Mastering Data Cleaning with Alteryx: Advanced Techniques Revealed
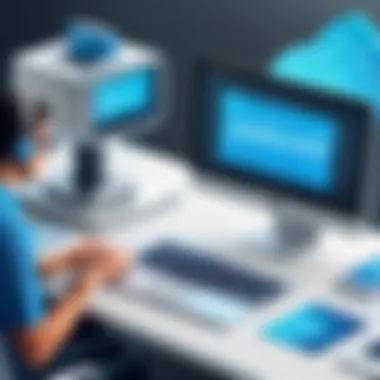
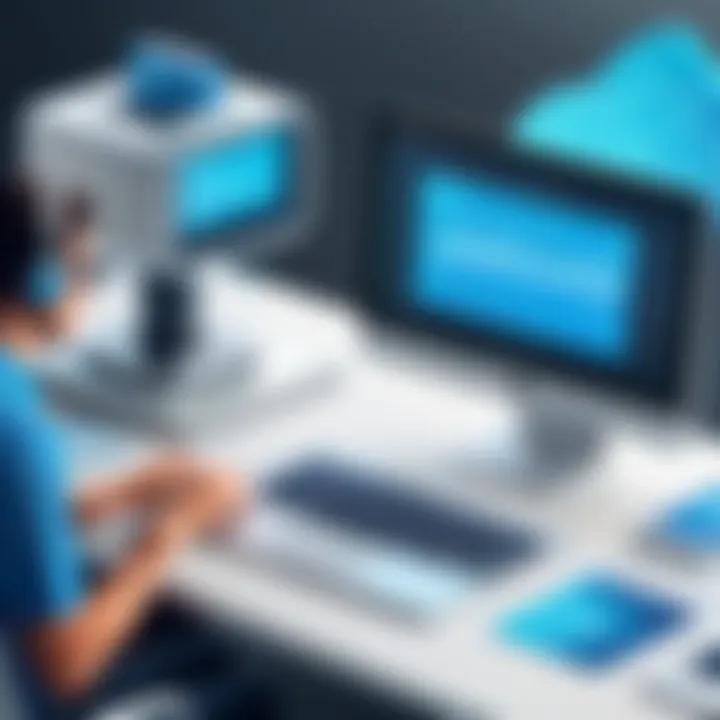
Understanding Storage, Security, or Networking Concepts
Data cleaning is a fundamental aspect of any data analysis process. It involves detecting and correcting errors, inconsistencies, and inaccuracies within datasets to ensure reliable insights. When using Alteryx for data cleaning, understanding the basics is crucial. Alteryx offers a user-friendly interface that allows IT professionals and students to perform various data cleansing tasks efficiently. Key terminology such as deduplication, normalization, and outlier detection are central to mastering data cleaning with Alteryx. Having an in-depth grasp of these concepts is imperative to streamline the data preparation workflow.
Best Practices and Tips for Data Cleaning with Alteryx
Optimizing data cleaning processes with Alteryx requires adherence to best practices and strategic tips. To enhance storage capabilities, consider implementing workflows that facilitate structured data storage and retrieval. Applying security best practices such as data encryption and access control within Alteryx workflows can safeguard sensitive information during the cleaning process. Networking strategies focusing on optimizing data transfer speeds and connectivity can improve the overall performance of Alteryx for data cleaning.
Industry Trends and Updates in Data Cleaning
Amid the evolving landscape of data cleaning technologies, staying abreast of the latest trends becomes paramount for IT professionals and cybersecurity experts. Cybersecurity threats continue to pose challenges in data cleaning processes, emphasizing the importance of robust security measures. Networking innovations, such as cloud-based solutions for distributed data cleaning, are gaining momentum in the industry. Understanding these trends equips practitioners with the knowledge needed to adapt their data cleaning strategies effectively.
Case Studies and Success Stories of Alteryx Implementation
Real-life examples serve as valuable insights into the successful application of Alteryx for data cleaning. Case studies showcasing how organizations have leveraged Alteryx to optimize their data cleansing workflows highlight the platform's effectiveness. Examining cybersecurity incidents and lessons learned from using Alteryx can provide essential guidance on implementing secure data cleaning practices. Networking case studies demonstrating the efficiency gains derived from Alteryx integration offer inspiration for streamlining data cleaning operations.
Reviews and Comparison of Data Cleaning Tools
In-depth evaluations of data cleaning software and tools tailored for Alteryx users yield essential insights for making informed decisions. Comparing cybersecurity tools compatible with Alteryx enables professionals to identify solutions that best align with their security requirements. Evaluating networking equipment and services that complement Alteryx workflows is crucial for optimizing data processing and communication. Accessing comprehensive reviews and comparisons empowers users to select the most suitable tools and products for their data cleaning endeavors.
Introduction to Alteryx
In the vast landscape of data analytics, understanding the fundamentals of Alteryx is akin to discovering a rare gem amidst a sea of stones. Alteryx stands out as a multifaceted tool that serves as a beacon of efficiency in the realm of data cleaning. This section serves as a gateway to unraveling the intricate tapestry of Alteryx, shedding light on its essential role in optimizing data preparation processes for enhanced analytical workflows. Exploring Alteryx not only equips professionals in the IT and cybersecurity domains with a robust tool but also empowers students to delve into the nuances of data cleansing with unparalleled precision and effectiveness.
Overview of Alteryx Software
Delving deeper into the labyrinth of Alteryx software reveals a plethora of features that redefine the landscape of data cleansing. The Features of Alteryx section unveils a treasure trove of functionalities that streamline the data cleaning process, making it a popular choice among data enthusiasts. One striking characteristic of Alteryx is its seamless integration of diverse data sources, simplifying the aggregation and processing of information. While its user-friendly interface enhances accessibility, its powerful data parsing capabilities set it apart as a versatile solution for intricate data cleaning tasks.
Benefits of Using Alteryx for Data Cleaning
Unleashing the full potential of Alteryx for data cleaning unveils a myriad of advantages that underpin its significance in this article. The Benefits of Using Alteryx for Data Cleaning segment illuminates the transformative impact of Alteryx on data preparation tasks and analytical workflows. One notable benefit lies in its robust data standardization features, ensuring consistency and accuracy in datasets. Additionally, Alteryx's efficiency in handling massive datasets accelerates the data cleaning process, fostering agility in decision-making processes.
Importance of Data Cleaning
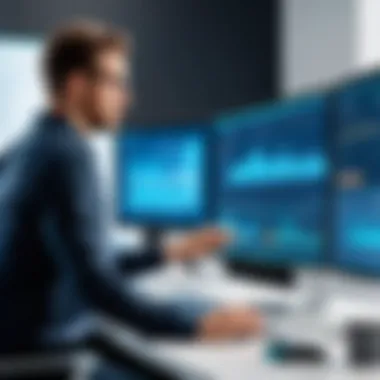
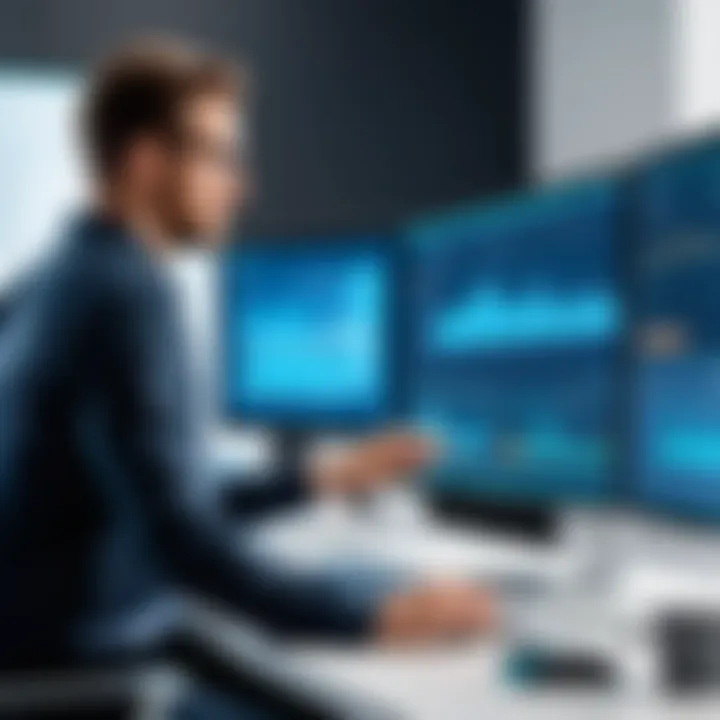
As the cornerstone of analytics, data cleaning plays a pivotal role in deciphering the complexities of data-driven insights. The Role of Data Cleaning in Analytics section delves into the crux of this importance, highlighting how meticulous data cleaning lays the foundation for robust analytical models. Recognizing the significance of clean data, Alteryx emerges as a beacon of reliability in ensuring data integrity and accuracy for informed decision-making processes.
Impact of Clean Data on Decision-Making
In the realm of decision-making, the impact of clean data transcends mere numbers to shape strategic outcomes. The Impact of Clean Data on Decision-Making elucidates how impeccable data quality paves the way for trustworthy insights, empowering decision-makers with reliable information. By leveraging Alteryx for data cleaning, professionals witness a paradigm shift in their ability to make informed decisions backed by clean, reliable data.
Key Steps in Data Cleaning with Alteryx
In this article, we delve deep into the crucial topic of Key Steps in Data Cleaning with Alteryx, shedding light on its significance in enhancing data processing efficiency and accuracy. Data cleaning is the cornerstone of any analytical process, ensuring that the data utilized for decision-making is accurate, reliable, and consistent. In the realm of Alteryx, mastering the key steps in data cleaning can significantly impact the quality of insights derived from the data.
Data Preparation
Data Profiling
Data Profiling plays a fundamental role in the data cleaning process with Alteryx. It involves analyzing the structure and quality of data sets to identify anomalies, patterns, and inconsistencies. By conducting thorough data profiling, analysts can gain a clear understanding of the data's characteristics, distribution, and quality, enabling them to make informed decisions regarding data cleaning strategies. One of the key characteristics of Data Profiling is its ability to highlight data outliers and anomalies effectively, allowing analysts to address data quality issues proactively. Despite its benefits, Data Profiling may require significant computational resources and time, especially when dealing with large datasets.
Data Parsing
Data Parsing is a critical aspect of data preparation in Alteryx, focusing on extracting structured information from unstructured data sources. This process involves breaking down complex data fields into meaningful components, such as separating names into first and last names or extracting dates from text strings. The key characteristic of Data Parsing lies in its ability to enhance data consistency and accessibility by converting unstructured data into a more organized format. While Data Parsing streamlines the data processing workflow, it may introduce complexities related to data integrity and compatibility with downstream analytical tools.
Data Quality Assessment
Identifying Missing Values
The identification of missing values is paramount in ensuring data cleanliness and accuracy within Alteryx workflows. Identifying and addressing missing data points prevent skewed analysis and inaccurate insights by maintaining data completeness. Alteryx provides robust tools for detecting and handling missing values, enhancing the overall data quality assessment process. A key characteristic of identifying missing values is its ability to differentiate genuine data gaps from data corruption or errors, enabling analysts to make informed decisions regarding data imputation strategies.
Handling Duplicates
Handling duplicates is another essential aspect of data quality assessment with Alteryx, focusing on identifying and removing redundant entries within datasets. By addressing duplicate records, analysts can avoid erroneous calculations and misleading analysis outcomes caused by redundant data points. The key characteristic of handling duplicates lies in its capacity to streamline data storage and improve computational efficiency by reducing data redundancy. However, managing duplicates effectively may pose challenges in scenarios where duplicate criteria are subjective or poorly defined.
Data Transformation
Standardizing Formats
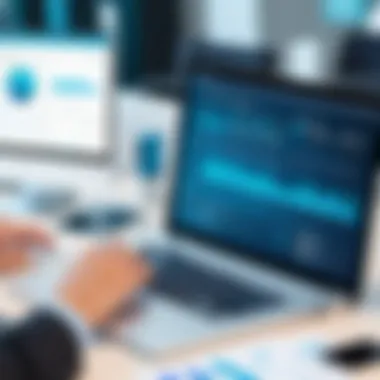
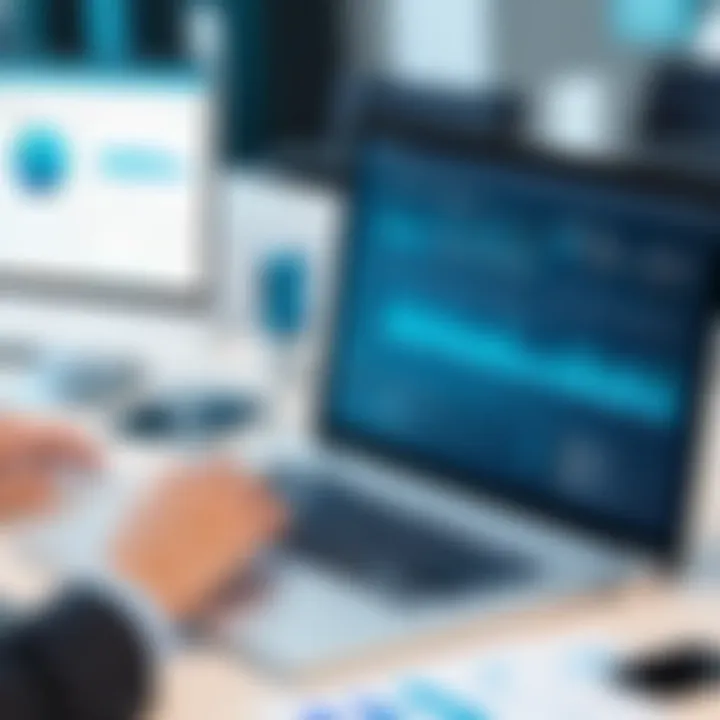
Standardizing formats is a pivotal step in data transformation with Alteryx, emphasizing the uniformity of data structures and attributes. By standardizing data formats, analysts can ensure data consistency and compatibility across different systems and analytical tools. The key characteristic of standardizing formats is its role in enhancing data integration and facilitating seamless data exchange between disparate sources. While standardizing formats simplifies data processing, it may require meticulous attention to detail and robust data mapping strategies to prevent information loss or format conflicts.
Normalizing Data
Data normalization is essential in data transformation within Alteryx, aiming to minimize redundancy and optimize data storage efficiency. Normalizing data involves structuring data fields to reduce duplication and improve database performance. The key characteristic of normalizing data is its ability to enhance data integrity and simplify query operations by organizing data into manageable and logical units. Despite its advantages, data normalization may introduce complexities in data retrieval and modification, especially in scenarios involving frequent data updates or complex relational databases.
Advanced Techniques in Alteryx Data Cleaning:
In this article, the discussion on Advanced Techniques in Alteryx Data Cleaning assumes immense significance. These advanced methods play a pivotal role in elevating data cleaning processes to a superior level of efficiency and effectiveness. By exploring predictive analytics and text analytics within Alteryx, users can harness more profound insights from their datasets, paving the way for more informed decision-making and enhanced analytical outcomes. The incorporation of advanced techniques not only accelerates the data cleaning process but also enhances the overall quality and accuracy of the data being analyzed. Readers will benefit from understanding the practical application and implications of these sophisticated approaches in the realm of data cleaning.
Predictive Analytics:
Building Predictive Models:
Delving into Building Predictive Models signifies a critical aspect of predictive analytics within Alteryx. This facet of data analysis involves constructing models that anticipate future trends or behaviors based on existing data patterns. The essence of Building Predictive Models lies in its ability to forecast potential outcomes with a notable degree of accuracy, guiding organizations in making data-driven decisions. The unique attribute of these models is their capacity to uncover hidden insights within datasets, enabling users to proactively address challenges and leverage opportunities. While Building Predictive Models offers significant advantages in enhancing predictive capabilities, it also demands a meticulous approach to data processing and model validation.
Data Imputation:
The focus on Data Imputation within the domain of Alteryx's predictive analytics is pivotal for ensuring data completeness and accuracy. Data Imputation involves the process of filling in missing values within a dataset, enhancing the data's integrity and reliability for subsequent analysis. This aspect of data cleaning carries considerable weight in mitigating the impact of data gaps and inconsistencies on analytical outcomes. The distinctive feature of Data Imputation lies in its capacity to optimize data resources by providing a comprehensive and coherent dataset for predictive modeling. However, while Data Imputation facilitates data enhancement, it also necessitates cautious consideration of imputation techniques and potential biases that may arise.
Text Analytics:
Sentiment Analysis:
Exploring Sentiment Analysis within the realm of text analytics utilizing Alteryx presents a valuable opportunity for understanding textual data sentiment. This analytical method focuses on discerning emotions and opinions expressed in text, offering insights into the subjective aspects of data. Sentiment Analysis serves as a robust tool for gauging customer feedback, market trends, and brand perception, aiding organizations in refining their strategies and enhancing customer experiences. The pivotal attribute of Sentiment Analysis is its capacity to distill complex textual information into quantifiable metrics, facilitating data-driven decision-making processes. While offering valuable sentiment insights, this analysis method requires attention to contextual nuances and sentiment classification challenges.
Keyword Extraction:
The integration of Keyword Extraction into text analytics workflows in Alteryx is instrumental for extracting meaningful terms and phrases from textual data. This process involves identifying and highlighting keywords that encapsulate the essence of the text, enabling swift information retrieval and content categorization. Keyword Extraction serves as a fundamental aspect of text mining, empowering users to distill crucial information from vast textual sources efficiently. The key feature of Keyword Extraction is its ability to condense extensive textual content into concise keyword sets, streamlining data interpretation and analysis. However, while facilitating data abstraction, the accuracy and relevance of extracted keywords warrant careful scrutiny to ensure informational integrity.
Optimizing Data Cleaning Workflows
Optimizing Data Cleaning Workflows plays a vital role in this exhaustive study on harnessing the capabilities of Alteryx for data cleaning. In the realm of data preparation and enhancement of analytical pipelines, optimizing workflows stands as a cornerstone for efficiency. By fine-tuning the data cleaning process, organizations can save precious time and resources, leading to streamlined operations and improved decision-making. The key elements of optimizing data cleaning workflows revolve around creating efficiencies, reducing redundancy, and maximizing output quality. Through a meticulous approach to workflow optimization, businesses can ensure data integrity, enhance performance, and derive actionable insights from their datasets.
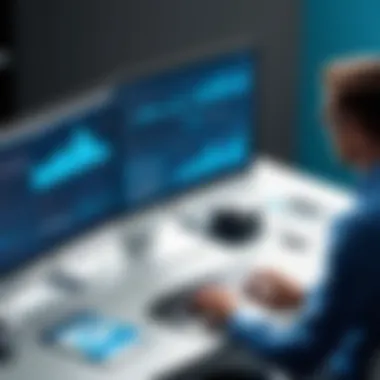
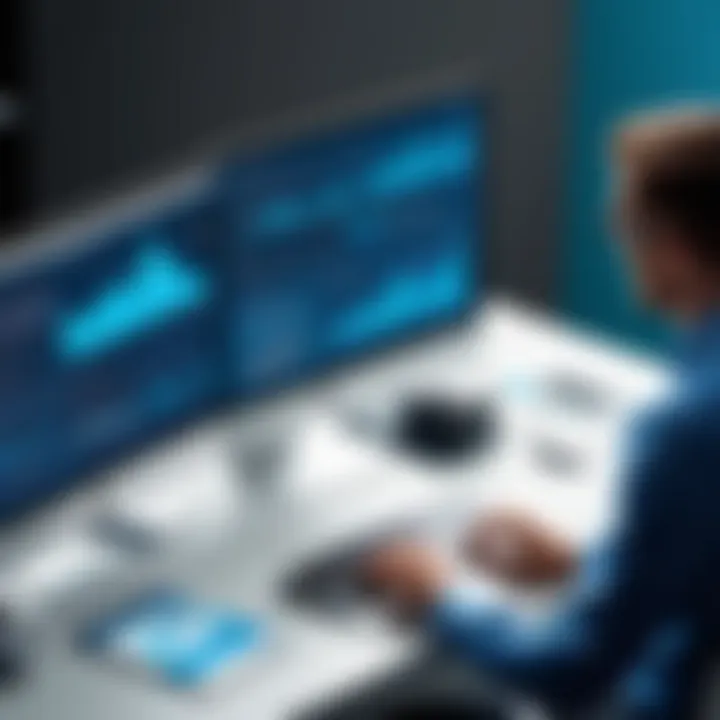
Automation
Creating Batch Macros
Delving into the specifics of creating batch macros within the context of this article, it is pivotal to grasp the significance of automation in expediting data cleaning workflows. Creating batch macros allows for the automation of repetitive tasks, enabling streamlined processing of large datasets with minimal manual intervention. The key characteristic of batch macros lies in their ability to execute a series of actions automatically, facilitating the handling of complex data cleaning operations with ease. The unique feature of batch macros is their scalability, making them a popular choice for optimizing data cleaning workflows in this article. While batch macros enhance efficiency and reduce manual effort, their limitation may lie in the complexity of setup and maintenance, requiring skilled individuals to create and manage them effectively.
Scheduling Workflows
Turning our attention to the realm of scheduling workflows, we encounter another essential aspect of automating data cleaning processes. Scheduling workflows enable the timely execution of data cleaning tasks, ensuring that operations run smoothly and consistently without manual intervention. The key characteristic of scheduled workflows is their ability to automate recurring processes, guaranteeing data cleaning tasks are performed at predefined intervals without fail. This facet makes scheduling workflows a valuable choice for this article, as it promotes consistency and adherence to data cleaning timelines. The unique feature of scheduling workflows is their flexibility in setting up custom schedules and triggering actions based on specific criteria. While scheduling workflows enhance productivity and operational efficiency, their disadvantage may lie in potential conflicts with overlapping tasks and resource constraints.
Performance Tuning
Workflow Optimization Tips
When it comes to performance tuning in the context of this article, delving into workflow optimization tips is key to enhancing data cleaning efficiency. Workflow optimization tips encompass strategies and best practices aimed at improving the speed, accuracy, and reliability of data cleaning processes. The key characteristic of workflow optimization tips is their focus on identifying bottlenecks, streamlining workflows, and improving overall data processing efficiency. This focus makes workflow optimization tips a beneficial choice for optimizing data cleaning workflows, as they enable organizations to enhance data quality and accelerate decision-making processes. The unique feature of workflow optimization tips is their adaptability to different data cleaning scenarios, providing tailored recommendations for optimizing specific workflows. While workflow optimization tips offer valuable insights and recommendations, their implementation may require expertise and experimentation to achieve optimal results.
Caching and Parallel Processing
In the realm of performance tuning, delving into the benefits of caching and parallel processing sheds light on advanced techniques for optimizing data cleaning workflows. Caching and parallel processing work in tandem to enhance data processing speeds and resource utilization, particularly when dealing with large datasets. The key characteristic of caching and parallel processing is their ability to distribute workloads efficiently across multiple resources, reducing processing times and improving overall system performance. This efficiency makes caching and parallel processing a popular choice for organizations seeking to optimize data cleaning workflows in this article. The unique feature of caching and parallel processing is their capacity to handle complex data transformations and analytical tasks in parallel, maximizing computational efficiency. While caching and parallel processing offer significant performance gains, their implementation complexities and resource requirements may pose challenges for organizations without substantial computational resources.
Challenges and Best Practices
Dealing with Big Data
Scalability Issues
Scalability plays a critical role in the realm of data processing, particularly in the context of Big Data. Within the scope of Alteryx for data cleaning, scalability issues refer to the capacity to manage and process vast amounts of data without compromising performance. The key characteristic of scalability in Alteryx is its ability to seamlessly handle growing datasets and increased computational demands. Scalability issues are highly beneficial for large-scale data projects, as they enable seamless expansion and resource allocation, ensuring uninterrupted operations. However, challenges such as maintaining data consistency and integrity across various scaling levels must be carefully managed to maximize the benefits of scalability in data cleaning processes.
Distributed Processing
Distributed processing is a key strategy employed in overcoming data processing bottlenecks and enhancing performance. In the context of Alteryx, distributed processing involves distributing data processing tasks across multiple nodes or machines to expedite computational speed and efficiency. The unique feature of distributed processing lies in its ability to parallelize data operations, enabling quicker processing times and optimized resource utilization. While distributed processing offers significant advantages in terms of speed and scalability, it may introduce complexities related to data synchronization and communication overhead. Therefore, meticulous planning and implementation are necessary to leverage the advantages of distributed processing effectively within Alteryx data cleaning workflows.
Ensuring Data Integrity
Data Validation Techniques
Data validation techniques play a crucial role in maintaining data integrity throughout the data cleaning process. These techniques involve verifying and validating data to ensure consistency, accuracy, and reliability. In the context of Alteryx, utilizing robust data validation techniques helps in identifying inaccuracies, inconsistencies, and anomalies within datasets, thus enhancing data quality and reliability. The key characteristic of data validation techniques is their capability to automate error detection and ensure data conformity to predefined standards. While data validation techniques contribute to improved data quality and decision-making, they require careful configuration and monitoring to avoid false positives or negatives that could impact the overall data integrity.
Error Handling Strategies
Effective error handling strategies are essential components of data cleaning practices to mitigate risks and maintain data quality. In Alteryx, error handling strategies involve defining protocols to manage and resolve errors, exceptions, and inconsistencies that may arise during data processing. The key characteristic of error handling strategies is their ability to identify, log, and rectify errors proactively, minimizing disruptions to data workflows and analysis. By implementing robust error handling mechanisms, organizations can ensure data consistency and reliability, fostering trust in analytical outcomes and business decisions. While error handling strategies enhance data integrity and operational efficiency, their successful implementation requires continuous monitoring and refinement to adapt to evolving data landscapes.