Understanding Graphical Analytics: Trends and Techniques
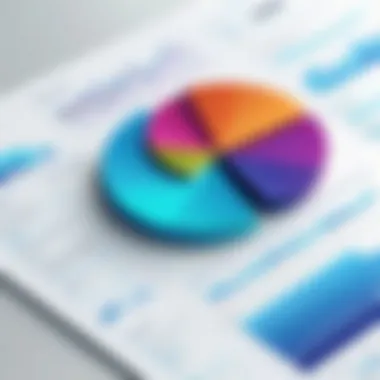
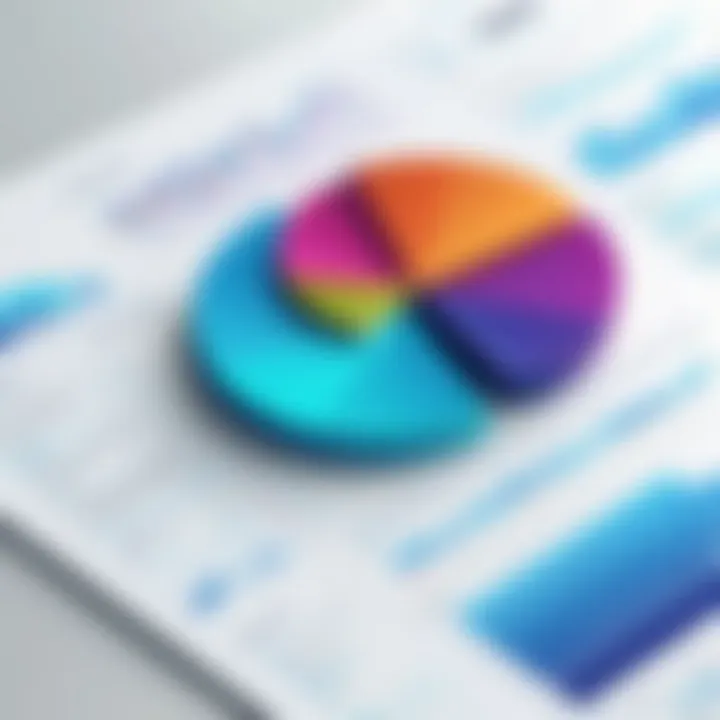
Intro
Graphical analytics emerges as a crucial intersection of data analysis and visualization. It acts as a powerful conduit for making sense of complex datasets. With vast amounts of data generated daily across various sectors, businesses face a pressing need for tools that not only capture this data but also render it intelligible and actionable.
Data visualization is more than just a trend; it has become an essential component in decision-making processes. A well-designed graph or chart can reveal insights that are not immediately obvious in raw data formats. Stakeholders and decision-makers depend on these visuals to identify patterns, trends, and potential areas of concern.
This article ventures into various aspects of graphical analytics. It examines the fundamental methodologies at play, the tools leading the field, and their applications across industries. Moreover, it strives to present real-world examples demonstrating the transformative power of effective graphical representations.
We aim to discuss key trends shaping the landscape of graphical analytics, thereby enabling readers to grasp the innovations that are setting the pace in this dynamic domain. Ultimately, this exploration seeks to enhance understanding of how graphical analytics can facilitate informed decisions and strategic planning in the contemporary data-driven environment.
Intro to Graphical Analytics
Graphical analytics serves as a pivotal tool in today’s data-driven landscape. As organizations and individuals consistently generate vast amounts of data, the ability to visualize these datasets greatly contributes to informed decision-making. This section introduces the key concepts and contextualizes the relevance of graphical analytics in various fields.
Definition and Scope
Graphical analytics refers to the process of employing visual representations of data to identify patterns, trends, and insights that drive strategy and operations. It encompasses a range of techniques, including charts, graphs, and interactive dashboards. The scope of graphical analytics is broad, affecting sectors like healthcare, finance, and marketing. These fields leverage visualization to understand complex data swiftly.
Some key characteristics include:
- Interactive Data Exploration: Allows users to manipulate visuals for deeper insights.
- Real-time Analysis: Facilitates quick adjustments based on incoming data.
- Enhanced Communication: Simplifies the sharing of insights with stakeholders who may not have a technical background.
The definition of graphical analytics emphasizes its role in translating complex datasets into comprehensible visual formats. This translation is essential in highlighting significant trends or anomalies that directly influence business strategies and outcomes.
Historical Context
The concept of graphical analytics did not develop overnight. Early forms of data visualization can be traced back to the work of pioneers like William Playfair in the 18th century. He introduced statistical graphics through bar and line charts. Over time, the evolution of technology led to more sophisticated tools and techniques.
The digital age brought new possibilities:
- Introduction of Computers: Computation made real-time data visualizations possible, shifting how analysts viewed data.
- Internet and Data Sharing: Easier access to vast datasets and collaborative tools enabled more stakeholders to engage with data.
- Emergence of Data Science: The intersection of statistics and computer science gave rise to specialized fields focusing heavily on analytics and visualization.
Graphical analytics has evolved alongside advancements in technology, reflecting larger trends in data management and usage. By understanding this historical context, readers can appreciate the importance of graphical analytics today. It is an essential element of modern data analysis, shaping how industries interpret and utilize information.
The Importance of Data Visualization
Data visualization emerges as a pivotal component in the realm of graphical analytics. This section explores why effective data visualization is crucial for understanding complex information. It examines its multifaceted benefits and critical considerations that enhance both communication and comprehension.
Enhancing Understanding
Data visualization translates data into visual formats, like graphs and charts, making intricate patterns accessible. Humans process visuals more quickly than text. When data is presented visually, it aids in identifying trends and outliers. This clarity allows users to comprehend large datasets without becoming overwhelmed.
Moreover, effective visual representation can clarify significant insights hidden within data. This becomes important, especially in disciplines like health and finance, where data instances might carry critical implications for decision-making. By displaying data effectively, users can grasp context, meaning, and relationships more swiftly.
The goal is to make information digestible. Studies show that visuals lead to a higher retention rate of information. Thus, enhancing understanding through graphical analytics is not merely beneficial; it is fundamental.
Facilitating Decision Making
Effective data visualization is more than a tool for understanding; it plays a vital role in decision-making processes. Decisions rely on clear insights drawn from data. When data is visualized effectively, stakeholders can make informed decisions based on the presented facts.
For instance, real-time dashboards allow management to monitor critical metrics instantly. When data is easy to interpret, decisions can be made quickly, which is particularly valuable in fast-paced environments such as finance and cybersecurity.
Furthermore, engaging visuals can support presentations to stakeholders, fostering collaboration. They can illustrate complex points succinctly to various audiences without losing detail. This cooperation leads to enhanced transparency and collective understanding among team members.
"Without data, you're just another person with an opinion."
In summary, the importance of data visualization is underscored by its capacity to enhance understanding and facilitate decision-making. These aspects contribute significantly to the effectiveness of graphical analytics across different sectors. As data continues to grow in complexity, the necessity for visual comprehension will become even more crucial.
Key Principles of Graphical Analytics
Graphical analytics stands as a critical methodology that combines statistical insight with the art of visual representation. Its principles serve as guiding factors for creating effective and meaningful visual data narratives. As organizations increasingly recognize the influence of visual data on decision-making, understanding these principles becomes essential in maximizing impact. Each principle—data integrity, appropriate use of visuals, and scalability and adaptability—plays a vital role in ensuring that visual analytics contribute positively to insights and actions.
Data Integrity
Data integrity is the foundation of any successful graphical analytics endeavor. It refers to the accuracy and consistency of data throughout its lifecycle. Without high-quality data, the process of visualization may not yield reliable results. When creating visual representations, it is paramount to ensure that data is sourced from credible databases and has been cleaned to remove any anomalies or errors.
Often overlooked, data integrity can cause significant issues if neglected. If faulty data leads to inaccurate visualizations, the decisions based on these visuals can be flawed. Thus, always verifying the validity of data is a must.

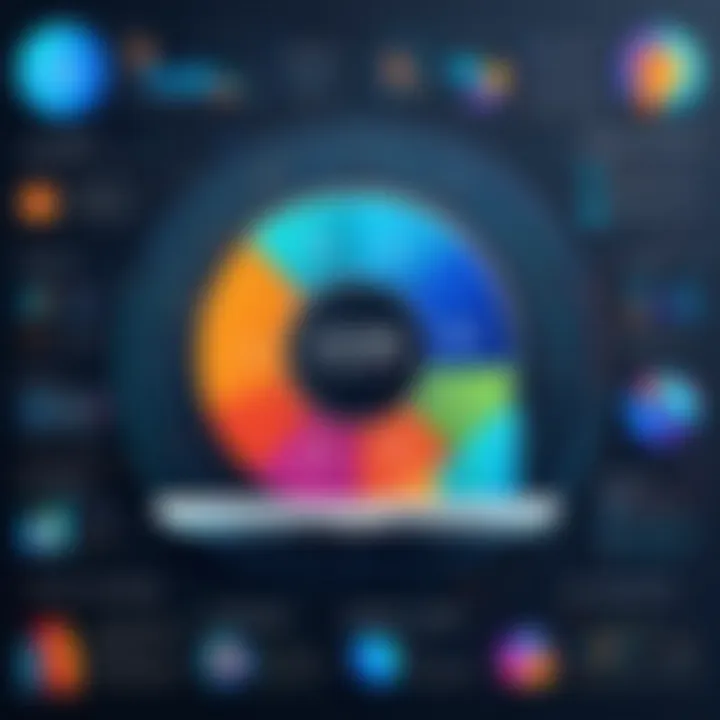
Here are some important aspects to consider regarding data integrity:
- Accuracy: Ensures that the data correctly reflects the real-world scenario it represents.
- Consistency: Guarantees that the same data can produce the same results under varying conditions.
- Completeness: Ensures that all necessary data points are present for a comprehensive view.
"Data integrity is critical in establishing trust and actionable insights in any analytics process."
Appropriate Use of Visuals
The appropriate selection of visuals is paramount in conveying information clearly. Different types of visuals serve different purposes and, when used effectively, can highlight trends, patterns, and anomalies in data that might not be immediately apparent in raw data sets. For instance, bar charts may be ideal for comparing quantities, while line graphs effectively showcase changes over time.
Consider the following factors when choosing visuals:
- Target Audience: Understand who will interact with the visuals. Technical audiences might prefer detailed graphics, while general audiences may require simpler visual formats.
- Data Type: The nature of the data influences the choice of visuals. Categorical data often works well with pie charts, while continuous data pairs nicely with line or scatter plots.
- Context and Purpose: Recognizing why and how the data will be used helps guide the selection. Visuals should serve to elucidate rather than obscure the message.
Scalability and Adaptability
As data volumes continue to grow, the principle of scalability and adaptability in graphical analytics becomes crucial. Visualization tools and techniques must be designed to handle increasing data sizes without degrading performance or clarity. This adaptability ensures that as new data streams are introduced, they can be integrated into existing visual frameworks without disruption.
Here are some considerations:
- Dynamic Updates: Visualizations should be able to refresh automatically as new data becomes available, keeping insights current.
- Multi-Platform Support: Engaging visuals should function across various devices and platforms, catering to diverse user preferences.
- Customization: The ability to modify graphics for different contexts or audiences allows for enhanced clarity and relevance.
Common Techniques in Graphical Analytics
Graphical analytics relies heavily on various techniques that transform data into visual formats. These techniques are crucial because they provide clarity, making complex data more understandable and actionable. The value of graphical representations lies not just in displaying data but in facilitating insights and decision-making. Here, we will explore some of the most common techniques used in graphical analytics and understand their specific applications and benefits.
Bar Charts
Bar charts are among the simplest yet most effective graphical tools. They display categorical data with rectangular bars, where the length of each bar is proportional to the value it represents. This format allows for quick comparisons between categories, making trends and differences apparent at a glance.
Benefits:
- Clear Comparisons: Bar charts make it straightforward to compare data across different categories.
- Versatility: They can represent various types of data, including counts, averages, or percentages.
- Ease of Understanding: Even non-technical audiences can interpret bar charts easily.
Considerations:
- Limit on Categories: Too many categories can clutter the chart and hinder readability.
- Scale: Ensuring the scale is accurate is critical to avoid misleading representations.
Line Graphs
Line graphs are particularly useful for showing trends over time. They plot data points on a Cartesian plane and connect them with lines. This technique is best suited for time series data where changes occur in a sequential manner.
Benefits:
- Trend Visualization: Line graphs excellently illustrate trends, making it easy to see increases or decreases over time.
- Continuity: They represent data points as a continuous flow, indicating how one point affects another.
Considerations:
- Clarity: If too many lines are plotted on the same graph, it may confuse the viewer.
- Data Density: Line graphs work best with linear data; non-linear relationships may need alternative representations.
Heat Maps
Heat maps provide a visual representation of data where individual values are depicted by color. This technique is beneficial when dealing with large data sets to highlight areas of higher or lower intensity of the data values.
Benefits:
- Quick Insight: Heat maps allow for rapid identification of hotspots or areas of interest.
- Complex Data Representation: They compress large data sets into a visually digestible format.
Considerations:
- Color Choices: Poor color selection can lead to misinterpretation.
- Context: Additional context is often necessary to understand what the colors represent.
Scatter Plots
Scatter plots display values for two variables for a set of data. They are particularly powerful in identifying correlations between variables and understanding the distribution of data points.
Benefits:
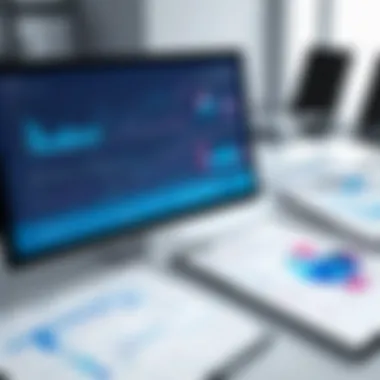
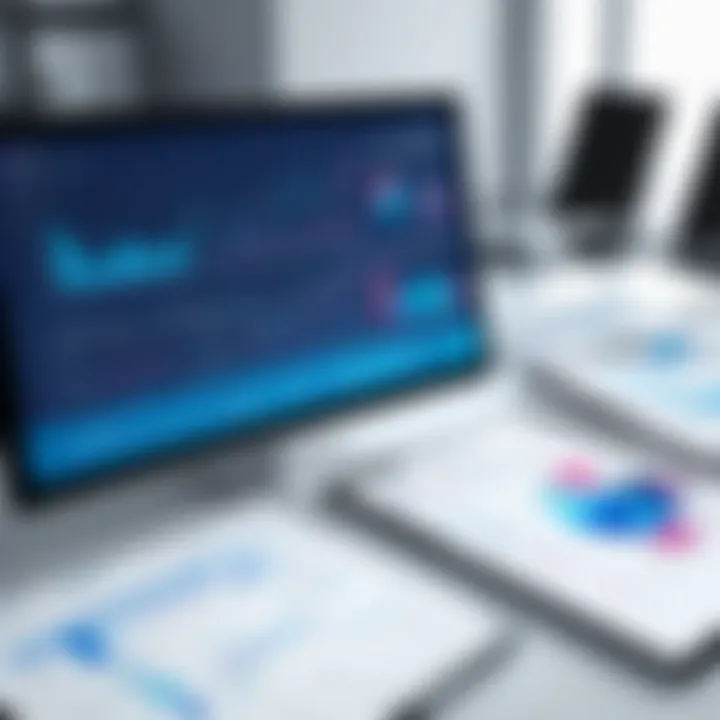
- Correlation Identification: They can highlight relationships and correlations between variables effectively.
- Outlier Detection: Scatter plots can easily reveal outliers in a dataset.
Considerations:
- Clarity: Overlapping points can obscure data analysis, especially with large datasets.
- Limited Context: Without additional context or axes labels, interpretation may be challenging.
In summary, each technique in graphical analytics offers specific advantages and challenges. The choice of method depends on the nature of the data and the insights one seeks to derive. Understanding these techniques enables professionals to present data in a manner that enhances comprehension and drives informed decision-making.
Tools and Technologies for Graphical Analytics
Tools and technologies play a critical role in graphical analytics. They enable data professionals to create visual representations that are informative and insightful. Using the right tools can significantly enhance the effectiveness of data visualization, allowing for better analysis and decision-making. Additionally, current software and technologies adapt to the growing demand for real-time data and complex datasets.
The importance of selecting suitable tools cannot be overstated. Proper tools not only facilitate efficient data processing but also help in conveying the intended message clearly. Therefore, understanding the options available and their functionalities is essential for any individual or organization utilizing graphical analytics. These technologies can improve data interpretation while optimizing the workflow.
Business Intelligence Software
Business intelligence software remains a cornerstone in the realm of graphical analytics. Software like Tableau, QlikView, and Microsoft Power BI offers robust functionalities for analyzing and visualizing data effectively. These tools allow businesses to harness their data to uncover insights that would be challenging to gather manually.
Features of business intelligence software include:
- User-friendly interfaces which make it easier to create complex visualizations.
- Integration with a variety of data sources, from databases to cloud services, ensuring flexibility.
- Drill-down capabilities that help in examining data at various levels.
The accessibility of these features empowers users, even those with limited technical expertise, to derive actionable insights. As a result, organizations can make informed decisions swiftly.
Open-source Visualization Tools
Open-source visualization tools provide an alternative to commercial software. These tools, such as D3.js and Plotly, offer flexibility and customization options that proprietary software often does not. They are accessible for modification, allowing users to adapt them to specific requirements.
Benefits of using open-source tools include:
- Cost-effectiveness, as many of these tools are free to use.
- A community-driven development model facilitates continuous improvement and updates based on user feedback.
- Extensive libraries and resources available for users to enhance their learning curve.
Open-source tools can be ideal for individuals or organizations wanting more control over their visual analytics without incurring high expenses.
Programming Libraries
Programming libraries are essential for advanced graphical analytics. Libraries such as Matplotlib, Seaborn, and ggplot2 allow data analysts and scientists to create sophisticated visualizations programmatically. Utilizing these libraries requires some programming knowledge, usually in Python or R, but the results can be immensely rewarding.
Key advantages of programming libraries include:
- The ability to automate the generation of graphics, which is particularly useful for handling large datasets.
- Customization options that enable users to create tailored visuals that meet specific analytical needs.
- High-quality output that can be used for publications and presentations.
Using programming libraries allows skilled users to leverage their coding knowledge to create complex visualizations that might not be achievable through standard software. In essence, they can transform raw data into compelling graphical representations that enhance understanding and communication.
In summary, mastering tools and technologies is crucial for anyone involved in graphical analytics. Choosing the right software, whether it be business intelligence, open-source solutions, or programming libraries, can optimize the data visualization process.
These tools not only aid in effective data interpretation but also provide deeper insights that are essential in today's data-driven world.
Case Studies: Successful Applications of Graphical Analytics
Case studies serve as effective tools to illustrate the real-world implications of graphical analytics. They not only provide concrete examples of how graphical representations of data can lead to significant insights but also show the practical benefits that various industries can derive from using these techniques. By examining these case studies, readers can better understand how organizations leverage graphical analytics to enhance decision-making, optimize operations, and improve overall performance. Through specific examples from different sectors, it becomes clear that graphical analytics is not just theoretical; it has a profound impact in practical applications.
Healthcare Sector
In the healthcare sector, graphical analytics is transforming how practitioners analyze data. Hospitals and clinics employ dashboards to visualize patient information and treatment outcomes. For instance, the use of heat maps helps to identify infection hotspots within a hospital. These visualizations enable healthcare professionals to quickly assess situations and take necessary actions promptly.
A notable case involved the implementation of graphical analytics at a major hospital to monitor patients’ vital signs. By integrating real-time data visualization techniques, clinicians managed to reduce response times for critical conditions significantly. The graphical representation of this data allowed medical teams to recognize trends and anomalies more quickly than traditional methods.
Additionally, healthcare providers can track improvements in patient care and treatment effectiveness through the use of line graphs. These visuals display changes in health metrics over time, which can be instrumental in refining protocols and improving patient education strategies. Overall, the application of graphical analytics in healthcare leads not only to enhanced patient outcomes but also fosters better operational efficiencies.
Finance Sector
The finance sector is another area where graphical analytics plays a crucial role. Financial institutions rely on visuals to analyze market trends, track investment performance, and manage risk. A prominent example is the use of scatter plots in portfolio management. These plots allow analysts to visualize risk versus return, aiding in the optimization of investment strategies.
A significant case study can be seen in a large investment firm that adopted advanced graphical analytics tools to visualize vast quantities of market data. They used bar charts to compare the performance of different assets within their portfolio. This led not only to improved investment decisions but also enhanced communication among stakeholders, as the visuals facilitated clearer presentations of complex data.
Moreover, financial analysts use dashboards to monitor key performance indicators such as revenue growth and expense ratios. This integration of graphical analytics into their daily workflows promotes timely responses to market changes and contributes towards achieving strategic financial goals.
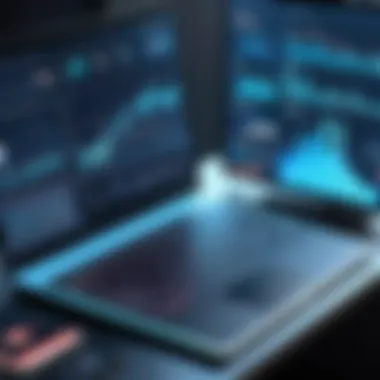
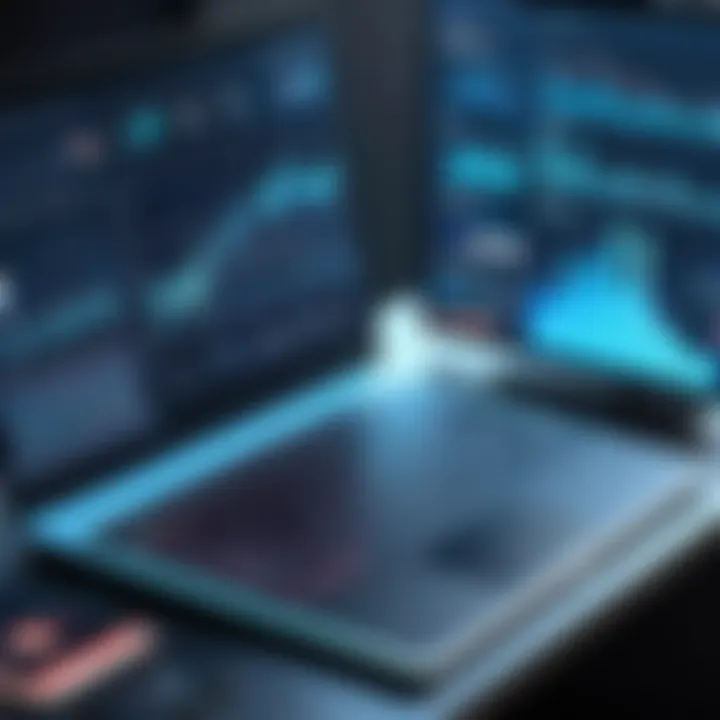
Marketing Industry
In the marketing industry, graphical analytics is essential for understanding consumer behavior and market dynamics. Companies utilize various visualization tools to analyze digital marketing metrics, such as website traffic and social media engagement. For example, pie charts are often employed to display the share of different traffic sources, making it easier for marketers to assess the effectiveness of their campaigns.
One successful case study highlighted a campaign by a leading brand that implemented graphical analytics to track customer engagement across multiple platforms. Utilizing line graphs, the marketing team was able to visualize trends in customer interactions over time, leading to deeper insights into customer preferences. This analysis resulted in the creation of more targeted marketing strategies that significantly improved conversion rates.
Additionally, heat maps are widely used to visualize consumer demographics and engagement patterns. These visualizations allow marketers to identify which segments of their audience are most engaged, allowing for tailored promotional efforts. In this way, graphical analytics helps businesses make informed decisions that ultimately drive growth and enhance customer satisfaction.
Challenges in Graphical Analytics
In the evolving landscape of graphical analytics, several challenges can impede effective data visualization and interpretation. Addressing these challenges is crucial for maximizing the insights derived from graphical representations. This section details two major obstacles that practitioners often face: data overload and misinterpretation of visuals. Understanding these challenges can enhance the clarity and utility of graphical analytics, therefore providing significant benefits in decision-making.
Data Overload
Data overload occurs when too much information is presented in a graphical format. It can lead to confusion instead of clarity. With vast amounts of data available, there's a temptation to include excessive details in visual analyses. This not only complicates the visual but also overwhelms the audience.
Several factors contribute to data overload:
- Complexity of Data: When datasets are intricate, displaying all variables can clutter visuals. This negatively impacts their readability.
- Inconsistent Formatting: Different formats used for data presentation can confuse viewers. Uniformity in style aids comprehension.
- Unclear Objectives: If the purpose of the visualization is not defined clearly, it may lead to extraneous information being included.
To combat data overload, it is important to prioritize information. Focus on key messages and use simplified visuals. Techniques such as filtering data and creating interactive visuals can help in managing the volume of information displayed. Effectively managing data overload ensures that the audience retains necessary insights without being overwhelmed.
Misinterpretation of Visuals
Another significant challenge in graphical analytics is the misinterpretation of visuals. Different viewers may perceive the same graphic in divergent ways. Factors leading to misinterpretation may include:
- Ambiguity in Design: Poorly designed graphics can lead to different interpretations. Clarity in representation is critical to prevent misunderstandings.
- Lack of Context: If viewers are unaware of the underlying data or the story behind the visual, misinterpretations can arise. Providing context is essential for proper understanding.
- Cognitive Bias: Viewers' personal biases can skew their interpretation of data. Engaging with visuals critically is required to draw accurate conclusions.
To mitigate misinterpretation, it is essential to employ best practices in design and presentation. Utilize clear labeling, avoid misleading scales, and provide descriptions or annotations when necessary. Training audience members on how to read and interpret visuals can also help reduce misinterpretation.
"In graphical analytics, effective communication hinges on clarity and accessibility of visuals to ensure accurate data interpretation."
In summary, addressing challenges such as data overload and misinterpretation of visuals is fundamental to the success of graphical analytics. By focusing on clarity, simplicity, and user understanding, we can harness the true power of graphical representation in data analysis.
Future Trends in Graphical Analytics
The landscape of graphical analytics is rapidly evolving. By anticipating shifts in this field, professionals can harness new tools and techniques to gain better insights from their data. Understanding future trends is essential as it shapes the methodology and applications of graphical analytics in various sectors. Key elements include the integration of advanced technologies such as artificial intelligence and the demand for real-time data visualization. These trends not only bring benefits but also present considerations that professionals must navigate for successful implementation.
Artificial Intelligence Integration
Artificial intelligence is reshaping the domain of graphical analytics. Its ability to analyze vast amounts of data and predict trends is invaluable. AI can enhance data interpretation by identifying patterns that may not be immediately visible to humans. For instance, machine learning algorithms can automatically generate visualizations based on the data's context and complexity. This reduces the burden on analysts and increases the speed of decision-making. However, there are challenges. The reliance on AI raises concerns regarding data bias and accuracy. Decision-makers must ensure that the training data is representative to avoid misleading results.
Real-time Data Visualization
In today's fast-paced world, the ability to visualize data in real-time is crucial. Businesses need immediate access to insights to adapt quickly to market changes. Real-time data visualization allows professionals to monitor trends as they occur, fostering timely reactions to emerging patterns. Technologies such as streaming data architecture and dashboards equipped with live updates are becoming standard tools.
The importance of this capability cannot be overstated:
- It enhances situational awareness.
- It supports proactive decision-making.
- It enables organizations to stay competitive.
However, implementing real-time visualization comes with its own set of challenges. Organizations must invest in infrastructure that can handle high data volumes without lagging. The need for data accuracy in real-time is paramount; any delay or error can lead to significant consequences. Thus, while embracing this trend, companies should also focus on optimizing their systems for efficiency.
"The future of graphical analytics lies in how well we integrate intelligent systems and real-time capabilities into our workflows."
These future trends in graphical analytics signify a shift towards more dynamic, interactive data exploration, paving the way for improved decision-making across industries.
Closure and Key Takeaways
In the realm of graphical analytics, the conclusion synthesizes the essential elements discussed in this article. It is vital to recognize how graphical analytics not only enhances data interpretation but also facilitates informed decision-making. As organizations increasingly rely on data-driven methodologies, understanding this field becomes crucial. By implementing graphical analytics effectively, one can unlock insights that impact strategy and operational efficiency.
Summary of Insights
Throughout this article, we have explored several key facets of graphical analytics:
- Definition and Scope: We established what graphical analytics entails and its various applications across industries.
- Importance of Data Visualization: The role of visual aids in improving understanding and decision-making processes was highlighted.
- Key Principles: Strong emphasis was placed on data integrity, appropriate use of visuals, and the need for scalability.
- Common Techniques: Techniques such as bar charts, line graphs, heat maps, and scatter plots were discussed as essential tools.
- Tools and Technologies: We examined both software and programming libraries that are commonly used in graphical analytics.
- Case Studies: Real-world applications in sectors like healthcare and finance illustrated the practical benefits of graphical analytics.
- Challenges: We addressed potential pitfalls like data overload and misinterpretation, showcasing the need for careful implementation.
- Future Trends: The integration of artificial intelligence and real-time data visualization points to exciting advancements on the horizon.
Final Thoughts on Graphical Analytics
Graphical analytics is a constantly evolving field. As data volumes increase, the need for effective visualization will only intensify. Organizations must remain adaptive, embracing new technologies and methods to stay ahead. A balanced approach, focusing on data accuracy and interpretation, will help mitigate challenges associated with graphical analytics.
In closing, investing time in mastering graphical analytics will empower professionals across sectors to make data-driven decisions. Understanding trends, techniques, and applications in this area can thus be a significant asset for IT professionals, cybersecurity experts, and students eager to delve into data-centric careers.
"Data visualization is not just about making data look good, it is about telling a story that leads to actionable insights."
Ultimately, embracing the principles of graphical analytics equips individuals and organizations to navigate data landscapes more effectively.