multiple times in a dataset, often leading to confusion in analytics.
- Data Validation: The process of ensuring that a dataset meets the required quality standards before it is processed or analyzed.
Overview of Important Concepts and Technologies
Data is often stored in databases, cloud storage, and data lakes. Understanding how these systems manage data is crucial.
- Databases: Relational databases, like MySQL and PostgreSQL, utilize structured query language (SQL) to manage data integrity and quality.
- Cloud Storage: Solutions like Amazon S3 and Google Cloud Storage enable organizations to store and retrieve data flexibly.
- Data Lakes: These allow large amounts of unstructured data to be stored in its native format and can be cleansed when accessed.
Best Practices and Tips for Data Cleansing
While the need for data cleansing is clear, effective strategies to implement it are equally essential. Here are some best practices to consider:
- Automate Where Possible: Utilize tools such as Talend or Alteryx to automate repetitive cleansing tasks.
- Regular Audits: Conduct regular audits of data quality to identify issues before they escalate.
- User Training: Ensure employees understand the importance of data hygiene and how their actions can affect data quality.
Industry Trends and Updates
Staying updated on industry trends is crucial for professionals to remain agile in their data management strategies. Some noteworthy trends include:
- The increasing adoption of AI and machine learning to enhance data cleansing processes.
- The rise of decentralized storage solutions that claim to help in reducing data redundancy and improving quality.
Case Studies and Success Stories
Here are a few enlightening cases that demonstrate successful data cleansing:
- Retail Chain: A leading retail chain implemented an automated system to cleanse its customer database, reducing duplicate entries by over 60%.
- Healthcare Provider: After adopting robust data validation techniques, a major healthcare provider improved patient outcome analytics, recognizing critical care needs more accurately.
"Ensuring data integrity isn't just a task; it's a commitment to excellence in decision-making."
Reviews and Comparison of Tools and Products
Various tools are available for data cleansing. Here’s a glimpse:
- Data Ladder: Offers data matching and cleansing features that are user-friendly and accessible.
- Informatica: Provides a comprehensive suite for managing data quality and cleansing across multiple platforms.
With so many options on the market, evaluating which tool best meets your organization's needs requires careful consideration of features, ease of use, and integration capabilities.
By embarking on this journey to understand data cleansing, organizations can ensure their data remains an asset rather than a liability, securing a foundation upon which informed decisions can be built.
Defining Data Cleansing
Data cleansing, at its core, is the practice of identifying and rectifying errors and inconsistencies in a dataset. It's an essential operation for any organization, especially those that rely heavily on data for decision making. Without proper data cleansing, the insights derived from data can lead organizations down the wrong path, much like trying to navigate a ship using outdated charts.
Basic Concepts
The foundation of data cleansing revolves around several key concepts. Usually, people think of it merely as removing duplicates or correcting misspellings, but there’s much more to the picture. Data cleansing engages processes such as:
- Error Correction: Fixing inaccuracies caused by human error or system glitches.
- Normalization: Standardizing formats so that data from different sources can harmonize seamlessly, like tuning various instruments to play one symphony.
- De-duplication: This is akin to cleaning house—getting rid of unnecessary duplicates to create a lean, efficient dataset.
A common saying in the data world is, "garbage in, garbage out." This highlights that if data is flawed from the outset, any analysis or reporting that comes from it is equally flawed, leading to misguided strategies and poor business outcomes. Thus, a robust understanding of these concepts is paramount.
Importance in Data Management
Now, let’s delve into why data cleansing holds significance in data management. Imagine trying to build a house on a shaky foundation—all subsequent structures would be compromised. In data management, this shaky ground can be the quality of data. Data cleansing tames the chaos that often pervades raw data, bringing forth order and clarity that organizations desperately need.
When data is cleansed effectively, several benefits emerge:
- Increased Accuracy: Reliable data leads to accurate analytics. Without it, predictions based on flawed data are risky at best, fatal at worst.
- Improved Efficiency: Streamlined datasets simplify the processes across departments. It’s like clearing a congested roadway, enabling smoother traffic flow.
- Enhanced Compliance: Regulations often require adherence to specific guidelines regarding data accuracy. By investing time in data cleansing, organizations cushion themselves against potential legal issues.
In essence, data cleansing allows for more informed decision-making by ensuring that the fundamental building blocks—data—are correct and consistent. As we navigate further into the depths of data management, recognizing this importance can steer many organizations toward success. As stated in Data Management 101, "quality data is a business asset; poor data is a liability."
"Data cleansing turns chaos into clarity, allowing organizations to see the world more clearly and make wiser decisions."
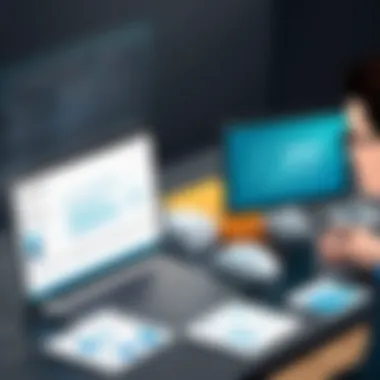
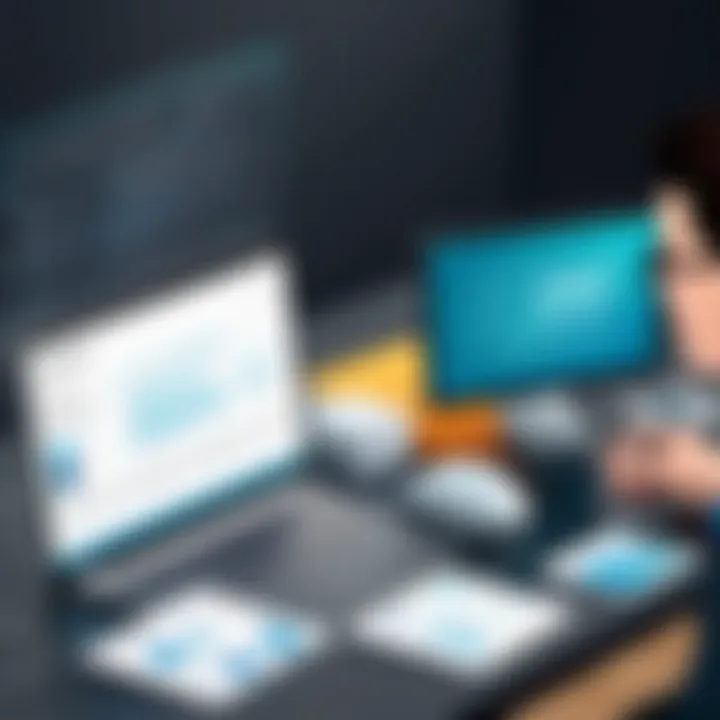
Core Objectives of Data Cleansing
Data cleansing, often seen as a necessary evil, has some core objectives that organizations cannot afford to overlook. Engaging in data cleansing not only repairs trends in dirty data but turns it into a strategic asset. The following sections break down these objectives into two fundamental aspects: improving data quality and enhancing decision-making.
Improving Data Quality
In the fast-paced world of technology, the significance of improving data quality can't be overstated. High-quality data serves as the backbone of successful operations, guiding strategies and actions. Organizations need to ensure their data is accurate, consistent, and trustworthy.
By focusing on data cleanliness, businesses can avoid pitfalls that arise from faulty information. For instance, when a dataset contains inaccuracies, it tends to mislead stakeholders, which can lead to ineffective strategies and wasted resources. An example of this would be a marketing team relying on outdated customer information, resulting in campaigns that misses the mark completely.
Moreover, tackling redundancies within datasets helps prevent confusion and ensures unique entries for vital records. The time investment in cleaning data translates into enhanced productivity. - Less time spent tackling errors means personnel can focus on more pressing tasks.
Therefore, prioritizing data quality through cleansing activities not only provides immediate benefits but also establishes a robust framework for future data handling. Quality data supports the foundation of business intelligence strategies while safeguarding customer trust and relationships.
Enhancing Decision-Making
The second core objective of data cleansing revolves around enhancing decision-making across various levels of an organization. Good data translates directly into sound decision-making. When leadership relies on high-quality data, they can make informed choices that drive the organization forward.
When faced with decisions about resource allocation, product development, or market expansion, having solid data is like having a compass in uncharted waters. Inaccurate data can mislead executives into making poor decisions, risking financial losses and reputational damage. For example, if a company uses flawed sales data to forecast future income, it may overestimate financial health, leading to impulsive and dangerous investment choices.
Moreover, quality data enhances operational efficiency. A well-maintained dataset provides real-time insights, allowing teams to react swiftly to market demands. Decisions based on current and precise data can lead to reduced risks and more effective strategies.
To sum up, understanding the core objectives of data cleansing—improving data quality and enhancing decision-making—is crucial for any organization striving for excellence. With reliable data at their fingertips, teams can sidestep ambiguity and confidently navigate the ever-changing market landscape.
"Data quality is fundamentally linked to the strategic success of an organization. Poor data leads to poor decisions, which can have disastrous consequences."
By embracing a culture of continuous data cleansing, businesses will not only meet today's challenges but will also position themselves advantageously for tomorrow's opportunities.
Common Data Quality Issues
In the realm of data cleansing, understanding common data quality issues is paramount. When organizations fail to recognize and address these issues, they risk making decisions based on flawed information. Consequently, problems can escalate across departments, disrupting business operations and leading to a loss of trust in data-driven decisions. The necessity of identifying these challenges cannot be overstated. Thus, let's explore three critical categories of data quality issues: inaccuracies, redundancies, and inconsistencies.
Inaccuracies
Inaccuracies in data represent a significant setback for any organization. These can stem from various sources, including human error during data entry, technical glitches, or outdated information. For instance, imagine a sales team relying on a customer database where addresses have not been updated. If delivery personnel attempt to ship products to incorrect locations, not only does this cost money, but it also tarnishes customer relations.
"Inaccurate data can skew reporting and analytics, leading to misguided business strategies."
To combat inaccuracies, it is vital to establish validation processes. Automated systems can be designed to flag anomalies in data inputs, and regular updates should be scheduled to ensure that the information is current.
Redundancies
Redundancies occur when the same piece of information is stored multiple times across a system. This often happens when data is collected from different sources without proper integration. For example, in a healthcare setting, if patient records are duplicated in multiple databases, it can lead to conflicting treatment histories, potentially compromising patient care.
To tackle redundancies, organizations should implement deduplication strategies, which may include automated scripts or manual processes to merge duplicate entries. It's not just about cleaning; it’s about turning a tangled mess of data into a streamlined resource.
Inconsistencies
Inconsistencies refer to discrepancies within datasets that can arise from different formatting or definitions. Think about a scenario where one database uses "NY" to denote New York, while another uses the full state name. Such inconsistencies can create confusion, especially when aggregate reports are generated or when systems are integrated.
To mitigate inconsistencies, a standardization process becomes essential. Organizations should develop common guidelines for data entry and ensure that all teams adhere to these rules. Training sessions can serve as a great way to instill the importance of data consistency in everyday tasks.
In summary, addressing inaccuracies, redundancies, and inconsistencies in data is not merely an exercise in tidiness. Each has distinct implications for decision-making and operational efficiency. Ignoring these common data quality issues can lead to severe downstream effects, emphasizing the need for meticulous data management strategies.
Steps in the Data Cleansing Process
Data cleansing is not just a quick fix for data issues; it’s a meticulous process that can mean the difference between navigating smoothly through business challenges or getting stuck in a quagmire of bad information. Engaging in this process meticulously allows organizations to maintain high data quality, thus ensuring that decisions made based on this data are sound and reliable.
Data Profiling
Data profiling serves as a starting line in the cleansing race. This is where the data's characteristics are analyzed to understand its quality and current state. By examining the data's structure, consistency, and content, organizations can uncover various hidden issues that need to be addressed. For instance, it might not just be about finding missing values; it could involve understanding whether there are unusual patterns that could signify errors in data entry or even malicious tampering.
Benefits of Data Profiling:
- Identifies anomalies: Helps in revealing unexpected patterns in datasets.
- Informs strategy: Provides insight for prioritizing data quality initiatives.
- Sets benchmarks: Establishes a baseline for ongoing data quality assessment.
Data Deduplication
In the digital age, redundancy can sneak into your databases like an unwanted squatter. Data deduplication tackles this by identifying and removing duplicate entries from your datasets. This step is crucial for ensuring that analysis made from these records reflects true and singular accounts rather than inflated ones.
In practical terms, imagine having multiple addresses for the same customer due to input inconsistencies; that’s just one example. Consolidating these records leads to clearer insights, and usually, a more enjoyable UX for both users and analysts alike.
Validation Techniques
Validation techniques check the data against predefined rules or standards. Think of it as having a set of bouncers at the door of your data club: if the data doesn't meet the established criteria, it doesn’t get in. This could include ensuring that all email addresses follow a standard format or that all numerical entries lie within set ranges.
Implementing these techniques helps in catching issues early and can save organizations from costly mistakes further down the line.
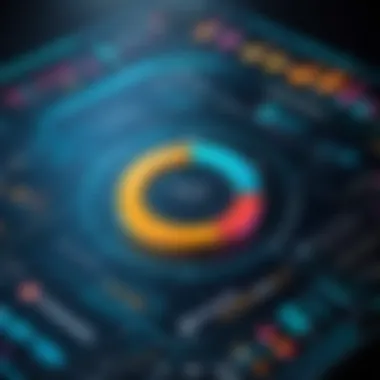
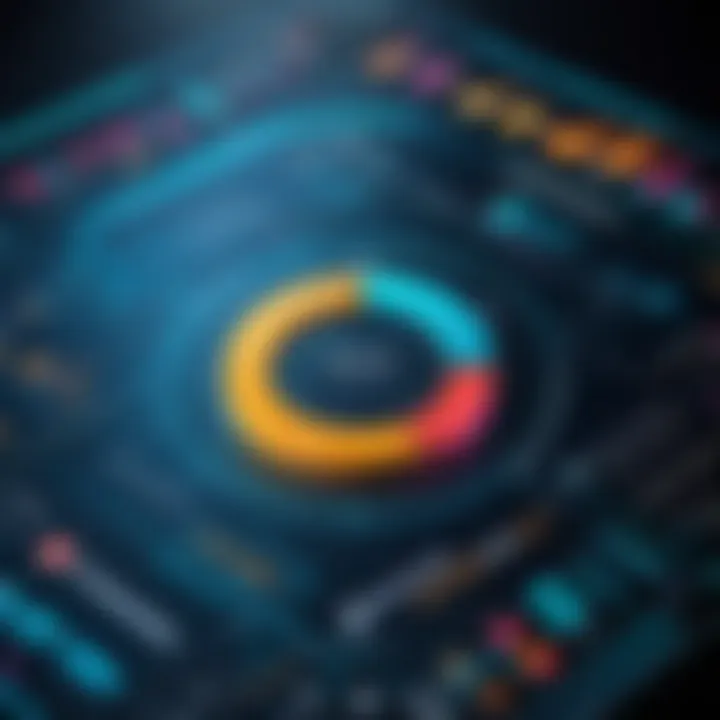
"By proactively validating data at the entry points, organizations can significantly reduce the occurrence of future data quality issues."
Enrichment and Standardization
Once data has been cleaned and validated, enrichment and standardization come into play. This involves enhancing the data by adding missing information and ensuring that all entries are in a consistent format. Standardization addresses various aspects such as naming conventions or units of measure. For example, if one dataset refers to 'USD' while another records it as '$', converting them to a common standard facilitates more effective analysis.
This step not only boosts the overall quality of the data but also enriches it, making it more useful for generating meaningful insights.
In summary, the steps in the data cleansing process are foundational for maintaining a high level of data integrity. Each step contributes to improving data quality and aligning it with business needs. When organizations invest time and resources into these processes, they create a formidable defense against the pitfalls of poor data.
Tools and Technologies for Data Cleansing
In today's data-driven environment, the significance of effective tools and technologies for data cleansing cannot be overstated. As organizations grapple with vast amounts of information, these tools serve as the backbone for maintaining high data quality, enabling companies to make informed decisions. The right technology assists in identifying and rectifying numerous issues, ranging from simple inaccuracies to complex inconsistencies, ultimately producing cleaner datasets crucial for analysis.
When businesses invest in data cleansing tools, they are purchasing more than just software; they're acquiring the means to enhance operational efficiency and boost productivity. Here's what to consider:
- Cost-effectiveness: Investment in good tools can save expense in the long run by reducing errors and improving project timelines.
- Scalability: Proper technologies can adjust to the growing amounts of data without overloading systems.
- User-friendliness: Tools should be accessible, ensuring users with varying levels of expertise can navigate them.
- Integration: Consideration must be made for how these tools can mesh with existing systems to streamline the workflow.
Software Solutions
A plethora of software solutions exist in the market aimed at effectively tackling data cleansing. Each tool brings a unique blend of features tailored to meet specific organizational needs. Here’s a look at some popular software which IT professionals trust:
- Talend: An open-source platform that provides robust solutions for data integration, often lauded for its user-friendly interface and vast capabilities.
- Informatica: It enables businesses to maintain data quality across various platforms, making data integrity a priority.
- Microsoft Power Query: A feature embedded in several Microsoft products, Power Query excels at transforming and shaping data.
The advantages of deploying a software solution go beyond merely automating processes. They also facilitate ongoing monitoring of data quality, enabling users to adjust their strategies in real-time.
Automated Scripts and Tools
Automated scripts represent a strategic approach to data cleansing, offering precise and replicable processes to address data quality issues quickly. By creating scripts to cleanse data, organizations can:
- Reduce Human Error: With automation, there's less room for error inherent in manual processes.
- Save Time: Automated scripts can run on a schedule, freeing up personnel to focus on analysis rather than routine tasks.
- Enhance Consistency: Scripts promote uniformity in data cleansing practices across different datasets, ensuring consistent outcomes.
Example of a simple Python script for data deduplication:
As shown here, even straightforward scripts can play a pivotal role in maintaining data quality by automating tedious tasks. This combination of software solutions and automated scripts nurtures not only data integrity but also a culture of proactive data management within organizations.
"In the world of data, quality often trumps quantity; investing in the right tools is essential for success."
In summary, the selection of suitable tools and technologies for data cleansing is a vital step toward ensuring high-quality data. The options range widely, and understanding their capabilities enables informed choices that can significantly impact a company's overall effectiveness.
Best Practices in Data Cleansing
Data cleansing is not just a one-off chore; it’s a continual process that significantly impacts an organization’s data quality. Implementing best practices plays a crucial role in smoothing out the wrinkles in data management. This section delves into two primary components that enhance the efficacy of data cleansing: establishing clear guidelines and performing regular data audits.
Establishing Clear Guidelines
Setting clear guidelines is like drawing a roadmap before embarking on a journey. Without direction, businesses can find themselves lost in a maze of data discrepancies. In defining these guidelines, several elements must be taken into consideration:
- Consistency: Data formats should be uniform across all databases. For instance, capturing dates in the format helps avoid confusion and errors in analysis.
- Validation Rules: Create specific rules that data must adhere to before it enters the database. For example, ensure only valid email formats are accepted, decreasing the chances of inaccuracies.
- Roles and Responsibilities: Clearly delineate who is responsible for which part of the data cleansing process. This prevents overlap and confusion within teams, enhancing accountability.
- Documentation: Maintaining comprehensive records on data sources, cleansing techniques, and procedures educates the team and serves as a reference point, making future data handling smoother.
A structured approach to generating these guidelines encourages a culture of discipline and diligence in dealing with data. It acts as a foundation upon which data integrity can flourish, leading to more accurate reporting and decision-making.
Regular Data Audits
Conducting regular data audits is another practice that should not be overlooked. Think of this step as taking your car in for routine check-ups; it might be running fine, but you never know when something might go awry. Periodic audits serve various purposes:
- Identify and Fix Issues: A well-planned audit allows teams to spot inaccuracies, redundancies, and inconsistencies that may have slipped through the cracks.
- Assessment of Data Quality: By assessing the existing quality of data, organizations can understand the effectiveness of their ongoing cleansing strategies and where they might need to improve.
- Adapt to Changes: Data environments are constantly shifting due to market changes, regulations, or business strategies. Regular audits enable organizations to adapt their data practices accordingly.
- Stakeholder Confidence: Regularly auditing data can provide stakeholders with assurance that the data being used for decision-making is reliable and robust. This increases trust and might influence investment or operational decisions.
Conducting a data audit is not merely an administrative task; it’s a strategic move to safeguard the integrity of business operations.
In essence, best practices like establishing clear guidelines and conducting regular audits create a safety net for organizations navigating the complex world of data. They foster an environment where data quality can thrive, thus influencing broader business intelligence and analytics efforts.
The approach goes beyond mere cleanup; it infuses a proactive culture around data quality, encouraging IT professionals and business analysts alike to treat data as a crucial asset rather than just a by-product of operations.
Challenges in Data Cleansing
In the realm of data management, the process of data cleansing is not as straightforward as wiping dust off a table. It carries with it a bouquet of challenges that organizations must navigate to ensure the integrity and usefulness of their data. Understanding these hurdles is essential for IT professionals, cybersecurity experts, and students if one hopes to achieve reliable data quality. The complexity involved can hinder progress, but recognizing these challenges is the first step to addressing them effectively.
Complexity of Data Sets
One of the primary challenges in data cleansing is the sheer complexity of the data sets that organizations handle. In today’s world, data does not come neatly packaged. It arrives in various formats—spreadsheets, databases, web services, and even user-generated content from platforms like Facebook or Reddit, creating a rich but chaotic tapestry.
- Diverse Sources: Data is often sourced from numerous channels, leading to inconsistencies. Data from a customer relationship management tool might not align with data from marketing analytics tools. Such discrepancies demand meticulous attention.
- Volume and Velocity: Organizations not only have to deal with large volumes of data but also with its velocity. Real-time data feeds can quickly become outdated, necessitating immediate cleansing efforts.
The intricate nature of this data landscape demands a keen eye and considerable skill in data profiling and analysis, which creates added workload for data managers. Failure to address the complexity may result in actionable insights being based on flawed data, steering decisions off course.
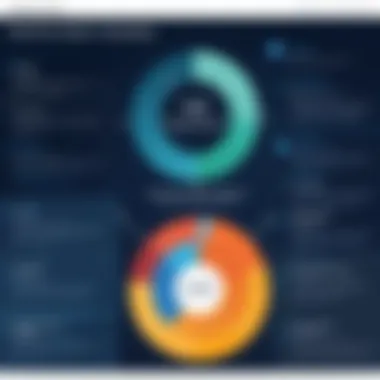
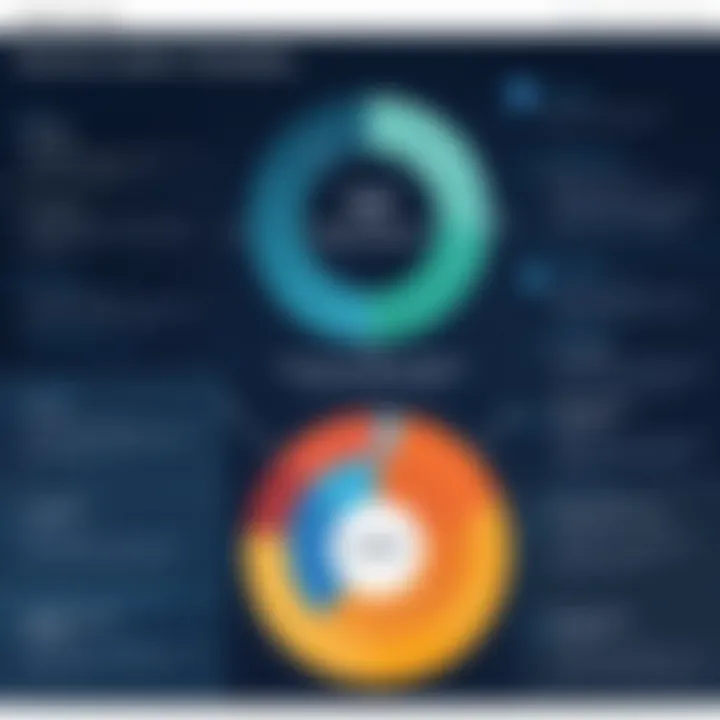
Resource Allocation
Another hurdle in effective data cleansing is resource allocation. It's a balancing act that often finds data teams juggling multiple priorities, each competing for time and funding.
- Budget Constraints: Many organizations operate under tight budgets, making it difficult to invest in advanced data cleansing tools or hire specialized talent. This often leads to individuals carrying heavy loads, stretching their skills too thin.
- Time Limitations: Tight project timelines can prompt staff to cut corners during the data cleansing process. "A rushed job is a jumbled job," as the saying goes. Never underestimate how this can snowball into bigger issues down the line.
Successful data cleansing requires a commitment of proper resources—both financial and human. Insufficient allocation can prevent data teams from providing the thorough cleansing that truly propels data insights, leading to undermined business objectives.
User Adoption and Training
Finally, user adoption and training constitute formidable challenges in the data cleansing arena. Even the most sophisticated tools and methodologies fall flat if the users are not on board.
- Change Resistance: Employees might resist new data practices, clinging to familiar (but outdated) methods. Changing mindsets is crucial for improvement, but it can be a tough nut to crack. Training sessions may be met with eye rolls or resistance, particularly if personnel feel overwhelmed.
- Skill Gaps: Not all users come equipped with the technical knowledge necessary for effective data management. Organizations must invest in ongoing training to bridge these gaps and cultivate a data-aware culture.
In summary, overcoming challenges in data cleansing is about much more than just applying techniques. It involves recognizing the complexity of data sets, ensuring adequate resource allocation, and fostering user adoption through training. An organization that tackles these challenges head-on can achieve superior data quality and thereby glean truly insightful analyses.
The Role of Data Cleansing in Business Intelligence
Data cleansing stands as a cornerstone for effective business intelligence. In a world overflowing with data, just having a pile of numbers and figures isn't enough. Businesses need clarity, accuracy, and relevance. That's where data cleansing struts onto the stage. This process plays a fundamental role in shaping business intelligence strategies and ensuring that organizations can make informed decisions wholeheartedly.
The benefits of data cleansing in business intelligence are manifold:
- Enhanced Accuracy: Data cleansing ensures that the information you analyze is correct and reliable. This accuracy means fewer mishaps in reporting and, consequently, better decision-making.
- Consistent Data: Data can come from various sources, each with its quirks and inconsistencies. Cleansing it helps standardize these different data sets, making the analysis process smoother and more coherent.
- Increased Efficiency: Organizations can save countless man-hours when they maintain clean data. Time spent correcting errors is time that could be better used for strategic planning and analysis.
- Better Compliance: For industries that must abide by regulators, maintaining clean data is non-negotiable. Data cleansing helps organizations adhere to compliance requirements, thereby avoiding hefty penalties.
Without proper data cleansing, an organization might find itself navigating through a maze of errors, dragged down by duplicates, entry mistakes, and outdated information. This muddled data can distort reporting and analytics, leading businesses to make poor decisions.
"Garbage in, garbage out. This old adage rings true when it comes to data management. If the data is flawed, the insights derived from it are equally misleading."
Impact on Reporting
When it comes to reporting, clean data can make or break an organization's ability to glean useful insights. Well-structured, accurate data enables organizations to produce reports that reflect the genuine state of affairs, without fluff or misleading figures. An accurate report can:
- Drive Accountability: Managers and teams can be held accountable for their performance when reports are based on clear, cleansed data.
- Facilitate Transparency: Stakeholders can trust the resources they access, ensuring that vital decisions draw on factual information.
- Support Forecasting: Trend analyses become more precise when data is cleansed, leading to better predictions and proactive strategies.
On the contrary, if the data isn't clean, reporting could lead to misguided conclusions. Poorly managed reports can create confusion, mistrust, and possibly lead to a cascading failure in the decision-making process.
Supporting Data Analytics
Data cleansing also significantly supports data analytics efforts. In the analytical sphere, practitioners rely heavily upon the quality of data to derive insights. When the data is cleansed, analytics yield:
- Precise Insights: High-quality analytics stem from clean data. The accuracy of conclusions drawn from analytics directly correlates with data integrity.
- Improved Predictive Modeling: When data problems are ironed out, predictive models can function at their best, giving organizations insights that can shape strategic initiatives.
- Actionable Recommendations: Cleansed data not only uncovers trends but also aids in making specific recommendations that can optimize business processes.
In summary, the symbiotic relationship between data cleansing and business intelligence cannot be overstated. Organizations that prioritize data hygiene stand to reap the rewards in efficiency, accuracy, and decision-making prowess. Hence, the journey of data cleansing is a critical path to illuminating the foggy terrain of business intelligence.*
Future Trends in Data Cleansing
The landscape of data cleansing is rapidly evolving, driven by technological advancements and the ever-increasing volume of data. As businesses strive to harness the power of accurate, high-quality data, they must stay ahead of the curve. Understanding future trends in data cleansing is essential not only for maintaining data integrity but also for ensuring that organizations can make informed decisions that lead to strategic success. Here, we delve into the emerging trends shaping the future of data cleansing.
Artificial Intelligence and Machine Learning
Artificial intelligence (AI) and machine learning (ML) are revolutionizing various fields, and data cleansing is no exception. These technologies facilitate the automation of processes that were once time-consuming and error-prone, enhancing the efficiency of data management.
- Enhanced Accuracy: AI algorithms can identify and correct inconsistencies within data sets with greater precision than traditional methods. The iterative learning process allows these algorithms to improve over time, thereby increasing the reliability of data cleansing efforts.
- Real-Time Cleansing: With the integration of machine learning, organizations can achieve real-time data cleansing. This capability means that as data is ingested, it is immediately analyzed for quality, allowing businesses to act promptly rather than after the fact.
- Predictive Analytics: By employing AI, companies can not only cleanse data but also predict potential data quality issues before they arise. This proactive approach helps in mitigating risks associated with poor data quality, ultimately leading to better decision-making.
Adapting to these AI and ML innovations does require investment in skills and resources, but the outcomes often far surpass initial challenges. Organizations must focus on training staff to work effectively alongside these technologies.
Integration with Big Data Technologies
As big data continues to expand the horizons of data analysis and processing, its integration with data cleansing processes is becoming increasingly vital. The challenges presented by big data—volume, velocity, and variety—all necessitate more sophisticated data cleansing methods.
- Scalability: One of the primary benefits of integrating big data technologies is the ability to scale data cleansing operations. Tools designed for big data, such as Apache Hadoop or Spark, can handle vast amounts of information and perform cleansing operations more efficiently than traditional systems.
- Data Lakes and Warehousing: Companies often utilize data lakes for storage due to their flexibility. However, this can lead to chaos without proper cleansing protocols in place. Thus, the interplay between data lakes and cleansing strategies must be carefully managed to ensure that they serve analytical purposes effectively.
- Cross-Platform Compatibility: Big data systems often utilize different storage formats and structures, creating potential for discrepancies. Effective data cleansing must embrace the compatibility of various platforms to harmonize data across the board, promoting a unified view of information.
"In the era of big data, the quality of your inputs directly influences the quality of your outcomes. Embracing advanced data cleansing techniques is not just a priority, it's a necessity for long-term success."
Organizations must keep a watchful eye on these trends, as they are pivotal in shaping a future where high-quality data becomes the standard rather than the exception.
Epilogue: The Essentiality of Data Cleansing
In the fast-paced digital landscape we navigate today, organizations can't affor to overlook the necessity of data cleansing. This process does not merely remediate data inaccuracies; it serves as the foundation upon which sound business strategies and insights are built. With the growing volumes of data generated every day, the importance of maintaining data quality cannot be overstated. Poor data quality can lead to misguided decision-making, wasted resources, and ultimately, significant losses for businesses.
The vitality of data cleansing lies in its capability to enhance the overall quality of data that organizations use. High-quality data directly influences an organization’s performance and its ability to respond to market dynamics. In short, data cleansing is not an optional extra; it’s a crucial investment. Organizations often witness long-lasting advantages when they invest in a robust data cleansing strategy. From heightened operational efficiency to improved customer satisfaction, the benefits effect not just the immediate context but ripple through to long-term success and profitability.
Long-Term Benefits for Organizations
The long-term benefits of data cleansing are plentiful. Here are a few crucial points:
- Enhanced Decision Making: Clean data ensures that decisions are made based on accurate and reliable information, reducing the risks of errors that could have costly repercussions.
- Cost Efficiency: By eliminating redundancies and inaccuracies, organizations can save money over time. It’s like cutting the fat off a steak—you get more substance for less waste.
- Better Customer Relationships: Clean data allows companies to understand their customers more effectively. Personalized experiences become a reality when businesses have access to neat and complete datasets.
- Regulatory Compliance: Organizations must comply with various data regulations, and a clean dataset helps them stay within legal guidelines while avoiding potential fines.
"Clean data is not just about accuracy; it’s about making informed decisions that shape the future of an organization."
Final Thoughts on Data Quality
In summary, data quality is not just a technical concern but a strategic one. Organizations must prioritize data cleansing as a continual practice rather than a one-off project. It’s a bit like keeping up with your car maintenance; if you ignore those little check-ups, you may find yourself in a heap of trouble down the line.
In the world of data management, adopting a proactive approach to data cleansing can mean the difference between thriving and simply surviving. As we move further into an era where data reigns supreme, establishing a culture of continuous data quality improvement should be top-of-mind for anyone involved in data management. Building systems that intrinsically support data quality will set organizations on the path to sustainable success as they journey through their unique data landscapes.